My Conception of a Swarm of Agents. Courtesy of Cocoa Yeo (2008).
what's new
ICML 2021 Expert Reviewer ICML 2020 Top 33% Reviewer ICML 2019 Top 5% Reviewer
|
|
Invited to serve as a World Economic Forum’s Global Future Councils Fellow for the Council on the Future of Artificial Intelligence and Robotics, Sep 2016 - Jun 2018
|
|
Keynote speaker at 20th IEEE/WIC/ACM International Conference on Web Intelligence and Intelligent Agent Technology, 14-17 Dec 2021
|
|
Organizing Co-Chair for NeurIPS 2021 Workshop on Closing the Gap between Academia and Industry in Federated Learning: Challenges on Privacy, Fairness, Robustness, Personalization and Data Ownership
|
|
Keynote speaker at 2nd
International Symposium on Multi-Robot and Multi-Agent Systems, 22-23 Aug 2019
|
|
IEEE RAS Distinguished Lecturer for the
IEEE RAS Technical Committee on Multi-Robot Systems, Mar 2019
|
|
Recipient of Faculty Teaching Excellence Award
Aug 2017 - Jul 2018
|
|
Our 6 NeurIPS 2023, 3 ICML 2023, 3 ICLR 2023, 2 AISTATS 2023, AAAI 2023, 1 MLJ, 1 AIJ submissions are accepted!
|
|
AI Singapore Research Programme : Toward Trustable Model-centric Sharing for Collaborative Machine Learning,
S$8,401,002.40, Apr 2021 - Mar 2025
|
|
RIE2020 AME Programmatic Fund : Learning with Less Data,
SGD $1,218,600, Apr 2021 - Mar 2024
|
|
DSTA Project Agreement : Tactics Discovery and Recommendation,
SGD $1,143,120, Jul 2021 - Aug 2023
|
|
MOE AcRF Tier 1 Reimagine Research Scheme Funding : Scalable AI Phenome Platform towards Fast-Forward Plant Breeding (Machine Learning)
SGD $348,600, Mar 2021 - Mar 2024
|
|
RIE2020 AME IAF-PP :
High Performance Precision Agriculture (HiPPA) System, SGD $1,197,960, Mar 2020 - Feb 2024
|
|
Invited to serve as
area chair of ICML 2024, ICLR 2023, 2024, AISTATS 2023, 2024, ECAI 2023, AAAI 2022, 2024, RSS 2022, CoRL 2020, associate editors of IROS 2012, 2020-2023 & ICRA 2011, 2020-2023, IEEE RA-L
senior program committee members of AAAI 2019, AAMAS 2018-2019, 2023, IJCAI 2015, 2020-2022, 2024, ECAI 2020, ICRA 2022
program committee members of AAAI 2010, 2016-2018, 2020-2021, 2023, IJCAI 2011, 2015, 2017, 2019, UAI 2021-2023 AAMAS 2011-2014, 2016, 2021-2022, RSS 2014, 2018, 2020,
CVPR 2021,
ICAPS 2010-2012, 2018-2019, 2021-2022, and
reviewer of NeurIPS 2013-2016, 2018-2023, ICML 2019-2022, AISTATS 2019-2022, ICLR 2019-2021
|
|
Students interested to join MapleCG, click here for more info
|
|
members
I am looking for talented undergraduate and graduate students in NUS to join my MapleCG research group.
If you are really excited and motivated to be involved in novel research in the fields of
artificial intelligence, planning under uncertainty (i.e., decision-theoretic, information-theoretic),
robotics, multi-agent systems (i.e., multi-agent coordination, planning, and learning), game theory,
statistical machine learning, optimization, and/or swarm intelligence, please email me and we can set
up a time for discussion. Please also take some time to view our research projects.
I am currently advising the following students and research staff:
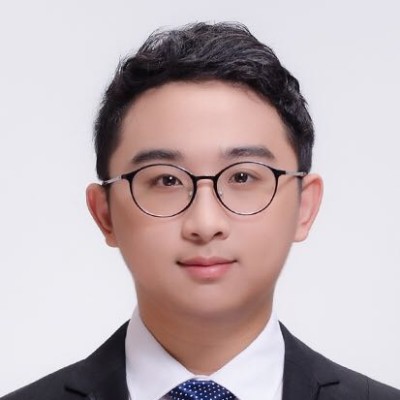 |
Zhao, Zitong
Ph.D.
B.Sc. in Computer Science and B.Sc. in Data Science > University of Michigan -- Ann Arbor
Research Interests: large language models
|
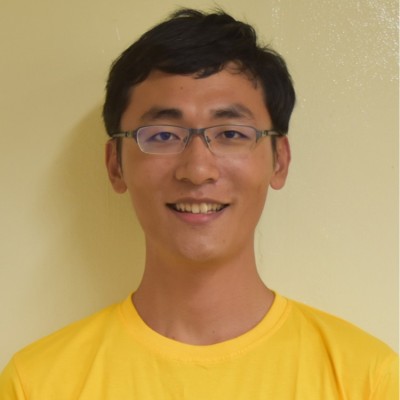 |
Niu, Xinyuan
Ph.D. (Co-advised with Chuan-Sheng Foo, A*STAR)
Recipient of A*STAR Graduate Scholarship (AGS)
B.Eng. in Mechanical Engineering (Hons. 1st Class) with Second Major in Innovation & Design and Minor in Computer Science > National University of Singapore
M.Eng. in Mechanical Engineering > National University of Singapore
Research Interests: robotics
|
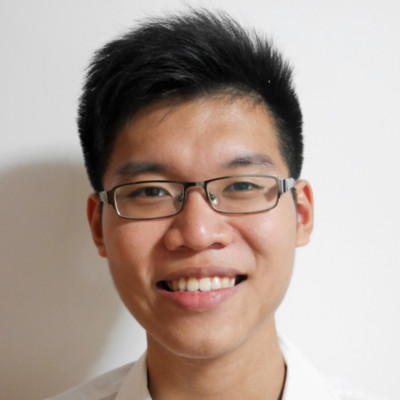 |
Chen, Zhi Liang
Ph.D. (Co-advised with Chuan-Sheng Foo, A*STAR)
Recipient of A*STAR Computing and Information Science Scholarship (ACIS)
B.Sc. in Computer Science (Hons. 1st Class) and B.Sc. in Applied Mathematics (Hons. 1st Class) > National University of Singapore
Research Interests: automated machine learning
|
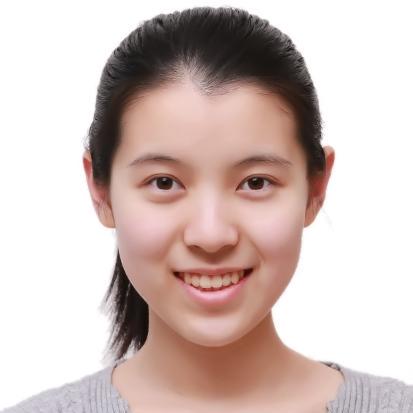 |
Wang, Jingtan
Ph.D. (Co-advised with Chuan-Sheng Foo, A*STAR)
Recipient of A*STAR Computing and Information Science Scholarship (ACIS)
B.Eng. in Computer Science with Minor in Mathematics > Nanyang Technological University
Research Interests: large language models
|
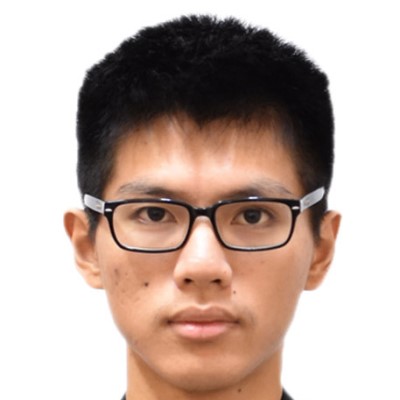 |
Chen, Jiangwei
Ph.D. (Co-advised with Chuan-Sheng Foo, A*STAR)
Recipient of A*STAR Computing and Information Science Scholarship (ACIS)
B.Sc. in Computer Science (Hons. 1st Class) with Major in Mathematics > National University of Singapore
Research Interests: collaborative machine learning, machine unlearning
|
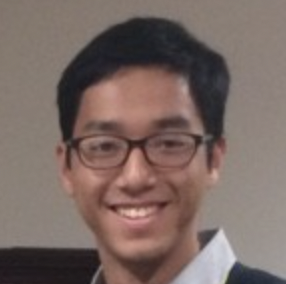 |
Apivich Hemachandra
Ph.D. (Co-advised with See-Kiong Ng)
Recipient of NUS Research Achievement Award and Teaching Fellowship Scheme
B.Sc. in Physics with Minors in Computer Science and Mathematics > Mahidol University International College
Research Interests: deep active learning
|
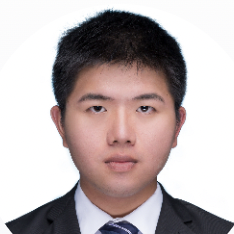 |
Lin, Xiaoqiang
Ph.D. (Co-advised with See-Kiong Ng)
Recipient of NUS Research Achievement Award and Teaching Fellowship Scheme
B.Sc. in Statistics (Data Science & Technology) > Fudan University
Research Interests: incentives in collaborative machine learning and federated learning, data valuation
|
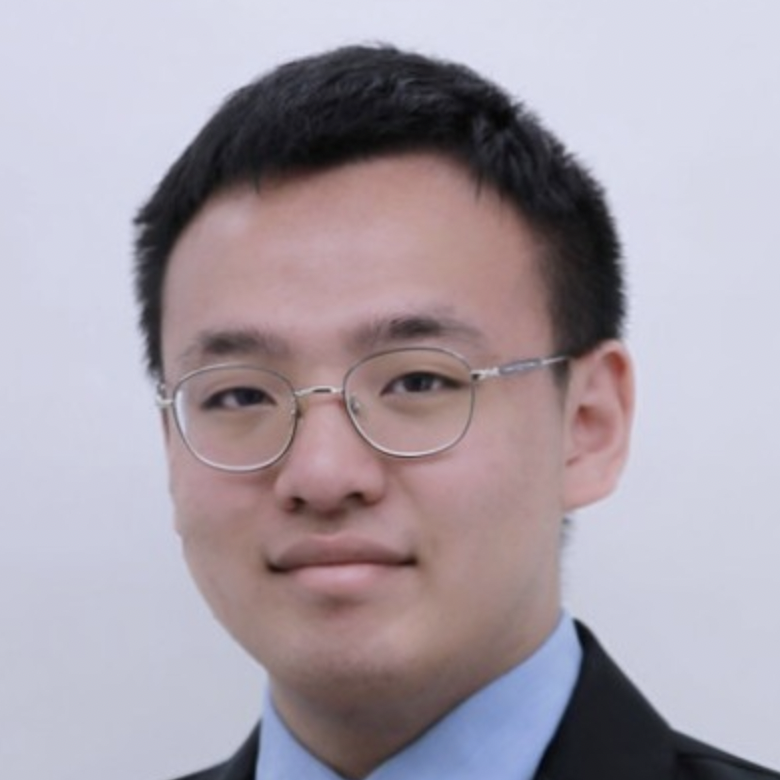 |
He, Zhenfeng
Ph.D.
B.Sc. in Computer Science (Hons. 1st Class) > National University of Singapore
Research Interests: automated machine learning
|
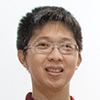 |
Sng, Weicong
Ph.D.
Recipient of Graduate Tutorship
B.Sc. in Statistics (Hons. 2nd Upper) > National University of Singapore
M.Comp. in Computer Science > National University of Singapore
Research Interests: reinforcement learning
|
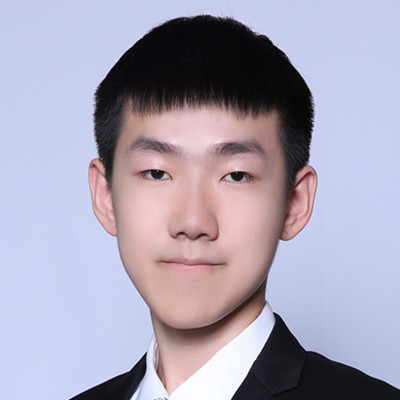 |
Qiao, Rui
Ph.D.
Recipient of NUS Research Achievement Award, AI Singapore Ph.D. Fellowship, and Keppel Award of Excellence for the Top 2 students in B.Eng. in Information Systems Technology and Design (ISTD) pillar in Singapore University of Technology and Design
B.Eng. in Information Systems Technology and Design > Singapore University of Technology and Design
Research Interests: causal inference
|
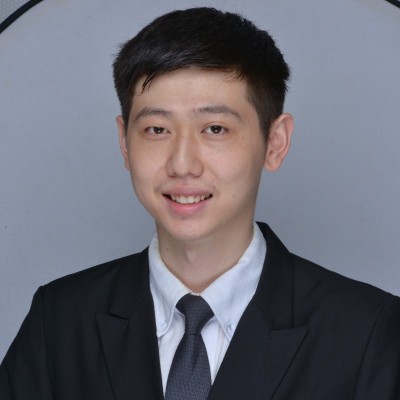 |
Zhang, Zeyu
Ph.D. (Co-advised with Bolin Ding, Alibaba Group)
Recipient of Economic Development Board Industrial Postgraduate Programme (EDB IPP)
B.Eng. in Electrical and Electronic Engineering (Hons. 1st Class) with Minor in Physics and Mathematics > Nanyang Technological University
Research Interests: machine unlearning, federated learning
|
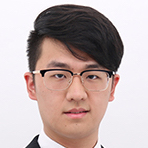 |
Wu, Zhaoxuan
Ph.D.
Recipient of Singapore Data Science Consortium (SDSC) Dissertation Research Fellowship, NUS Graduate School for Integrative Sciences and Engineering Scholarship (NGSS), Lijen Industrial Development Medal for the best student in the Honours Year term project in B.Sc. (Honours) - Data Science and Analytics programme
B.Sc. in Data Science and Analytics (Hons. 1st Class) > National University of Singapore
Research Interests: incentives in collaborative machine learning and federated learning, data valuation
|
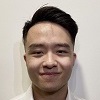 |
Tay, Sebastian Shenghong
Ph.D. (Co-advised with Chuan-Sheng Foo, A*STAR)
Recipient of NUS Research Achievement Award x 2, A*STAR Computing and Information Science Scholarship (ACIS)
B.Sc. in Computer Science (Hons. 1st Class) > National University of Singapore
Research Interests: incentives in collaborative machine learning and federated learning, data valuation, Bayesian optimization
|
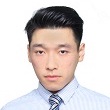 |
Xu, Xinyi |徐信羿|
Ph.D. (Co-advised with Chuan-Sheng Foo, A*STAR)
Recipient of NUS Research Achievement Award x 2, A*STAR Computing and Information Science Scholarship (ACIS), Honor List of Student Tutors for Excellence in Teaching, Teaching Fellowship Scheme
B.Sc. in Computer Science (Hons. 1st Class) > National University of Singapore
Research Interests: incentives in collaborative machine learning and federated learning, reinforcement learning, data valuation
|
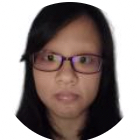 |
Sim, Rachael Hwee Ling
Ph.D. (Co-advised by Patrick Jaillet, MIT)
Recipient of NUS Research Achievement Award x 2, Teaching Fellowship Scheme (selected), SMART Graduate Fellowship, Lee Kuan Yew Gold Medal for best performing graduate in B.Comp. (Computer Science) programme, Tata Consultancy Services Asia Pacific Prize for Best Year 3 B.Comp. (Computer Science) student
B.Sc. in Computer Science (Hons. 1st Class) > National University of Singapore
Research Interests: incentives in collaborative machine learning and federated learning, data valuation, Bayesian optimization
|
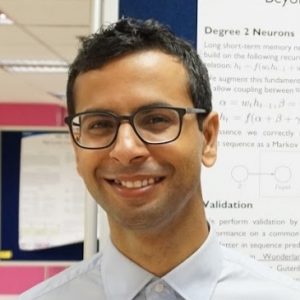 |
Mohit Rajpal
Ph.D.
Recipient of NUS President's Graduate Fellowship
M.Sc. in Computer Science > Columbia University
B.Sc. in Computer Science > University of Illinois Urbana-Champaign
Research Interests: deep learning
|
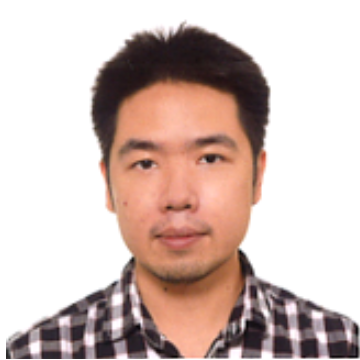 |
Lucas Agussurja
Ph.D.
Recipient of NUS Research Achievement Award
B.Sc. in Computer Science (Hons. 2nd Upper Class) > National University of Singapore
Research Interests: data valuation
|
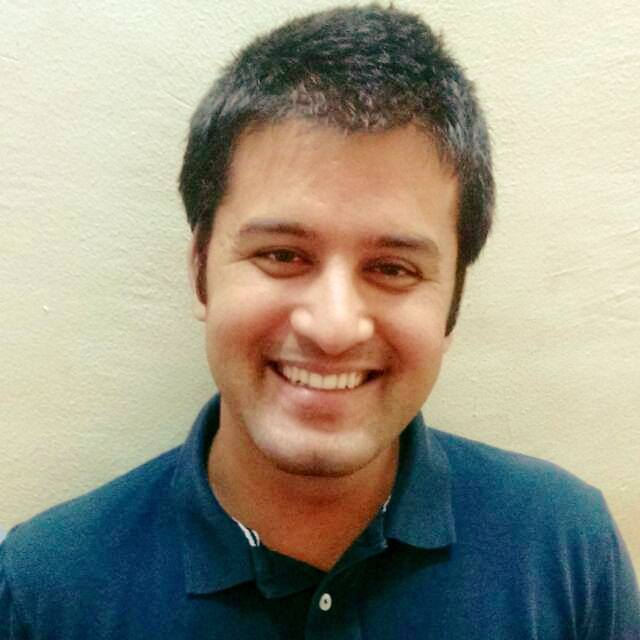 |
Arun Verma
Postdoctoral Fellow
Recipient of Naik and Rastogi Excellence in Ph.D. Thesis Award in IIT Bombay, COMSNETS 2022 Best Ph.D. Thesis Award, & Academic Excellence Award (Gold Medal) for highest CGPA in B.Tech. in CS & Eng., Shobhit University
B.Tech. in Computer Science and Engineering > Shobhit University, 2014
Ph.D. in Industrial Engineering and Operations Research > Indian Institute of Technology Bombay, Dec 2020
Ph.D. Thesis: Sequential Decision Problems with Weak Feedback
Research Interests: reinforcement learning, deep learning, and federated learning
|
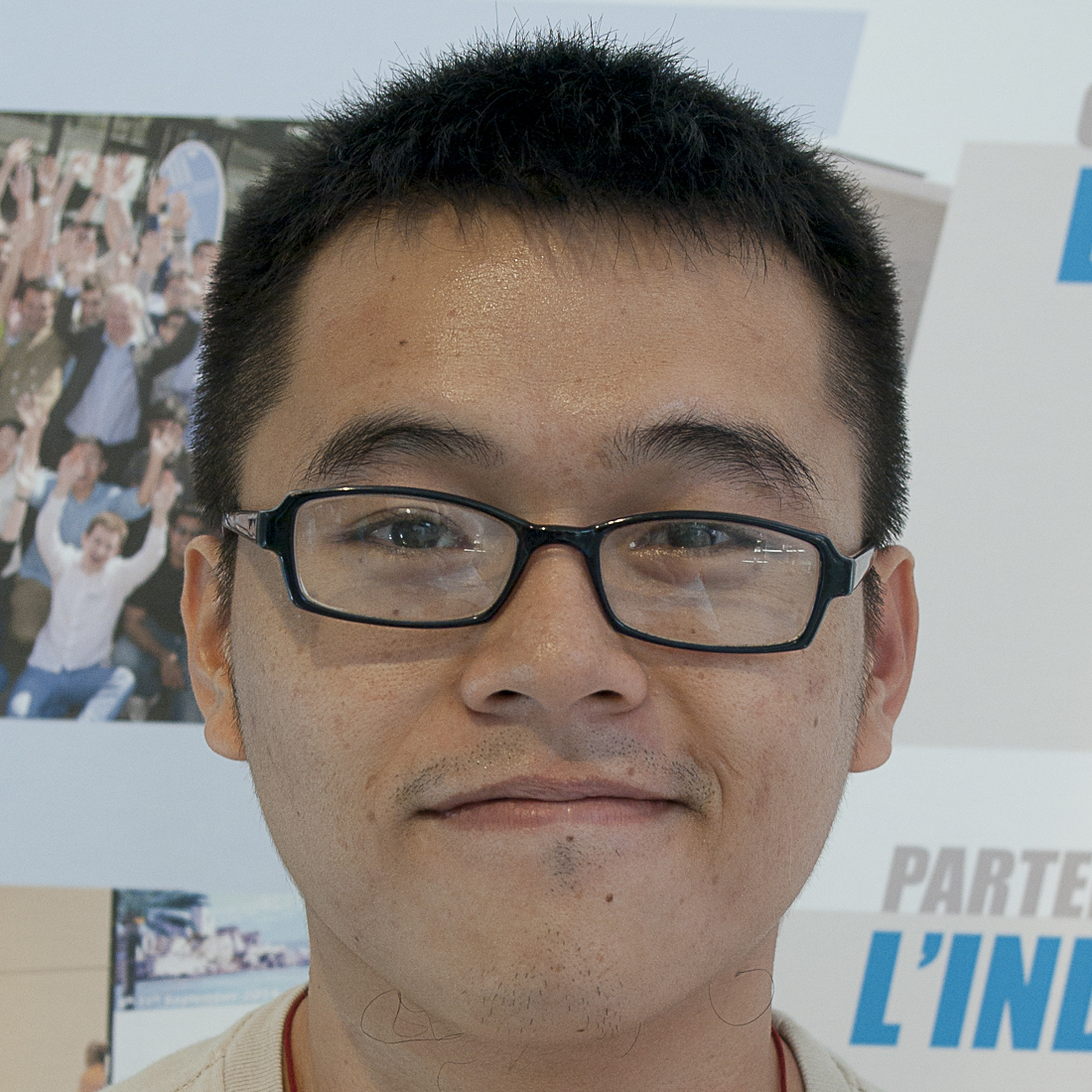 |
Trần, Gia Lạc
Postdoctoral Fellow
B.Sc. in Computer Science > Honors Program of Faculty of Information Technology > Vietnam National University Ho Chi Minh City - University of Science, Sep 2014
M.Eng. in Data Science > Télécom Paris, Sep 2017
Ph.D. in Computer Science > Sorbonne Université, Dec 2020
Ph.D. Thesis: Advances of Deep Gaussian Processes: Calibration and Sparsification
Research Interests: Gaussian process
|
Former Members
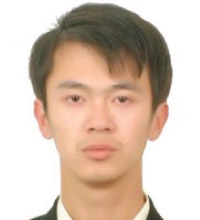 |
Dai, Zhongxiang |代忠祥|
Postdoctoral Fellow, Massachusetts Institute of Technology, Jan 2024 (Advised by Patrick Jaillet, MIT)
Recipient of NUS Dean's Graduate Research Excellence Award, NUS Research Achievement Award x 2, SMART Graduate Fellowship, & ST Electronics Prizes for being the top Year 1 and Year 2 student in Electrical Engineering
B.Eng. in Electrical Engineering (Hons. 1st Class) > National University of Singapore
Ph.D. in Computer Science > National University of Singapore (Co-advised by Patrick Jaillet, MIT), Jul 2021
Postdoctoral Fellow > National University of Singapore, Aug 2021 - Dec 2023
Ph.D. Thesis: Sample-Efficient Automated Machine Learning with Bayesian Optimization
Research Interests: Bayesian optimization
|
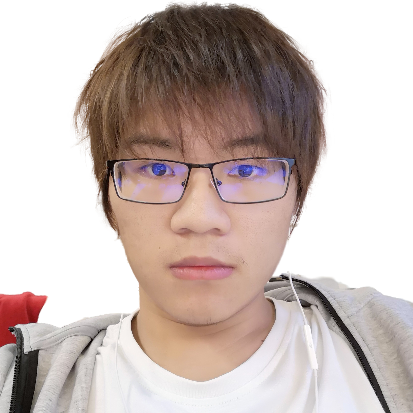 |
Shu, Yao |舒瑶|
Senior Researcher, Tencent AI Lab, Jun 2023
Recipient of IMDA Excellence in Computing Prize (Best Ph.D. Thesis in NUS School of Computing) 2023 & NUS Dean's Graduate Research Excellence Award, Valedictorian for the class of NUS School of Computing Ph.D. graduates 2023
B.Sc. in Computer Science > Huazhong University of Science and Technology
Ph.D. in Computer Science > National University of Singapore, May 2022
Postdoctoral Fellow > National University of Singapore, Jun 2022 - May 2023
Ph.D. Thesis: Understanding and Improving Neural Architecture Search
Research Interests: automated machine learning (AutoML), neural architecture search, hyperparameter optimization
|
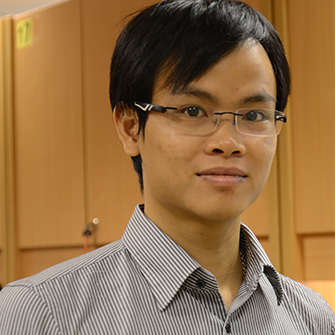 |
Nguyễn, Quốc Phong
Postdoctoral Associate, Massachusetts Institute of Technology, Apr 2023 (Advised by Patrick Jaillet, MIT)
Recipient of NUS Research Achievement Award, SMART SMA3 Graduate Fellowship, Lee Kuan Yew Gold Medal for best performing graduate in B.Eng. (Computing Engineering) programme, & IES Gold Medal for top graduating student in B.Eng. in Computer Engineering
B.Eng. in Computer Engineering > National University of Singapore, 2013
Ph.D. in Computer Science > National University of Singapore (Co-advised by Patrick Jaillet, MIT), Dec 2018
Postdoctoral Fellow & Senior Postdoctoral Fellow > National University of Singapore, Jan 2019 - Mar 2023
Ph.D. Thesis: An Alternative Information-Theoretic Criterion for Active Learning
Research Interests: probabilistic machine learning, Bayesian optimization, inverse reinforcement learning, collaborative machine learning
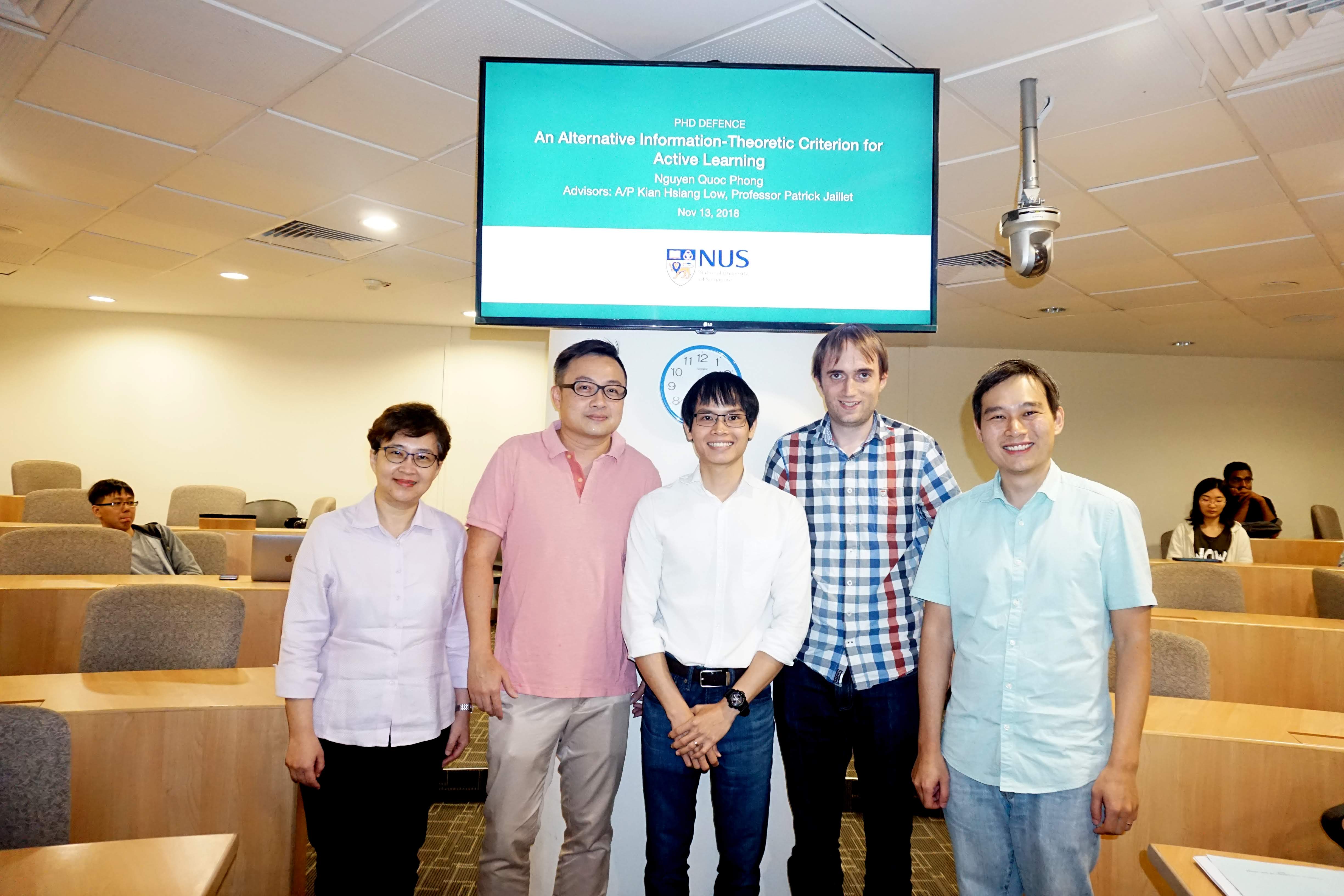
+ oral defense ◊ nov 13 ◊ 2K+18
|
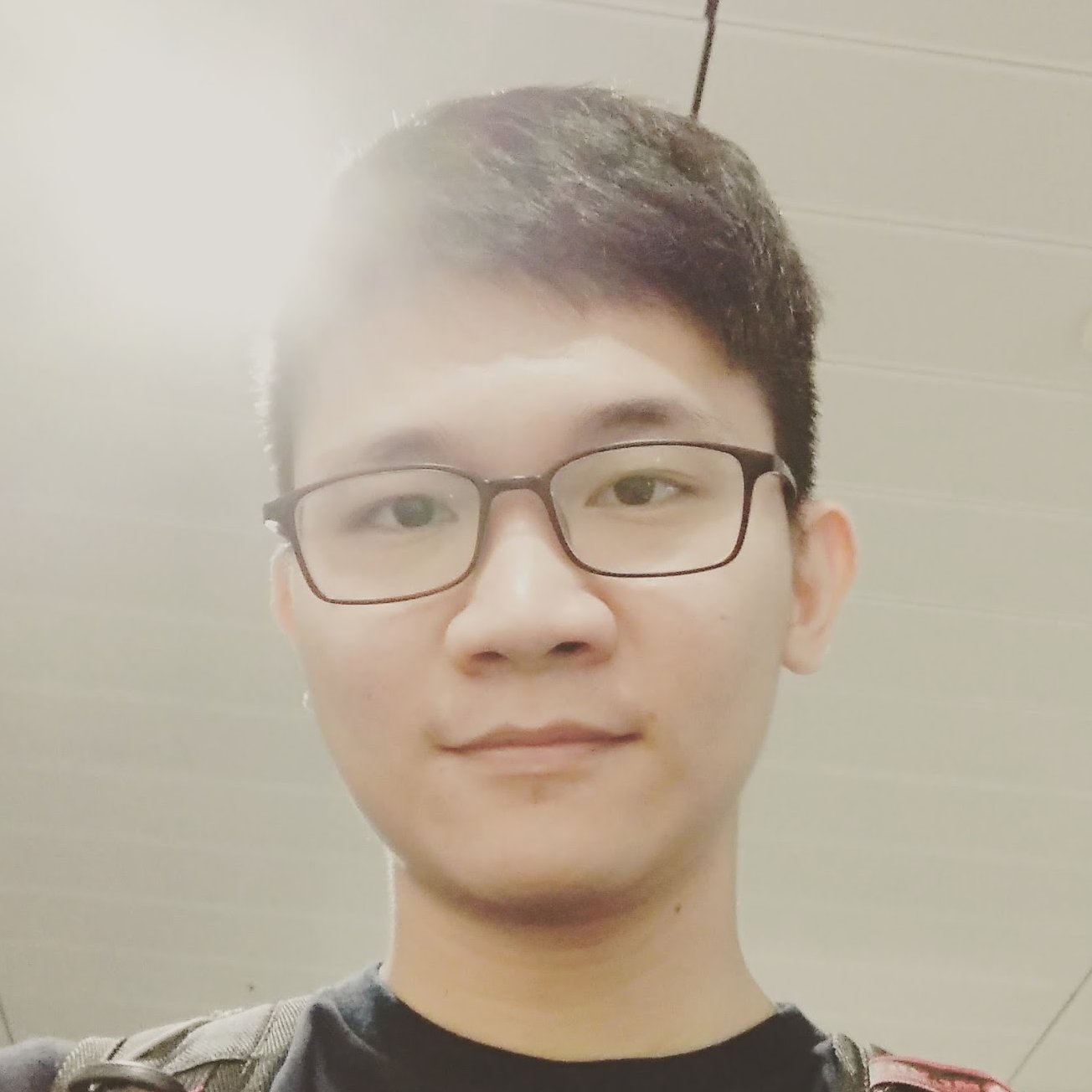 |
Lam, Chi Thanh
Quantitative Researcher, Citadel Securities, Jun 2023
Recipient of NUS Research Achievement Award x 2, Honor List of Student Tutors for Excellence in Teaching, & SMART Graduate Fellowship
B.Sc. in Computer Science (Hons. 1st Class) > National University of Singapore
Ph.D. in Computer Science > National University of Singapore (Co-advised by Patrick Jaillet, MIT), May 2023
Ph.D. Thesis: Algorithms for Collaborative Machine Learning: Data Sharing and Model Sharing Perspectives
Research Interests: meta-learning, collaborative machine learning, reinforcement learning
|
 |
Sreejith Balakrishnan
Senior Responsible AI Scientist, Aicadium, Apr 2023
Recipient of NUS Research Achievement Award and Honor List of Student Tutors for Excellence in Teaching
B.Eng. in Electrical and Electronics Engineering (Hons. 1st Class) with Minor in Computing > Nanyang Technological University
M.Eng. in Electrical Engineering with Specialization in Computer Engineering > National University of Singapore
Ph.D. in Computer Science > National University of Singapore (Co-advised by Harold Soh), Mar 2023
Ph.D. Thesis: Towards Human-Centric AI: Inverse Reinforcement Learning Meets Algorithmic Fairness
Research Interests: robotics, machine learning
|
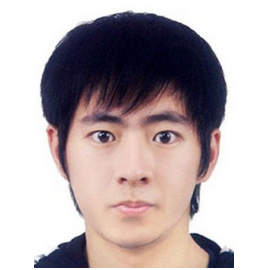 |
Chen, Yizhou
Machine Learning Engineer, Shopee, May 2022
B.Sc. in Physics (Hons. 1st Class) with Minor in Mathematics > Nanyang Technological University
Ph.D. in Computer Science > National University of Singapore, May 2022
Ph.D. Thesis: Exploiting Gradient Information for Modern Machine Learning Problems
Research Interests: meta-learning, adversarial machine learning, deep Gaussian process
|
 |
Teng, Tong |滕茼|
Research Engineer, Huawei Technologies, Dec 2021
Recipient of NUS Research Achievement Award
B.Eng. in Computer Science > Shandong University
Ph.D. in Computer Science > National University of Singapore, Dec 2021
Ph.D. Thesis: Automated Kernel Selection for Gaussian Processes on Large Datasets
Research Interests: probabilistic machine learning
|
 |
Dmitrii Kharkovskii |Дмитрий Алексеевич Харьковский|
Assistant Vice President, Data Science Lead, OCBC Group Data Office, Aug 2020
Recipient of NUS Research Achievement Award
Specialist degree in Math > St. Petersburg State University
Ph.D. in Computer Science > National University of Singapore, Dec 2020
Ph.D. Thesis: Automated Machine Learning: New Advances on Bayesian Optimization
Research Interests: privacy-preserving machine learning, Bayesian optimization
|
 |
Yu, Haibin |于海斌|
Senior Research Engineer, Tencent, Shenzhen, Jan 2021
Recipient of NUS Research Achievement Award and SMART Graduate Fellowship
B.Eng. in Mechanical Engineering and Automation > Beihang University
Ph.D. in Computer Science > National University of Singapore, May 2020 (Co-advised by Patrick Jaillet, MIT)
Ph.D. Thesis: New Advances in Bayesian Inference for Gaussian Process and Deep Gaussian Process Models
Research Interests: Bayesian deep learning
|
 |
Zhang, Yehong |张叶红|
Research Scientist, Peng Cheng Laboratory, Shenzhen, Aug 2020
Recipient of AAAI 2016 Scholarship & NUS Research Achievement Award
B.Eng. in Computer Science > Harbin Institute of Technology
Ph.D. in Computer Science > National University of Singapore, Dec 2017 (Co-advised by Mohan Kankanhalli)
Ph.D. Thesis: Data-Efficient Machine Learning with Multiple Output Types and High Input Dimensions
Research Interests: probabilistic machine learning, active learning, Bayesian optimization
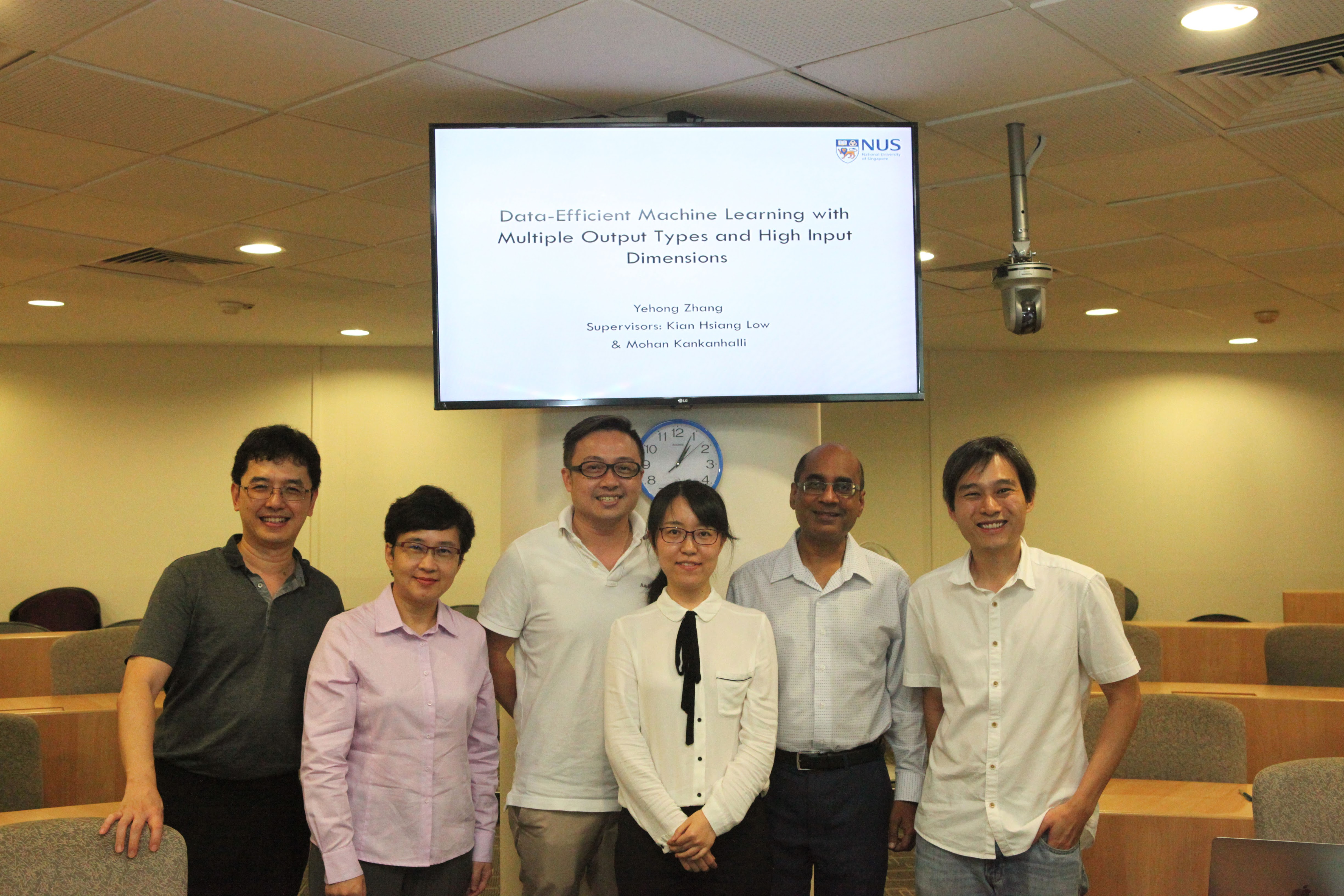
+ oral defense ◊ nov 20 ◊ 2K+17
|
 |
Chen, Jie |陈杰|
Assistant Professor, College of Computer Science and Software Engineering, Shenzhen University, Apr 2018
Recipient of NUS Dean's Graduate Research Excellence Award, NUS Research Achievement Award, & UAI 2012 Scholarship
B.Eng. in Electrical Engineering > Taizhou University
M.Eng. in Computer Science > Zhejiang University
Ph.D. in Computer Science > National University of Singapore, Dec 2013
Postdoctoral Associate > SMART FM, Jan 2014 - Mar 2018 (Co-advised by Patrick Jaillet, MIT)
Ph.D. Thesis: Gaussian Process-Based Decentralized Data Fusion & Active Sensing Agents: Towards Large-Scale Modeling & Prediction of Spatiotemporal Traffic Phenomena
Research Interests: robotics, multi-agent planning, machine learning
|
 |
Hoàng, Trọng Nghĩa
Assistant Professor, Washington State University, Jan 2023
Recipient of NUS Dean's Graduate Research Excellence Award, President's Graduate Fellowship, NUS Research Achievement Awards x 2, IJCAI 2013 Travel Grant Award, & AAMAS 2012 Scholarship
B.Sc. in Computer Science (Hons) > University of Science > Vietnam National University
Ph.D. in Computer Science > National University of Singapore, Feb 2015
Postdoctoral Fellow > Massachusetts Institute of Technology, Apr 2017 (Advised by Jonathan How)
Research Staff Member, MIT-IBM Watson AI Lab, Aug 2018
Senior Research Scientist, Amazon AWS AI Labs, Nov 2020
Ph.D. Thesis: New Advances on Bayesian and Decision-Theoretic Approaches for Interactive Machine Learning
Research Interests: machine learning, multi-agent planning
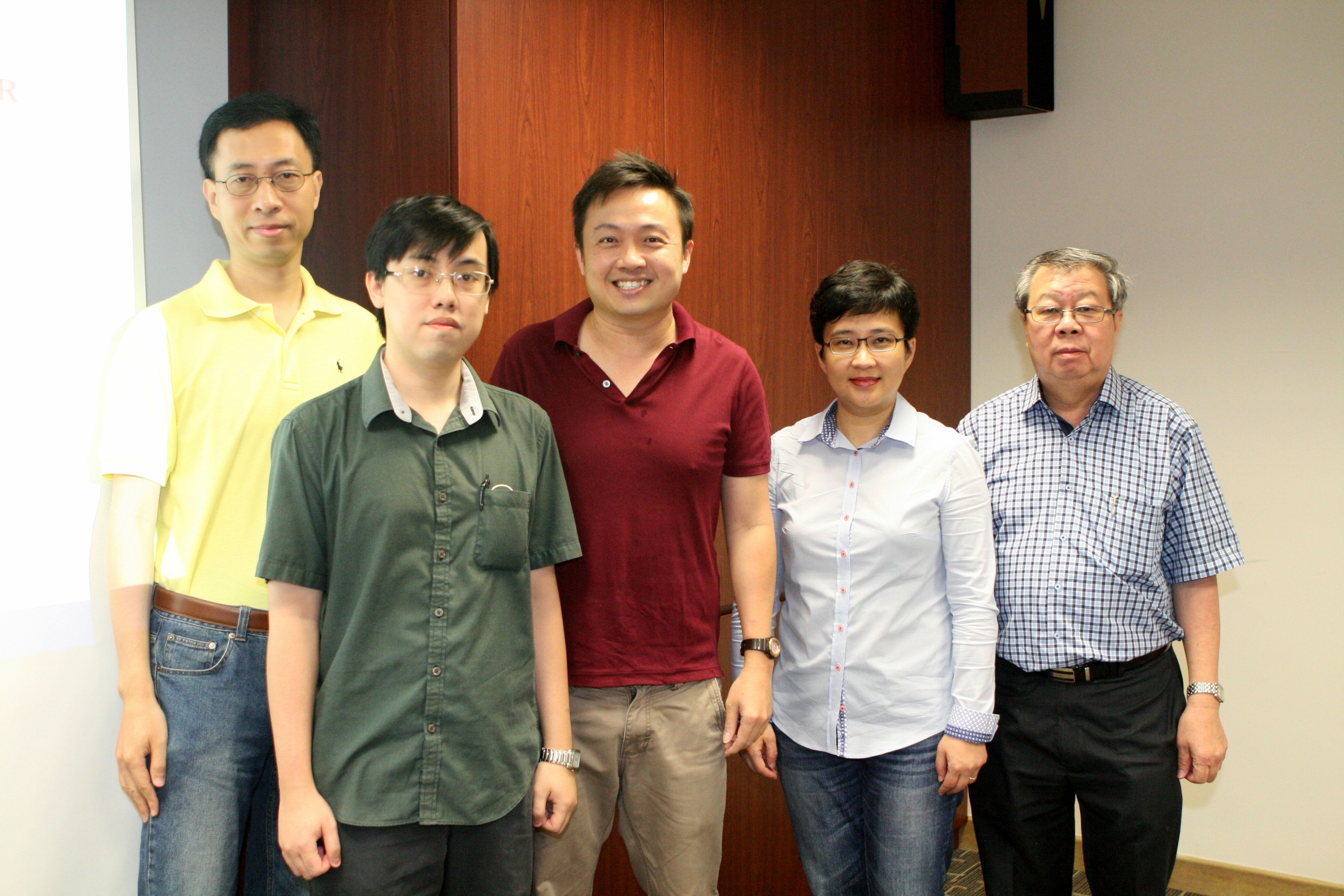
+ oral defense ◊ jan 21 ◊ 2K+15
|
 |
Ouyang, Ruofei |欧阳若飞|
Senior Data Scientist, Shopee
Recipient of AAMAS 2014 Scholarship
B.Sc. in Computer Science > East China Normal University
Ph.D. in Computer Science > National University of Singapore, Dec 2016
Ph.D. Thesis: Exploiting Decentralized Multiagent Coordination for Large-Scale Machine Learning Problems
Research Interests: Gaussian process, active sensing/learning, data fusion, Bayesian optimization

+ oral defense ◊ nov 7 ◊ 2K+16
|
 |
Xu, Nuo |许诺|
Backend Engineer, Grab
Recipient of AAAI 2014 Scholarship & NUS Research Achievement Award
Bachelor of Software Engineering > Harbin Institute of Technology
Ph.D. in Computer Science > National University of Singapore, Jan 2017
Ph.D. Thesis: Online Gaussian Process Filtering for Persistent Robot Localization With Arbitrary Sensor Modalities
Research Interests: machine learning, robotics
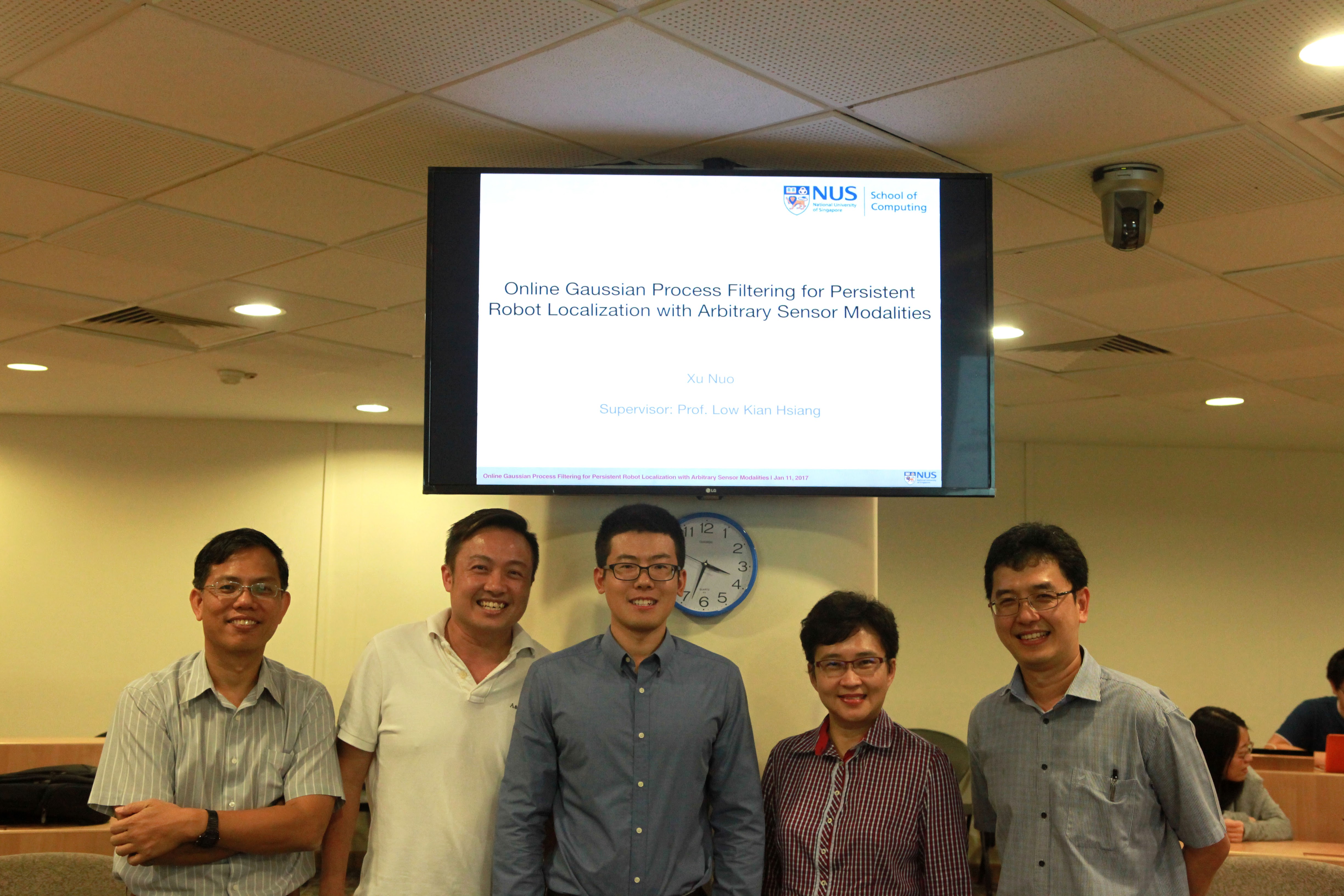
+ oral defense ◊ jan 11 ◊ 2K+17
|
 |
Prabhu Natarajan
Lecturer, Department of Computer Science, National University of Singapore, May 2021
Recipient of ICDSC 2012 Best PhD Forum Paper Award, NUS Research Achievement Award, & AAMAS 2012 Scholarship
B.Tech. in Information Technology > Anna University
M.Eng. in Computer Science & Engineering > Anna University
Ph.D. in Computer Science > National University of Singapore, Dec 2013 (Co-advised by Mohan Kankanhalli)
Assistant Professor, DigiPen Institute of Technology Singapore, Jun 2016 - Apr 2021
Ph.D. Thesis: A Decision-Theoretic Approach for Controlling & Coordinating Multiple Active Cameras in Surveillance
Research Interests: multi-camera surveillance, decision-theoretic planning & control for sensor networks
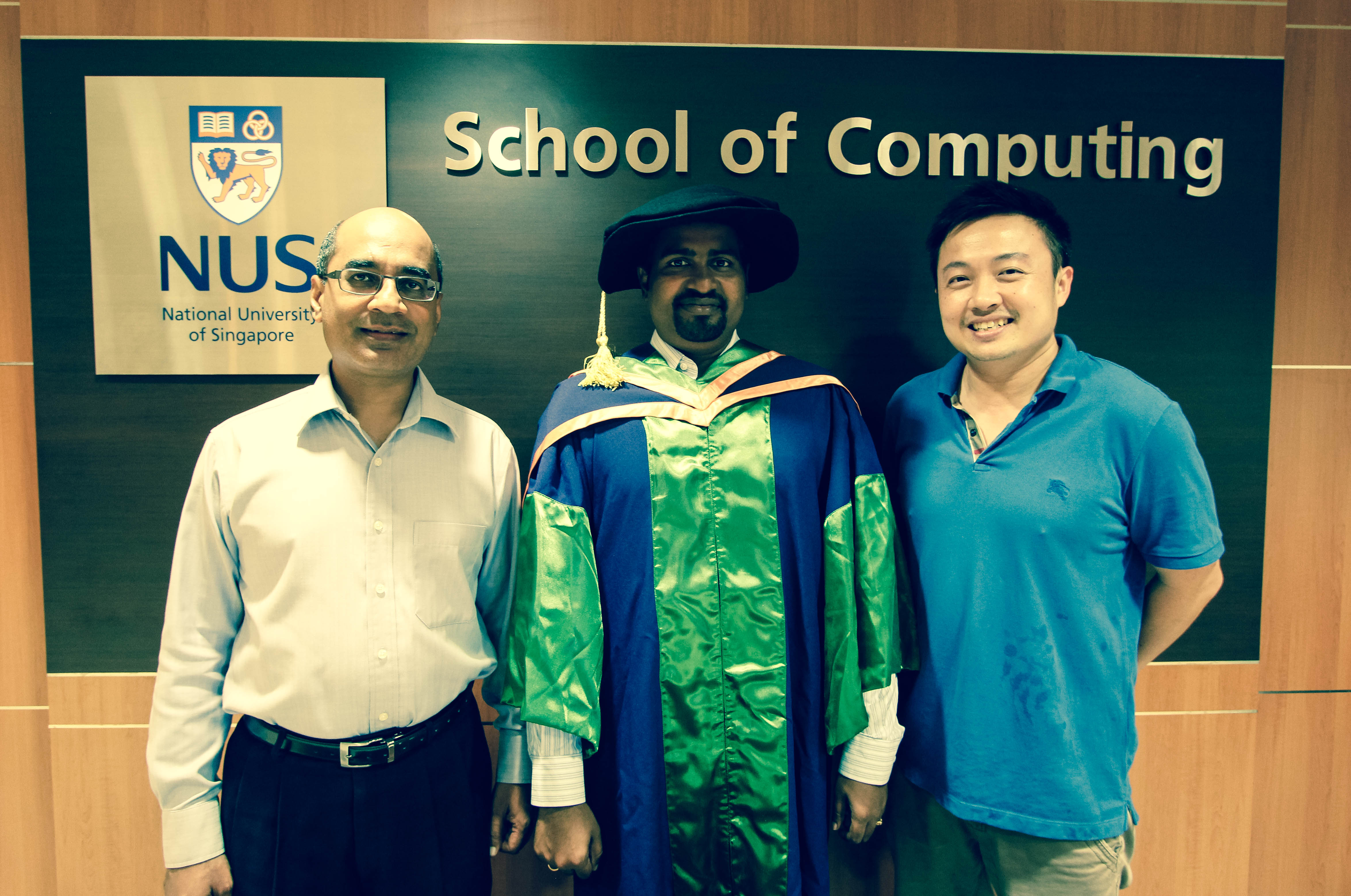
+ commencement ceremony ◊ aug 13 ◊ 2K+14
|
 |
Lim, Kar Wai
Research Staff Member, IBM Research, Singapore
Recipient of ACML 2016 Best Student Paper Award & AMP Prize for Honours Thesis in Actuarial Studies (Best Thesis Award)
Bachelor of Actuarial Studies (Hons. 1st Class) > Australian National University
Ph.D. in Computer Science > Australian National University, Dec 2016
Research Fellow > NUS-Singtel Cyber Security Research and Development Laboratory, Mar 2017 - May 2019 (Co-advised by Mun Choon Chan)
Ph.D. Thesis: Nonparametric Bayesian Topic Modelling with Auxiliary Data
Research Interests: machine learning, Bayesian nonparametric methods, stochastic processes, point processes, Hawkes processes, Bayesian inference, Markov chain Monte Carlo methods
|
 |
Tan, Wesley
Ph.D. in Computer Science, Nanyang Technological University, Aug 2017
Recipient of President's Graduate Fellowship in Nanyang Technological University
B.Sc. (Honors) in Economics > Purdue University
M.Sc. in Risk Management & Financial Engineering > Imperial College London, Oct 2015
M.Comp. in Computer Science > National University of Singapore, Jun 2017
M.Sc. Thesis: Variational Bayesian Actor Critic
Research Interests: reinforcement learning
|
 |
Son, Jaemin |손재민|
Bachelor of Comp Sci & Eng > Osaka University
M.Sc. in Computer Science > National University of Singapore, Nov 2016 (Co-advised by Gary Tan)
M.Sc. Thesis: High-Dimensional Bayesian Optimization with Application to Traffic Simulation
Research Interests: machine learning
|
 |
Cao, Nannan |曹楠楠|
Former Research Assistant
Bachelor of Software Engineering > East China Normal University
M.Sc. in Computer Science > National University of Singapore, Sep 2012
M.Sc. Thesis: Information-Theoretic Multi-Robot Path Planning
Research Interests: machine learning, Gaussian process, environmental sensing
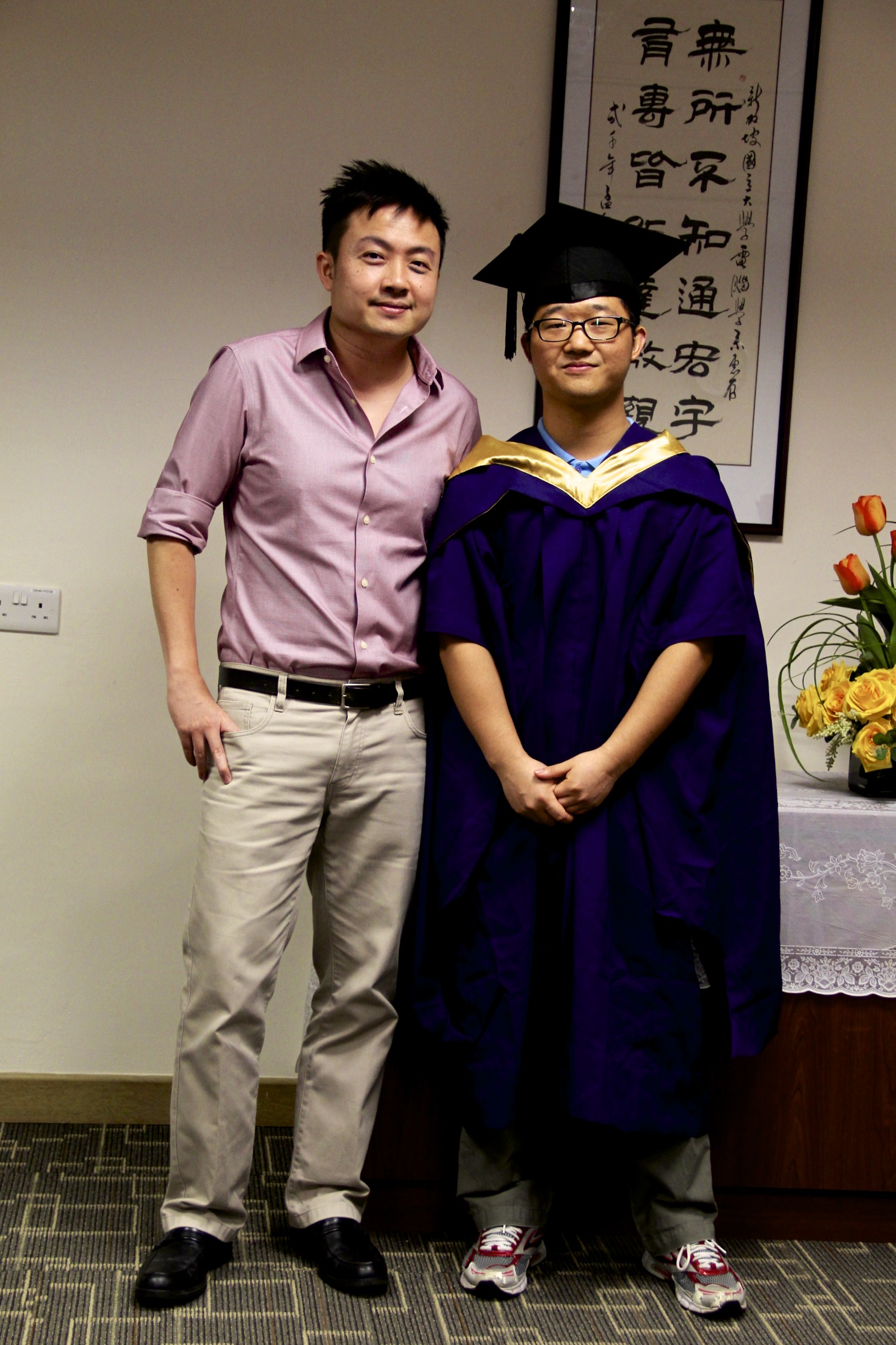
+ commencement ceremony ◊ sep 19 ◊ 2K+13
|
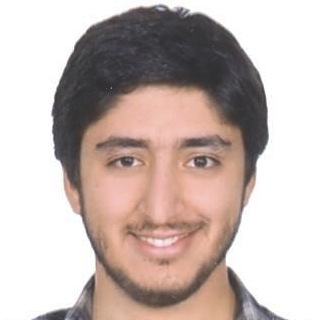 |
Etkin Barış Özgül
B.Sc. in Computer Science > Bilkent University
M.Sc. in Computer Science > National University of Singapore, Jan 2017
M.Sc. Thesis: Shuttle-line Routing for Mobility-on-Demand Systems with Ridesharing
Research Interests: AI, multi-agent systems
|
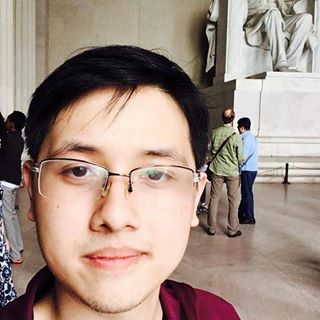 |
Hoang, Quang Minh
Ph.D. in Computer Science, CMU, Aug 2018
Recipient of Lee Kuan Yew Gold Medal for best performing graduate in B.Comp. (Computational Biology) programme, Outstanding Undergraduate Researcher Prize in National University of Singapore, & ICML 2015 Scholarship
B.Comp. in Computational Biology (Hons. 1st Class) > National University of Singapore, 2016
FYP Dissertation: A Probabilistic Approach for Protein Function Prediction with Hierarchical Structured Outputs
Research Interests: machine learning
|
 |
Ling, Chun Kai
Ph.D. in Computer Science, CMU, Aug 2017
Recipient of Lee Kuan Yew Gold Medal for best performing graduate in B.Eng. (Computing Engineering) programme, IES Gold Medal for top graduating student in B.Eng. in Computing Engineering, Defence Science Technology Agency Gold Medal for best local final year student for the degree of B.Eng. (Computer Engineering), Micron Prize for being one of the top two local Year 2 Computer Engineering students, & Alcatel-Lucent Telecommunications Prize for best performance in a module in the area of Communications and Networks in BEng (EE) or BEng (CEG) examinations
B.Eng. in Computer Engineering > National University of Singapore, 2015
FYP Dissertation: Planning and Learning in Spatiotemporal Environmental Phenomena
Research Interests: planning under uncertainty, machine learning
|
 |
Erik Alexander Daxberger
Ph.D., Department of Engineering, Univ. Cambridge, Jan 2019
Recipient of Cambridge-Tübingen Ph.D. fellowship in machine learning, LMU research award for excellent students for the Bachelor's thesis, and LMUexchange and PROSA scholarships for a student exchange program at NUS & ICML 2017 Travel Award
B.Sc. in Computer Science > Ludwig-Maximilians-Universität, Munich, 2017
B.Sc. Thesis: Distributed Batch Bayesian Optimization
Research Interests: machine learning, AI
|
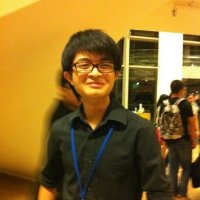 |
Khor, Shi-Jie
Software Engineer, Google Singapore
Recipient of Lee Kuan Yew Gold Medal for best performing graduate in B.Comp. (Computer Science) programme, IEEE Singapore Computer Society Book Prize for the best student in the Honours Year term project, and Tata Consultancy Services Asia Pacific Prize
B.Comp. in Computer Science (Honours Highest Distinction) > National University of Singapore, 2016
FYP Dissertation: Kernel Search for Gaussian Processes
Research Interests: machine learning
|
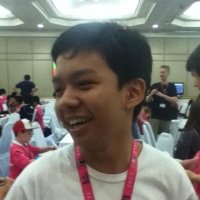 |
Nathan Azaria
Software Engineer, Facebook London
Recipient of National Computer Systems Medal And Prize for the top student in B.Comp. (Computer Science) programme
B.Comp. in Computer Science (Honours Highest Distinction) > National University of Singapore, 2016
FYP Dissertation: Stochastic Variational Inference on Multi-Output Gaussian Process
Research Interests: machine learning
|
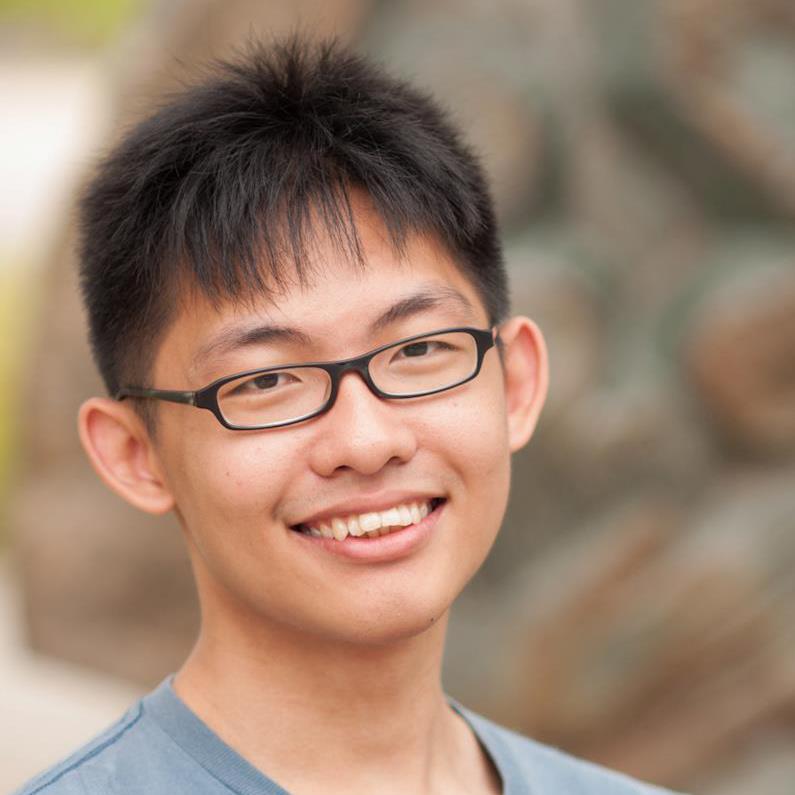 |
Lim, Keng Kiat
Software Engineer, Facebook HQ
B.Comp. in Computer Science > National University of Singapore, 2016
FYP Dissertation: Learning with High-Dimensional Data
Research Interests: machine learning
|
 |
Akshay Viswanathan
Software Engineer, Visa Inc.
B.Eng. in Computer Engineering (Hons. 1st Class) > National University of Singapore, 2015
FYP Dissertation: Scaling up Machine Learning Techniques via Parallelization for Large Data
Research Interests: machine learning
|
 |
Shailendra Khemka
Business Solutions: Software Engineer, Deutsche Bank AG - SG Branch
Recipient of Tata Consultancy Services Asia Pacific Medal and Prize for 2nd best graduate throughout the course of study for B.Comp, Defence Science & Technology Agency Prize for top UROP student in B.Comp., & Sung Kah Kay Memorial Prize Winner in NUS University Scholars Programme (USP)
University Scholars Programme & von Neumann Programme for B.Comp in Computer Science > National University of Singapore, 2013
FYP Dissertation: Autonomous Search for Victims in a Disaster Situation
Research Interests: multi-agent planning
|
 |
Yu, Jiangbo |余江波|
KAI Square
B.Sc. in Computer Science > Peking University
Research Interests: statistical machine learning
|
publications
RESEARCH SPOTLIGHTS lay articles for light reading
ACCEPTED PAPERS & PREPRINTS
Co-authors : My students and postdocs Former thesis advisors Collaborators
-
Fairness in federated learning.
Xiaoqiang Lin, Xinyi Xu, Zhaoxuan Wu, Rachael Hwee Ling Sim, See-Kiong Ng, Chuan-Sheng Foo, Patrick Jaillet, Trong Nghia Hoang & Bryan Kian Hsiang Low.
In L. M. Nguyen, T. N. Hoang, P.-Y. Chen, editors, Federated Learning:
Theory and Practice, chapter 8, pages 143-160, Academic Press, 2024.
Abstract. Federated learning (FL) enables a form of collaboration among multiple clients in jointly learning a machine learning (ML) model without centralizing their local datasets. Like in any collaboration, it is imperative to guarantee fairness so that the clients are willing to participate. For instance, it is unfair if one client benefits significantly more than others, or if some client benefits disproportionately to its contribution in the collaboration. Additionally, it is also unfair if the ML model makes biased predictions against certain groups of clients. This chapter discusses three specific notions of fairness by highlighting their motivations from real-world use-cases, examining several specific definitions for each notion and lastly describing the corresponding algorithms proposed to achieve each notion of fairness. At the end, this chapter will also summarize the identified gaps in current research efforts into open problems.
-
Federated sequential decision making: Bayesian optimization, reinforcement learning, and beyond.
Zhongxiang Dai, Flint Xiaofeng Fan, Cheston Tan, Trong Nghia Hoang, Bryan Kian Hsiang Low & Patrick Jaillet.
In L. M. Nguyen, T. N. Hoang, P.-Y. Chen, editors, Federated Learning:
Theory and Practice, chapter 14, pages 257-279, Academic Press, 2024.
Abstract. Federated learning (FL) in its classic form involves the collaborative training of supervised learning models (e.g., neural networks) among multiple agents/clients. However, in addition to supervised learning, many other machine learning tasks which are inherently sequential decision-making problems, such as Bayesian optimization (BO) and reinforcement learning (RL), also find important applications in the federated setting. For example, the crucial problem of hyperparameter tuning of neural networks in the federated setting calls for algorithms for federated BO; collaborative clinical treatment recommendation among multiple hospitals is a natural application for federated RL. However, the extension of these classic sequential decision-making algorithms into the federated setting is faced with immense challenges. Firstly, these algorithms (e.g., BO and RL) have to be adapted to satisfy the core principles of FL. For example, consistent with the requirement of FL, the raw data (e.g., the history of observations in BO and the trajectories in RL) of every agent can never be shared with the other agents. Next, it is challenging to preserve the rigorous theoretical guarantees of these classic sequential decision-making algorithms (e.g., the sub-linear regret upper bound of classic BO algorithms and the sample complexity of classic policy gradient algorithms for RL) and at the same time consistently improve their empirical performances by leveraging the federation of multiple agents. In this regard, a number of recent works have tackled these challenges and hence introduced federated versions of classic sequential decision-making algorithms (e.g., federated BO and federated RL algorithms) which satisfy the core principles of FL and are both theoretically grounded and practically effective. In light of these recent advances, this chapter discusses federated sequential decision-making problems with a focus on recent representative works on federated BO and federated RL, and describes open problems and potential future directions in these areas.
-
Data valuation in federated learning.
Zhaoxuan Wu, Xinyi Xu, Rachael Hwee Ling Sim, Yao Shu, Xiaoqiang Lin, Lucas Agussurja, Zhongxiang Dai, See-Kiong Ng, Chuan-Sheng Foo, Patrick Jaillet, Trong Nghia Hoang & Bryan Kian Hsiang Low.
In L. M. Nguyen, T. N. Hoang, P.-Y. Chen, editors, Federated Learning:
Theory and Practice, chapter 15, pages 281-296, Academic Press, 2024.
Abstract. Federated learning (FL) has become an increasingly popular solution paradigm for enabling collaborative machine learning (CML) in which multiple clients can collaboratively train a common model without sharing their private training data with others. However, broad adoption of FL in practice is still limited, as clients can often be reluctant to participate in such federated effort unless their contributions are accurately recognized and fairly compensated. Data valuation is thus extensively required to measure the relative contributions among clients. In this chapter, we review data valuation methods in the conventional supervised CML setting, followed by extensions to the FL paradigm. To better address the challenge that the private data from local clients cannot be made available to the server, we further discuss many specialized data valuation methods developed for both horizontal and vertical FL in detail. Overall, this chapter aims to provide a comprehensive suite of data valuation tools to empower FL practitioners in various practical scenarios.
-
Incentives in federated learning.
Rachael Hwee Ling Sim, Sebastian Shenghong Tay, Xinyi Xu, Yehong Zhang, Zhaoxuan Wu, Xiaoqiang Lin, See-Kiong Ng, Chuan-Sheng Foo, Patrick Jaillet, Trong Nghia Hoang & Bryan Kian Hsiang Low.
In L. M. Nguyen, T. N. Hoang, P.-Y. Chen, editors, Federated Learning:
Theory and Practice, chapter 16, pages 299-309, Academic Press, 2024.
Abstract. This chapter explores incentive schemes that encourage clients to participate in federated learning (FL) and contribute more valuable data. Such schemes are important to enable collaboration in competitive situations where clients need justifiable incentives to participate and benefit others with information acquired at significant costs and resources, such as collecting and processing data, computing and communicating model updates, risking the privacy of data via shared model updates. Incentivization addresses these concerns through three key components: (1) fair contribution evaluation of each client's data, (2) client selection to maximize the utility of the global model, and (3) reward allocation to clients. Intuitively, clients desire higher valued rewards which should at least outweigh their costs. These and other requirements will be formally described as incentives. The chapter will also discuss some recent solutions and open problems to achieve these incentives in various settings, which includes settings where the contribution evaluation is declared or measured while the rewards can be monetary- or model-based.
-
PINNACLE: PINN Adaptive ColLocation and Experimental points selection.
Gregory Kang Ruey Lau, Apivich Hemachandra, See-Kiong Ng & Bryan Kian Hsiang Low.
In Proceedings of the 12th International Conference on Learning Representations (ICLR-24), Vienna, Austria, May 7 - 11, 2024.
5% acceptance rate (spotlight)
Abstract. Physics-Informed Neural Networks (PINNs), which incorporate PDEs as soft constraints, train with a composite loss function that contains multiple training point types: different types of collocation points chosen during training to enforce each PDE and initial/boundary conditions, and experimental points which are usually costly to obtain via experiments or simulations. Training PINNs using this loss function is challenging as it typically requires selecting large numbers of points of different types, each with different training dynamics. Unlike past works that focused on the selection of either collocation or experimental points, this work introduces PINN Adaptive ColLocation and Experimental points selection (PINNACLE), the first algorithm that jointly optimizes the selection of all training point types, while automatically adjusting the proportion of collocation point types as training progresses. PINNACLE uses information on the interactions among training point types, which had not been considered before, based on an analysis of PINN training dynamics via the Neural Tangent Kernel (NTK). We theoretically show that the criterion used by PINNACLE is related to the PINN generalization error, and empirically demonstrate that PINNACLE is able to outperform existing point selection methods for forward, inverse, and transfer learning problems.
-
Robustifying and Boosting Training-Free Neural Architecture Search.
Zhenfeng He, Yao Shu, Zhongxiang Dai & Bryan Kian Hsiang Low.
In Proceedings of the 12th International Conference on Learning Representations (ICLR-24), Vienna, Austria, May 7 - 11, 2024.
31% acceptance rate
Abstract. Neural architecture search (NAS) has become a key component of AutoML and a standard tool to automate the design of deep neural networks. Recently, training-free NAS as an emerging paradigm has successfully reduced the search costs of standard training-based NAS by estimating the true architecture performance with only training-free metrics. Nevertheless, the estimation ability of these metrics typically varies across different tasks, making it challenging to achieve robust and consistently good search performance on diverse tasks with only a single training-free metric. Meanwhile, the estimation gap between training-free metrics and the true architecture performances limits training-free NAS to achieve superior performance. To address these challenges, we propose the robustifying and boosting training-free NAS (RoBoT) algorithm which (a) employs the optimized combination of existing training-free metrics explored from Bayesian optimization to develop a robust and consistently better-performing metric on diverse tasks, and (b) applies greedy search, i.e., the exploitation, on the newly developed metric to bridge the aforementioned gap and consequently to boost the search performance of standard training-free NAS further. Remarkably, the expected performance of our RoBoT can be theoretically guaranteed, which improves over the existing training-free NAS under mild conditions with additional interesting insights. Our extensive experiments on various NAS benchmark tasks yield substantial empirical evidence to support our theoretical results.
-
Incentive-Aware Federated Learning with Training-Time Model Rewards.
Zhaoxuan Wu, Mohammad Mohammadi Amiri, Ramesh Raskar & Bryan Kian Hsiang Low.
In Proceedings of the 12th International Conference on Learning Representations (ICLR-24), Vienna, Austria, May 7 - 11, 2024.
31% acceptance rate
Abstract. In federated learning (FL), incentivizing contributions of training resources (e.g., data, compute) from potentially competitive clients is crucial. Existing incentive mechanisms often distribute post-training monetary rewards, which suffer from practical challenges of timeliness and feasibility of the rewards. Rewarding the clients after the completion of training may incentivize them to abort the collaboration, and monetizing the contribution is challenging in practice. To address these problems, we propose an incentive-aware algorithm that offers differentiated training-time model rewards for each client at each FL iteration. We theoretically prove that such a local design ensures the global objective of client incentivization. Through theoretical analyses, we further identify the issue of error propagation in model rewards and thus propose a stochastic reference-model recovery strategy to ensure theoretically that all the clients eventually obtain the optimal model in the limit. We perform extensive experiments to demonstrate the superior incentivizing performance of our method compared to existing baselines.
-
Understanding Domain Generalization: A Noise Robustness Perspective.
Rui Qiao &
Bryan Kian Hsiang Low.
In Proceedings of the 12th International Conference on Learning Representations (ICLR-24), Vienna, Austria, May 7 - 11, 2024.
31% acceptance rate
Abstract. Despite the rapid development of machine learning algorithms for domain generalization (DG), there is no clear empirical evidence that the existing DG algorithms outperform the classic empirical risk minimization (ERM) across standard benchmarks. To better understand this phenomenon, we investigate whether there are benefits of DG algorithms over ERM through the lens of label noise. Specifically, our finite-sample analysis reveals that label noise exacerbates the effect of spurious correlations for ERM, undermining generalization. Conversely, we illustrate that DG algorithms exhibit implicit label-noise robustness during finite-sample training even when spurious correlation is present. Such desirable property helps mitigate spurious correlations and improve generalization in synthetic experiments. However, additional comprehensive experiments on real-world benchmark datasets indicate that label-noise robustness does not necessarily translate to better performance compared to ERM. We conjecture that the failure mode of ERM arising from spurious correlations may be less prevalent in practice.
-
A Unified Framework for Bayesian Optimization under Contextual Uncertainty.
Sebastian Shenghong Tay, Chuan-Sheng Foo, Daisuke Urano, Richalynn Leong &
Bryan Kian Hsiang Low.
In Proceedings of the 12th International Conference on Learning Representations (ICLR-24), Vienna, Austria, May 7 - 11, 2024.
31% acceptance rate
Abstract. Bayesian optimization under contextual uncertainty (BOCU) is a family of BO problems in which the learner makes a decision prior to observing the context and must manage the risks involved. Distributionally robust BO (DRBO) is a subset of BOCU that affords robustness against context distribution shift, and includes the optimization of expected values and worst-case values as special cases. By considering the first derivatives of the DRBO objective, we generalize DRBO to one that includes several other uncertainty objectives studied in the BOCU literature such as worst-case sensitivity (and thus notions of risk such as variance, range, and conditional value-at-risk) and mean-risk tradeoffs. We develop a general Thompson sampling algorithm that is able to optimize any objective within the BOCU framework, analyze its theoretical properties, and compare it to suitable baselines across different experimental settings and uncertainty objectives.
-
Optimistic Bayesian Optimization with Unknown Constraints.
Quoc Phong Nguyen, Wan Theng Ruth Chew, Le Song,
Bryan Kian Hsiang Low & Patrick Jaillet.
In Proceedings of the 12th International Conference on Learning Representations (ICLR-24), Vienna, Austria, May 7 - 11, 2024.
31% acceptance rate
Abstract. Though some research efforts have been dedicated to constrained Bayesian optimization (BO), there remains a notable absence of a principled approach with a theoretical performance guarantee in the decoupled setting. Such a setting involves independent evaluations of the objective function and constraints at different inputs, and is hence a relaxation of the commonly-studied coupled setting where functions must be evaluated together. As a result, the decoupled setting requires an adaptive selection between evaluating either the objective function or a constraint, in addition to selecting an input (in the coupled setting). This paper presents a novel constrained BO algorithm with a provable performance guarantee that can address the above relaxed setting. Specifically, it considers the fundamental trade-off between exploration and exploitation in constrained BO, and, interestingly, affords a noteworthy connection to active learning. The performance of our proposed algorithms is also empirically evaluated using several synthetic and real-world optimization problems.
-
Leveraging Previous Tasks in Optimizing Risk Measures with Gaussian Processes.
Quoc Phong Nguyen,
Bryan Kian Hsiang Low & Patrick Jaillet.
In Proceedings of the 12th International Conference on Learning Representations (ICLR-24), Vienna, Austria, May 7 - 11, 2024.
31% acceptance rate
Abstract. Research on optimizing the risk measure of a blackbox function using Gaussian processes, especially Bayesian optimization (BO) of risk measures, has become increasingly important due to the inevitable presence of uncontrollable variables in real-world applications. Nevertheless, existing works on BO of risk measures start the optimization from scratch for every new task without considering the results of previous tasks. In contrast, its vanilla BO counterpart has received a thorough investigation on utilizing previous tasks to speed up the current task through the body of works on meta-BO which, however, have not considered risk measures. To bridge this gap, this paper presents the first algorithm for meta-BO of risk measures (i.e., value-at-risk (VaR) and the conditional VaR) by introducing a novel adjustment to the upper confidence bound acquisition function. Our proposed algorithm exhibits two desirable properties: (i) invariance to scaling and vertical shifting of the blackbox function and (ii) robustness to previous harmful tasks. We provide a theoretical performance guarantee for our algorithm and empirically demonstrate its performance using several synthetic function benchmarks and real-world objective functions.
- WASA: WAtermark-based Source Attribution for Large Language Model-Generated Data.
Jingtan Wang, Xinyang Lu, Zitong Zhao, Zhongxiang Dai, Chuan-Sheng Foo, See-Kiong Ng & Bryan Kian Hsiang Low.
arXiv:2310.00646, Oct 1, 2023.
Abstract. The impressive performances of large language models (LLMs) and their immense potential for commercialization have given rise to serious concerns over the intellectual property (IP) of their training data.
In particular, the synthetic texts generated by LLMs may infringe the IP of the data being used to train the LLMs.
To this end, it is imperative to be able to (a) identify the data provider who contributed to the generation of a synthetic text by an LLM (source attribution) and (b) verify whether the text data from a data provider has been used to train an LLM (data provenance).
In this paper, we show that both problems can be solved by watermarking, i.e., by enabling an LLM to generate synthetic texts with embedded watermarks that contain information about their source(s).
We identify the key properties of such watermarking frameworks (e.g., source attribution accuracy, robustness against adversaries), and propose a WAtermarking for Source Attribution (WASA) framework that satisfies these key properties due to our algorithmic designs.
Our WASA framework enables an LLM to learn an accurate mapping from the texts of different data providers to their corresponding unique watermarks, which sets the foundation for effective source attribution (and hence data provenance).
Extensive empirical evaluations show that our WASA framework achieves effective source attribution and data provenance.
- Use Your INSTINCT: INSTruction optimization usIng Neural bandits Coupled with Transformers.
Xiaoqiang Lin, Zhaoxuan Wu, Zhongxiang Dai, Wenyang Hu, Yao Shu, See-Kiong Ng, Patrick Jaillet & Bryan Kian Hsiang Low.
arXiv:2310.02905, Oct 2, 2023.
Abstract. Large language models (LLMs) have shown remarkable instruction-following capabilities and achieved impressive performances in various applications. However, the performances of LLMs depend heavily on the instructions given to them, which are typically manually tuned with substantial human efforts. Recent work has used the query-efficient Bayesian optimization (BO) algorithm to automatically optimize the instructions given to black-box LLMs. However, BO usually falls short when optimizing highly sophisticated (e.g., high-dimensional) objective functions, such as the functions mapping an instruction to the performance of an LLM. This is mainly due to the limited expressive power of the Gaussian process (GP) model which is used by BO as a surrogate to model the objective function. Meanwhile, it has been repeatedly shown that neural networks (NNs), especially pre-trained transformers, possess strong expressive power and can model highly complex functions. So, we adopt a neural bandit algorithm which replaces the GP in BO by an NN surrogate to optimize instructions for black-box LLMs. More importantly, the neural bandit algorithm allows us to naturally couple the NN surrogate with the hidden representation learned by a pre-trained transformer (i.e., an open-source LLM), which significantly boosts its performance. These motivate us to propose our INSTruction optimization usIng Neural bandits Coupled with Transformers (INSTINCT) algorithm. We perform instruction optimization for ChatGPT and use extensive experiments to show that our INSTINCT consistently outperforms the existing methods in different tasks, such as in various instruction induction tasks and the task of improving the zero-shot chain-of-thought instruction.
- Goat: Fine-tuned LLaMA Outperforms GPT-4 on Arithmetic Tasks.
Tiedong Liu & Bryan Kian Hsiang Low.
arXiv:2305.14201, May 23, 2023.
Abstract. We introduce Goat, a fine-tuned LLaMA model that significantly outperforms GPT-4 on a range of arithmetic tasks. Fine-tuned on a synthetically generated dataset, Goat achieves state-of-the-art performance on BIG-bench arithmetic sub-task. In particular, the zero-shot Goat-7B matches or even surpasses the accuracy achieved by the few-shot PaLM-540B. Surprisingly, Goat can achieve near-perfect accuracy on large-number addition and subtraction through supervised fine-tuning only, which is almost impossible with previous pretrained language models, such as Bloom, OPT, GPT-NeoX, etc. We attribute Goat's exceptional performance to LLaMA's consistent tokenization of numbers. To tackle more challenging tasks like large-number multiplication and division, we propose an approach that classifies tasks based on their learnability, and subsequently decomposes unlearnable tasks, such as multi-digit multiplication and division, into a series of learnable tasks by leveraging basic arithmetic principles. We thoroughly examine the performance of our model, offering a comprehensive evaluation of the effectiveness of our proposed decomposition steps. Additionally, Goat-7B can be easily trained using LoRA on a 24GB VRAM GPU, facilitating reproducibility for other researchers. We release our model, dataset, and the Python script for dataset generation.
- DeRDaVa: Deletion-Robust Data Valuation for Machine Learning.
Xiao Tian, Rachael Hwee Ling Sim, Jue Fan & Bryan Kian Hsiang Low.
In Proceedings of the 38th AAAI Conference on Artificial Intelligence (AAAI-24), Vancouver, Canada, Feb 20 - Feb 27, 2024.
23.75% acceptance rate
Abstract. Data valuation is concerned with determining a fair valuation of data from data sources to compensate them or to identify training examples that are the most or least useful for predictions. With the rising interest in personal data ownership and data protection regulations, model owners will likely have to fulfil more data deletion requests. This raises issues that have not been addressed by existing works: Are the data valuation scores still fair with deletions? Must the scores be expensively recomputed? The answer is no. To avoid recomputations, we propose using our data valuation framework DeRDaVa upfront for valuing each data source's contribution to preserving robust model performance after anticipated data deletions. DeRDaVa can be efficiently approximated and will assign higher value to data that are more useful or less likely to be deleted. We further generalize DeRDaVa to Risk-DeRDaVa to cater to risk-averse/seeking model owners who are concerned with the worst/best-cases model utility. We also empirically demonstrate the practicality of our solutions.
- Incremental Quasi-Newton Methods with Faster Superlinear Convergence Rates.
Zhuanghua Liu, Luo Luo & Bryan Kian Hsiang Low.
In Proceedings of the 38th AAAI Conference on Artificial Intelligence (AAAI-24), Vancouver, Canada, Feb 20 - Feb 27, 2024.
23.75% acceptance rate
Abstract. We consider the finite-sum optimization problem of the form minx ∈ Rd f(x) = (1/n) (f1(x)+ ... + fi(x) + ... + fn(x)) where each fi(.) is strongly convex and has Lipschitz continuous gradient and Hessian. The recently proposed incremental quasi-Newton method is based on BFGS update and achieves a local superlinear convergence rate of O((1 − (dκ)-1)⌈t/n⌉2) where κ is the condition number and t is the number of iterations. This paper proposes a more efficient quasi-Newton method by incorporating the Symmetric Rank-1 update into the incremental framework, which results in the better local convergence rate of O((1 − d-1)⌈t/n⌉2). Furthermore, we can boost our method by applying the block update on the Hessian approximation, which leads to the even faster convergence
rate of O((1 − (kd)-1)⌈t/n⌉2) where k < d is the rank of update matrix. The numerical experiments show the proposed methods significantly outperform the baseline methods.
- Decentralized Sum-of-Nonconvex Optimization.
Zhuanghua Liu & Bryan Kian Hsiang Low.
In Proceedings of the 38th AAAI Conference on Artificial Intelligence (AAAI-24), Vancouver, Canada, Feb 20 - Feb 27, 2024.
23.75% acceptance rate
Abstract. We consider the optimization problem of minimizing the sum-of-nonconvex function, i.e., a convex function that is the average of nonconvex components. The existing stochastic algorithms for such a problem only focus on a single machine and the centralized scenario. In this paper, we study the sum-of-nonconvex optimization in the decentralized setting. We present a new theoretical analysis of the PMGT-SVRG algorithm to this problem and prove the linear convergence of their approach. However, the convergence rate of the PMGT-SVRG algorithm has a linear dependency on the condition number, which is undesirable for the ill-conditioned problem. To remedy this issue, we propose an accelerated stochastic decentralized first-order algorithm by incorporating the techniques of acceleration, gradient tracking, and multi-consensus mixing into the SVRG algorithm.
The convergence rate of the proposed method has a square-root dependency on the condition number. The numerical experiments validate the theoretical guarantee of our proposed algorithms on both synthetic and real-world datasets.
- Quantum Bayesian Optimization.
Zhongxiang Dai, Gregory Kang Ruey Lau, Arun Verma, Yao Shu, Bryan Kian Hsiang Low & Patrick Jaillet.
In Advances in Neural Information Processing Systems 36: 37th Annual Conference on Neural Information Processing Systems (NeurIPS'23), New Orleans, LA, Dec 10 - Dec 16, 2023.
26.1% acceptance rate
Abstract. Kernelized bandits, also known as Bayesian optimization (BO), has been a prevalent method for optimizing complicated black-box reward functions. Various BO algorithms have been theoretically shown to enjoy upper bounds on their cumulative regret which are sub-linear in the number T of iterations, and a regret lower bound of
Omega(sqrt(T)) has been derived which represents the unavoidable regrets for any classical BO algorithm. Recent works on quantum bandits have shown that with the aid of quantum computing, it is possible to achieve tighter regret upper bounds better than their corresponding classical lower bounds. However, these works are restricted to either multi-armed or linear bandits, and are hence not able to solve sophisticated real-world problems with non-linear reward functions. To this end, we introduce the quantum-Gaussian process-upper confidence bound (Q-GP-UCB) algorithm. To the best of our knowledge, our Q-GP-UCB is the first BO algorithm able to achieve a regret upper bound of O(poly log T), which is significantly smaller than its regret lower bound of
Omega(sqrt(T)) in the classical setting. Moreover, thanks to our novel analysis of the confidence ellipsoid, our Q-GP-UCB with the linear kernel achieves a smaller regret than the quantum linear UCB algorithm from the previous work. We use simulations to verify that the theoretical quantum speedup achieved by our Q-GP-UCB is also potentially relevant in practice.
- Incentives in Private Collaborative Machine Learning.
Rachael Hwee Ling Sim, Yehong Zhang, Trong Nghia Hoang, Xinyi Xu, Bryan Kian Hsiang Low & Patrick Jaillet.
In Advances in Neural Information Processing Systems 36: 37th Annual Conference on Neural Information Processing Systems (NeurIPS'23), New Orleans, LA, Dec 10 - Dec 16, 2023.
26.1% acceptance rate
Abstract. Collaborative machine learning involves training models on data from multiple parties but must incentivize their participation. Existing data valuation methods fairly value and reward each party based on shared data or model parameters but neglect the privacy risks involved. To address this, we introduce differential privacy (DP) as an incentive. Each party can select its required DP guarantee and perturb its sufficient statistic (SS) accordingly. The mediator values the perturbed SS by the Bayesian surprise it elicits about the model parameters. As our valuation function enforces a privacy-valuation trade-off, parties are deterred from selecting excessive DP guarantees that reduce the utility of the grand coalition's model. Finally, the mediator rewards each party with different posterior samples of the model parameters. Such rewards still satisfy existing incentives like fairness but additionally preserve DP and a high similarity to the grand coalition's posterior. We empirically demonstrate the effectiveness and practicality of our approach on synthetic and real-world datasets.
- Model Shapley: Equitable Model Valuation with Black-box Access.
Xinyi Xu, Chi Thanh Lam, Chuan-Sheng Foo & Bryan Kian Hsiang Low.
In Advances in Neural Information Processing Systems 36: 37th Annual Conference on Neural Information Processing Systems (NeurIPS'23), New Orleans, LA, Dec 10 - Dec 16, 2023.
26.1% acceptance rate
Abstract. Valuation methods of data and machine learning (ML) models are essential to the establishment of AI marketplaces. Importantly, certain practical considerations (e.g., operational constraints, legal restrictions) favor the use of model valuation over data valuation. Also, existing marketplaces that involve trading of pre-trained ML models call for an equitable model valuation method to price them. In particular, we investigate the black-box access setting which allows querying a model (to observe predictions) without disclosing model-specific information (e.g., architecture and parameters). By exploiting a Dirichlet abstraction of a model's predictions, we propose a novel and equitable model valuation method called model Shapley. We also leverage a formal connection between the similarity in models and that in model Shapley values (MSVs) to devise a learning approach for predicting MSVs of many vendors' models (e.g., 150) in a large-scale marketplace. We perform extensive empirical validation on the effectiveness of model Shapley using various real-world datasets and heterogeneous model types.
-
Bayesian Optimization with Cost-varying Variable Subsets.
Sebastian Shenghong Tay, Chuan-Sheng Foo, Daisuke Urano, Richalynn Leong & Bryan Kian Hsiang Low.
In Advances in Neural Information Processing Systems 36: 37th Annual Conference on Neural Information Processing Systems (NeurIPS'23), New Orleans, LA, Dec 10 - Dec 16, 2023.
26.1% acceptance rate
Abstract. We introduce the problem of Bayesian optimization with cost-varying variable subsets (BOCVS) where in each iteration, the learner chooses a subset of query variables and specifies their values while the rest are randomly sampled. Each chosen subset has an associated cost. This presents the learner with the novel challenge of balancing between choosing more informative subsets for more directed learning versus leaving some variables to be randomly sampled to reduce incurred costs. This paper presents a novel Gaussian process upper confidence bound-based algorithm for solving the BOCVS problem that is provably no-regret. We analyze how the availability of cheaper control sets helps in exploration and reduces overall regret. We empirically show that our proposed algorithm can find significantly better solutions than comparable baselines with the same budget.
-
Exploiting Correlated Auxiliary Feedback in Parameterized Bandits.
Arun Verma, Zhongxiang Dai, Yao Shu & Bryan Kian Hsiang Low.
In Advances in Neural Information Processing Systems 36: 37th Annual Conference on Neural Information Processing Systems (NeurIPS'23), New Orleans, LA, Dec 10 - Dec 16, 2023.
26.1% acceptance rate
Abstract. We study a novel variant of the parameterized bandits problem in which the learner can observe auxiliary feedback that is correlated with the observed reward. The auxiliary feedback is readily available in many real-life applications, e.g., an online platform that wants to recommend the best-rated services to its users can observe the user's rating of service (rewards) and collect additional information like service delivery time (auxiliary feedback). We first develop a method that exploits auxiliary feedback to build a reward estimator with tight confidence bounds, leading to a smaller regret. We then characterize the regret reduction in terms of the correlation coefficient between reward and auxiliary feedback. Experimental results in different settings also verify the performance gain achieved by our proposed method.
- Batch Bayesian Optimization For Replicable Experimental Design.
Zhongxiang Dai, Quoc Phong Nguyen, Sebastian Shenghong Tay, Daisuke Urano, Richalynn Leong, Bryan Kian Hsiang Low & Patrick Jaillet.
In Advances in Neural Information Processing Systems 36: 37th Annual Conference on Neural Information Processing Systems (NeurIPS'23), New Orleans, LA, Dec 10 - Dec 16, 2023.
26.1% acceptance rate
Abstract. Many real-world experimental design problems (a) evaluate multiple experimental conditions in parallel and (b) replicate each condition multiple times due to large and heteroscedastic observation noise. Given a fixed total budget, this naturally induces a trade-off between evaluating more unique conditions while replicating each of them fewer times vs. evaluating fewer unique conditions and replicating each more times. Moreover, in these problems, practitioners may be risk-averse and hence prefer an input with both good average performance and small variability. To tackle both challenges, we propose the Batch Thompson Sampling for Replicable Experimental Design (BTS-RED) framework, which encompasses three algorithms. Our BTS-RED-Known and BTS-RED-Unknown algorithms, for, respectively, known and unknown noise variance, choose the number of replications adaptively rather than deterministically such that an input with a larger noise variance is replicated more times. As a result, despite the noise heteroscedasticity, both algorithms enjoy a theoretical guarantee and are asymptotically no-regret. Our Mean-Var-BTS-RED algorithm aims at risk-averse optimization and is also asymptotically no-regret. We also show the effectiveness of our algorithms in two practical real-world applications: precision agriculture and AutoML.
|
REFEREED PUBLICATIONS
dblp
Sorted by year 2K +
23 |
22 |
21 |
20 |
19 |
18 |
17 |
16 |
15 |
14 |
13 |
12 |
11 |
10 |
9 |
8 |
7 |
6 |
5 |
4 |
3 |
2
- Training-Free Neural Active Learning with Initialization-Robustness Guarantees.
Apivich Hemachandra, Zhongxiang Dai, Jasraj Singh, See-Kiong Ng & Bryan Kian Hsiang Low.
In Proceedings of the 40th International Conference on Machine Learning (ICML-23), pages 12931-12971, Honolulu, HI, Jul 23 - 29, 2023.
27.9% acceptance rate
Abstract. Existing neural active learning algorithms have aimed to optimize the predictive performance of neural networks (NNs) by selecting data for labeling. However, other than a good predictive performance, being robust against random parameter initializations is also a crucial requirement in safety-critical applications. To this end, we introduce our expected variance with Gaussian processes (EV-GP) criterion for neural active learning, which is theoretically guaranteed to select data points that lead to trained NNs with both good predictive performances and initialization robustness. Importantly, our EV-GP criterion is training-free, i.e., it does not require any training of the NN during data selection, which makes it computationally efficient. We empirically demonstrate that our EV-GP criterion is highly correlated with both initialization robustness and generalization performance, and show that it consistently outperforms baseline methods in terms of both desiderata, especially in situations with limited initial data or large batch sizes.
- Collaborative Causal Inference with Fair Incentives.
Rui Qiao, Xinyi Xu & Bryan Kian Hsiang Low.
In Proceedings of the 40th International Conference on Machine Learning (ICML-23), pages 28300-28320, Honolulu, HI, Jul 23 - 29, 2023.
27.9% acceptance rate
Abstract. Collaborative causal inference (CCI) aims to improve the estimation of the causal effect of treatment variables by utilizing data aggregated from multiple self-interested parties. However, since their source data are valuable proprietary assets that can be costly or tedious to obtain, every party has to be incentivized to be willing to contribute to the collaboration, for example, with a guaranteed fair and sufficiently valuable reward (than performing causal inference on its own). This paper presents a reward scheme designed using the unique statistical properties required by causal inference to guarantee certain desirable incentive criteria (such as fairness and benefit) for the parties based on their contributions. To achieve this, we first propose a data valuation method to value parties' data for CCI based on the distributional closeness of its resulting treatment effect estimate to that utilizing the aggregated data from all parties. Then, we show how to value the parties' rewards fairly based on a modified variant of the Shapley value arising from our proposed data valuation for CCI. Finally, the Shapley fair rewards are realized in the form of improved and stochastically perturbed treatment effect estimates to be returned to the parties. We empirically demonstrate the effectiveness of our reward scheme using simulated and real-world datasets.
- Fair yet Asymptotically Equal Collaborative Learning.
Xiaoqiang Lin, Xinyi Xu, See-Kiong Ng, Chuan-Sheng Foo & Bryan Kian Hsiang Low.
In Proceedings of the 40th International Conference on Machine Learning (ICML-23), pages 21223-21259, Honolulu, HI, Jul 23 - 29, 2023.
27.9% acceptance rate
Abstract. In collaborative learning with streaming data, nodes (e.g., organizations) jointly and continuously learn a machine learning model by sharing the latest model updates computed from their latest streaming data. For the more resourceful nodes to be willing to share their model updates, they need to be fairly incentivized. This paper explores an incentive design that guarantees fairness so that nodes receive rewards commensurate to their contributions. Our approach leverages an explore-then-exploit formulation to estimate the nodes' contributions (i.e., exploration) for realizing our theoretically guaranteed fair incentives (i.e., exploitation). However, we observe a "rich get richer" phenomenon arising from the existing approaches to guarantee fairness and it discourages the participation of the less resourceful nodes. To remedy this, we additionally preserve asymptotic equality, i.e., less resourceful nodes achieve equal performance eventually to the more resourceful/"rich" nodes. We empirically demonstrate in two settings with real-world streaming data: federated online incremental learning and federated reinforcement learning, that our proposed approach outperforms existing baselines in fairness and learning performance while remaining competitive in preserving equality.
- Pruning during Training by Network Efficacy Modeling.
Mohit Rajpal, Yehong Zhang & Kian Hsiang Low.
Machine Learning (Special Issue on ECML-PKDD 2022 Journal Track), volume 112, issue 7, pages 2653-2684, 2023.
Abstract. Deep neural networks (DNNs) are costly to train. Pruning, an approach to
alleviate model complexity by zeroing out or pruning DNN elements, has shown promise in reducing training costs for DNNs with little to no efficacy at a given task. This paper presents a novel method to perform early pruning of DNN elements (e.g., neurons or convolutional filters) during the training process while minimizing losses to model performance. To achieve this, we model the efficacy of DNN elements in a Bayesian manner conditioned upon efficacy data collected during the training and prune DNN elements with low predictive efficacy after training completion. Empirical evaluations show that the proposed Bayesian early pruning improves the computational efficiency of DNN training while better preserving model performance compared to other tested pruning approaches.
- FAIR: Fair Collaborative Active Learning with Individual Rationality for Scientific Discovery.
Xinyi Xu,
Zhaoxuan Wu, Arun Verma, Chuan-Sheng Foo & Kian Hsiang Low.
In Proceedings of the 26th International Conference on Artificial Intelligence and Statistics (AISTATS-23), pages 4033-4057, Valencia, Spain, Apr 25 - 27, 2023.
29% acceptance rate
Abstract. Scientific discovery aims to find new patterns and test specific hypotheses by analysing large-scale experimental data. However, various practical limitations, like high experimental costs or the inability to do some experiments, make it challenging for researchers to collect sufficient experimental data for successful scientific discovery. To this end, we propose a framework named collaborative active learning (CAL) that enables researchers to share their experimental data for mutual benefit. Specifically, our proposed coordinated acquisition function sets out to achieve individual rationality and fairness so that everyone can equitably benefit from collaboration. Finally, we empirically demonstrate that our method outperforms existing batch active learning methods adapted to the CAL setting in terms of both learning performance and fairness on various real-world scientific discovery datasets (biochemistry, material science, and physics).
- No-Regret Sample-Efficient Bayesian Optimization for Finding Nash Equilibria with Unknown Utilities.
Sebastian Tay,
Quoc Phong Nguyen, Chuan-Sheng Foo & Kian Hsiang Low.
In Proceedings of the 26th International Conference on Artificial Intelligence and Statistics (AISTATS-23), pages 3591-3619, Valencia, Spain, Apr 25 - 27, 2023.
29% acceptance rate
Abstract. The Nash equilibrium (NE) is a classic solution concept for normal-form games that is stable under potential unilateral deviations by self-interested agents. Bayesian optimization (BO) has been used to find NE in continuous general-sum games with unknown costly-to-sample utility functions in a sample-efficient manner. This paper presents the first no-regret BO algorithm that is sample-efficient in finding pure NE by leveraging theory on high probability confidence bounds with Gaussian processes and the maximum information gain of kernel functions. Unlike previous works, our algorithm is theoretically guaranteed to converge to the optimal solution (i.e., NE). We also introduce the novel setting of applying BO to finding mixed NE in unknown discrete general-sum games and show that our theoretical framework is general enough to be extended naturally to this setting by developing a no-regret BO algorithm that is sample-efficient in finding mixed NE. We empirically show that our algorithms are competitive w.r.t. suitable baselines in finding NE.
-
Risk-Aware Reinforcement Learning with Coherent Risk Measures and Non-Linear Function Approximation.
Chi Thanh Lam,
Arun Verma, Kian Hsiang Low & Patrick Jaillet.
In Proceedings of the 11th International Conference on Learning Representations (ICLR-23), Kigali, Rwanda, May 1 - 5, 2023.
31.8% acceptance rate
Abstract. We study the risk-aware reinforcement learning (RL) problem in the episodic finite-horizon Markov decision process with unknown transition and reward functions. In contrast to the risk-neutral RL problem, we consider minimizing the risk of having low rewards, which arise due to the intrinsic randomness of the MDPs and imperfect knowledge of the model. Our work provides a unified framework to analyze the regret of risk-aware RL policy with coherent risk measures in conjunction with non-linear function approximation, which gives the first sub-linear regret bounds in the setting. Finally, we validate our theoretical results via empirical experiments on synthetic and real-world data.
-
Federated Neural Bandits.
Zhongxiang Dai,
Yao Shu,
Arun Verma, Flint Xiaofeng Fan, Kian Hsiang Low & Patrick Jaillet.
In Proceedings of the 11th International Conference on Learning Representations (ICLR-23), Kigali, Rwanda, May 1 - 5, 2023.
31.8% acceptance rate
Abstract. Recent works on neural contextual bandits have achieved compelling performances due to their ability to leverage the strong representation power of neural networks (NNs) for reward prediction. Many applications of contextual bandits involve multiple agents who collaborate without sharing raw observations, thus giving rise to the setting of federated contextual bandits}. Existing works on federated contextual bandits rely on linear or kernelized bandits, which may fall short when modeling complex real-world reward functions. So, this paper introduces the federated neural-upper confidence bound (FN-UCB) algorithm. To better exploit the federated setting, FN-UCB adopts a weighted combination of two UCBs: UCBa allows every agent to additionally use the observations from the other agents to accelerate exploration (without sharing raw observations), while UCBb uses an NN with aggregated parameters for reward prediction in a similar way to federated averaging for supervised learning. Notably, the weight between the two UCBs required by our theoretical analysis is amenable to an interesting interpretation, which emphasizes UCBa initially for accelerated exploration and relies more on UCBb later after enough observations have been collected to train the NNs for accurate reward prediction (i.e., reliable exploitation). We prove sub-linear upper bounds on both the cumulative regret and the number of communication rounds of FN-UCB, and empirically demonstrate its competitive performance.
- Zeroth-Order Optimization with Trajectory-Informed Derivative Estimation.
Yao Shu,
Zhongxiang Dai, Weicong Sng, Arun Verma, Patrick Jaillet & Kian Hsiang Low.
In Proceedings of the 11th International Conference on Learning Representations (ICLR-23), Kigali, Rwanda, May 1 - 5, 2023.
31.8% acceptance rate
Abstract. Zeroth-order (ZO) optimization, in which the derivative is unavailable, has recently succeeded in many important machine learning applications. Existing algorithms rely on finite difference (FD) methods for derivative estimation and gradient descent (GD)-based approaches for optimization. However, these algorithms suffer from query inefficiency because additional function queries are required for derivative estimation in their every GD update, which typically hinders their deployment in applications where every function query is expensive. To this end, we propose a trajectory-informed derivative estimation method which only uses the optimization trajectory (i.e., the history of function queries during optimization) and hence eliminates the need for additional function queries to estimate a derivative. Moreover, based on our derivative estimation, we propose the technique of dynamic virtual updates, which allows us to reliably perform multiple steps of GD updates without reapplying derivative estimation. Based on these two contributions, we introduce the zeroth-order optimization with trajectory-informed derivative estimation (ZoRD) algorithm for query-efficient ZO optimization. We theoretically demonstrate that our trajectory-informed derivative estimation and our ZoRD algorithm improve over existing approaches, which is then supported by our real-world experiments such as black-box adversarial attack, non-differentiable metric optimization and derivative-free reinforcement learning.
- Recursive Reasoning-Based Training-Time Adversarial Machine Learning.
Yizhou Chen, Zhongxiang Dai, Haibin Yu, Kian Hsiang Low & Teck-Hua Ho.
Artificial Intelligence (Special Issue on Risk-Aware Autonomous Systems: Theory and Practice), volume 315, pages 103837, Feb 2023.
Abstract. The training process of a machine learning (ML) model may be subject to adversarial attacks from an attacker who attempts to undermine the test performance of the ML model by perturbing the training minibatches, and thus needs to be protected by a defender. Such a problem setting is referred to as training-time adversarial ML. We formulate it as a two-player game and propose a principled Recursive Reasoning-based Training-Time adversarial ML (R2T2) framework to model this game. R2T2 models the reasoning process between the attacker and the defender and captures their bounded reasoning capabilities (due to bounded computational resources) through the recursive reasoning formalism. In particular, we associate a deeper level of recursive reasoning with the use of a higher-order gradient to derive the attack (defense) strategy, which naturally improves its performance while requiring greater computational resources. Interestingly, our R2T2 framework encompasses a variety of existing adversarial ML methods which correspond to attackers (defenders) with different recursive reasoning capabilities. We show how an R2T2 attacker (defender) can utilize our proposed nested projected gradient descent-based method to approximate the optimal attack (defense) strategy at an arbitrary level of reasoning. R2T2 can empirically achieve state-of-the-art attack and defense performances on benchmark image datasets.
- Probably Approximate Shapley Fairness with Applications in Machine Learning.
Zijian Zhou,
Xinyi Xu, Rachael Hwee Ling Sim, Chuan-Sheng Foo & Kian Hsiang Low.
In Proceedings of the 37th AAAI Conference on Artificial Intelligence (AAAI-23), pages 5910-5918, Washington, DC, Feb 7 - Feb 14, 2023.
19.6% acceptance rate (oral presentation)
Abstract. The Shapley value (SV) is adopted in various scenarios in machine learning including data valuation, agent valuation, feature attribution etc. because it guarantees fairness (i.e., satisfying several fairness axioms) and that fairness is important in such valuations (e.g., for pricing in a marketplace). However, the exact calculation of SVs (with exponential time complexity) is infeasible in practice, so estimations are necessary. Consequently, it raises the following important question: The exact SVs guarantee fairness, but do the SV estimates also guarantee the same fairness? We show the fairness guaranteed by exact SVs is too restrictive (for SV estimates) and generalise it to a probably approximate fairness. We propose fidelity score, a metric to measure the variation of SV estimates and establish theoretically the relationship between fidelity score and fairness guarantee. We exploit the relationship between fidelity score and fairness guarantee to propose a novel greedy active estimation (GAE) with theoretical guarantees. We theoretically show GAE achieves better fairness guarantee than the de facto Monte-Carlo estimation and empirically verify GAE outperforms several existing methods in guaranteeing fairness while remaining competitive in estimation accuracy in various specific ML scenarios using real-world datasets.
- Trade-off between Payoff and Model Rewards in Shapley-Fair Collaborative Machine Learning.
Quoc Phong Nguyen, Kian Hsiang Low & Patrick Jaillet.
In Advances in Neural Information Processing Systems 35: 36th Annual Conference on Neural Information Processing Systems (NeurIPS'22), pages 30542-30553, New Orleans, LA, Nov 28 - Dec 3, 2022.
25.6% acceptance rate
Abstract. This paper investigates the problem of fairly trading off between payoff and model rewards in collaborative machine learning (ML) where parties aggregate their datasets together to obtain improved ML models over that of each party. Supposing parties can afford the optimal model trained on the aggregated dataset, we propose an allocation scheme that distributes the payoff fairly. Notably, the same scheme can be derived from two different approaches based on (1) desirable properties of the parties' payoffs or (2) that of the underlying payoff flows from one party to another. While the former is conceptually simpler, the latter can be used to handle the practical constraint on the budgets of parties. In particular, we propose desirable properties for achieving a fair adjustment of the payoff flows that can trade off between the model reward's performance and the payoff reward. We empirically demonstrate that our proposed scheme is a sensible solution in several scenarios of collaborative ML with different budget constraints.
- Unifying and Boosting Gradient-Based Training-Free Neural Architecture Search.
Yao Shu, Zhongxiang Dai, Zhaoxuan Wu & Kian Hsiang Low.
In Advances in Neural Information Processing Systems 35: 36th Annual Conference on Neural Information Processing Systems (NeurIPS'22), pages 33001-33015, New Orleans, LA, Nov 28 - Dec 3, 2022.
25.6% acceptance rate
Abstract. Neural architecture search (NAS) has gained immense popularity owing to its ability to automate neural architecture design. A number of training-free metrics are recently proposed to realize NAS without training, hence making NAS more scalable. Despite their competitive empirical performances, a unified theoretical understanding of these training-free metrics is lacking. As a consequence, (a) the relationships among these metrics are unclear, (b) there is no theoretical interpretation for their empirical performances, and (c) there may exist untapped potential in existing training-free NAS, which probably can be unveiled through a unified theoretical understanding. To this end, this paper presents a unified theoretical analysis of gradient-based training-free NAS, which allows us to (a) theoretically study their relationships, (b) theoretically guarantee their generalization performances, and (c) exploit our unified theoretical understanding to develop a novel framework named hybrid NAS (HNAS) which consistently boosts training-free NAS in a principled way. Remarkably, HNAS can enjoy the advantages of both training-free (i.e., superior search efficiency) and training-based (i.e., remarkable search effectiveness) NAS, which we have demonstrated through extensive experiments.
- Sample-Then-Optimize Batch Neural Thompson Sampling.
Zhongxiang Dai, Yao Shu, Kian Hsiang Low & Patrick Jaillet.
In Advances in Neural Information Processing Systems 35: 36th Annual Conference on Neural Information Processing Systems (NeurIPS'22), pages 23331-23344, New Orleans, LA, Nov 28 - Dec 3, 2022.
25.6% acceptance rate
Abstract. Bayesian optimization (BO), which uses a Gaussian process (GP) as a surrogate to model its objective function, is popular for black-box optimization. However, due to the limitations of GPs, BO underperforms in some problems such as those with categorical, high-dimensional or image inputs. To this end, recent works have used the highly expressive neural networks (NNs) as the surrogate model and derived theoretical guarantees using the theory of neural tangent kernel (NTK). However, these works suffer from the limitations of the requirement to invert an extremely large parameter matrix and the restriction to the sequential (rather than batch) setting. To overcome these limitations, we introduce two algorithms based on the Thompson sampling (TS) policy named Sample-Then-Optimize Batch Neural TS (STO-BNTS) and STO-BNTS-Linear. To choose an input query, we only need to train an NN (resp. a linear model) and then choose the query by maximizing the trained NN (resp. linear model), which is equivalently sampled from the GP posterior with the NTK as the kernel function. As a result, our algorithms sidestep the need to invert the large parameter matrix yet still preserve the validity of the TS policy. Next, we derive regret upper bounds for our algorithms with batch evaluations, and use insights from batch BO and NTK to show that they are asymptotically no-regret under certain conditions. Finally, we verify their empirical effectiveness using practical AutoML and reinforcement learning experiments.
- On Provably Robust Meta-Bayesian Optimization.
Zhongxiang Dai, Yizhou Chen, Haibin Yu, Kian Hsiang Low & Patrick Jaillet.
In Proceedings of the 38th Conference on Uncertainty in Artificial Intelligence (UAI-22), pages 475-485, Eindhoven, Netherlands, Aug 1-5, 2022.
32.3% acceptance rate
Abstract. Bayesian optimization (BO) has become popular for sequential optimization of black-box functions. When BO is used to optimize a target function, we often have access to previous evaluations of potentially related functions. This begs the question as to whether we can leverage these previous experiences to accelerate the current BO task through meta-learning (meta-BO), while ensuring robustness against potentially harmful dissimilar tasks that could sabotage the convergence of BO. This paper introduces two scalable and provably robust meta-BO algorithms: robust meta-Gaussian process-upper confidence bound (RM-GP-UCB) and RM-GP-Thompson sampling (RM-GP-TS). We prove that both algorithms are asymptotically no-regret even when some or all previous tasks are dissimilar to the current task, and show that RM-GP-UCB enjoys a better theoretical robustness than RM-GP-TS. We also exploit the theoretical guarantees to optimize the weights assigned to individual previous tasks through regret minimization via online learning, which diminishes the impact of dissimilar tasks and hence further enhances the robustness. Empirical evaluations show that (a) RM-GP-UCB performs effectively and consistently across various applications, and (b) RM-GP-TS, despite being less robust than RM-GP-UCB both in theory and in practice, performs competitively in some scenarios with less dissimilar tasks and is more computationally efficient.
- Neural Ensemble Search via Bayesian Sampling.
Yao Shu, Yizhou Chen, Zhongxiang Dai & Kian Hsiang Low.
In Proceedings of the 38th Conference on Uncertainty in Artificial Intelligence (UAI-22), pages 1803-1812, Eindhoven, Netherlands, Aug 1-5, 2022.
32.3% acceptance rate
Abstract. Recently, neural architecture search (NAS) has been applied to automate the design of neural networks in real-world applications. A large number of algorithms have been developed to improve the search cost or the performance of the final selected architectures in NAS. Unfortunately, these NAS algorithms aim to select only one single well-performing architecture from their search spaces and thus have overlooked the capability of neural network ensemble (i.e., an ensemble of neural networks with diverse architectures) in achieving improved performance over a single final selected architecture. To this end, we introduce a novel neural ensemble search algorithm, called neural ensemble search via Bayesian sampling (NESBS), to effectively and efficiently select well-performing neural network ensembles from a NAS search space. In our extensive experiments, NESBS algorithm is shown to be able to achieve improved performance over state-of-the-art NAS algorithms while incurring a comparable search cost, indicating the superior performance of our NESBS algorithm over these conventional NAS algorithms in practice.
- On the Convergence of the Shapley Value in Parametric Bayesian Learning Games.
Lucas Agussurja, Xinyi Xu & Kian Hsiang Low.
In Proceedings of the 39th International Conference on Machine Learning (ICML-22), pages 180-196, Baltimore, MD, Jul 17-23, 2022.
21.9% acceptance rate
Abstract. Measuring contributions is a classical problem in cooperative game theory where the Shapley value is the most well-known solution concept. In this paper, we establish the convergence property of the Shapley value in parametric Bayesian learning games where players perform a Bayesian inference using their combined data, and the posterior-prior KL divergence is used as the characteristic function. We show that for any two players, under some regularity conditions, their difference in Shapley value converges in probability to the difference in Shapley value of a limiting game whose characteristic function is proportional to the log-determinant of the joint Fisher information. As an application, we present an online collaborative learning framework that is asymptotically Shapley-fair. Our result enables this to be achieved without any costly computations of posterior-prior KL divergences. Only a consistent estimator of the Fisher information is needed. The framework's effectiveness is demonstrated with experiments using real-world data.
- DAVINZ: Data Valuation using Deep Neural Networks at Initialization.
Zhaoxuan Wu, Yao Shu & Kian Hsiang Low.
In Proceedings of the 39th International Conference on Machine Learning (ICML-22), pages 24150-24176, Baltimore, MD, Jul 17-23, 2022.
21.9% acceptance rate
Abstract. Recent years have witnessed a surge of interest in developing trustworthy methods to evaluate the value of data in many real-world applications, e.g., collaborative machine learning, data marketplaces, etc. Existing data valuation methods typically valuate data using the generalization performance of converged machine learning models after their long-term model training, making data valuation on large complex deep neural networks (DNNs) unaffordable. To this end, we theoretically derive a domain-aware generalization bound to estimate the generalization performance of DNNs without model training. We then exploit this theoretically derived generalization bound to develop a novel training-free data valuation method named data valuation at initialization (DAVINZ) on DNNs, which consistently achieves remarkable effectiveness and efficiency in practice. Moreover, our training-free DAVINZ, surprisingly, can even theoretically and empirically enjoy the desirable properties that training-based data valuation methods usually attain, making it more trustworthy in practice.
- Efficient Distributionally Robust Bayesian Optimization with Worst-case Sensitivity.
Sebastian Tay, Chuan-Sheng Foo, Urano Daisuke, Richalynn Leong & Kian Hsiang Low.
In Proceedings of the 39th International Conference on Machine Learning (ICML-22), pages 21180-21204, Baltimore, MD, Jul 17-23, 2022.
21.9% acceptance rate
Abstract. In distributionally robust Bayesian optimization (DRBO), an exact computation of the worst-case expected value requires solving an expensive convex optimization problem. We develop a fast approximation of the worst-case expected value based on the notion of worst-case sensitivity that caters to arbitrary convex distribution distances. We provide a regret bound for our novel DRBO algorithm with the fast approximation, and empirically show it is competitive with that using the exact worst-case expected value while incurring significantly less computation time. In order to guide the choice of distribution distance to be used with DRBO, we show that our approximation implicitly optimizes an objective close to an interpretable risk-sensitive value.
- Bayesian Optimization under Stochastic Delayed Feedback.
Arun Verma, Zhongxiang Dai & Kian Hsiang Low.
In Proceedings of the 39th International Conference on Machine Learning (ICML-22), pages 22145-22167, Baltimore, MD, Jul 17-23, 2022.
21.9% acceptance rate
Abstract. Bayesian optimization (BO) is a widely-used sequential method for zeroth-order optimization of complex and expensive-to-compute black-box functions. The existing BO methods assume that the function evaluation (feedback) is available to the learner immediately or after a fixed delay. Such assumptions may not be practical in many real-life problems like clinical trials, online recommendations, and hyper-parameters tuning, where feedback is available after a random delay. To benefit from the experimental parallelization in these problems, the learner needs to start new function evaluations without waiting for delayed feedback. In this paper, we consider the BO under stochastic delayed feedback problem. We propose algorithms with sub-linear regret guarantees that efficiently address the dilemma of selecting new function queries while waiting for randomly delayed feedback. Building on our results, we also make novel contributions to batch BO and contextual Gaussian process bandits. Our experiments on synthetic and real-life datasets verify the performance of proposed algorithms.
- Data Valuation in Machine Learning: "Ingredients", Strategies, and Open Challenges.
Rachael Hwee Ling Sim, Xinyi Xu
& Kian Hsiang Low.
In Proceedings of the 31st International Joint Conference on Artificial Intelligence (IJCAI-22), pages 5607-5614, Vienna, Austria, Jul 23-29, 2022.
18.2% acceptance rate
Abstract. Data valuation in machine learning (ML) is an emerging research area that studies the worth of data in ML. Data valuation is used in collaborative ML to determine a fair compensation for every data owner and in interpretable ML to identify the most responsible, noisy, or misleading training examples. This paper presents a comprehensive technical survey that provides a new formal study of data valuation in ML through its "ingredients" and the corresponding properties, grounds the discussion of common desiderata satisfied by existing data valuation strategies on our proposed ingredients, and identifies open research challenges for designing new ingredients, data valuation strategies, and cost reduction techniques.
- Markov Chain Monte Carlo-Based Machine Unlearning: Unlearning What Needs to be Forgotten.
Quoc Phong Nguyen, Ryutaro Oikawa, Dinil Mon Divakaran, Mun Choon Chan & Kian Hsiang Low.
In Proceedings of the 17th ACM ASIA Conference on Computer and Communications Security (ACM ASIACCS'22), pages 351-363, Nagasaki, Japan, May 30 - Jun 3, 2022.
18.4% acceptance rate
Abstract. As the use of machine learning (ML) models is becoming increasingly popular in many real-world applications, there are practical challenges that need to be addressed for model maintenance. One such challenge is to 'undo' the effect of a specific subset of dataset used for training a model. This specific subset may contain malicious or adversarial data injected by an attacker, which affects the model performance. Another reason may be the need for a service provider to remove data pertaining to a specific user to respect the user's privacy. In both cases, the problem is to 'unlearn' a specific subset of the training data from a trained model without incurring the costly procedure of retraining the whole model from scratch. Towards this goal, this paper presents a Markov chain Monte Carlo-based machine unlearning (MCU) algorithm. MCU helps to effectively and efficiently unlearn a trained model from subsets of training dataset. Furthermore, we show that with MCU, we are able to explain the effect of a subset of a training dataset on the model prediction. Thus, MCU is useful for examining subsets of data to identify the adversarial data to be removed. Similarly, MCU can be used to erase the lineage of a user's personal data from trained ML models, thus upholding a user's "right to be forgotten". We empirically evaluate the performance of our proposed MCU algorithm on real-world phishing and diabetes datasets. Results show that MCU can achieve a desirable performance by efficiently removing the effect of a subset of training dataset and outperform an existing algorithm that utilizes the remaining dataset.
- NASI: Label- and Data-agnostic Neural Architecture Search at Initialization.
Yao Shu, Shaofeng Cai,
Zhongxiang Dai, Beng Chin Ooi & Kian Hsiang Low.
In Proceedings of the 10th International Conference on Learning Representations (ICLR-22), Apr 25 - 29, 2022.
32.29% acceptance rate
Abstract. Recent years have witnessed a surging interest in Neural Architecture Search (NAS). Various algorithms have been proposed to improve the search efficiency and effectiveness of NAS, i.e., to reduce the search cost and improve the generalization performance of the selected architectures, respectively. However, the search efficiency of these algorithms is severely limited by the need for model training during the search process. To overcome this limitation, we propose a novel NAS algorithm called NAS at Initialization (NASI) that exploits the capability of a Neural Tangent Kernel in being able to characterize the performance of candidate architectures at initialization, hence allowing model training to be completely avoided to boost the search efficiency. Besides the improved search efficiency, NASI also achieves competitive search effectiveness on various datasets like CIFAR-10/100 and ImageNet. Further, NASI is shown to be label- and data-agnostic under mild conditions, which guarantees the transferability of architectures selected by our NASI over different datasets.
- Near-Optimal Task Selection for Meta-Learning with Mutual
Information and Online Variational Bayesian Unlearning.
Yizhou Chen,
Shizhuo Zhang & Kian Hsiang Low.
In Proceedings of the 25th International Conference on Artificial Intelligence and Statistics (AISTATS-22), pages 9091-9113, Mar 28 - 30, 2022.
29.2% acceptance rate
Abstract. This paper addresses the problem of active task selection which involves selecting the most informative tasks for meta-learning. We propose a novel active task selection criterion based on the mutual information between latent task vectors. Unfortunately, such a criterion scales poorly in the number of candidate tasks when optimized. To resolve this issue, we exploit the submodularity property of our new criterion for devising the first active task selection algorithm for meta-learning with a near-optimal performance guarantee. To further improve the efficiency of our algorithm, we propose an online variant of the Stein variational gradient descent to perform fast belief updates of the meta-parameters via maintaining a set of forward (and backward) particles when learning (or unlearning) from each selected task. We empirically demonstrate the performance of our proposed algorithm on real-world datasets.
- Incentivizing Collaboration in Machine Learning via Synthetic Data Rewards.
Sebastian Tay,
Xinyi Xu, Chuan-Sheng Foo & Kian Hsiang Low.
In Proceedings of the 36th AAAI Conference on Artificial Intelligence (AAAI-22), pages 9448-9456, Feb 22 - Mar 1, 2022.
4.26% acceptance rate (oral presentation)
Abstract. This paper presents a novel collaborative generative modeling (CGM) framework that incentivizes collaboration among self-interested parties to contribute data to a pool for training a generative model (e.g., GAN), from which synthetic data are drawn and distributed to the parties as rewards commensurate to their contributions. Distributing synthetic data as rewards (instead of trained models or money) offers task- and model-agnostic benefits for downstream learning tasks and is less likely to violate data privacy regulation. To realize the framework, we firstly propose a data valuation function using maximum mean discrepancy (MMD) that values data based on its quantity and quality in terms of its closeness to the true data distribution and provide theoretical results guiding the kernel choice in our MMD-based data valuation function. Then, we formulate the reward scheme as a linear optimization problem that when solved, guarantees certain incentives such as fairness in the CGM framework. We devise a weighted sampling algorithm for generating synthetic data to be distributed to each party as reward such that the value of its data and the synthetic data combined matches its assigned reward value by the reward scheme. We empirically show using simulated and real-world datasets that the parties' synthetic data rewards are commensurate to their contributions.
- Differentially Private Federated Bayesian Optimization with Distributed Exploration.
Zhongxiang Dai, Kian Hsiang Low & Patrick Jaillet.
In Advances in Neural Information Processing Systems 34: 35th Annual Conference on Neural Information Processing Systems (NeurIPS'21), pages 9125-9139, Dec 6-14, 2021.
25.6% acceptance rate
Abstract. Bayesian optimization (BO) has recently been extended to the federated learning (FL) setting by the federated Thompson sampling (FTS) algorithm. However, FTS is not equipped with a rigorous privacy guarantee which is an important consideration in FL. Recent works have incorporated differential privacy (DP) into the training of deep neural networks through a general framework for adding DP to iterative algorithms. Following this general DP framework, our work here integrates DP into FTS to preserve user-level privacy. We also leverage the ability of this general DP framework to handle different parameter vectors, as well as the technique of local modeling for BO, to further improve the utility of our algorithm through distributed exploration (DE). The resulting differentially private FTS with DE (DP-FTS-DE) algorithm is endowed with theoretical guarantees for both the privacy and utility and is amenable to interesting theoretical insights about the privacy-utility trade-off. We also use real-world experiments to show that DP-FTS-DE achieves high utility (competitive performance) with a strong privacy guarantee (small privacy loss) and induces a practical trade-off between privacy and utility.
- Optimizing Conditional Value-At-Risk of Black-Box Functions.
Quoc Phong Nguyen, Zhongxiang Dai, Kian Hsiang Low & Patrick Jaillet.
In Advances in Neural Information Processing Systems 34: 35th Annual Conference on Neural Information Processing Systems (NeurIPS'21), pages 4170-4180, Dec 6-14, 2021.
25.6% acceptance rate
Abstract. This paper presents two Bayesian optimization (BO) algorithms with theoretical performance guarantee to maximize the conditional value-at-risk (CVaR) of a black-box function: CV-UCB and CV-TS which are based on the well-established principle of optimism in the face of uncertainty and Thompson sampling, respectively. To achieve this, we develop an upper confidence bound of CVaR and prove the no-regret guarantee of CV-UCB by utilizing an interesting connection between CVaR and value-at-risk (VaR). For CV-TS, though it is straightforwardly performed with Thompson sampling, bounding its Bayesian regret is non-trivial because it requires a tail expectation bound for the distribution of CVaR of a black-box function, which has not been shown in the literature. The performances of both CV-UCB and CV-TS are empirically evaluated in optimizing CVaR of synthetic benchmark functions and simulated real-world optimization problems.
- Fault-Tolerant Federated Reinforcement Learning with Theoretical Guarantee.
Xiaofeng Fan, Yining Ma, Zhongxiang Dai, Wei Jing, Cheston Tan & Kian Hsiang Low.
In Advances in Neural Information Processing Systems 34: 35th Annual Conference on Neural Information Processing Systems (NeurIPS'21), pages 1007-1021, Dec 6-14, 2021.
25.6% acceptance rate
Abstract. The growing literature of Federated Learning (FL) has recently inspired Federated Reinforcement Learning (FRL) to encourage multiple agents to federatively build a better decision-making policy without sharing raw trajectories. Despite its promising applications, existing FRL work fails to I) provide theoretical analysis on its convergence; II) account for random system failures and adversarial attacks. Towards this end, we propose the first FRL framework, the convergence of which is tolerant to less than half of participating agents being random system failures or adversarial attackers. We prove that the sample efficiency of the proposed framework is guaranteed to scale with the number of agents, accounting for such potential failures or attacks. We empirically verify all theoretical results on various RL benchmarking tasks.
- Gradient Driven Rewards to Guarantee Fairness in Collaborative Machine Learning.
Xinyi Xu, Lingjuan Lyu, Xingjun Ma, Chenglin Miao, Chuan-Sheng Foo & Kian Hsiang Low.
In Advances in Neural Information Processing Systems 34: 35th Annual Conference on Neural Information Processing Systems (NeurIPS'21), pages 16104-16117, Dec 6-14, 2021.
25.6% acceptance rate
Abstract. In collaborative machine learning (CML), multiple agents pool their resources (e.g., data) together for a common learning task. In realistic CML settings where the agents are self-interested and not altruistic, they may be unwilling to share data or model information without adequate rewards. Furthermore, as the data/model information shared by the agents may differ in quality, designing rewards which are fair to them is important so that they would not feel exploited nor discouraged from sharing. In this paper, we adopt federated learning as the CML paradigm, propose a novel cosine gradient Shapley value (CGSV) to fairly evaluate the expected marginal contribution of each agent's uploaded model parameter update/gradient without needing an auxiliary validation dataset, and based on the CGSV, design a novel training-time gradient reward mechanism with a fairness guarantee by sparsifying the aggregated parameter update/gradient downloaded from the server as reward to each agent such that its resulting quality is commensurate to that of the agent’s uploaded parameter update/gradient. We empirically demonstrate the effectiveness of our fair gradient reward mechanism on multiple benchmark datasets in terms of fairness, predictive performance, and time overhead.
- Validation Free and Replication Robust Volume-based Data Valuation.
Xinyi Xu, Zhaoxuan Wu, Chuan-Sheng Foo & Kian Hsiang Low.
In Advances in Neural Information Processing Systems 34: 35th Annual Conference on Neural Information Processing Systems (NeurIPS'21), pages 10837-10848, Dec 6-14, 2021.
25.6% acceptance rate
Abstract. Data valuation arises as a non-trivial challenge in use cases such as collaborative data sharing, data markets and etc. The value of data is often related to the learning performance, e.g. validation accuracy, of the model trained on the data. While intuitive, this methodology introduces a high coupling between data valuation and validation, which may not be desirable in practice. For instance, data providers may disagree on the choice of the validation set, or the validation set may be (statistically) different from the actual application. A separate but practical issue is data replication. If some data points are valuable, a dishonest data provider may offer a dataset containing replications of these data points, trying to exploit the valuation to get a higher reward/payment. Based on the ordinary least squares framework, our data valuation method does not require validation, and still provides a useful connection between the value of data and learning performance. In particular, we utilize the volume of the data matrix (determinant of its left Gram), thus able to provide an intuitive interpretation of the value of data via the diversity in the data. Furthermore, we formalize the robustness to data replication, and propose a robust volume valuation with robustness guarantees. We conduct extensive experiments to demonstrate its consistency and practical advantages over existing baselines.
- Learning to Learn with Gaussian Processes.
Quoc Phong Nguyen, Kian Hsiang Low & Patrick Jaillet.
In Proceedings of the 37th Conference on Uncertainty in Artificial Intelligence (UAI-21), pages 1466-1475, Jul 27-30, 2021.
26.5% acceptance rate
Abstract. This paper presents Gaussian process meta-learning (GPML) for few-shot regression, which explicitly exploits the distance between regression problems/tasks using a novel task kernel. It contrasts sharply with the popular metric-based meta-learning approach which is based on the distance between data inputs or their embeddings in the few-shot learning literature. Apart from the superior predictive performance by capturing the diversity of different tasks, GPML offers a set of representative tasks that are useful for understanding the task distribution. We empirically demonstrate the performance and interpretability of GPML in several few-shot regression problems involving a multimodal task distribution and real-world datasets.
- Trusted-Maximizers Entropy Search for Efficient Bayesian Optimization.
Quoc Phong Nguyen, Zhaoxuan Wu, Kian Hsiang Low & Patrick Jaillet.
In Proceedings of the 37th Conference on Uncertainty in Artificial Intelligence (UAI-21), pages 1486-1495, Jul 27-30, 2021.
26.5% acceptance rate
Abstract. Information-based Bayesian optimization (BO) algorithms have achieved state-of-the-art performance in optimizing a black-box objective function. However, they usually require several approximations or simplifying assumptions (without clearly understanding their effects on the BO performance) and/or their generalization to batch BO is computationally unwieldy, especially with an increasing batch size. To alleviate these issues, this paper presents a novel trusted-maximizers entropy search (TES) acquisition function: It measures how much an input query contributes to the information gain on the maximizer over a finite set of trusted maximizers, i.e., inputs optimizing functions that are sampled from the Gaussian process posterior belief of the objective function. Evaluating TES requires either only a stochastic approximation with sampling or a deterministic approximation with expectation propagation, both of which are investigated and empirically evaluated using synthetic benchmark objective functions and real-world optimization problems, e.g., hyperparameter tuning of a convolutional neural network and synthesizing ‘physically realizable’ faces to fool a black-box face recognition system. Though TES can naturally be generalized to a batch variant with either approximation, the latter is amenable to be scaled to a much larger batch size in our experiments.
- Collaborative Bayesian Optimization with Fair Regret.
Rachael Hwee Ling Sim, Yehong Zhang, Kian Hsiang Low & Patrick Jaillet.
In Proceedings of the 38th International Conference on Machine Learning (ICML-21), pages 9691-9701, Jul 18-24, 2021.
21.5% acceptance rate
Abstract. Bayesian optimization (BO) is a popular tool for optimizing complex and costly-to-evaluate black-box objective functions. To further reduce the number of function evaluations, any party performing BO may be interested to collaborate with others to optimize the same objective function concurrently. To do this, existing BO algorithms have considered optimizing a batch of input queries in parallel and provided theoretical bounds on their cumulative regret reflecting inefficiency. However, when the objective function values are correlated with real-world rewards (e.g., money), parties may be hesitant to collaborate if they risk incurring larger cumulative regret (i.e., smaller real-world reward) than others. This paper shows that fairness and efficiency are both necessary for the collaborative BO setting. Inspired by social welfare concepts from economics, we propose a new notion of regret capturing these properties and a collaborative BO algorithm whose convergence rate can be theoretically guaranteed by bounding the new regret, both of which share an adjustable parameter for trading off between fairness vs. efficiency. We empirically demonstrate the benefits (e.g., increased fairness) of our algorithm using synthetic and real-world datasets.
- Model Fusion for Personalized Learning.
Chi Thanh Lam, Trong Nghia Hoang, Kian Hsiang Low & Patrick Jaillet.
In Proceedings of the 38th International Conference on Machine Learning (ICML-21), pages 5948-5958, Jul 18-24, 2021.
21.5% acceptance rate
Abstract. Production systems operating on a growing domain of analytic services often require generating warm-start solution models for emerging tasks with limited data. One potential approach to address this challenge is to adopt meta learning to generate a base model that can be adapted to solve unseen tasks with minimal fine-tuning. This however requires the training processes of previous solution models of existing tasks to be synchronized. This is not possible if these models were pre-trained separately on private data owned by different entities and cannot be synchronously re-trained. To accommodate for such scenarios, we develop a new personalized learning framework that synthesizes customized models for unseen tasks via fusion of independently pre-trained models of related tasks. We establish performance guarantee for the proposed framework and demonstrate its effectiveness on both synthetic and real datasets.
- Value-at-Risk Optimization with Gaussian Processes.
Quoc Phong Nguyen, Zhongxiang Dai, Kian Hsiang Low & Patrick Jaillet.
In Proceedings of the 38th International Conference on Machine Learning (ICML-21), pages 8063-8072, Jul 18-24, 2021.
21.5% acceptance rate
Abstract. Value-at-risk (VaR) is an established measure to assess risks in critical real-world applications with random environmental factors. This paper presents a novel VaR upper confidence bound (V-UCB) algorithm for maximizing the VaR of a black-box objective function with the first no-regret guarantee. To realize this, we first derive a confidence bound of VaR and then prove the existence of values of the environmental random variable (to be selected to achieve no regret) such that the confidence bound of VaR lies within that of the objective function evaluated at such values. Our V-UCB algorithm empirically demonstrates state-of-the-art performance in optimizing synthetic benchmark functions, a portfolio optimization problem, and a simulated robot task.
- AID: Active Distillation Machine to Leverage Pre-Trained Black-Box Models in Private Data Settings.
Trong Nghia Hoang, Shenda Hong, Cao Xiao, Kian Hsiang Low & Jimeng Sun.
In Proceedings of the 30th The Web Conference (WWW'21), pages 3569–3581, Apr 19-23, 2021.
20.6% acceptance rate
Abstract. This paper presents an active distillation method for a local institution (e.g., hospital) to find the best queries within its given budget to distill an on-server black-box model's predictive knowledge into a local surrogate with transparent parameterization. This allows local institutions to understand better the predictive reasoning of the black-box model in its own local context or to further customize the distilled knowledge with its private dataset that cannot be centralized and fed into the server model. The proposed method thus addresses several challenges of deploying machine learning in many industrial settings (e.g., healthcare analytics) with strong proprietary constraints. These include: (1) the opaqueness of the server model’s architecture which prevents local users from understanding its predictive reasoning in their local data contexts; (2) the increasing cost and risk of uploading local data on the cloud for analysis; and (3) the need to customize the server model with private onsite data. We evaluated the proposed method on both benchmark and real-world healthcare data where significant improvements over existing local distillation methods were observed. A theoretical analysis of the proposed method is also presented.
- Top-k Ranking Bayesian Optimization.
Quoc Phong Nguyen, Sebastian Tay, Kian Hsiang Low & Patrick Jaillet.
In Proceedings of the 35th AAAI Conference on Artificial Intelligence (AAAI-21), pages 9135-9143, Feb 2-9, 2021.
21.4% acceptance rate
Abstract. This paper presents a novel approach to top-k ranking Bayesian optimization (top-k ranking BO) which is a practical and significant generalization of preferential BO to handle top-k ranking and tie/indifference observations. We first design a surrogate model that is not only capable of catering to the above observations, but is also supported by a classic random utility model. Another equally important contribution is the introduction of the first information-theoretic acquisition function in BO with preferential observation called multinomial predictive entropy search (MPES) which is flexible in handling these observations and optimized for all inputs of a query jointly. MPES possesses superior performance compared with existing acquisition functions that select the inputs of a query one at a time greedily. We empirically evaluate the performance of MPES using several synthetic benchmark functions, CIFAR-10 dataset, and SUSHI preference dataset.
- An Information-Theoretic Framework for Unifying Active Learning Problems.
Quoc Phong Nguyen, Kian Hsiang Low & Patrick Jaillet.
In Proceedings of the 35th AAAI Conference on Artificial Intelligence (AAAI-21), pages 9126-9134, Feb 2-9, 2021.
21.4% acceptance rate
Abstract. This paper presents an information-theoretic framework for unifying active learning problems: level set estimation (LSE), Bayesian optimization (BO), and their generalized variant. We first introduce a novel active learning criterion that subsumes an existing LSE algorithm and achieves state-of-the-art performance in LSE problems with a continuous input domain. Then, by exploiting the relationship between LSE and BO, we design a competitive information-theoretic acquisition function for BO that has interesting connections to upper confidence bound and max-value entropy search (MES). The latter connection reveals a drawback of MES which has important implications on not only MES but also on other MES-based acquisition functions. Finally, our unifying information-theoretic framework can be applied to solve a generalized problem of LSE and BO involving multiple level sets in a data-efficient manner. We empirically evaluate the performance of our proposed algorithms using synthetic benchmark functions, a real-world dataset, and in hyperparameter tuning of machine learning models.
- Convolutional Normalizing Flows for Deep Gaussian Processes.
Haibin Yu, Dapeng Liu, Kian Hsiang Low & Patrick Jaillet.
In Proceedings of the International Joint Conference on Neural Networks (IJCNN'21), Jul 18-22, 2021.
Abstract. Deep Gaussian processes (DGPs), a hierarchical composition of GP models, have successfully boosted the expressive power of their single-layer counterpart. However, it is impossible to perform exact inference in DGPs, which has motivated the recent development of variational inference-based methods. Unfortunately, either these methods yield a biased posterior belief or it is difficult to evaluate their convergence. This paper introduces a new approach for specifying flexible, arbitrarily complex, and scalable approximate posterior distributions. The posterior distribution is constructed through a normalizing flow (NF) which transforms a simple initial probability into a more complex one through a sequence of invertible transformations. Moreover, a novel convolutional normalizing flow (CNF) is developed to improve the time efficiency and capture dependency between layers. Empirical evaluation shows that CNF DGP outperforms the state-of-the-art approximation methods for DGPs.
- Efficient Exploration of Reward Functions in Inverse Reinforcement Learning via Bayesian Optimization.
Sreejith Balakrishnan, Quoc Phong Nguyen, Kian Hsiang Low & Harold Soh.
In Advances in Neural Information Processing Systems 33: 34th Annual Conference on Neural Information Processing Systems (NeurIPS'20), pages 4187-4198, Dec 6-12, 2020.
20.1% acceptance rate
Abstract. In this paper, we focus on the problem of Inverse Reinforcement Learning (IRL), which is relevant for a variety of tasks including value alignment and robot learning from demonstration. Despite significant algorithmic contributions in recent years, IRL remains an ill-posed problem at its core; multiple reward functions coincide with the observed behavior, and the actual reward function is not identifiable without prior knowledge or supplementary information. Here, we propose Bayesian Optimization-IRL (BO-IRL), an IRL framework that identifies multiple solutions that are consistent with the expert demonstrations by efficiently exploring the reward function space. BO-IRL achieves this by utilizing Bayesian Optimization along with our newly proposed kernel that (a) projects the parameters of policy invariant reward functions to a single point in a latent space, and (b) ensures that nearby points in the latent space correspond to reward functions that yield similar likelihoods. This projection allows for the use of standard stationary kernels in the latent space to capture the correlations present across the reward function space. Empirical results on synthetic and real-world environments (model-free and model-based) show that BO-IRL discovers multiple reward functions while minimizing the number of expensive exact policy optimizations.
- Federated Bayesian Optimization via Thompson Sampling.
Zhongxiang Dai, Kian Hsiang Low & Patrick Jaillet.
In Advances in Neural Information Processing Systems 33: 34th Annual Conference on Neural Information Processing Systems (NeurIPS'20), pages 9687-9699, Dec 6-12, 2020.
20.1% acceptance rate
Abstract. Bayesian optimization (BO) is a prominent method for optimizing expensive-to-compute black-box functions. The massive computational capability of edge devices such as mobile phones, coupled with privacy concerns, has led to immense recent interest in federated learning (FL), which focuses on collaborative training of deep neural networks (DNN) via first-order optimization techniques. However, some common machine learning tasks such as hyperparameter tuning of DNN lack access to gradients and thus require zeroth-order optimization (black-box optimization). This hints at the considerable potential of extending BO to the FL setting (FBO), to allow agents to collaborate in these black-box optimization tasks. Here, we introduce federated Thompson sampling (FTS), which overcomes a number of key challenges of FBO and FL in a principled way: We (a) use random Fourier features to approximate the Gaussian process surrogate model used in BO which naturally produces the parameters to be exchanged between agents, (b) design FTS based on Thompson sampling which significantly reduces the number of parameters to be exchanged, and (c) provide a theoretical convergence guarantee that is robust against heterogeneous agents which is a major challenge in FL and FBO. We empirically demonstrate the effectiveness of FTS in terms of communication efficiency, computational efficiency and practical performance.
- Variational Bayesian Unlearning.
Quoc Phong Nguyen, Kian Hsiang Low & Patrick Jaillet.
In Advances in Neural Information Processing Systems 33: 34th Annual Conference on Neural Information Processing Systems (NeurIPS'20), pages 16025-16036, Dec 6-12, 2020.
20.1% acceptance rate
Abstract. This paper studies the problem of approximately unlearning a Bayesian model from a small subset of the training data to be erased. We frame this problem as one of minimizing the Kullback-Leibler distance between the approximate posterior belief of model parameters after directly unlearning from the erased data vs. the exact posterior belief from retraining with remaining data. Using the variational inference (VI) framework, we show that it is equivalent to minimizing an evidence upper bound which trades off between fully unlearning from erased data vs. not entirely forgetting the posterior belief given the full data (i.e., including the remaining data); the latter prevents catastrophic unlearning that can render the model useless. In model training with VI, only an approximate (instead of exact) posterior belief given the full data can be obtained, which makes unlearning even more challenging. We propose two novel tricks to tackle this challenge. We empirically demonstrate our unlearning methods on Bayesian models such as sparse Gaussian process and logistic regression using synthetic and real-world datasets.
- Collaborative Machine Learning with Incentive-Aware Model Rewards.
Rachael Hwee Ling Sim, Yehong Zhang, Mun Choon Chan & Kian Hsiang Low.
In Proceedings of the 37th International Conference on Machine Learning (ICML-20), pages 8927-8936, Jun 12-18, 2020.
21.8% acceptance rate
Abstract. Collaborative machine learning (ML) is an appealing paradigm to build high-quality ML models by training on the aggregated data from many parties. However, these parties are only willing to share their data when given enough incentives, such as a guaranteed fair reward based on their contributions. This motivates the need for measuring a party's contribution and designing an incentive-aware reward scheme accordingly. This paper proposes to value a party's reward based on Shapley value and information gain on model parameters given its data. Subsequently, we give each party a model as a reward. To formally incentivize the collaboration, we define some desirable properties (e.g., fairness and stability) which are inspired by cooperative game theory but adapted for our model reward that is uniquely freely replicable. Then, we propose a novel model reward scheme to satisfy fairness and trade off between the desirable properties via an adjustable parameter. The value of each party's model reward determined by our scheme is attained by injecting Gaussian noise to the aggregated training data with an optimized noise variance. We empirically demonstrate interesting properties of our scheme and evaluate its performance using synthetic and real-world datasets.
- R2-B2: Recursive Reasoning-Based Bayesian Optimization for No-Regret Learning in Games.
Zhongxiang Dai, Yizhou Chen, Kian Hsiang Low, Patrick Jaillet & Teck-Hua Ho.
In Proceedings of the 37th International Conference on Machine Learning (ICML-20), pages 2291-2301, Jun 12-18, 2020.
21.8% acceptance rate
Abstract. This paper presents a recursive reasoning formalism of Bayesian optimization (BO) to model the reasoning process in the interactions between boundedly rational, self-interested agents with unknown, complex, and costly-to-evaluate payoff functions in repeated games, which we call Recursive Reasoning-Based BO (R2-B2).
Our R2-B2 algorithm is general in that it does not constrain the relationship among the payoff functions of different agents and can thus be applied to various types of games such as constant-sum, general-sum, and common-payoff games. We prove that by reasoning at level 2 or more and at one level higher than the other agents, our R2-B2 agent can achieve faster asymptotic convergence to no regret than that without utilizing recursive reasoning. We also propose a computationally cheaper variant of R2-B2 called R2-B2-Lite at the expense of a weaker convergence guarantee. The performance and generality of our R2-B2 algorithm are empirically demonstrated using synthetic games, adversarial machine learning, and multi-agent reinforcement learning.
- Private Outsourced Bayesian Optimization.
Dmitrii Kharkovskii, Zhongxiang Dai & Kian Hsiang Low.
In Proceedings of the 37th International Conference on Machine Learning (ICML-20), pages 5231-5242, Jun 12-18, 2020.
21.8% acceptance rate
Abstract. This paper presents the private-outsourced-Gaussian process-upper confidence bound (PO-GP-UCB) algorithm, which is the first algorithm for privacy-preserving Bayesian optimization (BO) in the outsourced setting with a provable performance guarantee. We consider the outsourced setting where the entity holding the dataset and the entity performing BO are represented by different parties, and the dataset cannot be released non-privately. For example, a hospital holds a dataset of sensitive medical records and outsources the BO task on this dataset to an industrial AI company.
The key idea of our approach is to make the BO performance of our algorithm similar to that of non-private GP-UCB run using the original dataset, which is achieved by using a random projection-based transformation that preserves both privacy and the pairwise distances between inputs. Our main theoretical contribution is to show that a regret bound similar to that of the standard GP-UCB algorithm can be established for our PO-GP-UCB algorithm. We empirically evaluate the performance of our PO-GP-UCB algorithm with synthetic and real-world datasets.
- Learning Task-Agnostic Embedding of Multiple Black-Box Experts for Multi-Task Model Fusion.
Trong Nghia Hoang, Chi Thanh Lam, Kian Hsiang Low & Patrick Jaillet.
In Proceedings of the 37th International Conference on Machine Learning (ICML-20), pages 4282-4292, Jun 12-18, 2020.
21.8% acceptance rate
Abstract. Model fusion is an emerging study in collective learning where heterogeneous experts with private data and learning architectures need to combine their black-box knowledge for better performance. Existing literature achieves this via a local knowledge distillation scheme that transfuses the predictive patterns of each pre-trained expert onto a white-box imitator model, which can be incorporated efficiently into a global model. This scheme however does not extend to multi-task scenarios where different experts were trained to solve different tasks and only part of their distilled knowledge is relevant to a new task. To address this multi-task challenge, we develop a new fusion paradigm that represents each expert as a distribution over a spectrum of predictive prototypes, which are isolated from task-specific information encoded within the prototype distribution. The task-agnostic prototypes can then be reintegrated to generate a new model that solves a new task encoded with a different prototype distribution. The fusion and adaptation performance of the proposed framework is demonstrated empirically on several real-world benchmark datasets.
- Nonmyopic Gaussian Process Optimization with Macro-Actions.
Dmitrii Kharkovskii, Chun Kai Ling & Kian Hsiang Low.
In Proceedings of the 23rd International Conference on Artificial Intelligence and Statistics (AISTATS-20), pages 4593-4604, Aug 26-28, 2020.
28.7% acceptance rate
Abstract. This paper presents a multi-staged approach to nonmyopic adaptive Gaussian process optimization (GPO) for Bayesian optimization (BO) of unknown, highly complex objective functions that, in contrast to existing nonmyopic adaptive BO algorithms, exploits the notion of macro-actions for scaling up to a further lookahead to match up to a larger available budget. To achieve this, we generalize GP upper confidence bound to a new acquisition function defined w.r.t. a nonmyopic adaptive macro-action policy, which is intractable to be optimized exactly due to an uncountable set of candidate outputs. The contribution of our work here is thus to derive a nonmyopic adaptive ϵ-Bayes-optimal macro-action GPO (ϵ-Macro-GPO) policy. To perform nonmyopic adaptive BO in real time, we then propose an asymptotically optimal anytime variant of our ϵ-Macro-GPO policy with a performance guarantee. We empirically evaluate the performance of our ϵ-Macro-GPO policy and its anytime variant in BO with synthetic and real-world datasets.
- Scalable Variational Bayesian Kernel Selection for Sparse Gaussian Process Regression.
Tong Teng, Jie Chen, Yehong Zhang & Kian Hsiang Low.
In Proceedings of the 34th AAAI Conference on Artificial Intelligence (AAAI-20), pages 5997-6004, New York, NY, Feb 7-12, 2020.
20.6% acceptance rate
Abstract. This paper presents a variational Bayesian kernel selection (VBKS) algorithm for sparse Gaussian process regression (SGPR) models. In contrast to existing GP kernel selection algorithms that aim to select only one kernel with the highest model evidence, our proposed VBKS algorithm considers the kernel as a random variable and learns its belief from data such that the uncertainty of the kernel can be interpreted and exploited to avoid overconfident GP predictions. To achieve this, we represent the probabilistic kernel as an additional variational variable in a variational inference (VI) framework for SGPR models where its posterior belief is learned together with that of the other variational variables (i.e., inducing variables and kernel hyperparameters). In particular, we transform the discrete kernel belief into a continuous parametric distribution via reparameterization in order to apply VI. Though it is computationally challenging to jointly optimize a large number of hyperparameters due to many kernels being evaluated simultaneously by our VBKS algorithm, we show that the variational lower bound of the log-marginal likelihood can be decomposed into an additive form such that each additive term depends only on a disjoint subset of the variational variables and can thus be optimized independently. Stochastic optimization is then used to maximize the variational lower bound by iteratively improving the variational approximation of the exact posterior belief via stochastic gradient ascent, which incurs constant time per iteration and hence scales to big data. We empirically evaluate the performance of our VBKS algorithm on synthetic and massive real-world datasets.
- Gaussian Process Decentralized Data Fusion Meets Transfer Learning in Large-Scale Distributed Cooperative Perception.
Ruofei Ouyang & Kian Hsiang Low.
Autonomous Robots (Special Issue on Multi-Robot and Multi-Agent Systems), volume 44, issue 3, pages 359-376, Mar 2020.
Extended version of our
AAAI-18 paper
Abstract. This paper presents novel Gaussian process decentralized data fusion algorithms exploiting the notion of agent-centric support sets for distributed cooperative perception of large-scale environmental phenomena. To overcome the limitations of scale in existing works, our proposed algorithms allow every mobile sensing agent to utilize a different support set and dynamically switch to another during execution for encapsulating its own data into a local summary that, perhaps surprisingly, can still be assimilated with the other agents' local summaries (i.e., based on their current choices of support sets) into a globally consistent summary to be used for predicting the phenomenon. To achieve this, we propose a novel transfer learning mechanism for a team of agents capable of sharing and transferring information encapsulated in a summary based on a support set to that utilizing a different support set with some loss that can be theoretically bounded and analyzed. To alleviate the issue of information loss accumulating over multiple instances of transfer learning, we propose a new information sharing mechanism to be incorporated into our algorithms in order to achieve memory-efficient lazy transfer learning. Empirical evaluation on three real-world datasets for up to 128 agents show that our algorithms outperform the state-of-the-art methods.
- FCM-Sketch: Generic Network Measurements with Data Plane Support.
Cha Hwan Song, Pravein Govindan Kannan, Kian Hsiang Low & Mun Choon Chan.
In Proceedings of the 16th International Conference on emerging Networking EXperiments and Technologies (CoNEXT'20), pages 78-92, Dec 1-4, 2020.
24% acceptance rate
Abstract. Sketches have successfully provided accurate and fine-grained measurements (e.g., flow size and heavy hitters) which are imperative for network management. In particular, Count-Min (CM) sketch is widely utilized in many applications due to its simple design and ease of implementation. There have been many efforts to build monitoring frameworks based on Count-Min sketch. However, these frameworks either support very specific measurement tasks or they cannot be implemented on high-speed programmable hardware (PISA). In this work, we propose FCM, a framework that is designed to support generic network measurement with high accuracy. Our key contribution is FCM-Sketch, a data structure that has a lightweight implementation on the emerging PISA programmable switches. FCM-Sketch can also be used as a substitute for CM-Sketch in applications that use CM-Sketch. We have implemented FCM-Sketch on a commodity programmable switch (Barefoot Tofino) using the P4 language. Our evaluation shows that FCM-Sketch can reduce the errors in many measurement tasks by 50% to 80% compared to CM-Sketch and other state-of-the-art approaches.
- Implicit Posterior Variational Inference for Deep Gaussian Processes.
Haibin Yu, Yizhou Chen, Zhongxiang Dai, Kian Hsiang Low & Patrick Jaillet.
In Advances in Neural Information Processing Systems 32: 33rd Annual Conference on Neural Information Processing Systems (NeurIPS'19), pages 14475-14486, Vancouver, Canada, Dec 7-12, 2019.
3% acceptance rate (spotlight presentation)
Abstract. A multi-layer deep Gaussian process (DGP) model is a hierarchical composition of GP models with a greater expressive power. Exact DGP inference is intractable, which has motivated the recent development of deterministic and stochastic approximation methods. Unfortunately, the deterministic approximation methods yield a biased posterior belief while the stochastic one is computationally costly. This paper presents an implicit posterior variational inference (IPVI) framework for DGPs that can ideally recover an unbiased posterior belief and still preserve time efficiency. Inspired by generative adversarial networks, our IPVI framework achieves this by casting the DGP inference problem as a two-player game in which a Nash equilibrium, interestingly, coincides with an unbiased posterior belief. This consequently inspires us to devise a best-response dynamics algorithm to search for a Nash equilibrium (i.e., an unbiased posterior belief). Empirical evaluation shows that IPVI outperforms the state-of-the-art approximation methods for DGPs.
- Bayesian Optimization with Binary Auxiliary Information.
Yehong Zhang, Zhongxiang Dai & Kian Hsiang Low.
In Proceedings of the 35th Conference on Uncertainty in Artificial Intelligence (UAI-19), pages 1222-1232, Tel Aviv, Israel, Jul 22-25, 2019.
26.2% acceptance rate (plenary talk)
Subsumes our work on Information-Based Multi-Fidelity Bayesian Optimization presented in
NeurIPS'17 Workshop on Bayesian Optimization, Long Beach, CA, Dec 9, 2017.
Abstract. This paper presents novel mixed-type Bayesian optimization (BO) algorithms to accelerate the optimization of a target objective function by exploiting correlated auxiliary information of binary type that can be more cheaply obtained, such as in policy search for reinforcement learning and hyperparameter tuning of machine learning models with early stopping. To achieve this, we first propose a mixed-type multi-output Gaussian process (MOGP) to jointly model the continuous target function and binary auxiliary functions. Then, we propose information-based acquisition functions such as mixed-type entropy search (MT-ES) and mixed-type predictive ES (MT-PES) for mixed-type BO based on the MOGP predictive belief of the target and auxiliary functions. The exact acquisition functions of MT-ES and MT-PES cannot be computed in closed form and need to be approximated. We derive an efficient approximation of MT-PES via a novel mixed-type random features approximation of the MOGP model whose cross-correlation structure between the target and auxiliary functions can be exploited for improving the belief of the global target maximizer using the observations from evaluating these functions. We also propose new practical constraints to relate the global target maximizer to the binary auxiliary functions. We empirically evaluate the performance of MT-ES and MT-PES with synthetic and real-world experiments.
- Bayesian Optimization Meets Bayesian Optimal Stopping.
Zhongxiang Dai, Haibin Yu, Kian Hsiang Low & Patrick Jaillet.
In Proceedings of the 36th International Conference on Machine Learning (ICML-19), pages 1496-1506, Long Beach, CA, Jun 9-15, 2019.
22.6% acceptance rate
Abstract. Bayesian optimization (BO) is a popular paradigm for optimizing the hyperparameters of machine learning (ML) models due to its sample efficiency. Many ML models require running an iterative training procedure (e.g., stochastic gradient descent). This motivates the question whether information available during the training process (e.g., validation accuracy after each epoch) can be exploited for improving the epoch efficiency of BO algorithms by early-stopping model training under hyperparameter settings that will end up under-performing and hence eliminating unnecessary training epochs. This paper proposes to unify BO (specifically, Gaussian process-upper confidence bound (GP-UCB)) with Bayesian optimal stopping (BO-BOS) to boost the epoch efficiency of BO. To achieve this, while GP-UCB is sample-efficient in the number of function evaluations, BOS complements it with epoch efficiency for each function evaluation by providing a principled optimal stopping mechanism for early stopping. BO-BOS preserves the (asymptotic) no-regret performance of GP-UCB using our specified choice of BOS parameters that is amenable to an elegant interpretation in terms of the exploration-exploitation trade-off. We empirically evaluate the performance of BO-BOS and demonstrate its generality in hyperparameter optimization of ML models and two other interesting applications.
- Collective Model Fusion for Multiple Black-Box Experts.
Quang Minh Hoang, Trong Nghia Hoang, Kian Hsiang Low & Carleton Kingsford.
In Proceedings of the 36th International Conference on Machine Learning (ICML-19), pages 2742-2750, Long Beach, CA, Jun 9-15, 2019.
22.6% acceptance rate
Abstract. Model fusion is a fundamental problem in collective machine learning (ML) where independent experts with heterogeneous learning architectures are required to combine expertise to improve predictive performance. This is particularly challenging in information-sensitive domains (e.g., medical records in health-care analytics) where experts do not have access to each other's internal architecture and local data. To address this challenge, this paper presents the first collective model fusion framework for multiple experts with heterogeneous black-box architectures. The proposed method will enable this by addressing the following key issues of how black-box experts interact to understand the predictive behaviors of one another; how these understandings can be represented and shared efficiently among themselves; and how the shared understandings can be combined to generate high-quality consensus prediction. The performance of the resulting framework is analyzed theoretically and demonstrated empirically on several datasets.
- Collective Online Learning of Gaussian Processes in Massive Multi-Agent Systems.
Trong Nghia Hoang, Quang Minh Hoang, Kian Hsiang Low & Jonathan P. How.
In Proceedings of the 33rd AAAI Conference on Artificial Intelligence (AAAI-19), pages 7850-7857, Honolulu, HI, Jan 27-Feb 1, 2019.
16.2% acceptance rate (oral presentation)
Abstract. This paper presents a novel Collective Online Learning of Gaussian Processes (COOL-GP) framework for enabling a massive number of GP inference agents to simultaneously perform (a) efficient online updates of their GP models using their local streaming data with varying correlation structures and (b) decentralized fusion of their resulting online GP models with different learned hyperparameter settings and inducing inputs. To realize this, we exploit the notion of a common encoding structure to encapsulate the local streaming data gathered by any GP inference agent into summary statistics based on our proposed representation, which is amenable to both an efficient online update via an importance sampling trick as well as multi-agent model fusion via decentralized message passing that can exploit sparse connectivity among agents for improving efficiency and enhance the robustness of our framework against transmission loss. We provide a rigorous theoretical analysis of the approximation loss arising from our proposed representation to achieve efficient online updates and model fusion. Empirical evaluations show that COOL-GP is highly effective in model fusion, resilient to information disparity between agents, robust to transmission loss, and can scale to thousands of agents.
- Towards Robust ResNet: A Small Step but a Giant Leap.
Jingfeng Zhang, Bo Han,
Laura Wynter, Kian Hsiang Low & Mohan Kankanhalli.
In Proceedings of the 28th International Joint Conference on Artificial Intelligence (IJCAI-19), pages 4285-4291, Macao, Aug 10-16, 2019.
17.9% acceptance rate
Abstract. This paper presents a simple yet principled approach to boosting the robustness of the residual network (ResNet) that is motivated by the dynamical system perspective. Namely, a deep neural network can be interpreted using a partial differential equation, which naturally inspires us to characterize ResNet by an explicit Euler method. Our analytical studies reveal that the step factor h in the Euler method is able to control the robustness of ResNet in both its training and generalization. Specifically, we prove that a small step factor h can benefit the training robustness for back-propagation; from the view of forward-propagation, a small h can aid in the robustness of the model generalization. A comprehensive empirical evaluation on both vision CIFAR-10 and text AG-NEWS datasets confirms that a small h aids both the training and generalization robustness.
- GEE: A Gradient-based Explainable Variational Autoencoder for Network Anomaly Detection.
Quoc Phong Nguyen, Kar Wai Lim, Dinil Mon Divakaran, Kian Hsiang Low & Mun Choon Chan.
In Proceedings of the IEEE Conference on Communications and Network Security (CNS'19), pages 91-99, Washington, DC, Jun 10-12, 2019.
27.8% acceptance rate
Abstract. This paper looks into the problem of detecting network anomalies by analyzing NetFlow records. While many previous works have used statistical models and machine learning techniques in a supervised way, such solutions have the limitations that they require large amount of labeled data for training and are unlikely to detect zero-day attacks. Existing anomaly detection solutions also do not provide an easy way to explain or identify attacks in the anomalous traffic. To address these limitations, we develop and present GEE, a framework for detecting and explaining anomalies in network traffic. GEE comprises of two components: (i) Variational Autoencoder (VAE) —- an unsupervised deep-learning technique for detecting anomalies, and (ii) a gradient-based fingerprinting technique for explaining anomalies. Evaluation of GEE on UGR dataset demonstrates that our approach is effective in detecting different anomalies as well as identifying fingerprints that are good representations of these various attacks.
- Stochastic Variational Inference for Bayesian Sparse Gaussian Process Regression.
Haibin Yu, Trong Nghia Hoang, Kian Hsiang Low & Patrick Jaillet.
In Proceedings of the International Joint Conference on Neural Networks (IJCNN'19), Budapest, Hungary, Jul 14-19, 2019.
52.4% acceptance rate
Abstract. This paper presents a novel variational inference framework for deriving a family of Bayesian sparse Gaussian process regression (SGPR) models whose approximations are variationally optimal with respect to the full-rank GPR model enriched with various corresponding correlation structures of the observation noises. Our variational Bayesian SGPR (VBSGPR) models jointly treat both the distributions of the inducing variables and hyperparameters as variational parameters, which enables the decomposability of the variational lower bound that in turn can be exploited for stochastic optimization. Such a stochastic optimization involves iteratively following the stochastic gradient of the variational lower bound to improve its estimates of the optimal variational distributions of the inducing variables and hyperparameters (and hence the predictive distribution) of our VBSGPR models and is guaranteed to achieve asymptotic convergence to them. We show that the stochastic gradient is an unbiased estimator of the exact gradient and can be computed in constant time per iteration, hence achieving scalability to big data. We empirically evaluate the performance of our proposed framework on two real-world, massive datasets.
- Decentralized High-Dimensional Bayesian Optimization with Factor Graphs.
Trong Nghia Hoang, Quang Minh Hoang, Ruofei Ouyang & Kian Hsiang Low.
In Proceedings of the 32nd AAAI Conference on Artificial Intelligence (AAAI-18), pages 3231-3238, New Orleans, LA, Feb 2-8, 2018.
24.55% acceptance rate
Abstract. This paper presents a novel decentralized high-dimensional Bayesian optimization (DEC-HBO) algorithm that, in contrast to existing HBO algorithms, can exploit the interdependent effects of various input components on the output of the unknown objective function f for boosting the BO performance and still preserve scalability in the number of input dimensions without requiring prior knowledge or the existence of a low (effective) dimension of the input space. To realize this, we propose a sparse yet rich factor graph representation of f to be exploited for designing an acquisition function that can be similarly represented by a sparse factor graph and hence be efficiently optimized in a decentralized manner using distributed message passing. Despite richly characterizing the interdependent effects of the input components on the output of f with a factor graph, DEC-HBO can still guarantee (asymptotic) no-regret performance. Empirical evaluation on synthetic and real-world experiments shows that DEC-HBO outperforms the state-of-the-art HBO algorithms.
- Gaussian Process Decentralized Data Fusion Meets Transfer Learning in Large-Scale Distributed Cooperative Perception.
Ruofei Ouyang & Kian Hsiang Low.
In Proceedings of the 32nd AAAI Conference on Artificial Intelligence (AAAI-18), pages 3876-3883, New Orleans, LA, Feb 2-8, 2018.
24.55% acceptance rate
Abstract. This paper presents novel Gaussian process decentralized data fusion algorithms exploiting the notion of agent-centric support sets for distributed cooperative perception of large-scale environmental phenomena. To overcome the limitations of scale in existing works, our proposed algorithms allow every mobile sensing agent to choose a different support set and dynamically switch to another during execution for encapsulating its own data into a local summary that, perhaps surprisingly, can still be assimilated with the other agents' local summaries (i.e., based on their current choices of support sets) into a globally consistent summary to be used for predicting the phenomenon. To achieve this, we propose a novel transfer learning mechanism for a team of agents capable of sharing and transferring information encapsulated in a summary based on a support set to that utilizing a different support set with some loss that can be theoretically bounded and analyzed. To alleviate the issue of information loss accumulating over multiple instances of transfer learning, we propose a new information sharing mechanism to be incorporated into our algorithms in order to achieve memory-efficient lazy transfer learning. Empirical evaluation on real-world datasets show that our algorithms outperform the state-of-the-art methods.
- Artificial Intelligence Research in Singapore: Assisting the Development of a Smart Nation.
Pradeep Varakantham, Bo An, Bryan Low & Jie Zhang.
AI Magazine, volume 38, issue 3, pages 102-105, Fall 2017.
Abstract. Artificial Intelligence (AI) research in Singapore is focused on accelerating the country’s development into a Smart Nation. Specifically, AI has been employed extensively in either augmenting the intelligence of humans or in developing automated methods and systems to improve quality of life in Singapore.
- Distributed Batch Gaussian Process Optimization.
Erik Daxberger & Kian Hsiang Low.
In Proceedings of the 34th International Conference on Machine Learning (ICML-17), pages 951-960, Sydney, Australia, Aug 6-11, 2017.
25.9% acceptance rate
Abstract. This paper presents a novel distributed batch Gaussian process upper confidence bound (DB-GP-UCB) algorithm for performing batch Bayesian optimization (BO) of highly complex, costly-to-evaluate black-box objective functions. In contrast to existing batch BO algorithms, DB-GP-UCB can jointly optimize a batch of inputs (as opposed to selecting the inputs of a batch one at a time) while still preserving scalability in the batch size. To realize this, we generalize GP-UCB to a new batch variant amenable to a Markov approximation, which can then be naturally formulated as a multi-agent distributed constraint optimization problem in order to fully exploit the efficiency of its state-of-the-art solvers for achieving linear time in the batch size. Our DB-GP-UCB algorithm offers practitioners the flexibility to trade off between the approximation quality and time efficiency by varying the Markov order. We provide a theoretical guarantee for the convergence rate of DB-GP-UCB via bounds on its cumulative regret. Empirical evaluation on synthetic benchmark objective functions and a real-world optimization problem shows that DB-GP-UCB outperforms the state-of-the-art batch BO algorithms.
- A Generalized Stochastic Variational Bayesian Hyperparameter Learning Framework for Sparse Spectrum Gaussian Process Regression.
Quang Minh Hoang, Trong Nghia Hoang & Kian Hsiang Low.
In Proceedings of the 31st AAAI Conference on Artificial Intelligence (AAAI-17), pages 2007-2014, San Francisco, CA, Feb 4-9, 2017.
24.6% acceptance rate (oral presentation)
Abstract. While much research effort has been dedicated to scaling up sparse Gaussian process (GP) models based on inducing variables for big data, little attention is afforded to the other less explored class of low-rank GP approximations that exploit the sparse spectral representation of a GP kernel. This paper presents such an effort to advance the state of the art of sparse spectrum GP models to achieve competitive predictive performance for massive datasets. Our generalized framework of stochastic variational Bayesian sparse spectrum GP (sVBSSGP) models addresses their shortcomings by adopting a Bayesian treatment of the spectral frequencies to avoid overfitting, modeling these frequencies jointly in its variational distribution to enable their interaction a posteriori, and exploiting local data for boosting the predictive performance. However, such structural improvements result in a variational lower bound that is intractable to be optimized. To resolve this, we exploit a variational parameterization trick to make it amenable to stochastic optimization. Interestingly, the resulting stochastic gradient has a linearly decomposable structure that can be exploited to refine our stochastic optimization method to incur constant time per iteration while preserving its property of being an unbiased estimator of the exact gradient of the variational lower bound. Empirical evaluation on real-world datasets shows that sVBSSGP outperforms state-of-the-art stochastic implementations of sparse GP models.
- A Distributed Variational Inference Framework for Unifying Parallel Sparse Gaussian Process Regression Models.
Trong Nghia Hoang, Quang Minh Hoang & Kian Hsiang Low.
In Proceedings of the 33rd International Conference on Machine Learning (ICML-16), pages 382-391, New York City, NY, Jun 19-24, 2016.
24.3% acceptance rate
Abstract. This paper presents a novel distributed variational inference framework that unifies many parallel sparse Gaussian process regression (SGPR) models for scalable hyperparameter learning with big data. To achieve this, our framework exploits a structure of correlated noise process model that represents the observation noises as a finite realization of a high-order Gaussian Markov random process. By varying the Markov order and covariance function for the noise process model, different variational SGPR models result. This consequently allows the correlation structure of the noise process model to be characterized for which a particular variational SGPR model is optimal. We empirically evaluate the predictive performance and scalability of the distributed variational SGPR models unified by our framework on two real-world datasets.
- Near-Optimal Active Learning of Multi-Output Gaussian Processes.
Yehong Zhang, Trong Nghia Hoang, Kian Hsiang Low & Mohan Kankanhalli.
In Proceedings of the 30th AAAI Conference on Artificial Intelligence (AAAI-16), pages 2351-2357, Phoenix, AZ, Feb 12-17, 2016.
25.75% acceptance rate
Abstract. This paper addresses the problem of active learning of a multi-output Gaussian process (MOGP) model representing multiple types of coexisting correlated environmental phenomena. In contrast to existing works, our active learning problem involves selecting not just the most informative sampling locations to be observed but also the types of measurements at each selected location for minimizing the predictive uncertainty (i.e., posterior joint entropy) of a target phenomenon of interest given a sampling budget. Unfortunately, such an entropy criterion scales poorly in the numbers of candidate sampling locations and selected observations when optimized. To resolve this issue, we first exploit a structure common to sparse MOGP models for deriving a novel active learning criterion. Then, we exploit a relaxed form of sub-modularity property of our new criterion for devising a polynomial-time approximation algorithm that guarantees a constant-factor approximation of that achieved by the optimal set of selected observations. Empirical evaluation on real-world datasets shows that our proposed approach outperforms existing algorithms for active learning of MOGP and single-output GP models.
- Gaussian Process Planning with Lipschitz Continuous Reward Functions: Towards Unifying Bayesian Optimization, Active Learning, and Beyond.
Chun Kai Ling, Kian Hsiang Low & Patrick Jaillet.
In Proceedings of the 30th AAAI Conference on Artificial Intelligence (AAAI-16), pages 1860-1866, Phoenix, AZ, Feb 12-17, 2016.
25.75% acceptance rate
Abstract. This paper presents a novel nonmyopic adaptive Gaussian process planning (GPP) framework endowed with a general class of Lipschitz continuous reward functions that can unify some active learning/sensing and Bayesian optimization criteria and offer practitioners some flexibility to specify their desired choices for defining new tasks/problems. In particular, it utilizes a principled Bayesian sequential decision problem framework for jointly and naturally optimizing the exploration-exploitation trade-off. In general, the resulting induced GPP policy cannot be derived exactly due to an uncountable set of candidate observations. A key contribution of our work here thus lies in exploiting the Lipschitz continuity of the reward functions to solve for a nonmyopic adaptive ϵ-optimal GPP (ϵ-GPP) policy. To plan in real time, we further propose an asymptotically optimal, branch-and-bound anytime variant of ϵ-GPP with performance guarantee. We empirically demonstrate the effectiveness of our ϵ-GPP policy and its anytime variant in Bayesian optimization and an energy harvesting task.
- DrMAD: Distilling Reverse-Mode Automatic Differentiation for Optimizing Hyperparameters of Deep Neural Networks.
Jie Fu, Hongyin Luo, Jiashi Feng, Kian Hsiang Low & Tat-Seng Chua.
In Proceedings of the 25th International Joint Conference on Artificial Intelligence (IJCAI-16), pages 1469-1475, New York City, NY, Jul 9-15, 2016.
<25% acceptance rate
Abstract.
The performance of deep neural networks is well-known to be sensitive to the setting of their hyperparameters. Recent advances in reverse-mode automatic differentiation allow for optimizing hyperparameters with gradients. The standard way of computing these gradients involves a forward and backward pass of computations. However, the backward pass usually needs to consume unaffordable memory to store all the intermediate variables to exactly reverse the forward training procedure. In this work, we propose a simple but effective method, DrMAD, to distill the knowledge of the forward pass into a shortcut path, through which we approximately reverse the training trajectory. Experiments on two image benchmark datasets show that DrMAD is at least 45 times faster and consumes 100 times less memory compared to state-of-the-art methods for optimizing hyperparameters with minimal compromise to its effectiveness. To the best of our knowledge, DrMAD is the first research attempt to make it practical to automatically tune thousands of hyperparameters of deep neural networks.
- Multi-Agent Continuous Transportation with Online Balanced Partitioning.
Chao Wang, Somchaya Liemhetcharat & Kian Hsiang Low.
In Proceedings of the
15th International Conference on Autonomous Agents and MultiAgent Systems (AAMAS-16), pages 1303-1304, Singapore, May 9-13, 2016.
Abstract. We introduce the concept of continuous transportation task to the context of multi-agent systems. A continuous transportation task is one in which a multi-agent team visits a number of fixed locations, picks up objects, and delivers them to a transportation hub. The goal is to maximize the rate of transportation while the objects are replenished over time. In this extended abstract, we present a hybrid of centralized and distributed approaches that minimize communications in the multi-agent team. We contribute a novel online partitioning-transportation algorithm with information gathering in the multi-agent team.
- Concept-based Hybrid Fusion of Multimodal Event Signals.
Yuhui Wang, Christian von der Weth, Yehong Zhang, Kian Hsiang Low, Vivek Singh & Mohan Kankanhalli.
In Proceedings of the
IEEE International Symposium on Multimedia (ISM'16), pages 14-19, San Jose, CA, Dec 11-13, 2016.
26.1% acceptance rate
Abstract. Recent years have seen a significant increase in the number of sensors and resulting event related sensor data, allowing for a better monitoring and understanding of real-world events and situations. Event-related data come from not only physical sensors (e.g., CCTV cameras, webcams) but also social or microblogging platforms (e.g., Twitter). Given the wide-spread availability of sensors, we observe that sensors of different modalities often independently observe the same events. We argue that fusing multimodal data about an event can be helpful for more accurate detection, localization and detailed description of events of interest. However, multimodal data often include noisy observations, varying information densities and heterogeneous representations, which makes the fusion a challenging task. In this paper, we propose a hybrid fusion approach that takes the spatial and semantic characteristics of sensor signals about events into account. For this, we first adopt the concept of an image-based representation that expresses the situation of particular visual concepts (e.g., "crowdedness", "people marching") called Cmage for both physical and social sensor data. Based on this Cmage representation, we model sparse sensor information using a Gaussian process, fuses multimodal event signals with a Bayesian approach, and incorporates spatial relations between the sensor and social observations. We demonstrate the effectiveness of our approach as a proof-of-concept over real-world data. Our early results show that the proposed approach can reliably reduce the sensor-related noise, localize event place, improve event detection reliability, and add semantic context so that the fused data provide a better picture of the observed events or situations.
- Inverse Reinforcement Learning with Locally Consistent Reward Functions.
Quoc Phong Nguyen, Kian Hsiang Low & Patrick Jaillet.
In C. Cortes, N. D. Lawrence, D. D. Lee, M. Sugiyama, R. Garnett, editors, Advances in Neural Information Processing Systems 28: 29th Annual Conference on Neural Information Processing Systems (NeurIPS'15), pages 1747-1755, Curran Associates, Inc., Montreal, Canada, Dec 7-12, 2015.
21.9% acceptance rate
Abstract. Existing inverse reinforcement learning (IRL) algorithms have assumed each expert's demonstrated trajectory to be produced by only a single reward function. This paper presents a novel generalization of the IRL problem that allows each trajectory to be generated by multiple locally consistent reward functions, hence catering to more realistic and complex experts' behaviors.
Solving our generalized IRL problem thus involves not only learning these reward functions but also the stochastic transitions between them at any state (including unvisited states).
By representing our IRL problem with a probabilistic graphical model, an expectation-maximization (EM) algorithm can be devised to iteratively learn the reward functions and stochastic transitions between them in order to jointly improve the likelihood of the expert's demonstrated trajectories.
As a result, the most likely partition of a trajectory into segments that are generated from different locally consistent reward functions selected by EM can be derived.
Empirical evaluation on synthetic and real-world datasets shows that our IRL algorithm outperforms the state-of-the-art EM clustering with maximum likelihood IRL, which is, interestingly, a reduced variant of our approach.
- Gaussian Process Decentralized Data Fusion and Active Sensing for Spatiotemporal Traffic Modeling and Prediction in Mobility-on-Demand Systems.
Jie Chen, Kian Hsiang Low, Patrick Jaillet & Yujian Yao.
IEEE Transactions on Automation Science and Engineering
(Special Issue on Networked Cooperative Autonomous Systems), volume 12, issue 3, pages 901-921, Jul 2015.
Extended version of our UAI-12 and
RSS-13 papers
Abstract. Mobility-on-demand (MoD) systems have recently emerged as a
promising paradigm of one-way vehicle sharing for sustainable personal
urban mobility in densely populated cities. We assume the capability of
a MoD system to be enhanced by deploying robotic shared vehicles that
can autonomously cruise the streets to be hailed by users. A key
challenge of the MoD system is that of real-time, fine-grained mobility
demand and traffic flow sensing and prediction. This paper presents
novel Gaussian process (GP) decentralized data fusion and active
sensing algorithms for real-time, fine-grained traffic modeling and
prediction with a fleet of MoD vehicles. The predictive performance of
our decentralized data fusion algorithms are theoretically guaranteed to
be equivalent to that of sophisticated centralized sparse GP
approximations. We derive consensus filtering variants requiring only
local communication between neighboring vehicles. We theoretically
guarantee the performance of our decentralized active sensing
algorithms. When they are used to gather informative data for mobility
demand prediction, they can achieve a dual effect of fleet rebalancing
to service mobility demands. Empirical evaluation on real-world datasets
shows that our algorithms are significantly more time-efficient and
scalable in the size of data and fleet while achieving predictive
performance comparable to that of state-of-the-art algorithms.
- A Unifying Framework of Anytime Sparse Gaussian Process Regression Models with Stochastic Variational Inference for Big Data.
Trong Nghia Hoang, Quang Minh Hoang & Kian Hsiang Low.
In Proceedings of the 32nd International Conference on Machine Learning (ICML-15), pages 569-578, Lille, France, Jul 6-11, 2015.
26.0% acceptance rate
Abstract. This paper presents a novel unifying framework of anytime sparse Gaussian process regression (SGPR) models that can produce good predictive performance fast and improve their predictive performance over time. Our proposed unifying framework reverses the variational inference procedure to theoretically construct a non-trivial, concave functional that is maximized at the predictive distribution of any SGPR model of our choice.
As a result, a stochastic natural gradient ascent method can be derived that involves iteratively following the stochastic natural gradient of the functional to improve its estimate of the predictive distribution of the chosen SGPR model
and is guaranteed to achieve asymptotic convergence to it. Interestingly, we show that if the predictive distribution of the chosen SGPR model
satisfies certain decomposability conditions, then the stochastic natural gradient is an unbiased estimator of the exact natural gradient and can be computed in constant time (i.e., independent of data size) at each iteration. We empirically evaluate the trade-off between the predictive performance vs. time efficiency of the anytime SGPR models on two real-world million-sized datasets.
- Parallel Gaussian Process Regression for Big Data: Low-Rank Representation Meets Markov Approximation.
Kian Hsiang Low, Jiangbo Yu, Jie Chen & Patrick Jaillet.
In Proceedings of the 29th AAAI Conference on Artificial Intelligence (AAAI-15), pages 2821-2827, Austin, TX, Jan 25-29, 2015.
26.67% acceptance rate
Abstract. The expressive power of a Gaussian process (GP) model comes at a cost of poor scalability in the data size.
To improve its scalability, this paper presents a low-rank-cum-Markov approximation (LMA) of the GP model that is novel in leveraging the dual computational advantages stemming from complementing a low-rank approximate representation of the full-rank GP based on a support set of inputs with a Markov approximation of the resulting residual process; the latter approximation is guaranteed to be closest in the Kullback-Leibler distance criterion subject to some constraint
and is considerably more refined than that of existing sparse GP models utilizing low-rank representations due to its more relaxed conditional independence assumption (especially with larger data).
As a result, our LMA method can trade off between the size of the support set and the order of the Markov property to (a) incur lower computational cost than such sparse GP models while achieving predictive performance comparable to them and (b) accurately represent features/patterns of any scale.
Interestingly, varying the Markov order produces a spectrum of LMAs
with PIC approximation and full-rank GP at the two extremes.
An advantage of our LMA method is that it is amenable to parallelization on multiple machines/cores, thereby gaining greater scalability.
Empirical evaluation on three real-world datasets in clusters of up to 32 computing nodes shows that our centralized and parallel LMA methods are significantly more time-efficient and scalable than state-of-the-art sparse and full-rank GP regression methods
while achieving comparable predictive performances.
- Recent Advances in Scaling up Gaussian Process Predictive Models for Large Spatiotemporal Data.
Kian Hsiang Low, Jie Chen, Trong Nghia Hoang, Nuo Xu & Patrick Jaillet.
In S. Ravela, A. Sandu, editors,
Dynamic Data-Driven Environmental Systems Science - First International Conference, DyDESS'14, LNCS 8964, pages 167-181, Springer International Publishing, MIT, Cambridge, MA, Nov 5-7, 2014.
Oral presentation
Abstract. The expressive power of Gaussian process (GP) models comes at a cost of poor scalability in the size of the data. To improve their scalability, this paper presents an overview of our recent progress in scaling up GP models for large spatiotemporally correlated data through parallelization on clusters of machines, online learning, and nonmyopic active sensing/learning.
- Multi-Agent Ad Hoc Team Partitioning by Observing and Modeling Single-Agent Performance.
Etkin Baris Ozgul, Somchaya Liemhetcharat & Kian Hsiang Low.
In Proceedings of the
Asia-Pacific Signal and Information Processing Association Annual Summit and Conference (APSIPA ASC'14), pages 1-7, Siem Reap, city of Angkor Wat, Cambodia, Dec 9-12, 2014.
Abstract. Multi-agent research has focused on finding the optimal team for a task. Many approaches assume that the performance of the agents are known a priori. We are interested in ad hoc teams, where the agents' algorithms and performance are initially unknown. We focus on the task of modeling the performance of single agents through observation in training environments, and using the learned models to partition a new environment for a multi-agent team. The goal is to minimize the number of agents used, while maintaining a performance threshold of the multi-agent team. We contribute a novel model to learn the agent's performance through observations, and a partitioning algorithm that minimizes the team size. We evaluate our algorithms in simulation, and show the efficacy of our learn model and partitioning algorithm.
- Scalable Decision-Theoretic Coordination and Control for Real-time Active Multi-Camera Surveillance.
Prabhu Natarajan, Trong Nghia Hoang, Yongkang Wong, Kian Hsiang Low & Mohan Kankanhalli.
In Proceedings of the
8th ACM/IEEE International Conference on Distributed Smart Cameras (ICDSC'14) (Invited Paper to Special Session on Smart Cameras for Smart Environments), pages 115-120, Venezia, Italy, Nov 4-7, 2014.
Abstract. This paper presents an overview of our novel decision-theoretic multi-agent approach for controlling and coordinating multiple active cameras in surveillance. In this approach, a surveillance task is modeled as a stochastic optimization problem, where the active cameras are controlled and coordinated to achieve the desired surveillance goal in presence of uncertainties. We enumerate the practical issues in active camera surveillance and discuss how these issues are addressed in our decision-theoretic approach. We focus on two novel surveillance tasks: maximize the number of targets observed in active cameras with guaranteed image resolution and to improve the fairness in observation of multiple targets. We discuss the overview of our novel decision-theoretic frameworks: Markov decision process and partially observable Markov decision process frameworks for coordinating active cameras in uncertain and partially occluded environments.
- Active Learning is Planning: Nonmyopic ϵ-Bayes-Optimal Active Learning of Gaussian Processes.
Trong Nghia Hoang, Kian Hsiang Low, Patrick Jaillet and Mohan Kankanhalli.
In T. Calders, F. Esposito, E. Hüllermeier, R. Meo, editors, Machine Learning and Knowledge Discovery in Databases - European Conference, ECML/PKDD-14 Nectar (New Scientific and Technical Advances in Research) Track, Part III, LNCS 8726, pages 494-498, Springer Berlin Heidelberg, Nancy, France, Sep 15-19, 2014.
Abstract. A fundamental issue in active learning of Gaussian processes is that of the exploration-exploitation trade-off. This paper presents a novel nonmyopic ϵ-Bayes-optimal active learning (ϵ-BAL) approach that jointly optimizes the trade-off. In contrast, existing works have primarily developed greedy algorithms or performed exploration and exploitation separately. To perform active learning in real time, we then propose an anytime algorithm based on ϵ-BAL with performance guarantee and empirically demonstrate using a real-world dataset that, with limited budget, it outperforms the state-of-the-art algorithms.
- Generalized Online Sparse Gaussian Processes with Application to Persistent Mobile Robot Localization.
Kian Hsiang Low, Nuo Xu, Jie Chen, Keng Kiat Lim & Etkin Baris Ozgul.
In T. Calders, F. Esposito, E. Hüllermeier, R. Meo, editors, Machine Learning and Knowledge Discovery in Databases - European Conference, ECML/PKDD-14 Nectar (New Scientific and Technical Advances in Research) Track, Part III, LNCS 8726, pages 499-503, Springer Berlin Heidelberg, Nancy, France, Sep 15-19, 2014.
Abstract. This paper presents a novel online sparse Gaussian process (GP) approximation method that is capable of achieving constant time and memory (i.e., independent of the size of the data) per time step. We theoretically guarantee its predictive performance to be equivalent to that of a sophisticated offline sparse GP approximation method. We empirically demonstrate the practical feasibility of using our online sparse GP approximation method through a real-world persistent mobile robot localization experiment.
- No One is Left "Unwatched": Fairness in Observation of Crowds of Mobile Targets in Active Camera Surveillance.
Prabhu Natarajan, Kian Hsiang Low & Mohan Kankanhalli.
In Proceedings of the
21st European Conference on Artificial Intelligence (ECAI-14), including Prestigious Applications of Intelligent Systems (PAIS-14), pages 1155-1160, Prague, Czech Republic, Aug 18-22, 2014.
Abstract. Central to the problem of active multi-camera surveillance is the fundamental issue of fairness in the observation of crowds of targets such that no target is "starved" of observation by the cameras for a long time. This paper presents a principled decision-theoretic multi-camera coordination and control (MC2) algorithm called fair-MC2 that can coordinate and control the active cameras to achieve max-min fairness in the observation of crowds of targets moving stochastically. Our fair-MC2 algorithm is novel in demonstrating how (a) the uncertainty in the locations, directions, speeds, and observation times of the targets arising from the stochasticity of their motion can be modeled probabilistically, (b) the notion of fairness in observing targets can be formally realized in the domain of multi-camera surveillance for the first time by exploiting the max-min fairness metric to formalize our surveillance objective, that is, to maximize the expected minimum observation time over all targets while guaranteeing a predefined image resolution of observing them, and (c) a structural assumption in the state transition dynamics of a surveillance environment can be exploited to improve its scalability to linear time in the number of targets to be observed during surveillance. Empirical evaluation through extensive simulations in realistic surveillance environments shows that fair-MC2 outperforms the state-of-the-art and baseline MC2 algorithms. We have also demonstrated the feasibility of deploying our fair-MC2 algorithm on real AXIS 214 PTZ cameras.
- GP-Localize: Persistent Mobile Robot Localization using Online Sparse Gaussian Process Observation Model.
Nuo Xu, Kian Hsiang Low, Jie Chen, Keng Kiat Lim & Etkin Baris Ozgul.
In Proceedings of the 28th AAAI Conference on Artificial Intelligence (AAAI-14), pages 2585-2592, Quebec City, Canada, Jul 27-31, 2014.
16.6% acceptance rate (oral presentation)
Also appeared in
RSS-14 Workshop on Non-Parametric Learning in Robotics, Berkeley, CA, Jul 12, 2014.
Abstract. Central to robot exploration and mapping is the task of persistent localization in environmental fields characterized by spatially correlated measurements. This paper presents a Gaussian process localization (GP-Localize) algorithm that, in contrast to existing works, can exploit the spatially correlated field measurements taken during a robot's exploration (instead of relying on prior training data) for efficiently and scalably learning the GP observation model online through our proposed novel online sparse GP. As a result, GP-Localize is capable of achieving constant time and memory (i.e., independent of the size of the data) per filtering step, which demonstrates the practical feasibility of using GPs for persistent robot localization and autonomy. Empirical evaluation via simulated experiments with real-world datasets and a real robot experiment shows that GP-Localize outperforms existing GP localization algorithms.
- Nonmyopic ϵ-Bayes-Optimal Active Learning of Gaussian Processes.
Trong Nghia Hoang, Kian Hsiang Low, Patrick Jaillet and Mohan Kankanhalli.
In Proceedings of the 31st International Conference on Machine Learning (ICML-14), pages 739-747, Beijing, China, Jun 21-26, 2014.
22.4% acceptance rate (cycle 2)
Also appeared in
RSS-14 Workshop on Non-Parametric Learning in Robotics, Berkeley, CA, Jul 12, 2014.
Abstract. A fundamental issue in active learning of Gaussian processes is that of the exploration-exploitation trade-off.
This paper presents a novel nonmyopic ϵ-Bayes-optimal active learning (ϵ-BAL) approach that jointly and naturally optimizes the trade-off.
In contrast, existing works have primarily developed myopic/greedy algorithms or performed exploration and exploitation separately.
To perform active learning in real time, we then propose an anytime algorithm based on ϵ-BAL with performance guarantee and empirically demonstrate using synthetic and real-world datasets that, with limited budget, it outperforms the state-of-the-art algorithms.
- Multi-Robot Active Sensing of Non-Stationary Gaussian Process-Based Environmental Phenomena.
Ruofei Ouyang, Kian Hsiang Low, Jie Chen & Patrick Jaillet.
In Proceedings of the
13th International Conference on Autonomous Agents and MultiAgent Systems (AAMAS-14), pages 573-580, Paris, France, May 5-9, 2014.
23.8% acceptance rate
Also appeared in
RSS-14 Workshop on Non-Parametric Learning in Robotics, Berkeley, CA, Jul 12, 2014.
Abstract. A key challenge of environmental sensing and monitoring is that of sensing, modeling, and predicting large-scale, spatially correlated environmental phenomena, especially when they are unknown and non-stationary.
This paper presents a decentralized multi-robot active sensing (DEC-MAS) algorithm that can efficiently coordinate the exploration of multiple robots to gather the most informative observations for predicting an unknown, non-stationary phenomenon.
By modeling the phenomenon using a Dirichlet process mixture of Gaussian processes (DPM-GPs), our work here is novel in demonstrating how DPM-GPs and its structural properties can be exploited to (a) formalize an active sensing criterion that trades off between gathering the most informative observations for estimating the unknown, non-stationary spatial correlation structure vs. that for predicting the phenomenon given the current, imprecise estimate of the correlation structure, and (b) support efficient decentralized coordination.
We also provide a theoretical performance guarantee for DEC-MAS and analyze its time complexity.
We empirically demonstrate using two real-world datasets that DEC-MAS outperforms state-of-the-art MAS algorithms.
- Decision-Theoretic Approach to Maximizing Fairness in Multi-Target Observation in Multi-Camera Surveillance.
Prabhu Natarajan, Kian Hsiang Low & Mohan Kankanhalli.
In Proceedings of the
13th International Conference on Autonomous Agents and MultiAgent Systems (AAMAS-14), pages 1521-1522, Paris, France, May 5-9, 2014.
Abstract. Central to the problem of active multi-camera surveillance is the fundamental issue of fairness in the observation of multiple targets such that no target is left unobserved by the cameras for a long time. To address this important issue, we propose a novel principled decision-theoretic approach to control and coordinate multiple active cameras to achieve fairness in the observation of multiple moving targets.
- Interactive POMDP Lite: Towards Practical Planning to Predict and Exploit Intentions for Interacting with Self-Interested Agents.
Trong Nghia Hoang & Kian Hsiang Low.
In Proceedings of the 23rd International Joint Conference on Artificial Intelligence (IJCAI-13), pages 2298-2305, Beijing, China, Aug 3-9, 2013.
13.2% acceptance rate (oral presentation)
Abstract. A key challenge in non-cooperative multi-agent systems is that of developing efficient planning algorithms for intelligent agents to interact and perform effectively among boundedly rational, self-interested agents (e.g., humans). The practicality of existing works addressing this challenge is being undermined due to either the restrictive assumptions of the other agents' behavior, the failure in accounting for their rationality, or the prohibitively expensive cost of modeling and predicting their intentions. To boost the practicality of research in this field, we investigate how intention prediction can be efficiently exploited and made practical in planning, thereby leading to efficient intention-aware planning frameworks capable of predicting the intentions of other agents and acting optimally with respect to their predicted intentions. We show that the performance losses incurred by the resulting planning policies are linearly bounded by the error of intention prediction. Empirical evaluations through a series of stochastic games demonstrate that our policies can achieve better and more robust performance than the state-of-the-art algorithms.
- A General Framework for Interacting Bayes-Optimally with Self-Interested Agents using Arbitrary Parametric Model and Model Prior.
Trong Nghia Hoang & Kian Hsiang Low.
In Proceedings of the 23rd International Joint Conference on Artificial Intelligence (IJCAI-13), pages 1394-1400, Beijing, China, Aug 3-9, 2013.
28.0% acceptance rate
Abstract. Recent advances in Bayesian reinforcement learning (BRL) have shown that Bayes-optimality is theoretically achievable by modeling the environment's latent dynamics using Flat-Dirichlet-Multinomial (FDM) prior. In self-interested multi-agent environments, the transition dynamics are mainly controlled by the other agent's stochastic behavior for which FDM's independence and modeling assumptions do not hold. As a result, FDM does not allow the other agent's behavior to be generalized across different states nor specified using prior domain knowledge. To overcome these practical limitations of FDM, we propose a generalization of BRL to integrate the general class of parametric models and model priors, thus allowing practitioners' domain knowledge to be exploited to produce a fine-grained and compact representation of the other agent's behavior. Empirical evaluation shows that our approach outperforms existing multi-agent reinforcement learning algorithms.
- Parallel Gaussian Process Regression with Low-Rank Covariance Matrix Approximations.
Jie Chen, Nannan Cao, Kian Hsiang Low, Ruofei Ouyang, Colin Keng-Yan Tan & Patrick Jaillet.
In Proceedings of the 29th Conference on Uncertainty in Artificial Intelligence (UAI-13), pages 152-161, Bellevue, WA, Jul 11-15, 2013.
31.3% acceptance rate
Abstract. Gaussian processes (GP) are Bayesian non-parametric models that are widely used for probabilistic regression. Unfortunately, it cannot scale well with large data nor perform real-time predictions due to its cubic time cost in the data size. This paper presents two parallel GP regression methods that exploit low-rank covariance matrix approximations for distributing the computational load among parallel machines to achieve time efficiency and scalability. We theoretically guarantee the predictive performances of our proposed parallel GPs to be equivalent to that of some centralized approximate GP regression methods: The computation of their centralized counterparts can be distributed among parallel machines, hence achieving greater time efficiency and scalability. We analytically compare the properties of our parallel GPs such as time, space, and communication complexity. Empirical evaluation on two real-world datasets in a cluster of 20 computing nodes shows that our parallel GPs are significantly more time-efficient and scalable than their centralized counterparts and exact/full GP while achieving predictive performances comparable to full GP.
- Gaussian Process-Based Decentralized Data Fusion and Active Sensing for Mobility-on-Demand System.
Jie Chen, Kian Hsiang Low & Colin Keng-Yan Tan.
In Proceedings of the
Robotics: Science and Systems Conference (RSS-13), Berlin, Germany, Jun 24-28, 2013.
30.1% acceptance rate
Abstract. Mobility-on-demand (MoD) systems have recently emerged as a promising paradigm of one-way vehicle sharing for sustainable personal urban mobility in densely populated cities. In this paper, we enhance the capability of a MoD system by deploying robotic shared vehicles that can autonomously cruise the streets to be hailed by users. A key challenge to managing the MoD system effectively is that of real-time, fine-grained mobility demand sensing and prediction. This paper presents a novel decentralized data fusion and active sensing algorithm for real-time, fine-grained mobility demand sensing and prediction with a fleet of autonomous robotic vehicles in a MoD system. Our Gaussian process (GP)-based decentralized data fusion algorithm can achieve a fine balance between predictive power and time efficiency. We theoretically guarantee its predictive performance to be equivalent to that of a sophisticated centralized sparse approximation for the GP model: The computation of such a sparse approximate GP model can thus be distributed among the MoD vehicles, hence achieving efficient and scalable demand prediction. Though our decentralized active sensing strategy is devised to gather the most informative demand data for demand prediction, it can achieve a dual effect of fleet rebalancing to service the mobility demands. Empirical evaluation on real-world mobility demand data shows that our proposed algorithm can achieve a better balance between predictive accuracy and time efficiency than state-of-the-art algorithms.
- Multi-Robot Informative Path Planning for Active Sensing of Environmental Phenomena: A Tale of Two Algorithms.
Nannan Cao, Kian Hsiang Low & John M. Dolan.
In Proceedings of the
12th International Conference on Autonomous Agents and MultiAgent Systems (AAMAS-13), pages 7-14, Saint Paul, MN, May 6-10, 2013.
22.9% acceptance rate
Abstract. A key problem of robotic environmental sensing and monitoring is that of active sensing: How can a team of robots plan the most informative observation paths to minimize the uncertainty in modeling and predicting an environmental phenomenon? This paper presents two principled approaches to efficient information-theoretic path planning based on entropy and mutual information criteria for in situ active sensing of an important broad class of widely-occurring environmental phenomena called anisotropic fields. Our proposed algorithms are novel in addressing a trade-off between active sensing performance and time efficiency. An important practical consequence is that our algorithms can exploit the spatial correlation structure of Gaussian process-based anisotropic fields to improve time efficiency while preserving near-optimal active sensing performance. We analyze the time complexity of our algorithms and prove analytically that they scale better than state-of-the-art algorithms with increasing planning horizon length. We provide theoretical guarantees on the active sensing performance of our algorithms for a class of exploration tasks called transect sampling, which, in particular, can be improved with longer planning time and/or lower spatial correlation along the transect. Empirical evaluation on real-world anisotropic field data shows that our algorithms can perform better or at least as well as the state-of-the-art algorithms while often incurring a few orders of magnitude less computational time, even when the field conditions are less favorable.
- Adaptive Sampling of Time Series with Application to Remote Exploration.
David R. Thompson, Nathalie Cabrol, Michael Furlong, Craig Hardgrove, Kian Hsiang Low, Jeffrey Moersch & David Wettergreen.
In Proceedings of the IEEE International Conference on Robotics and Automation (ICRA'13), pages 3463-3468, Karlsruhe, Germany, May 6-10, 2013.
Abstract. We address the problem of adaptive information-optimal data collection in time series. Here a remote sensor or explorer agent throttles its sampling rate in order to track anomalous events while obeying constraints on time and power. This problem is challenging because the agent has limited visibility - all collected datapoints lie in the past, but its resource allocation decisions require predicting far into the future. Our solution is to continually fit a Gaussian process model to the latest data and optimize the sampling plan on line to maximize information gain. We compare the performance characteristics of stationary and nonstationary Gaussian process models. We also describe an application based on geologic analysis during planetary rover exploration. Here adaptive sampling can improve coverage of localized anomalies and potentially benefit mission science yield of long autonomous traverses.
- Decentralized Data Fusion and Active Sensing with Mobile Sensors for Modeling and Predicting Spatiotemporal Traffic Phenomena.
Jie Chen, Kian Hsiang Low, Colin Keng-Yan Tan, Ali Oran, Patrick Jaillet, John M. Dolan & Gaurav S. Sukhatme.
In Proceedings of the 28th Conference on Uncertainty in Artificial Intelligence (UAI-12), pages 163-173, Catalina Island, CA, Aug 15-17, 2012.
31.6% acceptance rate
Also appeared in AAMAS-12 Workshop on Agents in Traffic and Transportation (ATT-12), Valencia, Spain, June 4-8, 2012.
Abstract. The problem of modeling and predicting spatiotemporal traffic phenomena over an urban road network is important to many traffic applications such as detecting and forecasting congestion hotspots. This paper presents a decentralized data fusion and active sensing (D2FAS) algorithm for mobile sensors to actively explore the road network to gather and assimilate the most informative data for predicting the traffic phenomenon. We analyze the time and communication complexity of D2FAS and demonstrate that it can scale well with a large number of observations and sensors. We provide a theoretical guarantee on its predictive performance to be equivalent to that of a sophisticated centralized sparse approximation for the Gaussian process (GP) model: The computation of such a sparse approximate GP model can thus be parallelized and distributed among the mobile sensors (in a Google-like MapReduce paradigm), thereby achieving efficient and scalable prediction. We also theoretically guarantee its active sensing performance that improves under various practical environmental conditions. Empirical evaluation on real-world urban road network data shows that our D2FAS algorithm is significantly more time-efficient and scalable than state-of-the-art centralized algorithms while achieving comparable predictive performance.
- Hierarchical Bayesian Nonparametric Approach to Modeling and Learning the Wisdom of Crowds of Urban Traffic Route Planning Agents.
Jiangbo Yu, Kian Hsiang Low, Ali Oran & Patrick Jaillet.
In Proceedings of the IEEE/WIC/ACM International Conference on Intelligent Agent Technology (IAT'12)
(Invited Paper to Special Session on Large-Scale Application-Focused Multi-Agent Systems), pages 478-485, Macau, Dec 4-7, 2012.
Abstract. Route prediction is important to analyzing and understanding the route patterns and behavior of traffic crowds. Its objective is to predict the most likely or "popular" route of road segments from a given point in a road network. This paper presents a hierarchical Bayesian non-parametric approach to efficient and scalable route prediction that can harness the wisdom of crowds of route planning agents by aggregating their sequential routes of possibly varying lengths and origin-destination pairs. In particular, our approach has the advantages of (a) not requiring a Markov assumption to be imposed and (b) generalizing well with sparse data, thus resulting in significantly improved prediction accuracy, as demonstrated empirically using real-world taxi route data. We also show two practical applications of our route prediction algorithm: predictive taxi ranking and route recommendation.
- Decision-Theoretic Coordination and Control for Active Multi-Camera Surveillance in Uncertain, Partially Observable Environments.
Prabhu Natarajan, Trong Nghia Hoang, Kian Hsiang Low & Mohan Kankanhalli.
In Proceedings of the
6th ACM/IEEE International Conference on Distributed Smart Cameras (ICDSC'12), pages 1-6, Hong Kong, Oct 30 - Nov 2, 2012.
Abstract. A central problem of surveillance is to monitor multiple targets moving in a large-scale, obstacle-ridden environment with occlusions. This paper presents a novel principled Partially Observable Markov Decision Process-based approach to coordinating and controlling a network of active cameras for tracking and observing multiple mobile targets at high resolution in such surveillance environments. Our proposed approach is capable of (a) maintaining a belief over the targets' states (i.e., locations, directions, and velocities) to track them, even when they may not be observed directly by the cameras at all times, (b) coordinating the cameras' actions to simultaneously improve the belief over the targets' states and maximize the expected number of targets observed with a guaranteed resolution, and (c) exploiting the inherent structure of our surveillance problem to improve its scalability (i.e., linear time) in the number of targets to be observed. Quantitative comparisons with state-of-the-art multi-camera coordination and control techniques show that our approach can achieve higher surveillance quality in real time. The practical feasibility of our approach is also demonstrated using real AXIS 214 PTZ cameras.
- Decentralized Active Robotic Exploration and Mapping for Probabilistic Field Classification in Environmental Sensing.
Kian Hsiang Low, Jie Chen, John M. Dolan, Steve Chien & David R. Thompson.
In Proceedings of the
11th International Conference on Autonomous Agents and MultiAgent Systems (AAMAS-12), pages 105-112, Valencia, Spain, June 4-8, 2012.
20.4% acceptance rate
Also appeared in
IROS'11 Workshop on Robotics for Environmental Monitoring (WREM-11), San Francisco, CA, Sep 30, 2011.
Abstract. A central problem in environmental sensing and monitoring is to classify/label the hotspots in a large-scale environmental field. This paper presents a novel decentralized active robotic exploration (DARE) strategy for probabilistic classification/labeling of hotspots in a Gaussian process (GP)-based field. In contrast to existing state-of-the-art exploration strategies for learning environmental field maps, the time needed to solve the DARE strategy is independent of the map resolution and the number of robots, thus making it practical for in situ, real-time active sampling. Its exploration behavior exhibits an interesting formal trade-off between that of boundary tracking until the hotspot region boundary can be accurately predicted and wide-area coverage to find new boundaries in sparsely sampled areas to be tracked. We provide a theoretical guarantee on the active exploration performance of the DARE strategy: under reasonable conditional independence assumption, we prove that it can optimally achieve two formal cost-minimizing exploration objectives based on the misclassification and entropy criteria. Importantly, this result implies that the uncertainty of labeling the hotspots in a GP-based field is greatest at or close to the hotspot region boundaries. Empirical evaluation on real-world plankton density and temperature field data shows that, subject to limited observations, DARE strategy can achieve more superior classification of hotspots and time efficiency than state-of-the-art active exploration strategies.
- Decision-Theoretic Approach to Maximizing Observation of Multiple Targets in Multi-Camera Surveillance.
Prabhu Natarajan, Trong Nghia Hoang, Kian Hsiang Low & Mohan Kankanhalli.
In Proceedings of the
11th International Conference on Autonomous Agents and MultiAgent Systems (AAMAS-12), pages 155-162, Valencia, Spain, June 4-8, 2012.
20.4% acceptance rate
Abstract. This paper presents a novel decision-theoretic approach to control and coordinate multiple active cameras for observing a number of moving targets in a surveillance system. This approach offers the advantages of being able to (a) account for the stochasticity of targets' motion via probabilistic modeling, and (b) address the trade-off between maximizing the expected number of observed targets and the resolution of the observed targets through stochastic optimization. One of the key issues faced by existing approaches in multi-camera surveillance is that of scalability with increasing number of targets. We show how its scalability can be improved by exploiting the problem structure: as proven analytically, our decision-theoretic approach incurs time that is linear in the number of targets to be observed during surveillance. As demonstrated empirically through simulations, our proposed approach can achieve high-quality surveillance of up to 50 targets in real time and its surveillance performance degrades gracefully with increasing number of targets. We also demonstrate our proposed approach with real AXIS 214 PTZ cameras in maximizing the number of Lego robots observed at high resolution over a surveyed rectangular area. The results are promising and clearly show the feasibility of our decision-theoretic approach in controlling and coordinating the active cameras in real surveillance system.
- Intention-Aware Planning under Uncertainty for Interacting with Self-Interested, Boundedly Rational Agents.
Trong Nghia Hoang & Kian Hsiang Low.
In Proceedings of the
11th International Conference on Autonomous Agents and MultiAgent Systems (AAMAS-12), pages 1233-1234, Valencia, Spain, June 4-8, 2012.
Abstract. A key challenge in non-cooperative multi-agent systems is that of developing efficient planning algorithms for intelligent agents to perform effectively among boundedly rational, self-interested (i.e., non-cooperative) agents (e.g., humans). To address this challenge, we investigate how intention prediction can be efficiently exploited and made practical in planning, thereby leading to efficient intention-aware planning frameworks capable of predicting the intentions of other agents and acting optimally with respect to their predicted intentions.
- Active Markov Information-Theoretic Path Planning for Robotic Environmental Sensing.
Kian Hsiang Low, John M. Dolan & Pradeep Khosla.
In Proceedings of the
10th International Conference on Autonomous Agents and MultiAgent Systems (AAMAS-11), pages 753-760, Taipei, Taiwan, May 2-6, 2011.
22.1% acceptance rate
Abstract. Recent research in multi-robot exploration and mapping has focused on sampling environmental fields, which are typically modeled using the Gaussian process (GP). Existing information-theoretic exploration strategies for learning GP-based environmental field maps adopt the non-Markovian problem structure and consequently scale poorly with the length of history of observations. Hence, it becomes computationally impractical to use these strategies for in situ, real-time active sampling. To ease this computational burden, this paper presents a Markov-based approach to efficient information-theoretic path planning for active sampling of GP-based fields. We analyze the time complexity of solving the Markov-based path planning problem, and demonstrate analytically that it scales better than that of deriving the non-Markovian strategies with increasing length of planning horizon. For a class of exploration tasks called the transect sampling task, we provide theoretical guarantees on the active sampling performance of our Markov-based policy, from which ideal environmental field conditions and sampling task settings can be established to limit its performance degradation due to violation of the Markov assumption. Empirical evaluation on real-world temperature and plankton density field data shows that our Markov-based policy can generally achieve active sampling performance comparable to that of the widely-used non-Markovian greedy policies under less favorable realistic field conditions and task settings while enjoying significant computational gain over them.
- Autonomous Personal Vehicle for the First- and Last-Mile Transportation Services.
Zhuang Jie Chong, Baoxing Qin, Tirthankar Bandyopadhyay, Tichakorn Wongpiromsarn, Edward Samuel Rankin, Marcelo H. Ang, Jr., Emilio Frazzoli, Daniela Rus, David Hsu & Kian Hsiang Low.
In Proceedings of the
5th IEEE International Conference on Cybernetics and
Intelligent Systems and 5th IEEE International
Conference on Robotics, Automation and Mechatronics (CIS-RAM'11), pages 253-260, Qingdao, China, Sep 17-19, 2011.
Also appeared in IROS'11 Workshop on Perception and Navigation for Autonomous Vehicles in Human Environment, San Francisco, CA, Sep 30, 2011.
Abstract. This paper describes an autonomous vehicle testbed that aims at providing the first- and last- mile transportation services. The vehicle mainly operates in a crowded urban environment whose features can be extracted a priori. To ensure that the system is economically feasible, we take a minimalistic approach and exploit prior knowledge of the environment and the availability of the existing infrastructure such as cellular networks and traffic cameras. We present three main components of the system: pedestrian detection, localization (even in the presence of tall buildings) and navigation. The performance of each component is evaluated. Finally, we describe the role of the existing infrastructural sensors and show the improved performance of the system when they are utilized.
- Telesupervised Remote Surface
Water Quality Sensing.
Gregg Podnar, John M. Dolan, Kian Hsiang Low & Alberto Elfes.
In Proceedings of the IEEE Aerospace Conference, Big Sky, MT, Mar 6-13, 2010.
Abstract. We present a fleet of autonomous Robot Sensor Boats (RSBs) developed for lake and river fresh water quality assessment and controlled by our Multilevel Autonomy Robot Telesupervision Architecture (MARTA). The RSBs are low cost, highly maneuverable, shallow draft sensor boats, developed as part of the Sensor Web program supported under the Advanced Information Systems Technology program of NASA's Earth Systems Technology Office. They can scan large areas of lakes, and navigate up tributaries to measure water quality near outfalls that larger research vessels cannot reach. The MARTA telesupervision architecture has been applied to a number of domains from multi-platform autonomous wide area planetary mineral prospecting, to multi-platform ocean monitoring. The RSBs are a complementary expansion of a fleet of NOAA/NASA-developed extended-deployment surface autonomous vehicles that enable in-situ study of meteorological factors of the ocean/atmosphere interface, and which have been adapted to investigate harmful algal blooms under this program. The flexibility of the MARTA telesupervision architecture was proven as it supported simultaneous operation of these heterogenous autonomous sensor platforms while geographically widely separated. Results and analysis are presented of multiple tests carried out over three months using a multi-sensor water sonde to assess water quality in a small recreational lake. Inference Grids were used to produce maps representing temperature, pH, and dissolved oxygen. The tests were performed under various water conditions (clear vs. hair algae-laden) and both before and after heavy rains. Data from each RSB was relayed to a data server in our lab in Pittsburgh, Pennsylvania, and made available over the World Wide Web where it was acquired by team members at the Jet Propulsion Laboratory of NASA in Pasadena, California who monitored the boats and their sensor readings in real time, as well as using these data to model the water quality by producing Inference Grid-based maps.
- Information-Theoretic Approach to Efficient Adaptive Path Planning for Mobile Robotic Environmental Sensing.
Kian Hsiang Low, John M. Dolan & Pradeep Khosla.
In Proceedings of the 19th International Conference on Automated Planning and Scheduling (ICAPS-09), pages 233-240, Thessaloniki, Greece, Sep 19-23, 2009.
33.9% acceptance rate
Also appeared in IPSN-09 Workshop on Sensor Networks for Earth and Space Science Applications (ESSA-09), San Francisco, CA, Apr 16, 2009.
Also orally presented in RSS-09 Workshop on Aquatic Robots and Ocean Sampling, Seattle, WA, Jun 29, 2009.
Abstract. Recent research in robot exploration and mapping has focused on sampling environmental hotspot fields. This exploration task is formalized by Low, Dolan, and Khosla (2008) in a sequential decision-theoretic planning under uncertainty framework called MASP. The time complexity of solving MASP approximately depends on the map resolution, which limits its use in large-scale, high-resolution exploration and mapping. To alleviate this computational difficulty, this paper presents an information-theoretic approach to MASP (iMASP) for efficient adaptive path planning; by reformulating the cost-minimizing iMASP as a reward-maximizing problem, its time complexity becomes independent of map resolution and is less sensitive to increasing robot team size as demonstrated both theoretically and empirically. Using the reward-maximizing dual, we derive a novel adaptive variant of maximum entropy sampling, thus improving the induced exploration policy performance. It also allows us to establish theoretical bounds quantifying the performance advantage of optimal adaptive over non-adaptive policies and the performance quality of approximately optimal vs. optimal adaptive policies. We show analytically and empirically the superior performance of iMASP-based policies for sampling the log-Gaussian process to that of policies for the widely-used Gaussian process in mapping the hotspot field. Lastly, we provide sufficient conditions that, when met, guarantee adaptivity has no benefit under an assumed environment model.
- Cooperative Aquatic Sensing using the Telesupervised Adaptive Ocean Sensor Fleet.
John M. Dolan, Gregg W. Podnar, Stephen Stancliff, Kian Hsiang Low, Alberto Elfes, John Higinbotham, Jeffrey C. Hosler, Tiffany A. Moisan & John Moisan.
In Proceedings of the SPIE Conference on Remote Sensing of the Ocean, Sea Ice, and Large Water Regions, volume 7473, Berlin, Germany, Aug 31 - Sep 3, 2009.
Abstract. Earth science research must bridge the gap between the atmosphere and the ocean to foster understanding of Earth's climate and ecology. Typical ocean sensing is done with satellites or in situ buoys and research ships which are slow to reposition. Cloud cover inhibits study of localized transient phenomena such as Harmful Algal Blooms (HAB). A fleet of extended-deployment surface autonomous vehicles will enable in situ study of characteristics of HAB, coastal pollutants, and related phenomena. We have developed a multiplatform telesupervision architecture that supports adaptive reconfiguration based on environmental sensor inputs. Our system allows the autonomous repositioning of smart sensors for HAB study by networking a fleet of NOAA OASIS (Ocean Atmosphere Sensor Integration System) surface autonomous vehicles. In situ measurements intelligently modify the search for areas of high concentration. Inference Grid and complementary information-theoretic techniques support sensor fusion and analysis. Telesupervision supports sliding autonomy from high-level mission tasking, through vehicle and data monitoring, to teleoperation when direct human interaction is appropriate. This paper reports on experimental results from multi-platform tests conducted in the Chesapeake Bay and in Pittsburgh, Pennsylvania waters using OASIS platforms, autonomous kayaks, and multiple simulated platforms to conduct cooperative sensing of chlorophyll-a and water quality.
- Robot Boats as a Mobile Aquatic Sensor Network.
Kian Hsiang Low, Gregg Podnar, Stephen Stancliff, John M. Dolan & Alberto Elfes.
In Proceedings of the IPSN-09 Workshop on Sensor Networks for Earth and Space Science Applications (ESSA-09), San Francisco, CA, Apr 16, 2009.
Abstract. This paper describes the Multilevel Autonomy Robot Telesupervision Architecture (MARTA), an architecture for supervisory control of a heterogeneous fleet of net-worked unmanned autonomous aquatic surface vessels carrying a payload of environmental science sensors. This architecture allows a land-based human scientist to effectively supervise data gathering by multiple robotic assets that implement a web of widely dispersed mobile sensors for in situ study of physical, chemical or bio-logical processes in water or in the water/atmosphere interface.
- Adaptive Multi-Robot Wide-Area Exploration And Mapping.
Kian Hsiang Low, John M. Dolan & Pradeep Khosla.
In Proceedings of the
7th International Conference on Autonomous Agents and MultiAgent Systems (AAMAS-08), pages 23-30, Estoril, Portugal, May 12-16, 2008.
22.2% acceptance rate
Also presented as a poster in RSS-09 Workshop on Aquatic Robots and Ocean Sampling, Seattle, WA, Jun 29, 2009.
Abstract. The exploration problem is a central issue in mobile robotics. A complete terrain coverage is not practical if the environment is large with only a few small hotspots. This paper presents an adaptive multi-robot exploration strategy that is novel in performing both wide-area coverage and hotspot sampling using non-myopic path planning. As a result, the environmental phenomena can be accurately mapped. It is based on a dynamic programming formulation, which we call the Multi-robot Adaptive Sampling Problem (MASP). A key feature of MASP is in covering the entire adaptivity spectrum, thus allowing strategies of varying adaptivity to be formed and theoretically analyzed in their performance; a more adaptive strategy improves mapping accuracy. We apply MASP to sampling the Gaussian and log-Gaussian processes, and analyze if the resulting strategies are adaptive and maximize wide-area coverage and hotspot sampling. Solving MASP is non-trivial as it comprises continuous state components. So, it is reformulated for convex analysis, which allows discrete-state monotone-bounding approximation to be developed. We provide a theoretical guarantee on the policy quality of the approximate MASP (aMASP) for using in MASP. Although aMASP can be solved exactly, its state size grows exponentially with the number of stages. To alleviate this computational difficulty, anytime algorithms are proposed based on aMASP, one of which can guarantee its policy quality for MASP in real time.
- Adaptive Sampling for Multi-Robot Wide-Area Exploration.
Kian Hsiang Low, Geoffrey J. Gordon, John M. Dolan & Pradeep Khosla.
In Proceedings of the IEEE International Conference on Robotics and Automation (ICRA'07), pages 755-760, Rome, Italy, Apr 10-14, 2007.
Abstract. The exploration problem is a central issue in mobile robotics. A complete coverage is not practical if the environment is large with a few small hotspots, and the sampling cost is high. So, it is desirable to build robot teams that can coordinate to maximize sampling at these hotspots while minimizing resource costs, and consequently learn more accurately about properties of such environmental phenomena. An important issue in designing such teams is the exploration strategy. The contribution of this paper is in the evaluation of an adaptive exploration strategy called adaptive cluster sampling (ACS), which is demonstrated to reduce the resource costs (i.e., mission time and energy consumption) of a robot team, and yield more information about the environment by directing robot exploration towards hotspots. Due to the adaptive nature of the strategy, it is not obvious how the sampled data can be used to provide unbiased, low-variance estimates of the properties. This paper therefore discusses how estimators that are Rao-Blackwellized can be used to achieve low error. This paper also presents the first analysis of the characteristics of the environmental phenomena that favor the ACS strategy and estimators. Quantitative experimental results in a mineral prospecting task simulation show that our approach is more efficient in exploration by yielding more minerals and information with fewer resources and providing more precise mineral density estimates than previous methods.
- Autonomic Mobile Sensor Network with Self-Coordinated Task Allocation and Execution.
Kian Hsiang Low, Wee Kheng Leow & Marcelo H. Ang, Jr.
IEEE Transactions on Systems, Man, and Cybernetics - Part C: Applications and Reviews
(Special Issue on Engineering Autonomic Systems), volume 36, issue 3, pages 315-327, May 2006.
Extended version of our IJCAI-03, ICRA'04, and
AAAI-04 papers
Andrew P. Sage Best Transactions Paper Award for the best paper published in IEEE Trans. SMC - Part A, B, and C in 2006
Abstract. This paper describes a distributed layered architecture for resource-constrained multirobot cooperation, which is utilized in autonomic mobile sensor network coverage. In the upper layer, a dynamic task allocation scheme self-organizes the robot coalitions to track efficiently across regions. It uses concepts of ant behavior to self-regulate the regional distributions of robots in proportion to that of the moving targets to be tracked in a nonstationary environment. As a result, the adverse effects of task interference between robots are minimized and network coverage is improved. In the lower task execution layer, the robots use self-organizing neural networks to coordinate their target tracking within a region. Both layers employ self-organization techniques, which exhibit autonomic properties such as self-configuring, self-optimizing, self-healing, and self-protecting. Quantitative comparisons with other tracking strategies such as static sensor placements, potential fields, and auction-based negotiation show that our layered approach can provide better coverage, greater robustness to sensor failures, and greater flexibility to respond to environmental changes.
- An Ensemble of Cooperative Extended Kohonen Maps for Complex Robot Motion Tasks.
Kian Hsiang Low, Wee Kheng Leow & Marcelo H. Ang, Jr.
Neural Computation, volume 17, issue 6, pages 1411-1445, Jun 2005.
Extended version of our AAMAS-02, ICRA'03, and
IJCAI-03 papers
Abstract. Self-organizing feature maps such as extended Kohonen maps (EKMs) have been very successful at learning sensorimotor control for mobile robot tasks. This letter presents a new ensemble approach, cooperative EKMs with indirect mapping, to achieve complex robot motion. An indirect-mapping EKM self-organizes to map from the sensory input space to the motor control space indirectly via a control parameter space. Quantitative evaluation reveals that indirect mapping can provide finer, smoother, and more efficient motion control than does direct mapping by operating in a continuous, rather than discrete, motor control space. It is also shown to outperform basis function neural networks. Furthermore, training its control parameters with recursive least squares enables faster convergence and better performance compared to gradient descent. The cooperation and competition of multiple self-organized EKMs allow a nonholonomic mobile robot to negotiate unforeseen, concave, closely spaced, and dynamic obstacles. Qualitative and quantitative comparisons with neural network ensembles employing weighted sum reveal that our method can achieve more sophisticated motion tasks even though the weighted-sum ensemble approach also operates in continuous motor control space.
- Task Allocation via Self-Organizing Swarm Coalitions in Distributed Mobile Sensor Network.
Kian Hsiang Low, Wee Kheng Leow & Marcelo H. Ang, Jr.
In Proceedings of the 19th National Conference on Artificial Intelligence (AAAI-04), pages 28-33, San Jose, CA, Jul 25-29, 2004.
26.7% acceptance rate
Abstract. This paper presents a task allocation scheme via self-organizing swarm coalitions for distributed mobile sensor network coverage. Our approach uses the concepts of ant behavior to self-regulate the regional distributions of sensors in proportion to that of the moving targets to be tracked in a non-stationary environment. As a result, the adverse effects of task interference between robots are minimized and sensor network coverage is improved. Quantitative comparisons with other tracking strategies such as static sensor placement, potential fields, and auction-based negotiation show that our approach can provide better coverage and greater flexibility to respond to environmental changes.
- Reactive, Distributed Layered Architecture for Resource-Bounded Multi-Robot Cooperation: Application to Mobile Sensor Network Coverage.
Kian Hsiang Low, Wee Kheng Leow & Marcelo H. Ang, Jr.
In Proceedings of the IEEE International Conference on Robotics and Automation (ICRA'04), pages 3747-3752, New Orleans, LA, Apr 26 - May 1, 2004.
Abstract. This paper describes a reactive, distributed layered architecture for cooperation of multiple resource-bounded robots, which is utilized in mobile sensor network coverage. In the upper layer, a dynamic task allocation scheme self-organizes the robot coalitions to track efficiently in separate regions. It uses the concepts of ant behavior to self-regulate the regional distributions of robots in proportion to that of the targets to be tracked in the changing environment. As a result, the adverse effects of task interference between robots are minimized and sensor network coverage is improved. In the lower layer, the robots use self-organizing neural networks to coordinate their target tracking within a region. Quantitative comparisons with other tracking strategies such as static sensor placements, potential fields, and auction-based negotiation show that our approach can provide better coverage and greater flexibility in responding to environmental changes.
- Continuous-Spaced Action Selection for Single- and Multi-Robot Tasks Using Cooperative Extended Kohonen Maps.
Kian Hsiang Low, Wee Kheng Leow & Marcelo H. Ang, Jr.
In Proceedings of the IEEE International Conference on Networking, Sensing and Control (ICNSC'04)
(Invited Paper to Special Session on Visual Surveillance), pages 198-203, Taipei, Taiwan, Mar 21-23, 2004.
Abstract. Action selection is a central issue in the design of behavior-based control architectures for autonomous mobile robots. This paper presents an action selection framework based on an assemblage of self-organizing neural networks called Cooperative Extended Kohonen Maps. This framework encapsulates two features that significantly enhance a robot's action selection capability: self-organization in the continuous state and action spaces to provide smooth, efficient and fine motion control; action selection via the cooperation and competition of Extended Kohonen Maps so that more complex motion tasks can be achieved. Qualitative and quantitative comparisons for both single- and multi-robot motion tasks show that our framework can provide better action selection than do action superposition methods.
- Action Selection for Single- and Multi-Robot Tasks Using Cooperative Extended Kohonen Maps.
Kian Hsiang Low, Wee Kheng Leow & Marcelo H. Ang, Jr.
In Proceedings of the 18th International Joint Conference on Artificial Intelligence (IJCAI-03), pages 1505-1506, Acapulco, Mexico, Aug 9-15, 2003.
27.6% acceptance rate
Abstract. This paper presents an action selection framework based on an assemblage of self-organizing neural networks called Cooperative Extended Kohonen Maps. This framework encapsulates two features that significantly enhance a robot's action selection capability: self-organization in the continuous state and action spaces to provide smooth, efficient and fine motion control; action selection via the cooperation and competition of Extended Kohonen Maps to achieve more complex motion tasks. Qualitative and quantitative comparisons for single- and multi-robot tasks show our framework can provide better action selection than do potential fields method.
- Action Selection in Continuous State and Action Spaces by Cooperation and Competition of Extended Kohonen Maps.
Kian Hsiang Low, Wee Kheng Leow & Marcelo H. Ang, Jr.
In Proceedings of the
2nd International Joint Conference on Autonomous Agents and MultiAgent Systems (AAMAS-03), pages 1056-1057, Melbourne, Australia, Jul 14-18, 2003.
Abstract. This paper presents an action selection framework based on an assemblage of self-organizing neural networks called Cooperative Extended Kohonen Maps. This framework encapsulates two features that significantly enhance a robot's action selection capability: self-organization in the continuous state and action spaces to provide smooth, efficient and fine motion control; action selection via the cooperation and competition of Extended Kohonen Maps to achieve more complex motion tasks. Qualitative tests demonstrate the capability of our action selection method for both single- and multi-robot motion tasks.
- Enhancing the Reactive Capabilities of Integrated Planning and Control with Cooperative Extended Kohonen Maps.
Kian Hsiang Low, Wee Kheng Leow & Marcelo H. Ang, Jr.
In Proceedings of the
IEEE International Conference on Robotics and Automation (ICRA'03), pages 3428-3433, Taipei, Taiwan, May 12-17, 2003.
Abstract. Despite the many significant advances made in robot motion research, few works have focused on the tight integration of high-level deliberative planning with reactive control at the lowest level. In particular, the real-time performance of existing integrated planning and control architectures is still not optimal because the reactive control capabilities have not been fully realized. This paper aims to enhance the low-level reactive capabilities of integrated planning and control with Cooperative Extended Kohonen Maps for handling complex, unpredictable environments so that the work-load of the high-level planner can be consequently eased. The enhancements include fine, smooth motion control, execution of more complex motion tasks such as overcoming unforeseen concave obstacles and traversing between closely spaced obstacles, and asynchronous execution of behaviors.
- A Hybrid Mobile Robot Architecture with Integrated Planning and Control.
Kian Hsiang Low, Wee Kheng Leow & Marcelo H. Ang, Jr.
In Proceedings of the
1st International Joint Conference on Autonomous Agents and MultiAgent Systems (AAMAS-02), pages 219-226, Bologna, Italy, Jul 15-19, 2002.
26% acceptance rate
Abstract. Research in the planning and control of mobile robots has received much attention in the past two decades. Two basic approaches have emerged from these research efforts: deliberative vs. reactive. These two approaches can be distinguished by their different usage of sensed data and global knowledge, speed of response, reasoning capability, and complexity of computation. Their strengths are complementary and their weaknesses can be mitigated by combining the two approaches in a hybrid architecture. This paper describes a method for goal-directed, collision-free navigation in unpredictable environments that employs a behavior-based hybrid architecture with asynchronously operating behavioral modules. It differs from existing hybrid architectures in two important ways: (1) the planning module produces a sequence of checkpoints instead of a conventional complete path, and (2) in addition to obstacle avoidance, the reactive module also performs target reaching under the control of a self-organizing neural network. The neural network is trained to perform fine, smooth motor control that moves the robot through the checkpoints. These two aspects facilitate a tight integration between high-level planning and low-level control, which permits real-time performance and easy path modification even when the robot is en route to the goal position.
- Integrated Planning and Control of Mobile Robot with Self-Organizing Neural Network.
Kian Hsiang Low, Wee Kheng Leow & Marcelo H. Ang, Jr.
In Proceedings of the
IEEE International Conference on Robotics and Automation (ICRA'02), pages 3870-3875, Washington, DC, May 11-15, 2002.
Abstract. Despite the many significant advances made in robotics research, few works have focused on the tight integration of task planning and motion control. Most integration works involve the task planner providing discrete commands to the low-level controller, which performs kinematics and control computations to command the motor and joint actuators. This paper presents a framework of the integrated planning and control for mobile robot navigation. Unlike existing integrated approaches, it produces a sequence of checkpoints instead of a complete path at the planning level. At the motion control level, a neural network is trained to perform motor control that moves the robot from one checkpoint to the next. This method allows for a tight integration between high-level planning and low-level control, which permits real-time performance and easy modification of motion path while the robot is enroute to the goal position.
|
TECHNICAL REPORTS
- Understanding and Improving Neural Architecture Search.
Yao Shu.
Ph.D. Thesis, Department of Computer Science, National University of Singapore, Jan 2022.
Abstract.
Over the past decade, various famous deep neural network (DNN) architectures have been devised and have achieved superhuman performance for a wide range of tasks. Designing these neural networks, however, typically incurs substantial efforts from domain experts by trials and errors. Such human efforts gradually become unaffordable with an increasing demand for customizing DNNs for different tasks. To this end, neural architecture search (NAS) has been widely applied to automate the design of neural networks in recent years. In the literature, a number of NAS algorithms have been proposed, aiming to further improve the search efficiency and effectiveness of NAS, i.e., to reduce the search cost and improve the generalization performance of the selected architectures, respectively. Despite these advances, there are still certain essential aspects in NAS that have not been well investigated in the literature, which however may help us to understand and even further improve popular NAS algorithms.
Firstly, only a few efforts have been devoted to understanding the neural architectures selected by popular NAS algorithms in the literature. In the first work of this thesis, we take the first step of understanding popular NAS algorithms by answering the following questions: What types of architectures are selected by popular NAS algorithms and why they are selected? In particular, we reveal that existing NAS algorithms (e.g., DARTS, ENAS) tend to favor architectures with wide and shallow cell structures. These favorable architectures consistently achieve fast convergence and are consequently selected by NAS algorithms. Our empirical and theoretical studies further confirm that their fast convergence derives from their smooth loss landscape and accurate gradient information. Nonetheless, these architectures may not necessarily lead to better generalization performance than other candidate architectures in the same search space, and therefore further improvement is possible by revising existing NAS algorithms.
Secondly, standard NAS algorithms typically aim to select only a single neural architecture from the search spaces and thus have overlooked the capability of other candidate architectures in helping improve the performance of their final selected architecture. To this end, we present two novel sampling algorithms under our Neural Ensemble Search via Bayesian Sampling (NESBS) framework that can effectively and efficiently select a well-performing ensemble of neural architectures from NAS search space. Compared with state-of-the-art NAS algorithms and other well-known ensemble search baselines, our NESBS algorithms are shown to be able to achieve improved performance in both classification and adversarial defense tasks on various benchmark datasets while incurring a comparable search cost to these NAS algorithms.
Thirdly, the search efficiency of popular NAS algorithms in the literature is severely limited by the need for model training during the search process. To overcome this limitation, we propose a novel NAS algorithm called NAS at Initialization (NASI) that exploits the capability of Neural Tangent Kernel (NTK) in being able to characterize the converged performance of candidate architectures at initialization, hence allowing model training to be completely avoided to boost the search efficiency. Besides the improved search efficiency, NASI also achieves competitive search effectiveness on various datasets like CIFAR-10/100 and ImageNet. Further, NASI can guarantee the benefits of being label- and data-agnostic under mild conditions, i.e., the provable transferability of architectures selected by our NASI over different datasets.
Finally, though recent NAS algorithms using training-free metrics are able to select well-performing architectures in practice, the reason why training-free NAS using these metrics performs well and the answer to the question of how training-free NAS can be further boosted still have not been fully understood. To this end, we provide a unified theoretical analysis for gradient-based training-free NAS in this paper to understand why training-free metrics work well in practice. By exploiting these theoretical understandings, we then develop a novel NAS framework called Hybrid Neural Architecture Search (HNAS) that consistently improves training-free NAS in a principled way. Remarkably, HNAS can enjoy the advantages of both training-free (i.e., the superior search efficiency) and training-based NAS (i.e., the remarkable search effectiveness), which we have demonstrated through extensive experiments.
- Exploiting Gradient Information for Modern Machine Learning Problems.
Yizhou Chen.
Ph.D. Thesis, Department of Computer Science, National University of Singapore, Jan 2022.
Abstract. Many deep learning achievements are attributed to the back-propagation (BP) algorithm, which exploits gradient information of the deep neural network (DNN) models: BP efficiently computes the gradient of the loss function with respect to the weights of a DNN for a batch of examples, and such gradient can be used by stochastic gradient descent to perform learning / optimization of the DNN model. Despite recent advances in deep learning like DNN training, there are still important scenarios where we can also use gradient to tackle optimization difficulty. In a broader aspect of deep learning rather than DNN training, a significant challenge faced by ML practitioners is thus whether we can design efficient algorithms to use the model gradient in the training / optimization in various deep learning scenarios. This thesis identifies four important scenarios and, for each of them, proposes a novel algorithm to utilize the gradient information for effective optimization that is both theoretically grounded and practically effective.
Firstly, the training process of a machine learning (ML) model may be subject to adversarial attacks from an attacker who attempts to undermine the test performance of the ML model by perturbing the training minibatches, and thus needs to be protected by a defender. Such a problem setting is referred to as training-time adversarial ML. We formulate it as a two-player game and propose a principled Recursive Reasoning-based Training-Time adversarial ML (R2T2) framework to model this game. R2T2 models the reasoning process between the attacker and the defender and captures their bounded reasoning capabilities (due to bounded computational resources) through the recursive reasoning formalism. In particular, we associate a deeper level of recursive reasoning with the use of a higher-order gradient to derive the attack (defense) strategy, which naturally improves its performance while requiring greater computational resources. R2T2 can empirically achieve state-of-the-art attack and defense performances on benchmark image datasets.
Secondly, probabilistic modeling with neural network architectures constitute a well-studied and popular area of deep learning. In contrast to a frequentist approach which is easy to overfit to the available dataset and risk learning unwanted biasing in the dataset, Gaussian process (GP) models were introduced as a fully probabilistic substitute and is one of the dominant approaches in Bayesian learning. A multi-layer deep Gaussian process (DGP) model is a hierarchical composition of GP models with a greater expressive power, and is more useful when dealing with complicated dataset. Exact DGP inference is intractable, and the approximation methods either yields a biased posterior belief (deterministic approximation by variational inference) or is computationally costly (stochastic approximation by Monte Carlo sampling). These difficulties have motivated our recent development of an implicit posterior variational inference (IPVI) framework for DGPs that can ideally recover an unbiased posterior belief and still preserve time efficiency. However, as a generator and a discriminator are integrated in each layer of the DGP, the training becomes unstable and is prone to optimization difficulties. To resolve such issues, we propose a novel gradient-bridging architecture of the generator and discriminator for the DGP model, which uses the inducing inputs as the context, thus leads to faster training and more accurate predictions. Empirical evaluation shows that IPVI with our proposed architecture outperforms the state-of-the-art methods for DGPs.
Thirdly, many widely adopted Bayesian meta-learning frameworks model the uncertainty in the predictions with a set of particles or a variational distribution (of the
meta-parameters), which does not allow latent task modeling.1 We present a novel
implicit process-based meta-learning (IPML) algorithm that, in contrast to existing works, explicitly represents each task as a continuous latent vector and models its probabilistic belief within the highly expressive implicit processes (IP) framework. IP is a stochastic process with highly flexible implicit priors over functions, and is suitable as a Bayesian (meta) learning model for complicated datasets (e.g., when the priors are non-Gaussian unlike in GP). We tackle the meta-training in IPML with a novel expectation-maximization algorithm based on the stochastic gradient Hamiltonian Monte Carlo sampling method. Our delicate design of the neural network architecture for meta-training in IPML allows competitive meta-learning performance to be achieved. Unlike existing works, IPML offers the benefits of being amenable to the characterization of a principled distance measure between tasks using the maximum mean discrepancy, active task selection without needing the assumption of known task contexts, and synthetic task generation by modeling task-dependent input distributions. Empirical evaluation on benchmark datasets shows that IPML outperforms existing Bayesian meta-learning algorithms.
Last but not least, in the problem of active task selection which involves selecting the most informative tasks for meta-learning, we propose a novel active task selection criterion based on the mutual information between latent task vectors. Unfortunately, such a criterion scales poorly in the number of candidate tasks when optimized. To resolve this issue, we exploit the submodularity property of our new criterion for devising the first active task selection algorithm for meta-learning with a near-optimal performance guarantee. To further improve our efficiency, we propose an online variant of the Stein variational gradient descent to perform fast belief updates of the meta-parameters via maintaining a set of forward (and backward) particles when learning (or unlearning) from each selected task. We empirically demonstrate the superior performance of our proposed algorithm on real-world datasets.
- Sample-Efficient Automated Machine Learning with Bayesian Optimization.
Zhongxiang Dai.
Ph.D. Thesis, Department of Computer Science, National University of Singapore, Jul 2021.
Abstract. Automated hyperparameter optimization of machine learning (ML) models, referred to as AutoML, has been a challenging problem for practitioners, mainly due to the high computational cost of training modern ML models and the lack of gradient information with respect to the model hyperparameters. To this end, the black-box optimization method of Bayesian optimization (BO) has become a prominent method for optimizing the hyperparameters of ML models, which can be attributed to its impressive sample efficiency and theoretical convergence guarantee. Despite recent advances, there are still important scenarios where we can further improve the sample efficiency of BO for AutoML by exploiting naturally available auxiliary information, or extend the applicability of BO to other ML tasks. This thesis identifies five such important scenarios and, for each of them, proposes a novel BO algorithm that is both theoretically grounded and practically effective.
Firstly, many ML models require an iterative training process, which requires every hyperparameter evaluation during BO to run for a certain number of training epochs. As a result, the auxiliary observations from intermediate training epochs can be exploited to early-stop the evaluations of those unpromising hyperparameter configurations to save resource. We propose the BO with Bayesian optimal stopping (BO-BOS) algorithm, which incorporates BOS into BO in order to improve the epoch efficiency of BO using a principled optimal stopping mechanism. BO-BOS preserves the asymptotic no-regret property of BO with our specified setting of BOS parameters which is amenable to an elegant interpretation in terms of the exploration-exploitation trade-off, and performs competitively in a number of AutoML experiments.
Secondly, the widely celebrated federated learning (FL) setting requires first-order optimization techniques, and is hence unable to handle zeroth-order optimization tasks such as hyperparameter optimization. We extend BO into the FL setting (FBO) and derive the federated Thompson sampling (FTS) algorithm, to improve the efficiency of BO in the FL setting by employing auxiliary information from other agents. FTS tackles a number of major challenges faced by FBO in a principled way: FTS uses random Fourier features approximation to derive the parameters to be communicated in order to avoid sharing the raw data, adopts the Thompson sampling algorithm which reduces the number of parameters to be exchanged, and is robust against heterogeneous agents due to a robust theoretical convergence guarantee.
Thirdly, the above-mentioned FTS algorithm, unfortunately, is not equipped with a rigorous privacy guarantee, which is an important consideration in FL. To this end, we integrate differential privacy (DP) into FTS through a general framework for adding DP to iterative algorithms. Moreover, we leverage the ability of this general DP framework to handle different parameter vectors, as well as the technique of local modeling for BO, to further improve the utility of our algorithm through distributed exploration (DE). The resulting DP-FTS-DE algorithm is able to improve an agent’s sample efficiency by exploiting auxiliary information from other agents, while rigorously hiding its participation in the algorithm. DP-FTS-DE is amenable to a number of interesting theoretical insights regarding the privacy-utility trade-off, and achieves competitive utilities with strong privacy guarantees in real-world experiments.
Fourthly, when BO is used for hyperparameter optimization using a dataset, we often have access to previous completed hyperparameter optimization tasks using other potentially related datasets. This prompts the question as to whether we can leverage these previous completed tasks to improve the efficiency of the current BO task through meta-learning, while ensuring its robustness against dissimilar tasks. We introduce a scalable, principled and robust meta-BO algorithm called robust meta-Gaussian process-upper confidence bound (RM-GP-UCB). We show that RM-GP-UCB is asymptotically no-regret even when all previous tasks are dissimilar to the current task, and is amenable to a principled method to learn the weights assigned to the individual previous tasks through regret minimization via online learning. RM-GP-UCB achieves effective performances in a wide range of real-world experiments.
Lastly, many ML tasks such as adversarial ML can be modeled as repeated games between boundedly rational, self-interested agents with unknown, complex, and costly-to-evaluate payoff functions. We introduce a recursive reasoning formalism of BO, called Recursive Reasoning-Based BO (R2-B2), which extends the applicability of BO to provide efficient strategies for players in this type of game. Under certain conditions, using R2-B2 to reason at one level higher than the other agents achieves faster asymptotic convergence to no regret than without using recursive reasoning. R2-B2 performs effectively in practice in adversarial ML and multi-agent reinforcement learning experiments.
- Automated Machine Learning: New Advances on Bayesian Optimization.
Dmitrii Kharkovskii.
Ph.D. Thesis, Department of Computer Science, National University of Singapore, Dec 2020.
Abstract. Recent advances in Bayesian optimization (BO) have delivered a promising suite of tools for optimizing an unknown expensive to evaluate black-box objective function with a finite budget of evaluations. A significant advantage of BO is its general formulation: BO can be utilized to optimize any black-box objective function. As a result, BO has been applied in a wide range of applications such as automated machine learning, robotics or environmental monitoring, among others. Furthermore, its general formulation makes BO attractive for deployment in new applications. However, potential new applications can have additional requirements not satisfied by the classical BO setting. In this thesis, we aim to address some of these requirements in order to scale up BO technology for the practical use in new real-world applications.
Firstly, this thesis tackles the problem of data privacy, which is not addressed by the standard setting of BO. Specifically, we consider the outsourced setting where the entity holding the dataset and the entity performing BO are represented by different parties, and the dataset cannot be released non-privately. For example, a hospital holds a dataset of sensitive medical records and outsources the BO task on this dataset to an industrial AI company. We present the private-outsourced-Gaussian process-upper confidence bound (PO-GP-UCB) algorithm, which is the first algorithm for privacy-preserving BO in the outsourced setting with a provable performance guarantee. The key idea of our approach is to make the BO performance of our algorithm similar to that of non-private GP-UCB run using the original dataset, which is achieved by using a random projection-based transformation that preserves both privacy and the pairwise distances between inputs. Our main theoretical contribution is to show that a regret bound similar to that of the standard GP-UCB algorithm can be established for our PO-GP-UCB algorithm. We empirically evaluate the performance of our algorithm with synthetic and real-world datasets.
Secondly, we consider applications of BO for hotspot sampling in spatially varying phenomena. For such applications, we exploit the structure of the spatially varying phenomenon in order to increase the BO lookahead and, as a result, improve the performance of the algorithm and make it more suitable for practical use in real-world scenarios. To do this, we present a principled multi-staged Bayesian sequential decision algorithm for nonmyopic adaptive BO that, in particular, exploits macro-actions for scaling up to a further lookahead to match up to a larger available budget. To achieve this, we first generalize GP-UCB to a new acquisition function defined with respect to a nonmyopic adaptive macro-action policy, which, unfortunately, is intractable to be optimized exactly due to an uncountable set of candidate outputs. The key novel contribution of our work here is to show that it is in fact possible to solve for a nonmyopic adaptive ε-Bayes-optimal macro-action BO (ε-Macro-BO) policy given an arbitrary user-specified loss bound ε via stochastic sampling in each planning stage which requires only a polynomial number of samples in the length of macro-actions. To perform nonmyopic adaptive BO in real time, we then propose an asymptotically optimal anytime variant of our ε-Macro-BO algorithm with a performance guarantee. Empirical evaluation on synthetic and real-world datasets shows that our proposed approach outperforms existing state-of-the-art algorithms.
Finally, this thesis proposes a black-box attack for adversarial machine learning based on BO. Since the dimension of the inputs in adversarial learning is usually too high for applying BO directly, our proposed attack applies dimensionality reduction and searches for an adversarial perturbation in a low-dimensional latent space. The key idea of our approach is to automate both the selection of the latent space dimen- sion and the search of the adversarial perturbation in the selected latent space by using BO. Additionally, we use Bayesian optimal stopping to boost the query efficiency of our attack. Performance evaluation using image classification datasets shows that our proposed method outperforms the state-of-the-art black-box adversarial attacks.
- New Advances in Bayesian Inference for Gaussian Process and Deep Gaussian Process Models.
Haibin Yu.
Ph.D. Thesis, Department of Computer Science, National University of Singapore, May 2020.
Abstract.
Machine learning is the study of letting computers learn to perform a specific task in a data-driven manner. In particular, Bayesian machine learning has attracted enor- mous attention mainly due to their ability to provide uncertainty estimates following Bayesian inference. This thesis focuses on Gaussian processes (GPs), a rich class of Bayesian nonparametric models for performing Bayesian machine learning with formal measures of predictive uncertainty.
However, the applicability of GP in large datasets and in hierarchical composition of GPs is severely limited by computational issues and intractabilities. Therefore, it is crucial to develop accurate and efficient inference algorithms to address these challenges. To this end, this thesis aims at proposing a series of novel approximate Bayesian inference methods for a wide variety of GP models, which unifies the previous literatures, significantly extends them and hopefully lays the foundation for future inference methods.
To start with, this thesis presents a unifying perspective of existing inducing variables-based GP models, sparse GP (SGP) models and variational inference for SGP models (VSGP). Then, to further mitigate the issue of overfitting during optimization, we present a novel variational inference framework for deriving a family of Bayesian SGP regression models, referred to as variational Bayesian SGP (VBSGP) regression models.
Next, taking into account the fact that the expressiveness of GP and SGP depends heavily on the design of the kernel function, we further extend the expressive power of GP by introducing Deep GP (DGP), which is a hierarchical composition of GP models. Unfortunately, exact inference in DGP is intractable, which has motivated the recent development of deterministic and stochastic approximation methods. However, the deterministic approximation methods yield a biased posterior belief while the stochastic one is computationally costly. In this regard, we present the implicit posterior variational inference (IPVI) framework for DGPs that can ideally recover an unbiased posterior belief and still preserve time efficiency. Inspired by generative adversarial networks, our IPVI framework casts the DGP inference problem as a two- player game in which a Nash equilibrium, interestingly, coincides with an unbiased posterior belief.
We hope this thesis at least provides additional confidence and clarity for researchers who are devoting themselves to Bayesian nonparametric models, Gaussian process models in particular. Moreover, we also wish this thesis to offer inspirations for future works, and some thoughts that could be useful for future solutions.
- Data-Efficient Machine Learning with Multiple Output Types and High Input Dimensions.
Yehong Zhang.
Ph.D. Thesis, Department of Computer Science, National University of Singapore, Dec 2017.
Abstract.
Recent research works in machine learning (ML) have focused on learning some target variables of interest to achieve competitive (or state-of-the-art) predictive performance in less time but without requiring large quantities of data, which is known as data-efficient ML. This thesis focuses on two important data-efficient ML approaches: active learning (AL) and Bayesian optimization (BO) which, instead of learning passively from a given small set of data, need to select and gather the most informative observations for learning the target variables of interest more accurately given some budget constraints. To advance the state-of-the-art of data-efficient ML, novel generalizations of AL and BO algorithms are proposed in this thesis for addressing the issues arising from multiple output types and high input dimensions which are the practical settings in many real-world applications.
In particular, this thesis aims to (a) exploit the auxiliary types of outputs which usually coexist and correlate well with the target output types, and more importantly, are less noisy and/or less tedious to sample for improving the learning performance of the target output type in both AL and BO algorithms and (b) scale up the state-of-the-art BO algorithm to high input dimensions. To achieve this, the specific data with multiple output types or high input dimensions is represented using some form of Gaussian process (GP)-based probabilistic regression models which allow the predictive uncertainty of the outputs to be formally quantified and consequently exploited for developing efficient AL and BO algorithms.
To achieve above objectives, an AL algorithm of multi-output GP (MOGP) is first developed for minimizing the predictive uncertainty (i.e., posterior joint entropy) of the target output type. In contrast to existing works, our AL problems involve selecting not just the most informative sampling inputs to be observed but also the types of outputs at each selected input for improving the learning performance of only the target output type given a sampling budget. Unfortunately, such an entropy criterion scales poorly in the numbers of candidate sampling inputs and selected observations when optimized. To resolve this issue, we exploit a structure common to sparse MOGP models for deriving a novel AL criterion. Furthermore, we exploit a relaxed form of submodularity property of our new criterion for devising a polynomial-time approximation algorithm that guarantees a constant-factor approximation of that achieved by the optimal set of selected observations. Empirical evaluation on real-world datasets shows that our proposed approach outperforms existing algorithms for AL of MOGP and single-output GP models.
Secondly, to boost the BO performance by exploiting the cheaper and/or less noisy observations of some auxiliary functions with varying fidelities, we proposed a novel generalization of predictive entropy search (PES) for multi-fidelity BO called multi-fidelity PES (MF-PES). In contrast to existing multi-fidelity BO algorithms, our proposed MF-PES algorithm can naturally trade off between exploitation vs. exploration over the target and auxiliary functions with varying fidelities without needing to manually tune any such parameters. To achieve this, we model the unknown target and auxiliary functions jointly as a convolved MOGP (CMOGP) whose convolutional structure is exploited to formally characterize the fidelity of each auxiliary function through its cross-correlation with the target function. Although the exact acquisition function of MF-PES cannot be computed in closed form, we show that it is in fact possible to derive an efficient approximation of MF-PES via a novel multi-output random features approximation of the CMOGP model whose cross-correlation (i.e., multi-fidelity) structure between the target and auxiliary functions can be exploited for improving the belief of the global target maximizer using the observations from evaluating these functions. Practical constraints are proposed to relate the global target maximizer to that of auxiliary functions. Empirical evaluation on synthetic and real-world experiments shows that MF-PES outperforms the state-of-the-art multi-fidelity BO algorithms.
Lastly, to improve the BO performance in real-world applications with high input
dimensions (e.g., computer vision, biology), we generalize PES for high-dimensional BO by exploiting an additive structure of the target function. New practical constraints are proposed and approximated efficiently such that the proposed acquisition function of additive PES (add-PES) can be optimized independently for each local and low-dimensional input component. The empirical results show that our add-PES considerably improves the performance of the state-of-the-art high-dimensional BO algorithms by using a simple and common setting for optimizing different tested functions with varying input dimensions, which makes it a superior alternative to existing high-dimensional BO algorithms.
- Exploiting Decentralized Multi-Agent Coordination for Large-Scale Machine Learning Problems.
Ruofei Ouyang.
Ph.D. Thesis, Department of Computer Science, National University of Singapore, Dec 2016.
Abstract.
Nowadays, the scale of machine learning problems becomes much larger than before. It raises a huge demand in distributed perception and distributed computation. A multi-agent system provides exceptional scalability for problems like active sensing and data fusion. However, many rich characteristics of large-scale machine learning problems have not been addressed yet such as large input domain, nonstationarity, and high dimensionality. This thesis identifies the challenges related to these characteristics from multi-agent perspective. By exploiting the correlation structure of data in large-scale problems, we propose multi-agent coordination schemes that can improve the scalability of the machine learning models while preserving the computation accuracy. To elaborate, the machine learning problems we are solving with multi-agent coordination techniques are:
- Gaussian process regression. To perform distributed regression on a large-scale environmental phenomenon, data compression is often required due to the communication costs. Currently, decentralized data fusion methods encapsulate the data into local summaries based on a fixed support set. However in a large-scale field, this fixed support set, acting as a centralized component in the decentralized system, cannot approximate the correlation structure of the entire phenomenon well. It leads to evident losses in data summarization. Consequently, the regression performance will be significantly reduced.
In order to approximate the correlation structure accurately, we propose an agent-centric support set to allow every agent in the data fusion system to choose a possibly different support set and dynamically switch to another one during execution for encapsulating its own data into a local summary which, perhaps surprisingly, can still be assimilated with the other agents’ local summaries into a globally consistent summary. Together with an information sharing mechanism we designed, the new decentralized data fusion methods with agent-centric support set can be applied to regression problems on a much larger environmental phenomenon with high performance.
- Active learning. In the context of environmental sensing, active learning/active sensing is a process of taking observations to minimize the uncertainty in an environmental field. The uncertainty is quantified based on the correlation structure of the phenomenon which is traditionally assumed to be stationary for computational sake. In a large-scale environmental field, this stationary assumption is often violated. Therefore, existing active sensing algorithms perform sub-optimally for a nonstationary environmental phenomenon.
To the best of our knowledge, our decentralized multi-robot active sensing (DEC-MAS) algorithm is the first work to address nonstationarity issue in the context of active sensing. The uncertainty in the phenomenon is quantified based on the nonstationary correlation structure estimated by Dirichlet process mixture of Gaussian processes. Further, our DEC-MAS algorithm can efficiently coordinate the exploration of multiple robots to automatically trade-off between learning the unknown, nonstationary correlation structure and minimizing the uncertainty of the environmental phenomenon. It enables multi-agent active sensing techniques to be applied to a large-scale nonstationary environmental phenomenon.
- Bayesian optimization. Optimizing an unknown objective function is challenging for traditional optimization methods. Alternatively, in this situation, people use Bayesian optimization which is a modern optimization technique that can optimize a function by only utilizing the observation information (input and output values) collected through simulations. When the input dimension of the function is low, a few simulated observations can generate good result already. However, for high dimensional function, a huge number of observations are required which is impractical when the simulation consumes lots of time and resources.
Fortunately, many high dimensional problems have sparse correlation structure. Our ANOVA-DCOP work can decompose the correlation structure in the original high-dimensional problem into many correlation structures of subsets of dimensions based on ANOVA kernel function. It significantly reduces the size of input space into a collection of lower-dimensional subspaces. Additionally, we reformulate the Bayesian optimization problem as a decentralized constrained optimization problem (DCOP) that can be efficiently solved by multi-agent coordination techniques so that it can scale up to problems with hundreds of dimensions.
- New Advances on Bayesian and Decision-Theoretic Approaches for Interactive Machine Learning.
Trong Nghia Hoang.
Ph.D. Thesis, Department of Computer Science, National University of Singapore, Feb 2015.
Abstract.
The exploration-exploitation trade-off is a fundamental dilemma in many interactive learning scenarios which include both aspects of reinforcement learning (RL) and active learning (AL): An autonomous agent, situated in an unknown environment, has to actively extract knowledge from the environment by taking actions (or conducting experiments) based on its previously collected information to make accurate predictions or to optimize some utility functions. Thus, to make the most effective use of their resource-constrained budget (e.g., processing time, experimentation cost), the agent must choose carefully between (a) exploiting options (e.g., actions, experiments) which are recommended by its current, possibly incomplete model of the environment, and (b) exploring the other ostensibly sub-optimal choices to gather more information.
For example, an RL agent has to face a dilemma between (a) exploiting the most-rewarding action according to the current statistical model of the environment at the risk of running into catastrophic situations if the model is not accurate, and (b) exploring a sub-optimal action to gather more information so as to improve the model's accuracy at the potential price of losing the short-term reward. Similarly, an AL algorithm/agent has to consider between (a) conducting the most informative experiments according to its current estimation of the environment model's parameters (i.e., exploitation), and (b) running experiments that help improving the estimation accuracy of these parameters (i.e., exploration).
More often, learning strategies that ignore exploration will likely exhibit sub-optimal performance due to their imperfect knowledge while, conversely, those that entirely focus on exploration might suffer the cost of learning without benefitting from it. Therefore, a good exploration-exploitation trade-off is critical to the success of those interactive learning agents: In order to perform well, they must strike the right balance between these two conflicting objectives. Unfortunately, while this trade-off has been well-recognized since the early days of RL, the studies of exploration-exploitation have mostly been developed for theoretical settings in the respective field of RL and, perhaps surprisingly, glossed over in the existing AL literature. From a practical point of view, we see three limiting factors:
- Previous works addressing the exploration-exploitation trade-off in RL have largely focused on simple choices of the environment model and consequently, are not practical enough to accommodate real-world applications that have far more complicated environment structures. In fact, we find that most recent advances in Bayesian reinforcement learning (BRL) have only been able to analytically trade off between exploration and exploitation under a simple choice of models such as Flat-Dirichlet-Multinomial (FDM) whose independence and modeling assumptions do not hold for many real-world applications.
- Nearly all of the notable works in the AL literature primarily advocate the use of greedy/myopic algorithms whose rates of convergence (i.e., the number of experiments required by the learning algorithm to achieve a desired performance in the worst case) are provably minimax optimal for simple classes of learning tasks (e.g., threshold learning). While these results have greatly ad- vanced our understanding about the limit of myopic AL in worst-case scenarios, significantly less is presently known about whether it is possible to devise nonmyopic AL strategies which optimize the exploration-exploitation trade-off to achieve the best expected performance in budgeted learning scenarios.
- The issue of scalability of the existing predictive models (e.g., Gaussian processes) used in AL has generally been underrated since the majority of literature considers small-scale environments which only consist of a few thousand candidate experiments to be selected by single-mode AL algorithms one at a time prior to retraining the model. In contrast, large-scale environments usually have a massive set of million candidate experiments among which tens or hundreds of thousands should be actively selected for learning. For such data-intensive problems, it is often more cost-effective to consider batch-mode AL algorithms which select and conduct multiple experiments in parallel at each stage to collect observations in batch. Retraining the predictive model after incorporating each batch of observations then becomes a computational bottleneck as the collected dataset at each stage quickly grows up to tens or even hundreds of thousand data points.
This thesis outlines some recent progresses that we have been able to make while working toward satisfactory answers to the above challenges, along with practical algorithms that achieve them:
- In particular, in order to put BRL into practice for more complicated and practical problems, we propose a novel framework called Interactive Bayesian Reinforcement Learning (I-BRL) to integrate the general class of parametric models and model priors, thus allowing the practitioners' domain knowledge to be exploited to produce a fine-grained and compact representation of the environment as often required in many real-world applications. Interestingly, we show how the nonmyopic Bayes-optimal policy can be derived analytically by solving I-BRL exactly and propose an approximation algorithm to compute it efficiently in polynomial time. Our empirical studies show that the proposed approach performs competitively with the existing state-of-the-art algorithms.
- Then, to establish a theoretical foundation for the exploration-exploitation trade-off in single-mode active learning scenarios with resource-constrained budgets, we present a novel ϵ-Bayes-optimal Decision-Theoretic Active Learning (ϵ-BAL) framework which advocates the use of differential entropy as a performance measure and consequently, derives a learning policy that can approximate the optimal expected performance arbitrarily closely (i.e., within an arbitrary loss bound ϵ). To meet the real-time requirement in time-critical applications, we then propose an asymptotically ϵ-optimal, branch-and-bound anytime algorithm based on ϵ-BAL with performance guarantees. In practice, we empirically demonstrate with both synthetic and real-world datasets that the proposed approach outperforms the state-of-the-art algorithms in budgeted scenarios.
- Lastly, to facilitate the future developments of large-scale, nonmyopic AL applications, we further introduce a highly scalable family of anytime predictive models for AL which provably converge toward a well-known class of sparse Gaussian processes (SGPs). Unlike the existing predictive models of AL which cannot be updated incrementally and are only capable of processing middle-sized datasets (i.e., a few thousands of data points), our proposed models can process massive datasets in an anytime fashion, thus providing a principled trade-off between the processing time and the predictive accuracy. The efficiency of our framework is then demonstrated empirically on a variety of large-scale real-world datasets which contains hundreds of thousand data points.
- Gaussian Process-Based Decentralized Data Fusion and Active Sensing Agents: Towards Large-Scale Modeling and Prediction of Spatiotemporal Traffic Phenomena.
Jie Chen.
Ph.D. Thesis, Department of Computer Science, National University of Singapore, Dec 2013.
Abstract.
Knowing and understanding the environmental phenomena is important to many real world applications. This thesis is devoted to study large-scale modeling and prediction of spatiotemporal environmental phenomena (i.e., urban traffic phenomena). Towards this goal, our proposed approaches rely on a class of Bayesian non-parametric models: Gaussian processes (GP).
To accurately model spatiotemporal urban traffic phenomena in real world situation, a novel relational GP taking into account both the road segment features and road network topology information is proposed to model real world traffic conditions over road network. Additionally, a GP variant called log-Gaussian process (lGP) is exploited to model an urban mobility demand pattern which contains skewness and extremity in demand measurements.
To achieve efficient and scalable urban traffic phenomenon prediction given a large phenomenon data, we propose three novel parallel GPs: parallel partially independent training conditional (pPITC), parallel partially independent conditional(pPIC) and parallel incomplete Cholesky factorization (pICF)-based approximations of GP model, which can distribute their computational load into a cluster of parallel/multi-core machines, thereby achieving time efficiency. The predictive performances of such parallel GPs are theoretically guaranteed to be equivalent to that of some centralized approaches to approximate full/exact GP regression. The proposed parallel GPs are implemented using the message passing interface (MPI) framework and tested on two large real world datasets. The theoretical and empirical results show that our parallel GPs achieve significantly better time efficiency and scalability than that of full GP, while achieving comparable accuracy. They also achieve fine speedup performance that is the ratio of time required by the parallel algorithms and their centralized counterparts.
To exploit active mobile sensors to perform decentralized perception of the spatiotemporal urban traffic phenomenon, we propose a decentralized algorithm framework: Gaussian process-based decentralized data fusion and active sensing (D2FAS) which is composed of a decentralized data fusion (DDF) component and a decentralized active sensing (DAS) component. The DDF component includes a novel Gaussian process-based decentralized data fusion (GP-DDF) algorithm that can achieve remarkably efficient and scalable prediction of phenomenon and a novel Gaussian process-based decentralized data fusion with local augmentation (GP-DDF+) algorithm that can achieve better predictive accuracy while preserving time efficiency of GP-DDF. The predictive performances of both GP-DDF and GP-DDF+ are theoretically guaranteed to be equivalent to that of some sophisticated centralized sparse approximations of exact/full GP. For the DAS component, we propose a novel partially decentralized active sensing (PDAS) algorithm that exploits property in correlation structure of GP-DDF to enable mobile sensors cooperatively gathering traffic phenomenon data along a near-optimal joint walk with theoretical guarantee, and a fully decentralized active sensing (FDAS) algorithm that guides each mobile sensor gather phenomenon data along its locally optimal walk.
Lastly, to justify the practicality of the D2FAS framework, we develop and test D2FAS algorithms running with active mobile sensors on real world datasets for monitoring traffic conditions and sensing/servicing urban mobility demands. Theoretical and empirical results show that the proposed algorithms are significantly more time-efficient, more scalable in the size of data and in the number of sensors than the state-of-the-art centralized approaches, while achieving comparable predictive accuracy.
- A Decision-Theoretic Approach for Controlling and Coordinating Multiple Active Cameras in Surveillance.
Prabhu Natarajan.
Ph.D. Thesis, Department of Computer Science, National University of Singapore, Dec 2013.
Abstract.
The use of active cameras in surveillance is becoming increasingly popular as they try to meet the demands of capturing high-resolution images/videos of targets in surveillance for face recognition, target identification, forensic video analysis, etc. These active cameras are endowed with pan, tilt, and zoom capabilities, which can be exploited to provide high-quality surveillance. In order to achieve effective, real-time surveillance, an efficient collaborative mechanism is needed to control and coordinate these cameras' actions, which is the focus of this thesis. The central problem in surveillance is to monitor a set of targets with guaranteed image resolution. Controlling and coordinating multiple active cameras to achieve this surveillance task is non-trivial and challenging because: (a) presence of inherent uncertainties in the surveillance environment (targets motion, location, and noisy camera observation); (b) there exists a non-trivial trade-off between number of targets and the resolution of observing these targets; and (c) more importantly, the coordination framework should be scalable with increasing number of targets and cameras.
In this thesis, we formulate a novel decision-theoretic multi-agent planning approach for controlling and coordinating multiple active cameras in surveillance. Our decision-theoretic approach offers advantages of (a) accounting the uncertainties using probabilistic models; (b) the non-trivial trade-off is addressed by coordinating the active cameras' actions to maximize the number of targets with guaranteed resolution; and (c) the scalability in number of targets and cameras is achieved by exploiting the structures and properties that are present in our surveillance problem. We focus on two novel problems in active camera surveillance: (a) maximizing observations of multiple targets (MOMT), i.e., maximizing the number of targets observed in active cameras with guaranteed image resolution; and (b) improving fairness in observation of multiple targets (FOMT), i.e., no target is "starved" of observation by active cameras for long duration of time.
We propose two formal decision-theoretic frameworks (a) Markov Decision Process (MDP) and (b) Partially Observable Markov Decision Process (POMDP) frameworks for coordinating active cameras in surveillance. MDP framework controls active cameras in fully observable surveillance environments where the active cameras are supported by one or more wide-view static/fixed cameras to observe the entire surveillance environment at low-resolution. POMDP framework controls active cameras in partially observable surveillance environments where it is impractical to observe the entire surveillance environment using static/fixed cameras due to occlusions caused by physical infrastructures. Hence the POMDP framework do not have a complete view of the surveillance environment.
Specifically, we propose (a) MDP frameworks to solve MOMT problem and FOMT problem in fully observable surveillance environment; and (b) POMDP framework to solve MOMT problem in partially observable surveillance environment. As proven analytically, our MDP and POMDP frameworks incurs time that is linear in number of targets to be observed during surveillance. We have used max-plus algorithm with our MDP framework to improve its scalability in number of cameras for MOMT problem. Empirical evaluation through simulations in realistic surveillance environment reveals that our proposed approach can achieve high-quality surveillance in real time. We also demonstrate our pro- posed approach with real Axis 214 PTZ cameras to show the practicality of our approach in real world surveillance. Both the simulations and real camera experiments show that our decision-theoretic approach can control and coordinate active cameras efficiently and hence contributes significantly towards improving the active camera surveillance research.
- Information-Theoretic Multi-Robot Path Planning.
Nannan Cao.
M.Sc. Thesis, Department of Computer Science, National University of Singapore, Sep 2012.
Abstract.
Research in environmental sensing and monitoring is especially important in supporting environmental sustainability efforts worldwide, and has recently attracted significant attention and interest. A key direction of this research lies in modeling and predicting the spatiotemporally varying environmental phenomena. One approach is to use a team of robots to sample the area and model the measurement values at unobserved points. For smoothly varying and hotspot fields, there is some work which has been done to model the fields well. However, there is still a class of common environmental fields called anisotropic fields in which the spatial phenomena are highly correlated along one direction and less correlated along the perpendicular direction. We exploit the environmental structure to improve the sampling performance and time efficiency of planning for anisotropic fields.
In this thesis, we cast the planning problem into a stagewise decision-theoretic problem. we adopt Gaussian Process to model spatial phenomena. Maximum entropy criterion and maximum mutual information criterion are used to measure the informativeness of the observation paths. It is found that for many GPs, correlation of two points exponentially decreases with the distance between the two points. With this property, for maximum entropy criterion, we propose a polynomial-time approximation algorithm, MEPP, to find the maximum entropy paths. We also provide a theoretical performance guarantee for this algorithm. For maximum mutual information criterion, we propose another polynomial-time approximation algorithm, M2IPP. Similar to the MEPP, a performance guarantee is also provided for this algorithm. We demonstrate the performance advantages of our algorithms on two real data sets. To get lower prediction error, three priciples have also been proposed to select the criterion for different environmental fields.
- Multi-Robot Adaptive Exploration and Mapping for Environmental Sensing Applications.
Kian Hsiang Low.
Ph.D. Thesis, Technical Report CMU-ECE-2009-024, Department of Electrical and Computer Engineering, Carnegie Mellon University, Pittsburgh, PA, Aug 2009.
Abstract.
Recent research in robot exploration and mapping has focused on sampling hotspot fields, which often arise in environmental and ecological sensing applications. Such a hotspot field is characterized by continuous, positively skewed, spatially correlated measurements with the hotspots exhibiting extreme measurements and much higher spatial variability than the rest of the field.
To map a hotspot field of the above characterization, we assume that it is realized from non-parametric probabilistic models such as the Gaussian and log-Gaussian processes (respectively, GP and lGP), which can provide formal measures of map uncertainty. To learn a hotspot field map, the exploration strategy of a robot team then has to plan resource-constrained observation paths that minimize the uncertainty of a spatial model of the hotspot field. This exploration problem is formalized in a sequential decision-theoretic planning under uncertainty framework called the multi-robot adaptive sampling problem (MASP). So, MASP can be viewed as a sequential, non-myopic version of active learning. In contrast to finite-state Markov decision problems, MASP adopts a more complex but realistic continuous-state, non-Markovian problem structure so that its induced exploration policy can be informed by the complete history of continuous, spatially correlated observations for selecting paths. It is unique in unifying formulations of non-myopic exploration problems along the entire adaptivity spectrum, thus subsuming existing non-adaptive formulations and allowing the performance advantage of a more adaptive policy to be theoretically realized. Through MASP, it is demonstrated that a more adaptive strategy can exploit clustering phenomena in a hotspot field to produce lower expected map uncertainty. By measuring map uncertainty using the mean-squared error criterion, a MASP-based exploration strategy consequently plans adaptive observation paths that minimize the expected posterior map error or equivalently, maximize the expected map error reduction.
The time complexity of solving MASP (approximately) depends on the map resolution, which limits its practical use in large-scale, high-resolution exploration and mapping. This computational difficulty is alleviated through an information-theoretic approach to MASP (iMASP), which measures map uncertainty based on the entropy criterion instead. As a result, an iMASP-based exploration strategy plans adaptive observation paths that minimize the expected posterior map entropy or equivalently, maximize the expected entropy of observation paths. Unlike MASP, reformulating the cost-minimizing iMASP as a reward-maximizing dual problem causes its time complexity of being solved approximately to be independent of the map resolution and less sensitive to larger robot team size as demonstrated both analytically and empirically. Furthermore, this reward-maximizing dual transforms the widely-used non-adaptive maximum entropy sampling problem into a novel adaptive variant, thus improving the performance of the induced exploration policy.
One advantage stemming from the reward-maximizing dual formulations of MASP and iMASP is that they allow observation selection properties of the induced exploration policies to be realized for sampling the hotspot field. These properties include adaptivity, hotspot sampling, and wide-area coverage. We show that existing GP-based exploration strategies may not explore and map the hotspot field well with the selected observations because they are non-adaptive and perform only wide-area coverage. In contrast, the lGP-based exploration policies can learn a high-quality hotspot field map because they are adaptive and perform both wide-area coverage and hotspot sampling.
The other advantage is that even though MASP and iMASP are non-trivial to solve due to their continuous state components, the convexity of their reward-maximizing duals can be exploited to derive, in a computationally tractable manner, discrete-state monotone-bounding approximations and subsequently, approximately optimal exploration policies with theoretical performance guarantees. Anytime algorithms based on approximate MASP and iMASP are then proposed to alleviate the computational difficulty that arises from their non-Markovian structure.
It is of practical interest to be able to quantitatively characterize the "hotspotness" of an environmental field. We propose a novel "hotspotness" index, which is defined in terms of the spatial correlation properties of the hotspot field. As a result, this index can be related to the intensity, size, and diffuseness of the hotspots in the field.
We also investigate how the spatial correlation properties of the hotspot field affect the performance advantage of adaptivity. In particular, we derive sufficient and necessary conditions of the spatial correlation properties for adaptive exploration to yield no performance advantage.
Lastly, we develop computationally efficient approximately optimal exploration strategies for sampling the GP by assuming the Markov property in iMASP planning. We provide theoretical guarantees on the performance of the Markov-based policies, which improve with decreasing spatial correlation. We evaluate empirically the effects of varying spatial correlations on the mapping performance of the Markov-based policies as well as whether these Markov-based path planners are time-efficient for the transect sampling task.
Through the abovementioned work, this thesis establishes the following two claims: (1) adaptive, non-myopic exploration strategies can exploit clustering phenomena to plan observation paths that produce lower map uncertainty than non-adaptive, greedy methods; and (2) Markov-based exploration strategies can exploit small spatial correlation to plan observation paths which achieve map uncertainty comparable to that of non-Markovian policies using significantly less planning time.
- Adaptive Sampling for Multi-Robot Wide Area Prospecting.
Kian Hsiang Low, Geoffrey J. Gordon, John M. Dolan, and Pradeep Khosla.
In Technical Report CMU-RI-TR-05-51, Robotics Institute, Carnegie Mellon University, Pittsburgh, PA, Oct 2005.
Abstract. Prospecting for in situ mineral resources is essential for establishing settlements on the Moon and Mars. To reduce human effort and risk, it is desirable to build robotic systems to perform this prospecting. An important issue in designing such systems is the sampling strategy: how do the robots choose where to prospect next? This paper argues that a strategy called Adaptive Cluster Sampling (ACS) has a number of desirable properties: compared to conventional strategies, (1) it reduces the total mission time and energy consumption of a team of robots, and (2) returns a higher mineral yield and more information about the prospected region by directing exploration towards areas of high mineral density, thus providing detailed maps of the boundaries of such areas. Due to the adaptive nature of the sampling scheme, it is not immediately obvious how the resulting sampled data can be used to provide an unbiased, low-variance estimate of the regional mineral density. This paper therefore investigates new mineral density estimators, which have lower error than previously-developed estimators; they are derived from the older estimators via a process called Rao-Blackwellization. Since the efficiency of estimators depends on the type of mineralogical population sampled, the population characteristics that favor ACS estimators are also analyzed. The ACS scheme and our new estimators are evaluated empirically in a detailed simulation of the prospecting task, and the quantitative results show that our approach can yield more minerals with less resources and provide more accurate mineral density estimates than previous methods.
- Integrated Robot Planning and Control with Extended Kohonen Maps.
Kian Hsiang Low.
M.Sc. Thesis, Department of Computer Science, School of Computing, National University of Singapore, Jul 2002.
Singapore Computer Society Prize for best M.Sc. Thesis 2002-2003
Abstract. The problem of goal-directed, collision-free motion in a complex, unpredictable environment can be solved by tightly integrating high-level deliberative planning with low-level reactive control. This thesis presents two such architectures for a nonholonomic mobile robot. To achieve real-time performance, reactive control capabilities have to be fully realized so that the deliberative planner can be simplified. These architectures are enriched with reactive target reaching and obstacle avoidance modules. Their target reaching modules use indirect-mapping Extended Kohonen Map to provide finer and smoother motion control than direct-mapping methods. While one architecture fuses these modules indirectly via command fusion, the other one couples them directly using cooperative Extended Kohonen Maps, enabling the robot to negotiate unforeseen concave obstacles. The planner for both architectures use a slippery cells technique to decompose the free workspace into fewer cells, thus reducing search time. Any two points in the cell can still be traversed by reactive motion.
- Mobile Robots That Learn to Navigate.
Kian Hsiang Low.
Honors Thesis, Department of Computer Science,
School of Computing, National University of Singapore, Apr 2001.
Abstract. A sensorimotor controller has been implemented to enable a mobile robot to learn its motion control autonomously and perform simple target-reaching movements. This controller is able to perform fine motion by reducing its self-positioning error and also, reach a designated target location with minimum delay. The control architecture is in the form of a neural network known as the Self-Organizing Map. Besides implementing the motor control and the online learning algorithms, the essentiality of a pre-learning phase is also evaluated. Then, we explore the possibility of incorporating a novel concept known as Local Linear Smoothing into our batch training algorithm; this notion allows the elimination of the boundary bias phenomenon. Lastly, we suggest a simple approach to learning in an obstacle-ridden environment.
This document, research.html, has been accessed 53865 times since 26-Jun-21 15:35:21 SGT.
This is the 28th time it has been accessed today.
A total of 17160 different hosts have accessed this document in the
last 1037 days; your host, ec2-3-144-84-155.us-east-2.compute.amazonaws.com, has accessed it 1 times.
If you're interested, complete statistics for
this document are also available, including breakdowns by top-level
domain, host name, and date.
|
TRUSTED MODEL/DATA SHARING AND DATA VALUATION
PROJECT DURATION : Oct 2018 - Present
PROJECT AFFILIATION
-
Trusted Collaborative Machine Learning (Trusted CollabML) Lab, NUS Institute of Data Science (IDS) (Collaborator: See-Kiong Ng)
-
NUS Centre for Research in Privacy Technologies (N-CRiPT) Centre (Collaborator: Mohan Kankanhalli)
MEDIA NEWS :
-
N-CRiPT Public Seminar (29 Dec 2020) - Trusted Data Sharing: Incentivizing Collaboration and Rights to be Forgotten (aka Unlearning) in Machine Learning
-
N-CRiPT Blog (3 Jan 2020) - 'Sharing, without oversharing, in collective machine learning'
PROJECT FUNDING
- AI Singapore Research Programme : Toward Trustable Model-centric Sharing for Collaborative Machine Learning, S$8,401,002.40, Apr 2021 - Mar 2025
PROBLEM MOTIVATION
- Incentivizing Collaboration for Trusted Data Sharing. Collaborative machine learning (ML) is an appealing paradigm to build high-quality ML models. While an individual party may have limited data, it is possible to build improved, high-quality ML models by training on the aggregated data from many parties. For example, in healthcare, a hospital or healthcare firm whose data diversity and quantity are limited due to its small patient base can draw on data from other hospitals and firms to improve the prediction of some disease progression (e.g., diabetes). This collaboration can be encouraged by a government agency, such as the National Institute of Health in the United States.
In precision agriculture, a farmer with limited land area and sensors can combine his collected data with the other farmers to improve the modeling of the effect of various influences (e.g., weather, pest) on his crop yield. Such data sharing also benefits other application domains, including real estate in which a property agency can pool together its limited transactional data with that of the other agencies to improve the prediction of property prices.
However, any party would have incurred some nontrivial cost to collect its data. So, they would not altruistically donate their data and risk losing their competitive edge.
These parties will be motivated to share their data when given enough incentives,
such as a guaranteed benefit from the collaboration and a fair higher reward from contributing more valuable data.
- Collective Learning/Fusion of Heterogeneous, Black-Box ML Models. Practical scenarios that involve learning in complex environments often require the collaboration of multiple experts operating concurrently on different sub-domains. Motivated under this context, collective learning is an emerging study of a distributed paradigm where each local expert learns independently from its data and exchange knowledge with others to achieve better performance.
Existing collective learning literature, however, usually assumes perfect clarity of local expert models, which entails fully transparent model architectures and publicly accessible local data used to train these experts. To facilitate model fusion, local experts are further expected to have employed a homogeneous design with limited freedom in their choices of parameters. Despite enabling collective learning, these restrictive assumptions have imposed a rigidity on the algorithmic level that is generally undesirable for practical purposes. For example, applications learning from private medical records are often prohibited from publicizing sensitive patient information; model architectures in confidential domains such as financial forecasting are preferably kept undisclosed to guard against adversarial attacks. As such, it is unrealistic for a collaboration scheme among these experts to presume prior understanding of their behaviors, much less subjecting them to conceptual homogeneity.
Another central issue of collective learning is the computational and communication bottleneck arising from having one single or a few central servers to coordinate the collective agents. In practice, such a centralized collective architecture also often leads to having undesirable choke points of failure in the system.
- Multi-Task Model Fusion of Black-Box Experts.
In various disciplines such as environmental sensing, traffic monitoring, and healthcare analytics, observational data that describe the same phenomenon or concept are often acquired from multiple experiments, which are conducted on different subjects. The data that they generated would therefore have different distributions or statistical properties. In practice, due to privacy concerns, data collected from different acquisition frameworks (e.g., sensors and/or experiments) are also private and cannot be shared among themselves. This creates private datasets of the same phenomenon, where each is used to train a separate model from a local perspective. For example, in clinical research, patient information is often recorded across different institutions, which do not share data with each other due to protect the patient's sensitive information. As such, each institution can only model the patient population using data collected from a single demographic region, which might not generalize well to others.
Furthermore, in settings with strict security requirements, parameters of a local model also need to be kept private due to a recently discovered threat of adversarial ML attack, which essentially makes it a black box to others. This violates the model transparency requirement of existing distributed modeling works addressing this data federation issue, which results in the black-box challenge.
In addition, the existing works in federated learning assumes that local models were trained to solve the same task (albeit with different data). Their proposed methods in fact do not isolate task-specific (irrelevant knowledge) from task-agnostic information (relevant knowledge) which are implicitly entangled in the parameter representation of each model. This entanglement presents a problem in multi-task setting since it does not tell us which part of an existing model is relevant to a new task and which part is not. This is also an issue in a remotely relevant literature of meta learning which tackles the model adaptation problem in multi-task scenarios from a different technical setting that does not accommodate black-box and pre-trained models with private training data.
PROPOSED METHODOLOGY
- Incentivizing Collaboration for Trusted Data Sharing. We propose to value each party's contributed data and design an incentive-aware reward scheme to give each party a separate ML model as a reward (in short, model reward) accordingly. We use only model rewards and exclude monetary compensations as (a) in the above-mentioned applications, every party such as a hospital is mainly interested in improving model quality for unlimited future test predictions; (b) there may not be a feasible and clear source of monetary revenue to compensate participants (e.g., due to restrictions, the government may not be able to pay firms using tax revenues); and (c) if parties have to pay to participate in the collaboration, then the total payment is debatable and many may lack funding while still having valuable data.
- How then should we value a party's data and its effect on model quality?
To answer this first question, we propose a valuation method based on the informativeness of data. In particular, more informative data induces a greater reduction in the uncertainty of the model parameters, hence improving model quality.
In contrast, existing data valuation methods measure model quality via its validation accuracy, which calls for a tedious or even impossible process of needing all parties to agree on a common validation dataset. Inaccurate valuation can also arise due to how a party's test queries, which are likely to change over time, differ from the validation dataset. Our data valuation method does not make any assumption about the current or future distribution of test queries.
- Next, how should we design a reward scheme to decide the values of model rewards for incentivizing a collaboration?
Intuitively, a party will be motivated to collaborate if it can receive a better ML model than others who have contributed less valuable data, and than what it can build alone or get from collaborating separately with some parties.
Also, the parties often like to maximize the total benefit from the collaboration.
These incentives appear related to solution concepts (fairness, individual rationality, stability, and group welfare) from cooperative game theory (CGT), respectively. However, as CGT assumptions are restrictive for the uniquely freely replicable nature of our model reward, these CGT concepts have to be adapted for defining incentives for our model reward. We then design a novel model reward scheme to provide these incentives.
- Finally, how can we realize the values of the model rewards decided by our scheme?
How should we modify the model rewards or the data used to train them?
An obvious approach to control the values of the model rewards is to select and only train on subsets of the aggregated data. However, this requires considering an exponential number of discrete subsets of data, which is intractable for large datasets such as medical records. We avoid this issue by injecting noise into the aggregated data from multiple parties instead. The value of each party's model reward can then be realized by simply optimizing the continuous noise variance parameter.
Current efforts on designing incentive mechanisms for federated learning can build upon our work presented here. For future work, we plan to address privacy preservation in our reward scheme. The noise injection method used for realizing the model rewards in this work is closely related to the Gaussian mechanism of differential privacy. This motivates us to explore how the injected noise will affect privacy in the model rewards.
- Collective Learning/Fusion of Heterogeneous, Black-Box ML Models. We propose a novel collective learning platform for black-box fusion that addresses the following challenges: (a) performing fusion without access to the black-box training data and architectures, (b) performing fusion when the black-box models are not permanently available, and (c) avoiding centralized bottlenecks and risk of failure for large-scale fusion with numerous black-box experts.
To achieve this, we first develop a collective fusion paradigm that allows black-box experts to interact and learn the predictive behaviors of one another, which are then succinctly encoded into information summaries with constant memory footprint for efficient communication and assimilation. A decentralized communication algorithm is further developed to regulate the propagation flow of these local summaries to optimize the expected improvement rate of the experts while guaranteeing that they reach a consensus upon convergence.
- Multi-Task Model Fusion of Black-Box Experts. We propose a fusion paradigm that represents each black-box expert as a task-dependent distribution over an infinite spectrum of task-agnostic predictive prototypes. The prototypes intuitively represent the task-agnostic information, which can be transferred among tasks to corroborate their statistical strength, whereas their distribution encodes domain-specific information that needs to be adapted to suit a new task.
PUBLICATIONS
- Collaborative Bayesian Optimization with Fair Regret.
Rachael Hwee Ling Sim, Yehong Zhang, Kian Hsiang Low & Patrick Jaillet.
In Proceedings of the 38th International Conference on Machine Learning (ICML-21), pages 9691-9701, Jul 18-24, 2021.
21.5% acceptance rate
Abstract. Bayesian optimization (BO) is a popular tool for optimizing complex and costly-to-evaluate black-box objective functions. To further reduce the number of function evaluations, any party performing BO may be interested to collaborate with others to optimize the same objective function concurrently. To do this, existing BO algorithms have considered optimizing a batch of input queries in parallel and provided theoretical bounds on their cumulative regret reflecting inefficiency. However, when the objective function values are correlated with real-world rewards (e.g., money), parties may be hesitant to collaborate if they risk incurring larger cumulative regret (i.e., smaller real-world reward) than others. This paper shows that fairness and efficiency are both necessary for the collaborative BO setting. Inspired by social welfare concepts from economics, we propose a new notion of regret capturing these properties and a collaborative BO algorithm whose convergence rate can be theoretically guaranteed by bounding the new regret, both of which share an adjustable parameter for trading off between fairness vs. efficiency. We empirically demonstrate the benefits (e.g., increased fairness) of our algorithm using synthetic and real-world datasets.
- Model Fusion for Personalized Learning.
Chi Thanh Lam, Trong Nghia Hoang, Kian Hsiang Low & Patrick Jaillet.
In Proceedings of the 38th International Conference on Machine Learning (ICML-21), pages 5948-5958, Jul 18-24, 2021.
21.5% acceptance rate
Abstract. Production systems operating on a growing domain of analytic services often require generating warm-start solution models for emerging tasks with limited data. One potential approach to address this challenge is to adopt meta learning to generate a base model that can be adapted to solve unseen tasks with minimal fine-tuning. This however requires the training processes of previous solution models of existing tasks to be synchronized. This is not possible if these models were pre-trained separately on private data owned by different entities and cannot be synchronously re-trained. To accommodate for such scenarios, we develop a new personalized learning framework that synthesizes customized models for unseen tasks via fusion of independently pre-trained models of related tasks. We establish performance guarantee for the proposed framework and demonstrate its effectiveness on both synthetic and real datasets.
- AID: Active Distillation Machine to Leverage Pre-Trained Black-Box Models in Private Data Settings.
Trong Nghia Hoang, Shenda Hong, Cao Xiao, Kian Hsiang Low & Jimeng Sun.
In Proceedings of the 30th The Web Conference (WWW'21), pages 3569–3581, Apr 19-23, 2021.
20.6% acceptance rate
Abstract. This paper presents an active distillation method for a local institution (e.g., hospital) to find the best queries within its given budget to distill an on-server black-box model's predictive knowledge into a local surrogate with transparent parameterization. This allows local institutions to understand better the predictive reasoning of the black-box model in its own local context or to further customize the distilled knowledge with its private dataset that cannot be centralized and fed into the server model. The proposed method thus addresses several challenges of deploying machine learning in many industrial settings (e.g., healthcare analytics) with strong proprietary constraints. These include: (1) the opaqueness of the server model’s architecture which prevents local users from understanding its predictive reasoning in their local data contexts; (2) the increasing cost and risk of uploading local data on the cloud for analysis; and (3) the need to customize the server model with private onsite data. We evaluated the proposed method on both benchmark and real-world healthcare data where significant improvements over existing local distillation methods were observed. A theoretical analysis of the proposed method is also presented.
- Federated Bayesian Optimization via Thompson Sampling.
Zhongxiang Dai, Kian Hsiang Low & Patrick Jaillet.
In Advances in Neural Information Processing Systems 33: 34th Annual Conference on Neural Information Processing Systems (NeurIPS'20), pages 9687-9699, Dec 6-12, 2020.
20.1% acceptance rate
Abstract. Bayesian optimization (BO) is a prominent method for optimizing expensive-to-compute black-box functions. The massive computational capability of edge devices such as mobile phones, coupled with privacy concerns, has led to immense recent interest in federated learning (FL), which focuses on collaborative training of deep neural networks (DNN) via first-order optimization techniques. However, some common machine learning tasks such as hyperparameter tuning of DNN lack access to gradients and thus require zeroth-order optimization (black-box optimization). This hints at the considerable potential of extending BO to the FL setting (FBO), to allow agents to collaborate in these black-box optimization tasks. Here, we introduce federated Thompson sampling (FTS), which overcomes a number of key challenges of FBO and FL in a principled way: We (a) use random Fourier features to approximate the Gaussian process surrogate model used in BO which naturally produces the parameters to be exchanged between agents, (b) design FTS based on Thompson sampling which significantly reduces the number of parameters to be exchanged, and (c) provide a theoretical convergence guarantee that is robust against heterogeneous agents which is a major challenge in FL and FBO. We empirically demonstrate the effectiveness of FTS in terms of communication efficiency, computational efficiency and practical performance.
- Variational Bayesian Unlearning.
Quoc Phong Nguyen, Kian Hsiang Low & Patrick Jaillet.
In Advances in Neural Information Processing Systems 33: 34th Annual Conference on Neural Information Processing Systems (NeurIPS'20), pages 16025-16036, Dec 6-12, 2020.
20.1% acceptance rate
Abstract. This paper studies the problem of approximately unlearning a Bayesian model from a small subset of the training data to be erased. We frame this problem as one of minimizing the Kullback-Leibler distance between the approximate posterior belief of model parameters after directly unlearning from the erased data vs. the exact posterior belief from retraining with remaining data. Using the variational inference (VI) framework, we show that it is equivalent to minimizing an evidence upper bound which trades off between fully unlearning from erased data vs. not entirely forgetting the posterior belief given the full data (i.e., including the remaining data); the latter prevents catastrophic unlearning that can render the model useless. In model training with VI, only an approximate (instead of exact) posterior belief given the full data can be obtained, which makes unlearning even more challenging. We propose two novel tricks to tackle this challenge. We empirically demonstrate our unlearning methods on Bayesian models such as sparse Gaussian process and logistic regression using synthetic and real-world datasets.
- Collaborative Machine Learning with Incentive-Aware Model Rewards.
Rachael Hwee Ling Sim, Yehong Zhang, Mun Choon Chan & Kian Hsiang Low.
In Proceedings of the 37th International Conference on Machine Learning (ICML-20), pages 8927-8936, Jun 12-18, 2020.
21.8% acceptance rate
Abstract. Collaborative machine learning (ML) is an appealing paradigm to build high-quality ML models by training on the aggregated data from many parties. However, these parties are only willing to share their data when given enough incentives, such as a guaranteed fair reward based on their contributions. This motivates the need for measuring a party's contribution and designing an incentive-aware reward scheme accordingly. This paper proposes to value a party's reward based on Shapley value and information gain on model parameters given its data. Subsequently, we give each party a model as a reward. To formally incentivize the collaboration, we define some desirable properties (e.g., fairness and stability) which are inspired by cooperative game theory but adapted for our model reward that is uniquely freely replicable. Then, we propose a novel model reward scheme to satisfy fairness and trade off between the desirable properties via an adjustable parameter. The value of each party's model reward determined by our scheme is attained by injecting Gaussian noise to the aggregated training data with an optimized noise variance. We empirically demonstrate interesting properties of our scheme and evaluate its performance using synthetic and real-world datasets.
- Learning Task-Agnostic Embedding of Multiple Black-Box Experts for Multi-Task Model Fusion.
Trong Nghia Hoang, Chi Thanh Lam, Kian Hsiang Low & Patrick Jaillet.
In Proceedings of the 37th International Conference on Machine Learning (ICML-20), pages 4282-4292, Jun 12-18, 2020.
21.8% acceptance rate
Abstract. Model fusion is an emerging study in collective learning where heterogeneous experts with private data and learning architectures need to combine their black-box knowledge for better performance. Existing literature achieves this via a local knowledge distillation scheme that transfuses the predictive patterns of each pre-trained expert onto a white-box imitator model, which can be incorporated efficiently into a global model. This scheme however does not extend to multi-task scenarios where different experts were trained to solve different tasks and only part of their distilled knowledge is relevant to a new task. To address this multi-task challenge, we develop a new fusion paradigm that represents each expert as a distribution over a spectrum of predictive prototypes, which are isolated from task-specific information encoded within the prototype distribution. The task-agnostic prototypes can then be reintegrated to generate a new model that solves a new task encoded with a different prototype distribution. The fusion and adaptation performance of the proposed framework is demonstrated empirically on several real-world benchmark datasets.
- Collective Model Fusion for Multiple Black-Box Experts.
Quang Minh Hoang, Trong Nghia Hoang, Kian Hsiang Low & Carleton Kingsford.
In Proceedings of the 36th International Conference on Machine Learning (ICML-19), pages 2742-2750, Long Beach, CA, Jun 9-15, 2019.
22.6% acceptance rate
Abstract. Model fusion is a fundamental problem in collective machine learning (ML) where independent experts with heterogeneous learning architectures are required to combine expertise to improve predictive performance. This is particularly challenging in information-sensitive domains (e.g., medical records in health-care analytics) where experts do not have access to each other's internal architecture and local data. To address this challenge, this paper presents the first collective model fusion framework for multiple experts with heterogeneous black-box architectures. The proposed method will enable this by addressing the following key issues of how black-box experts interact to understand the predictive behaviors of one another; how these understandings can be represented and shared efficiently among themselves; and how the shared understandings can be combined to generate high-quality consensus prediction. The performance of the resulting framework is analyzed theoretically and demonstrated empirically on several datasets.
PRESENTATIONS
- Trusted Data Sharing: Incentivizing Collaboration and Rights to be Forgotten (aka Unlearning) in Machine Learning.
Kian Hsiang Low.
Invited keynote speaker at the 20th IEEE/WIC/ACM International Conference on Web Intelligence and Intelligent Agent Technology, Melbourne, Australia, Dec 14-17, 2021.
- Collective Online Learning and Model Fusion in Large Multiagent Systems.
Kian Hsiang Low.
Invited keynote speaker at the 2nd International Symposium on Multi-Robot and Multi-Agent Systems, Rutgers University, New Brunswick, NJ, USA, Aug 22-23, 2019.
AUTOMATED MACHINE LEARNING : BAYESIAN OPTIMIZATION
PROJECT DURATION : Feb 2016 - Present
PROJECT AFFILIATION
-
Temasek Life Sciences Laboratory (TLL) (Collaborator: Nam Hai Chua)
-
Singapore-MIT Alliance for Research and Technology (SMART) Future Urban Mobility (FM) IRG (Collaborator: Patrick Jaillet, MIT)
-
Sensor-Enhanced Social Media (SeSaMe) Centre (Collaborator: Mohan Kankanhalli)
MEDIA NEWS
-
AI Singapore Spotlight SG AI Researchers (1 Sep 2020) - 'Discovering the Science behind Hyperparameter Tuning'
-
8th QuantumBlack Singapore Meetup (8 July 2020) - 'Probabilistic Machine Learning'
-
NUS School of Computing Faculty Feature (28 May 2019) - 'What Bayesian Optimization can teach us about baking better cookies and more'
PROJECT FUNDING
- RIE2020 AME Programmatic Fund : Learning with Less Data, SGD $1,218,600, Apr 2021 - Mar 2024
- DSTA Project Agreement : Tactics Discovery and Recommendation, SGD $1,143,120, Jul 2021 - Aug 2023
- MOE AcRF Tier 1 Reimagine Research Scheme Funding : Scalable AI Phenome Platform towards Fast-Forward Plant Breeding (Machine Learning),
SGD $348,600, Mar 2021 - Mar 2024
- RIE2020 AME IAF-PP : High Performance Precision Agriculture (HiPPA) System, S$1,197,960, Mar 2020 - Feb 2024
- MOE AcRF Tier 2 Grant : Scaling up Gaussian Process Predictive Models for Big Data, SGD $737,461, Jul 2017 - Jul 2020
- SMART Subaward Agreement - FM IRG :
Automatic Probabilistic Machine Learning for Traffic Modeling and Prediction,
SGD $184,999.20, Apr 2017 - May 2021
- Research Collaboration Agreement with Panasonic R&D Center Singapore : Hyperparameters Tuning using Bayesian Optimization, SGD $69,336, Mar 2016 - Mar 2017
PROBLEM MOTIVATION
- Batch Bayesian Optimization. Bayesian optimization (BO) has recently gained considerable traction due to its capability of finding the global maximum of a highly complex (e.g., non-convex, no closed-form expression nor derivative), noisy black-box objective function with a limited budget of (often costly) function evaluations, consequently witnessing its use in an increasing diversity of application domains such as robotics, environmental sensing/monitoring, automated machine learning, among others.
A number of acquisition functions (e.g., probability of improvement or expected improvement over the currently found maximum, entropy-based, and upper confidence bound (UCB)) have been devised to perform BO: They repeatedly select an input for evaluating/querying the black-box function (i.e., until the budget is depleted) that intuitively trades off between sampling where the maximum is likely to be given the current, possibly imprecise belief of the function modeled by a Gaussian process (GP) (i.e., exploitation) vs. improving the GP belief of the function over the entire input domain (i.e., exploration) to guarantee finding the global maximum.
The rapidly growing affordability and availability of hardware resources (e.g., computer clusters, sensor networks, robot teams/swarms) have motivated the recent development of BO algorithms that can repeatedly select a batch of inputs for querying the black-box function in parallel instead. Such batch/parallel BO algorithms can be classified into two types: On one extreme, batch BO algorithms like multi-points expected improvement, parallel predictive entropy search, and the parallel knowledge gradient method jointly optimize the batch of inputs and hence scale poorly in the batch size.
On the other extreme, greedy batch BO algorithms boost the scalability by selecting the inputs of the batch one at a time. We argue that such a highly suboptimal approach to gain scalability is an overkill: In practice, each function evaluation is often much more computationally and/or economically costly (e.g., hyperparameter tuning for deep learning, drug testing on human subjects), which justifies dedicating more time to obtain better BO performance.
- Nonmyopic Bayesian Optimization. The fundamental challenge of integrated planning and learning is to design an autonomous agent that can plan its actions to maximize its expected total rewards while interacting with an unknown task environment. Recent research efforts tackling this challenge have progressed from the use of simple Markov models assuming discrete-valued, independent observations to that of a rich class of Bayesian nonparametric Gaussian process (GP) models characterizing continuous-valued, correlated observations in order to represent the latent structure of complex, possibly noisy task environments with higher fidelity. Such a challenge is posed by the problem of Bayesian optimization (BO).
Its objective is to select and gather the most informative (possibly noisy) observations for finding the global maximum of an unknown, highly complex (e.g., non-convex, no closed-form expression nor derivative) objective function (i.e., task environment) modeled by a GP given a sampling budget (e.g., number of costly function evaluations). The rewards of a BO agent are defined using an improvement-based (e.g., probability of improvement or expected improvement over currently found maximum), entropy-based, or upper confidence bound (UCB) acquisition function. A limitation of most BO algorithms is that they are myopic. To overcome this limitation, approximation algorithms for nonmyopic adaptive BO have been proposed, but their performances are not theoretically guaranteed.
PROPOSED METHODOLOGY
- Batch Bayesian Optimization. To tackle the first problem, we show that it is in fact possible to jointly optimize the batch of inputs and still preserve scalability in the batch size by giving practitioners the flexibility to trade off BO performance for time efficiency.
To achieve this, we first observe that, interestingly, batch BO can be perceived as a cooperative multi-agent decision making problem whereby each agent optimizes a separate input of the batch while coordinating with the other agents doing likewise.
To the best of our knowledge, this has not been considered in the BO literature.
In particular, if batch BO can be framed as some known class of multi-agent decision making problems, then it can be solved efficiently and scalably by the latter's state-of-the-art solvers.
The key technical challenge would therefore be to investigate how batch BO can be cast as one of such to exploit its advantage of scalability in the number of agents (hence, batch size) while at the same time theoretically guaranteeing the resulting BO performance.
To tackle the above challenge, our first work presents a novel distributed batch BO algorithm that, in contrast to greedy batch BO algorithms,
can jointly optimize a batch of inputs and, unlike the batch BO algorithms, still preserve scalability in the batch size.
To realize this, we generalize GP-UCB to a new batch variant amenable to a Markov approximation, which can then be naturally formulated as a multi-agent distributed constraint optimization problem (DCOP) in order to fully exploit the efficiency of its state-of-the-art solvers for achieving linear time in the batch size.
Our proposed distributed batch GP-UCB (DB-GP-UCB) algorithm offers practitioners the flexibility to trade off between the approximation quality and time efficiency by varying the Markov order. We provide a theoretical guarantee for the convergence rate of our DB-GP-UCB algorithm via bounds on its cumulative regret. We empirically evaluate the cumulative regret incurred by our DB-GP-UCB algorithm and its scalability in the batch size on synthetic benchmark objective functions and a real-world optimization problem.
- Nonmyopic Bayesian Optimization. To address the second problem, our second work presents a novel nonmyopic adaptive Gaussian process planning (GPP) framework endowed with a general class of Lipschitz continuous reward functions that can unify some active learning and BO criteria (e.g., UCB) and offer practitioners some flexibility to specify their desired choices for defining new tasks/problems. In particular, it utilizes a principled Bayesian sequential decision problem framework for jointly and naturally optimizing the exploration-exploitation trade-off, consequently allowing planning and learning to be integrated seamlessly and performed simultaneously instead of separately. In general, the resulting induced GPP policy cannot be derived exactly due to an uncountable set of candidate observations. A key contribution of our work here thus lies in exploiting the Lipschitz continuity of the reward functions to solve for a nonmyopic adaptive ε-optimal GPP (ε-GPP) policy given an arbitrarily user-specified loss bound ε. To plan in real time, we further propose an asymptotically optimal, branch-and-bound anytime variant of ε-GPP with performance guarantee. Finally, we empirically evaluate the performances of our ε-GPP policy and its anytime variant in BO and an energy harvesting task on simulated and real-world environmental fields.
PUBLICATIONS
- Trusted-Maximizers Entropy Search for Efficient Bayesian Optimization.
Quoc Phong Nguyen, Zhaoxuan Wu, Kian Hsiang Low & Patrick Jaillet.
In Proceedings of the 37th Conference on Uncertainty in Artificial Intelligence (UAI-21), pages 1486-1495, Jul 27-30, 2021.
26.5% acceptance rate
Abstract. Information-based Bayesian optimization (BO) algorithms have achieved state-of-the-art performance in optimizing a black-box objective function. However, they usually require several approximations or simplifying assumptions (without clearly understanding their effects on the BO performance) and/or their generalization to batch BO is computationally unwieldy, especially with an increasing batch size. To alleviate these issues, this paper presents a novel trusted-maximizers entropy search (TES) acquisition function: It measures how much an input query contributes to the information gain on the maximizer over a finite set of trusted maximizers, i.e., inputs optimizing functions that are sampled from the Gaussian process posterior belief of the objective function. Evaluating TES requires either only a stochastic approximation with sampling or a deterministic approximation with expectation propagation, both of which are investigated and empirically evaluated using synthetic benchmark objective functions and real-world optimization problems, e.g., hyperparameter tuning of a convolutional neural network and synthesizing ‘physically realizable’ faces to fool a black-box face recognition system. Though TES can naturally be generalized to a batch variant with either approximation, the latter is amenable to be scaled to a much larger batch size in our experiments.
- Value-at-Risk Optimization with Gaussian Processes.
Quoc Phong Nguyen, Zhongxiang Dai, Kian Hsiang Low & Patrick Jaillet.
In Proceedings of the 38th International Conference on Machine Learning (ICML-21), pages 8063-8072, Jul 18-24, 2021.
21.5% acceptance rate
Abstract. Value-at-risk (VaR) is an established measure to assess risks in critical real-world applications with random environmental factors. This paper presents a novel VaR upper confidence bound (V-UCB) algorithm for maximizing the VaR of a black-box objective function with the first no-regret guarantee. To realize this, we first derive a confidence bound of VaR and then prove the existence of values of the environmental random variable (to be selected to achieve no regret) such that the confidence bound of VaR lies within that of the objective function evaluated at such values. Our V-UCB algorithm empirically demonstrates state-of-the-art performance in optimizing synthetic benchmark functions, a portfolio optimization problem, and a simulated robot task.
- Collaborative Bayesian Optimization with Fair Regret.
Rachael Hwee Ling Sim, Yehong Zhang, Kian Hsiang Low & Patrick Jaillet.
In Proceedings of the 38th International Conference on Machine Learning (ICML-21), pages 9691-9701, Jul 18-24, 2021.
21.5% acceptance rate
Abstract. Bayesian optimization (BO) is a popular tool for optimizing complex and costly-to-evaluate black-box objective functions. To further reduce the number of function evaluations, any party performing BO may be interested to collaborate with others to optimize the same objective function concurrently. To do this, existing BO algorithms have considered optimizing a batch of input queries in parallel and provided theoretical bounds on their cumulative regret reflecting inefficiency. However, when the objective function values are correlated with real-world rewards (e.g., money), parties may be hesitant to collaborate if they risk incurring larger cumulative regret (i.e., smaller real-world reward) than others. This paper shows that fairness and efficiency are both necessary for the collaborative BO setting. Inspired by social welfare concepts from economics, we propose a new notion of regret capturing these properties and a collaborative BO algorithm whose convergence rate can be theoretically guaranteed by bounding the new regret, both of which share an adjustable parameter for trading off between fairness vs. efficiency. We empirically demonstrate the benefits (e.g., increased fairness) of our algorithm using synthetic and real-world datasets.
- Top-k Ranking Bayesian Optimization.
Quoc Phong Nguyen, Sebastian Tay, Kian Hsiang Low & Patrick Jaillet.
In Proceedings of the 35th AAAI Conference on Artificial Intelligence (AAAI-21), pages 9135-9143, Feb 2-9, 2021.
21.4% acceptance rate
Abstract. This paper presents a novel approach to top-k ranking Bayesian optimization (top-k ranking BO) which is a practical and significant generalization of preferential BO to handle top-k ranking and tie/indifference observations. We first design a surrogate model that is not only capable of catering to the above observations, but is also supported by a classic random utility model. Another equally important contribution is the introduction of the first information-theoretic acquisition function in BO with preferential observation called multinomial predictive entropy search (MPES) which is flexible in handling these observations and optimized for all inputs of a query jointly. MPES possesses superior performance compared with existing acquisition functions that select the inputs of a query one at a time greedily. We empirically evaluate the performance of MPES using several synthetic benchmark functions, CIFAR-10 dataset, and SUSHI preference dataset.
- An Information-Theoretic Framework for Unifying Active Learning Problems.
Quoc Phong Nguyen, Kian Hsiang Low & Patrick Jaillet.
In Proceedings of the 35th AAAI Conference on Artificial Intelligence (AAAI-21), pages 9126-9134, Feb 2-9, 2021.
21.4% acceptance rate
Abstract. This paper presents an information-theoretic framework for unifying active learning problems: level set estimation (LSE), Bayesian optimization (BO), and their generalized variant. We first introduce a novel active learning criterion that subsumes an existing LSE algorithm and achieves state-of-the-art performance in LSE problems with a continuous input domain. Then, by exploiting the relationship between LSE and BO, we design a competitive information-theoretic acquisition function for BO that has interesting connections to upper confidence bound and max-value entropy search (MES). The latter connection reveals a drawback of MES which has important implications on not only MES but also on other MES-based acquisition functions. Finally, our unifying information-theoretic framework can be applied to solve a generalized problem of LSE and BO involving multiple level sets in a data-efficient manner. We empirically evaluate the performance of our proposed algorithms using synthetic benchmark functions, a real-world dataset, and in hyperparameter tuning of machine learning models.
- Efficient Exploration of Reward Functions in Inverse Reinforcement Learning via Bayesian Optimization.
Sreejith Balakrishnan, Quoc Phong Nguyen, Kian Hsiang Low & Harold Soh.
In Advances in Neural Information Processing Systems 33: 34th Annual Conference on Neural Information Processing Systems (NeurIPS'20), pages 4187-4198, Dec 6-12, 2020.
20.1% acceptance rate
Abstract. In this paper, we focus on the problem of Inverse Reinforcement Learning (IRL), which is relevant for a variety of tasks including value alignment and robot learning from demonstration. Despite significant algorithmic contributions in recent years, IRL remains an ill-posed problem at its core; multiple reward functions coincide with the observed behavior, and the actual reward function is not identifiable without prior knowledge or supplementary information. Here, we propose Bayesian Optimization-IRL (BO-IRL), an IRL framework that identifies multiple solutions that are consistent with the expert demonstrations by efficiently exploring the reward function space. BO-IRL achieves this by utilizing Bayesian Optimization along with our newly proposed kernel that (a) projects the parameters of policy invariant reward functions to a single point in a latent space, and (b) ensures that nearby points in the latent space correspond to reward functions that yield similar likelihoods. This projection allows for the use of standard stationary kernels in the latent space to capture the correlations present across the reward function space. Empirical results on synthetic and real-world environments (model-free and model-based) show that BO-IRL discovers multiple reward functions while minimizing the number of expensive exact policy optimizations.
- Federated Bayesian Optimization via Thompson Sampling.
Zhongxiang Dai, Kian Hsiang Low & Patrick Jaillet.
In Advances in Neural Information Processing Systems 33: 34th Annual Conference on Neural Information Processing Systems (NeurIPS'20), pages 9687-9699, Dec 6-12, 2020.
20.1% acceptance rate
Abstract. Bayesian optimization (BO) is a prominent method for optimizing expensive-to-compute black-box functions. The massive computational capability of edge devices such as mobile phones, coupled with privacy concerns, has led to immense recent interest in federated learning (FL), which focuses on collaborative training of deep neural networks (DNN) via first-order optimization techniques. However, some common machine learning tasks such as hyperparameter tuning of DNN lack access to gradients and thus require zeroth-order optimization (black-box optimization). This hints at the considerable potential of extending BO to the FL setting (FBO), to allow agents to collaborate in these black-box optimization tasks. Here, we introduce federated Thompson sampling (FTS), which overcomes a number of key challenges of FBO and FL in a principled way: We (a) use random Fourier features to approximate the Gaussian process surrogate model used in BO which naturally produces the parameters to be exchanged between agents, (b) design FTS based on Thompson sampling which significantly reduces the number of parameters to be exchanged, and (c) provide a theoretical convergence guarantee that is robust against heterogeneous agents which is a major challenge in FL and FBO. We empirically demonstrate the effectiveness of FTS in terms of communication efficiency, computational efficiency and practical performance.
- Automated Machine Learning: New Advances on Bayesian Optimization.
Dmitrii Kharkovskii.
Ph.D. Thesis, Department of Computer Science, National University of Singapore, Dec 2020.
Abstract. Recent advances in Bayesian optimization (BO) have delivered a promising suite of tools for optimizing an unknown expensive to evaluate black-box objective function with a finite budget of evaluations. A significant advantage of BO is its general formulation: BO can be utilized to optimize any black-box objective function. As a result, BO has been applied in a wide range of applications such as automated machine learning, robotics or environmental monitoring, among others. Furthermore, its general formulation makes BO attractive for deployment in new applications. However, potential new applications can have additional requirements not satisfied by the classical BO setting. In this thesis, we aim to address some of these requirements in order to scale up BO technology for the practical use in new real-world applications.
Firstly, this thesis tackles the problem of data privacy, which is not addressed by the standard setting of BO. Specifically, we consider the outsourced setting where the entity holding the dataset and the entity performing BO are represented by different parties, and the dataset cannot be released non-privately. For example, a hospital holds a dataset of sensitive medical records and outsources the BO task on this dataset to an industrial AI company. We present the private-outsourced-Gaussian process-upper confidence bound (PO-GP-UCB) algorithm, which is the first algorithm for privacy-preserving BO in the outsourced setting with a provable performance guarantee. The key idea of our approach is to make the BO performance of our algorithm similar to that of non-private GP-UCB run using the original dataset, which is achieved by using a random projection-based transformation that preserves both privacy and the pairwise distances between inputs. Our main theoretical contribution is to show that a regret bound similar to that of the standard GP-UCB algorithm can be established for our PO-GP-UCB algorithm. We empirically evaluate the performance of our algorithm with synthetic and real-world datasets.
Secondly, we consider applications of BO for hotspot sampling in spatially varying phenomena. For such applications, we exploit the structure of the spatially varying phenomenon in order to increase the BO lookahead and, as a result, improve the performance of the algorithm and make it more suitable for practical use in real-world scenarios. To do this, we present a principled multi-staged Bayesian sequential decision algorithm for nonmyopic adaptive BO that, in particular, exploits macro-actions for scaling up to a further lookahead to match up to a larger available budget. To achieve this, we first generalize GP-UCB to a new acquisition function defined with respect to a nonmyopic adaptive macro-action policy, which, unfortunately, is intractable to be optimized exactly due to an uncountable set of candidate outputs. The key novel contribution of our work here is to show that it is in fact possible to solve for a nonmyopic adaptive ε-Bayes-optimal macro-action BO (ε-Macro-BO) policy given an arbitrary user-specified loss bound ε via stochastic sampling in each planning stage which requires only a polynomial number of samples in the length of macro-actions. To perform nonmyopic adaptive BO in real time, we then propose an asymptotically optimal anytime variant of our ε-Macro-BO algorithm with a performance guarantee. Empirical evaluation on synthetic and real-world datasets shows that our proposed approach outperforms existing state-of-the-art algorithms.
Finally, this thesis proposes a black-box attack for adversarial machine learning based on BO. Since the dimension of the inputs in adversarial learning is usually too high for applying BO directly, our proposed attack applies dimensionality reduction and searches for an adversarial perturbation in a low-dimensional latent space. The key idea of our approach is to automate both the selection of the latent space dimen- sion and the search of the adversarial perturbation in the selected latent space by using BO. Additionally, we use Bayesian optimal stopping to boost the query efficiency of our attack. Performance evaluation using image classification datasets shows that our proposed method outperforms the state-of-the-art black-box adversarial attacks.
- R2-B2: Recursive Reasoning-Based Bayesian Optimization for No-Regret Learning in Games.
Zhongxiang Dai, Yizhou Chen, Kian Hsiang Low, Patrick Jaillet & Teck-Hua Ho.
In Proceedings of the 37th International Conference on Machine Learning (ICML-20), pages 2291-2301, Jun 12-18, 2020.
21.8% acceptance rate
Abstract. This paper presents a recursive reasoning formalism of Bayesian optimization (BO) to model the reasoning process in the interactions between boundedly rational, self-interested agents with unknown, complex, and costly-to-evaluate payoff functions in repeated games, which we call Recursive Reasoning-Based BO (R2-B2).
Our R2-B2 algorithm is general in that it does not constrain the relationship among the payoff functions of different agents and can thus be applied to various types of games such as constant-sum, general-sum, and common-payoff games. We prove that by reasoning at level 2 or more and at one level higher than the other agents, our R2-B2 agent can achieve faster asymptotic convergence to no regret than that without utilizing recursive reasoning. We also propose a computationally cheaper variant of R2-B2 called R2-B2-Lite at the expense of a weaker convergence guarantee. The performance and generality of our R2-B2 algorithm are empirically demonstrated using synthetic games, adversarial machine learning, and multi-agent reinforcement learning.
- Private Outsourced Bayesian Optimization.
Dmitrii Kharkovskii, Zhongxiang Dai & Kian Hsiang Low.
In Proceedings of the 37th International Conference on Machine Learning (ICML-20), pages 5231-5242, Jun 12-18, 2020.
21.8% acceptance rate
Abstract. This paper presents the private-outsourced-Gaussian process-upper confidence bound (PO-GP-UCB) algorithm, which is the first algorithm for privacy-preserving Bayesian optimization (BO) in the outsourced setting with a provable performance guarantee. We consider the outsourced setting where the entity holding the dataset and the entity performing BO are represented by different parties, and the dataset cannot be released non-privately. For example, a hospital holds a dataset of sensitive medical records and outsources the BO task on this dataset to an industrial AI company.
The key idea of our approach is to make the BO performance of our algorithm similar to that of non-private GP-UCB run using the original dataset, which is achieved by using a random projection-based transformation that preserves both privacy and the pairwise distances between inputs. Our main theoretical contribution is to show that a regret bound similar to that of the standard GP-UCB algorithm can be established for our PO-GP-UCB algorithm. We empirically evaluate the performance of our PO-GP-UCB algorithm with synthetic and real-world datasets.
- Nonmyopic Gaussian Process Optimization with Macro-Actions.
Dmitrii Kharkovskii, Chun Kai Ling & Kian Hsiang Low.
In Proceedings of the 23rd International Conference on Artificial Intelligence and Statistics (AISTATS-20), pages 4593-4604, Aug 26-28, 2020.
28.7% acceptance rate
Abstract. This paper presents a multi-staged approach to nonmyopic adaptive Gaussian process optimization (GPO) for Bayesian optimization (BO) of unknown, highly complex objective functions that, in contrast to existing nonmyopic adaptive BO algorithms, exploits the notion of macro-actions for scaling up to a further lookahead to match up to a larger available budget. To achieve this, we generalize GP upper confidence bound to a new acquisition function defined w.r.t. a nonmyopic adaptive macro-action policy, which is intractable to be optimized exactly due to an uncountable set of candidate outputs. The contribution of our work here is thus to derive a nonmyopic adaptive ϵ-Bayes-optimal macro-action GPO (ϵ-Macro-GPO) policy. To perform nonmyopic adaptive BO in real time, we then propose an asymptotically optimal anytime variant of our ϵ-Macro-GPO policy with a performance guarantee. We empirically evaluate the performance of our ϵ-Macro-GPO policy and its anytime variant in BO with synthetic and real-world datasets.
- Bayesian Optimization with Binary Auxiliary Information.
Yehong Zhang, Zhongxiang Dai & Kian Hsiang Low.
In Proceedings of the 35th Conference on Uncertainty in Artificial Intelligence (UAI-19), pages 1222-1232, Tel Aviv, Israel, Jul 22-25, 2019.
26.2% acceptance rate (plenary talk)
Subsumes our work on Information-Based Multi-Fidelity Bayesian Optimization presented in
NeurIPS'17 Workshop on Bayesian Optimization, Long Beach, CA, Dec 9, 2017.
Abstract. This paper presents novel mixed-type Bayesian optimization (BO) algorithms to accelerate the optimization of a target objective function by exploiting correlated auxiliary information of binary type that can be more cheaply obtained, such as in policy search for reinforcement learning and hyperparameter tuning of machine learning models with early stopping. To achieve this, we first propose a mixed-type multi-output Gaussian process (MOGP) to jointly model the continuous target function and binary auxiliary functions. Then, we propose information-based acquisition functions such as mixed-type entropy search (MT-ES) and mixed-type predictive ES (MT-PES) for mixed-type BO based on the MOGP predictive belief of the target and auxiliary functions. The exact acquisition functions of MT-ES and MT-PES cannot be computed in closed form and need to be approximated. We derive an efficient approximation of MT-PES via a novel mixed-type random features approximation of the MOGP model whose cross-correlation structure between the target and auxiliary functions can be exploited for improving the belief of the global target maximizer using the observations from evaluating these functions. We also propose new practical constraints to relate the global target maximizer to the binary auxiliary functions. We empirically evaluate the performance of MT-ES and MT-PES with synthetic and real-world experiments.
- Bayesian Optimization Meets Bayesian Optimal Stopping.
Zhongxiang Dai, Haibin Yu, Kian Hsiang Low & Patrick Jaillet.
In Proceedings of the 36th International Conference on Machine Learning (ICML-19), pages 1496-1506, Long Beach, CA, Jun 9-15, 2019.
22.6% acceptance rate
Abstract. Bayesian optimization (BO) is a popular paradigm for optimizing the hyperparameters of machine learning (ML) models due to its sample efficiency. Many ML models require running an iterative training procedure (e.g., stochastic gradient descent). This motivates the question whether information available during the training process (e.g., validation accuracy after each epoch) can be exploited for improving the epoch efficiency of BO algorithms by early-stopping model training under hyperparameter settings that will end up under-performing and hence eliminating unnecessary training epochs. This paper proposes to unify BO (specifically, Gaussian process-upper confidence bound (GP-UCB)) with Bayesian optimal stopping (BO-BOS) to boost the epoch efficiency of BO. To achieve this, while GP-UCB is sample-efficient in the number of function evaluations, BOS complements it with epoch efficiency for each function evaluation by providing a principled optimal stopping mechanism for early stopping. BO-BOS preserves the (asymptotic) no-regret performance of GP-UCB using our specified choice of BOS parameters that is amenable to an elegant interpretation in terms of the exploration-exploitation trade-off. We empirically evaluate the performance of BO-BOS and demonstrate its generality in hyperparameter optimization of ML models and two other interesting applications.
- Decentralized High-Dimensional Bayesian Optimization with Factor Graphs.
Trong Nghia Hoang, Quang Minh Hoang, Ruofei Ouyang & Kian Hsiang Low.
In Proceedings of the 32nd AAAI Conference on Artificial Intelligence (AAAI-18), pages 3231-3238, New Orleans, LA, Feb 2-8, 2018.
24.55% acceptance rate
Abstract. This paper presents a novel decentralized high-dimensional Bayesian optimization (DEC-HBO) algorithm that, in contrast to existing HBO algorithms, can exploit the interdependent effects of various input components on the output of the unknown objective function f for boosting the BO performance and still preserve scalability in the number of input dimensions without requiring prior knowledge or the existence of a low (effective) dimension of the input space. To realize this, we propose a sparse yet rich factor graph representation of f to be exploited for designing an acquisition function that can be similarly represented by a sparse factor graph and hence be efficiently optimized in a decentralized manner using distributed message passing. Despite richly characterizing the interdependent effects of the input components on the output of f with a factor graph, DEC-HBO can still guarantee (asymptotic) no-regret performance. Empirical evaluation on synthetic and real-world experiments shows that DEC-HBO outperforms the state-of-the-art HBO algorithms.
- Data-Efficient Machine Learning with Multiple Output Types and High Input Dimensions.
Yehong Zhang.
Ph.D. Thesis, Department of Computer Science, National University of Singapore, Dec 2017.
Abstract.
Recent research works in machine learning (ML) have focused on learning some target variables of interest to achieve competitive (or state-of-the-art) predictive performance in less time but without requiring large quantities of data, which is known as data-efficient ML. This thesis focuses on two important data-efficient ML approaches: active learning (AL) and Bayesian optimization (BO) which, instead of learning passively from a given small set of data, need to select and gather the most informative observations for learning the target variables of interest more accurately given some budget constraints. To advance the state-of-the-art of data-efficient ML, novel generalizations of AL and BO algorithms are proposed in this thesis for addressing the issues arising from multiple output types and high input dimensions which are the practical settings in many real-world applications.
In particular, this thesis aims to (a) exploit the auxiliary types of outputs which usually coexist and correlate well with the target output types, and more importantly, are less noisy and/or less tedious to sample for improving the learning performance of the target output type in both AL and BO algorithms and (b) scale up the state-of-the-art BO algorithm to high input dimensions. To achieve this, the specific data with multiple output types or high input dimensions is represented using some form of Gaussian process (GP)-based probabilistic regression models which allow the predictive uncertainty of the outputs to be formally quantified and consequently exploited for developing efficient AL and BO algorithms.
To achieve above objectives, an AL algorithm of multi-output GP (MOGP) is first developed for minimizing the predictive uncertainty (i.e., posterior joint entropy) of the target output type. In contrast to existing works, our AL problems involve selecting not just the most informative sampling inputs to be observed but also the types of outputs at each selected input for improving the learning performance of only the target output type given a sampling budget. Unfortunately, such an entropy criterion scales poorly in the numbers of candidate sampling inputs and selected observations when optimized. To resolve this issue, we exploit a structure common to sparse MOGP models for deriving a novel AL criterion. Furthermore, we exploit a relaxed form of submodularity property of our new criterion for devising a polynomial-time approximation algorithm that guarantees a constant-factor approximation of that achieved by the optimal set of selected observations. Empirical evaluation on real-world datasets shows that our proposed approach outperforms existing algorithms for AL of MOGP and single-output GP models.
Secondly, to boost the BO performance by exploiting the cheaper and/or less noisy observations of some auxiliary functions with varying fidelities, we proposed a novel generalization of predictive entropy search (PES) for multi-fidelity BO called multi-fidelity PES (MF-PES). In contrast to existing multi-fidelity BO algorithms, our proposed MF-PES algorithm can naturally trade off between exploitation vs. exploration over the target and auxiliary functions with varying fidelities without needing to manually tune any such parameters. To achieve this, we model the unknown target and auxiliary functions jointly as a convolved MOGP (CMOGP) whose convolutional structure is exploited to formally characterize the fidelity of each auxiliary function through its cross-correlation with the target function. Although the exact acquisition function of MF-PES cannot be computed in closed form, we show that it is in fact possible to derive an efficient approximation of MF-PES via a novel multi-output random features approximation of the CMOGP model whose cross-correlation (i.e., multi-fidelity) structure between the target and auxiliary functions can be exploited for improving the belief of the global target maximizer using the observations from evaluating these functions. Practical constraints are proposed to relate the global target maximizer to that of auxiliary functions. Empirical evaluation on synthetic and real-world experiments shows that MF-PES outperforms the state-of-the-art multi-fidelity BO algorithms.
Lastly, to improve the BO performance in real-world applications with high input
dimensions (e.g., computer vision, biology), we generalize PES for high-dimensional BO by exploiting an additive structure of the target function. New practical constraints are proposed and approximated efficiently such that the proposed acquisition function of additive PES (add-PES) can be optimized independently for each local and low-dimensional input component. The empirical results show that our add-PES considerably improves the performance of the state-of-the-art high-dimensional BO algorithms by using a simple and common setting for optimizing different tested functions with varying input dimensions, which makes it a superior alternative to existing high-dimensional BO algorithms.
- Distributed Batch Gaussian Process Optimization.
Erik Daxberger & Kian Hsiang Low.
In Proceedings of the 34th International Conference on Machine Learning (ICML-17), pages 951-960, Sydney, Australia, Aug 6-11, 2017.
25.9% acceptance rate
Abstract. This paper presents a novel distributed batch Gaussian process upper confidence bound (DB-GP-UCB) algorithm for performing batch Bayesian optimization (BO) of highly complex, costly-to-evaluate black-box objective functions. In contrast to existing batch BO algorithms, DB-GP-UCB can jointly optimize a batch of inputs (as opposed to selecting the inputs of a batch one at a time) while still preserving scalability in the batch size. To realize this, we generalize GP-UCB to a new batch variant amenable to a Markov approximation, which can then be naturally formulated as a multi-agent distributed constraint optimization problem in order to fully exploit the efficiency of its state-of-the-art solvers for achieving linear time in the batch size. Our DB-GP-UCB algorithm offers practitioners the flexibility to trade off between the approximation quality and time efficiency by varying the Markov order. We provide a theoretical guarantee for the convergence rate of DB-GP-UCB via bounds on its cumulative regret. Empirical evaluation on synthetic benchmark objective functions and a real-world optimization problem shows that DB-GP-UCB outperforms the state-of-the-art batch BO algorithms.
- Exploiting Decentralized Multi-Agent Coordination for Large-Scale Machine Learning Problems.
Ruofei Ouyang.
Ph.D. Thesis, Department of Computer Science, National University of Singapore, Dec 2016.
Abstract.
Nowadays, the scale of machine learning problems becomes much larger than before. It raises a huge demand in distributed perception and distributed computation. A multi-agent system provides exceptional scalability for problems like active sensing and data fusion. However, many rich characteristics of large-scale machine learning problems have not been addressed yet such as large input domain, nonstationarity, and high dimensionality. This thesis identifies the challenges related to these characteristics from multi-agent perspective. By exploiting the correlation structure of data in large-scale problems, we propose multi-agent coordination schemes that can improve the scalability of the machine learning models while preserving the computation accuracy. To elaborate, the machine learning problems we are solving with multi-agent coordination techniques are:
- Gaussian process regression. To perform distributed regression on a large-scale environmental phenomenon, data compression is often required due to the communication costs. Currently, decentralized data fusion methods encapsulate the data into local summaries based on a fixed support set. However in a large-scale field, this fixed support set, acting as a centralized component in the decentralized system, cannot approximate the correlation structure of the entire phenomenon well. It leads to evident losses in data summarization. Consequently, the regression performance will be significantly reduced.
In order to approximate the correlation structure accurately, we propose an agent-centric support set to allow every agent in the data fusion system to choose a possibly different support set and dynamically switch to another one during execution for encapsulating its own data into a local summary which, perhaps surprisingly, can still be assimilated with the other agents’ local summaries into a globally consistent summary. Together with an information sharing mechanism we designed, the new decentralized data fusion methods with agent-centric support set can be applied to regression problems on a much larger environmental phenomenon with high performance.
- Active learning. In the context of environmental sensing, active learning/active sensing is a process of taking observations to minimize the uncertainty in an environmental field. The uncertainty is quantified based on the correlation structure of the phenomenon which is traditionally assumed to be stationary for computational sake. In a large-scale environmental field, this stationary assumption is often violated. Therefore, existing active sensing algorithms perform sub-optimally for a nonstationary environmental phenomenon.
To the best of our knowledge, our decentralized multi-robot active sensing (DEC-MAS) algorithm is the first work to address nonstationarity issue in the context of active sensing. The uncertainty in the phenomenon is quantified based on the nonstationary correlation structure estimated by Dirichlet process mixture of Gaussian processes. Further, our DEC-MAS algorithm can efficiently coordinate the exploration of multiple robots to automatically trade-off between learning the unknown, nonstationary correlation structure and minimizing the uncertainty of the environmental phenomenon. It enables multi-agent active sensing techniques to be applied to a large-scale nonstationary environmental phenomenon.
- Bayesian optimization. Optimizing an unknown objective function is challenging for traditional optimization methods. Alternatively, in this situation, people use Bayesian optimization which is a modern optimization technique that can optimize a function by only utilizing the observation information (input and output values) collected through simulations. When the input dimension of the function is low, a few simulated observations can generate good result already. However, for high dimensional function, a huge number of observations are required which is impractical when the simulation consumes lots of time and resources.
Fortunately, many high dimensional problems have sparse correlation structure. Our ANOVA-DCOP work can decompose the correlation structure in the original high-dimensional problem into many correlation structures of subsets of dimensions based on ANOVA kernel function. It significantly reduces the size of input space into a collection of lower-dimensional subspaces. Additionally, we reformulate the Bayesian optimization problem as a decentralized constrained optimization problem (DCOP) that can be efficiently solved by multi-agent coordination techniques so that it can scale up to problems with hundreds of dimensions.
- Gaussian Process Planning with Lipschitz Continuous Reward Functions: Towards Unifying Bayesian Optimization, Active Learning, and Beyond.
Chun Kai Ling, Kian Hsiang Low & Patrick Jaillet.
In Proceedings of the 30th AAAI Conference on Artificial Intelligence (AAAI-16), pages 1860-1866, Phoenix, AZ, Feb 12-17, 2016.
25.75% acceptance rate
Abstract. This paper presents a novel nonmyopic adaptive Gaussian process planning (GPP) framework endowed with a general class of Lipschitz continuous reward functions that can unify some active learning/sensing and Bayesian optimization criteria and offer practitioners some flexibility to specify their desired choices for defining new tasks/problems. In particular, it utilizes a principled Bayesian sequential decision problem framework for jointly and naturally optimizing the exploration-exploitation trade-off. In general, the resulting induced GPP policy cannot be derived exactly due to an uncountable set of candidate observations. A key contribution of our work here thus lies in exploiting the Lipschitz continuity of the reward functions to solve for a nonmyopic adaptive ϵ-optimal GPP (ϵ-GPP) policy. To plan in real time, we further propose an asymptotically optimal, branch-and-bound anytime variant of ϵ-GPP with performance guarantee. We empirically demonstrate the effectiveness of our ϵ-GPP policy and its anytime variant in Bayesian optimization and an energy harvesting task.
PRESENTATIONS
- Learning with Less Data: Automated Machine Learning and Bayesian Optimization.
Kian Hsiang Low.
Invited speaker at the KAUST Research Conference on Robotics and Autonomy, KAUST, Saudi Arabia, Feb 28, 2022.
- Informative Gaussian Process Planning with Lipschitz Continuous Reward Functions: Towards Unifying Adaptive Sampling, Bayesian Optimization, Active Learning, and Beyond.
Kian Hsiang Low.
Invited speaker at the ICRA'18 Workshop on Informative Path Planning and Adaptive Sampling, Brisbane, Australia, May 21, 2018.
Invited speaker at the Symposium on Oceanographic Data Analytics, Norwegian University of Science and Technology, Trondheim, Norway, Nov 27-30, 2018.
EXPLORATION-EXPLOITATION DILEMMA IN ACTIVE LEARNING OF GAUSSIAN PROCESSES
PROJECT DURATION : Aug 2013 - Present
PROJECT AFFILIATION
-
Singapore-MIT Alliance for Research and Technology (SMART) Future Urban Mobility (FM) IRG (Collaborator: Patrick Jaillet, MIT)
-
Sensor-Enhanced Social Media (SeSaMe) Centre (Collaborator: Mohan Kankanhalli)
PROJECT FUNDING
- RIE2020 AME Programmatic Fund : Learning with Less Data, SGD $1,218,600, Apr 2021 - Mar 2024
- Research Collaboration Agreement with Info-Communications Media Development Authority (IMDA) : Robust and Scalable Computer Vision for Scene Understanding, SGD $260,000, Oct 2020 - Oct 2021
- MOE AcRF Tier 2 Grant : Scaling up Gaussian Process Predictive Models for Big Data, SGD $737,461, Jul 2017 - Jul 2020
- SMART Subaward Agreements - FM IRG : Spatiotemporal Modeling and Prediction of Traffic Patterns,
SGD $361,456.17, Oct 2011 - Mar 2017
PROBLEM MOTIVATION
The exploration-exploitation dilemma arises in the following three problems of active learning of Gaussian processes:
- Non-Stationary Gaussian Processes. A key challenge of environmental sensing and monitoring is that of sensing, modeling, and predicting complex urban and natural environmental phenomena, which are typically characterized by spatially correlated measurements. To tackle this challenge, recent research efforts in the robotics community have focused on developing multi-robot active sensing (MAS) algorithms: Their objective is to coordinate the exploration of a team of mobile robots to actively
gather the most informative observations for predicting a spatially varying phenomenon of interest while being subject to resource cost constraints (e.g., number of deployed robots, energy consumption, mission time). To achieve this, a number of MAS algorithms have modeled the phenomenon as a Gaussian process (GP), which allows its spatial correlation structure to be formally characterized and its predictive uncertainty to be formally quantified (e.g., based on mean-squared error, entropy, or mutual information criterion) and subsequently exploited for directing the robots to explore its highly uncertain areas. In order not to incur high computational expense, these algorithms have assumed the spatial correlation structure to be known (or estimated crudely using sparse prior data) and stationary (i.e., degree of smoothness in the spatial variation of the measurements is the same across the entire phenomenon), properties of which are often violated in real-world environmental sensing applications and limited to small-scale phenomena.
In practice, the spatial correlation structure of possibly large-scale environmental phenomena is usually not known and non-stationary (i.e., separate areas of a phenomenon exhibit different local degrees of smoothness in the spatial variation of the measurements). For example, in some ocean phenomena (e.g., temperature, salinity, sea surface height), their measurements far offshore are more smoothly varying (i.e., more spatially correlated) in the cross-shore direction than nearshore. Urban traffic networks also display non-stationary phenomena (e.g., traffic speeds, taxi demands), which pose important considerations to traffic routing and signal control.
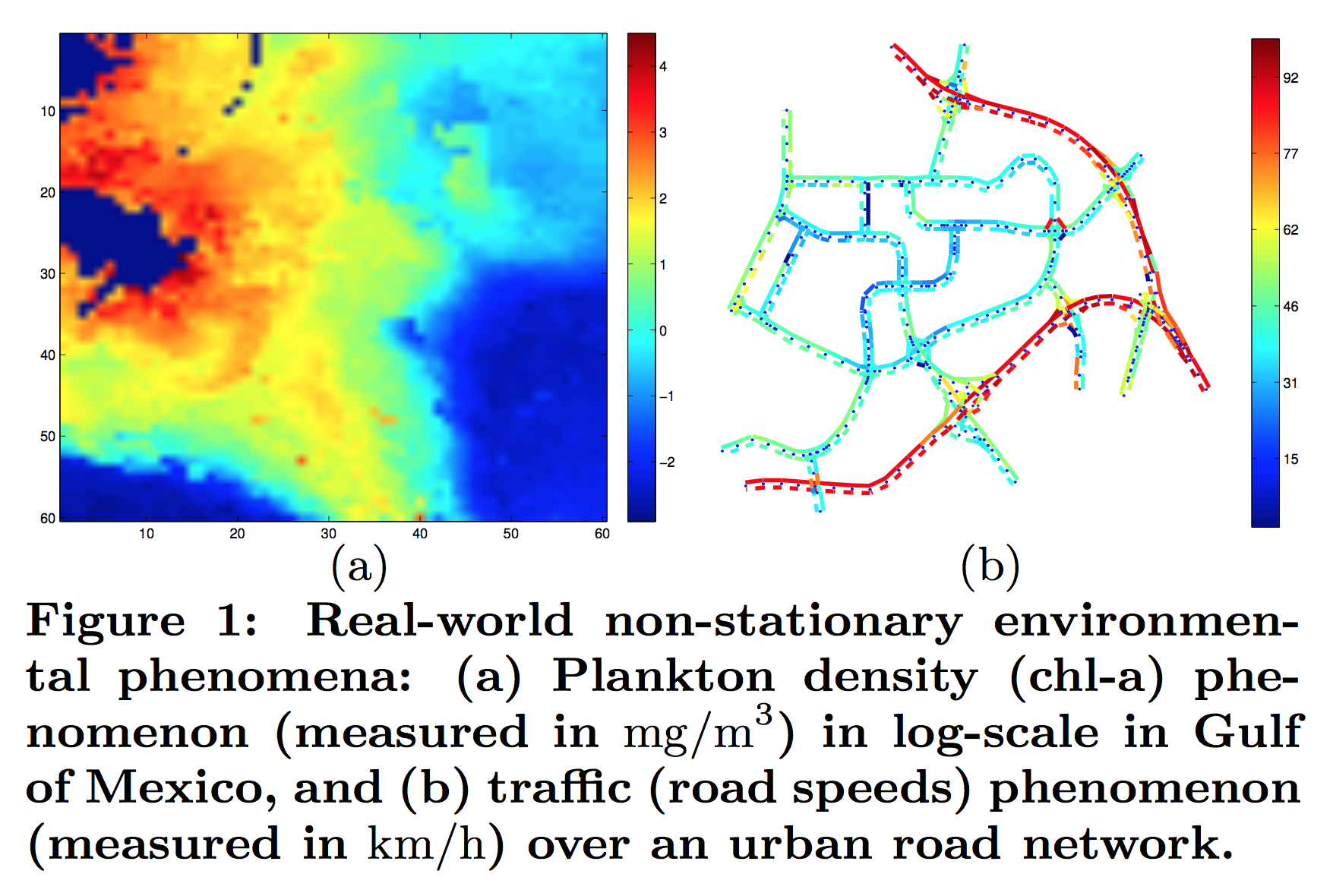
Existing MAS algorithms can still be used for sampling a non-stationary phenomenon by assuming, albeit incorrectly, its spatial correlation structure to be known and stationary in order to preserve time efficiency. So, though they can gather the most informative observations under an assumed stationary correlation structure, they will perform sub-optimally with respect to the true non-stationary correlation structure.
A more desirable MAS algorithm should instead be designed to consider the informativeness of its selected observations for both estimating the unknown spatial correlation structure of a phenomenon (i.e., exploration) as well as predicting the phenomenon given the true correlation structure (i.e., exploitation). According to previous geostatistical studies, the most informative observations that are gathered for achieving the former active sensing criterion are not necessarily as informative for satisfying the latter. This raises a fundamental issue faced by active sensing: How can a MAS algorithm trade off between these two possibly conflicting criteria?
- Nonmyopic Active Learning. Active learning has become an increasingly important focal theme in many environmental sensing and monitoring applications (e.g., precision agriculture, mineral prospecting, monitoring of ocean and freshwater phenomena like harmful algal blooms, forest ecosystems, or pollution) where a high-resolution in situ sampling of the spatial phenomenon of interest is impractical due to prohibitively costly sampling budget requirements (e.g., number of deployed sensors, energy consumption, mission time): For such applications, it is thus desirable to select and gather the most informative observations/data for modeling and predicting the spatially varying phenomenon subject to some budget constraints, which is the goal of active learning and also known as the active sensing problem.
To elaborate, solving the active sensing problem amounts to deriving an optimal sequential policy that plans/decides the most informative locations to be observed for minimizing the predictive uncertainty of the unobserved areas of a phenomenon given a sampling budget. To achieve this, many existing active sensing algorithms have modeled the phenomenon as a Gaussian process (GP), which allows its spatial correlation structure to be formally characterized and its predictive uncertainty to be formally quantified (e.g., based on mean-squared error, entropy, or mutual information criterion). However, they have assumed the spatial correlation structure (specifically, the parameters defining it) to be known, which is often violated in real-world applications, or estimated crudely using sparse prior data. So, though they aim to select sampling locations that are optimal with respect to the assumed or estimated parameters, these locations tend to be sub-optimal with respect to the true parameters, thus degrading the predictive performance of the learned GP model.
In practice, the spatial correlation structure of a phenomenon is usually not known. Then, the predictive performance of the GP modeling the phenomenon depends on how informative the gathered observations/data are for both parameter estimation as well as spatial prediction given the true parameters. Interestingly, as revealed in previous geostatistical studies, policies that are efficient for parameter estimation are not necessarily efficient for spatial prediction with respect to the true model. Thus, the active sensing problem involves a potential trade-off between sampling the most informative locations for spatial prediction given the current, possibly incomplete knowledge of the model parameters (i.e., exploitation) vs. observing locations that gain more information about the parameters (i.e., exploration): How then does an active sensing algorithm trade off between these two possibly conflicting sampling objectives?
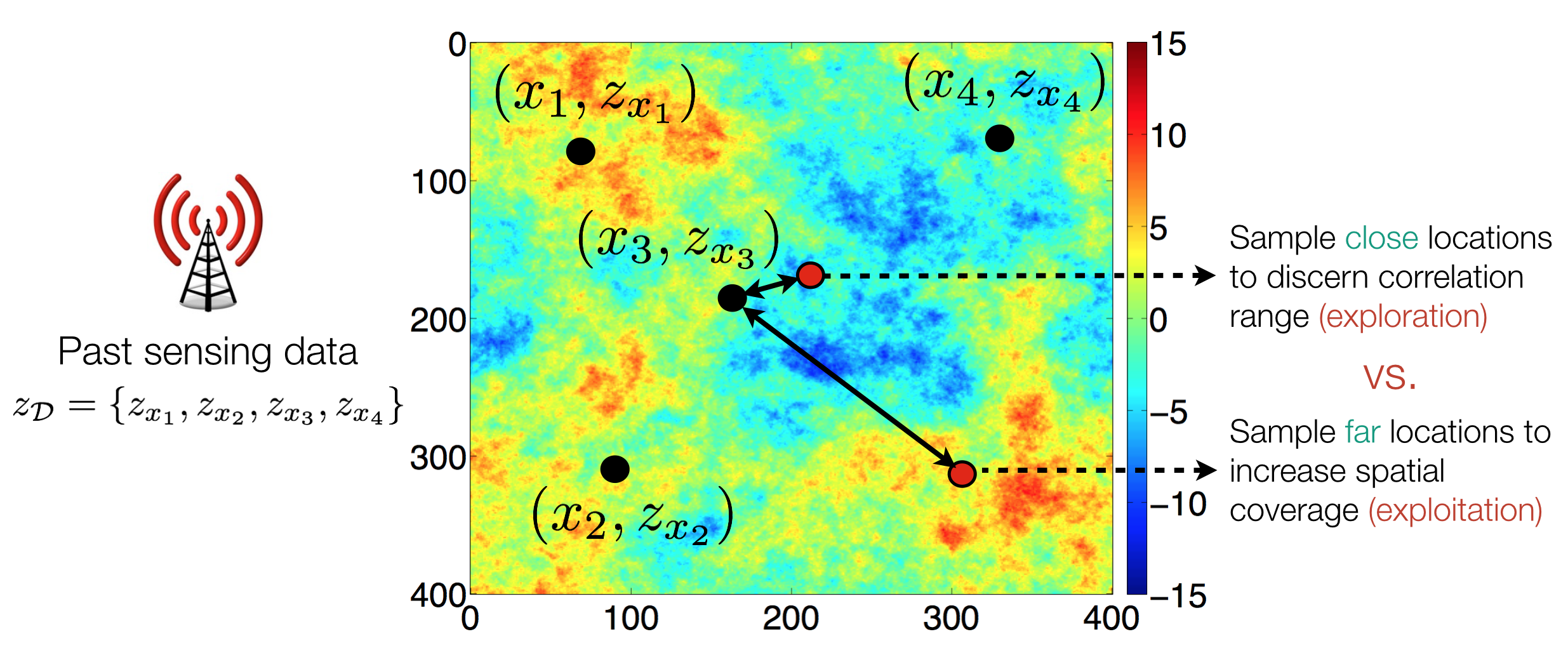
To tackle this question, one principled approach is to frame active sensing as a sequential decision problem that jointly and naturally optimizes the above exploration-exploitation trade-off while maintaining a Bayesian belief over the model parameters. This intuitively means a policy that biases towards observing informative locations for spatial prediction given the current model prior may be penalized if it entails a highly dispersed posterior over the model parameters. So, the resulting induced policy is guaranteed to be optimal in the expected active sensing performance. Unfortunately, such a nonmyopic Bayes-optimal policy cannot be derived exactly due to an uncountable set of candidate observations and unknown model parameters. As a result, most existing works have circumvented the trade-off by resorting to the use of myopic/greedy (hence, sub-optimal) policies. To the best of our knowledge, the only notable nonmyopic active sensing algorithm for GPs advocates tackling exploration and exploitation separately, instead of jointly and naturally optimizing their trade-off, to sidestep the difficulty of solving the Bayesian sequential decision problem. Specifically, it performs a probably approximately correct (PAC)-style exploration until it can verify that the performance loss of greedy exploitation lies within a user-specified threshold. But, such an algorithm is sub-optimal in the presence of budget con- straints due to the following limitations: (a) It is unclear how an optimal threshold for exploration can be determined given a sampling budget, and (b) even if such a threshold is available, the PAC-style exploration is typically designed to satisfy a worst-case sample complexity rather than to be optimal in the expected active sensing performance, thus resulting in an overly-aggressive exploration.
- Multi-Output Gaussian Processes. For many budget-constrained environmental sensing and monitoring applications in the real world, active learning/sensing is an attractive, frugal alternative to passive high-resolution (hence, prohibitively costly) sampling of the spatially varying target phenomenon of interest. Different from the latter, active learning aims to select and gather the most informative observations for modeling and predicting the spatially varying phenomenon given some sampling budget constraints (e.g., quantity of deployed sensors, energy consumption, mission time).
In practice, the target phenomenon often coexists and correlates well with some auxiliary type(s) of phenomena whose measurements may be more spatially correlated, less noisy (e.g., due to higher-quality sensors), and/or less tedious to sample (e.g., due to greater availability/quantity, higher sampling rate, and/or lower sampling cost of deployed sensors of these type(s)) and can consequently be exploited for improving its prediction. For example, to monitor soil pollution by some heavy metal (e.g., Cadmium), its complex and time-consuming extraction from soil samples can be alleviated by supplementing its prediction with correlated auxiliary types of soil measurements (e.g., pH) that are easier to sample. Similarly, to monitor algal bloom in the coastal ocean, plankton abundance correlates well with auxiliary types of ocean measurements (e.g., chlorophyll a, temperature, and salinity) that can be sampled more readily. Other examples of real-world applications include remote sensing, traffic monitoring, monitoring of groundwater and indoor environmental quality, and precision agriculture, among others. All of the above practical examples motivate the need to design and develop an active learning algorithm that selects not just the most informative sampling locations to be observed but also the types of measurements (i.e., target and/or auxiliary) at each selected location for minimizing the predictive uncertainty of unobserved areas of a target phenomenon given a sampling budget, which is the focus of our work here.
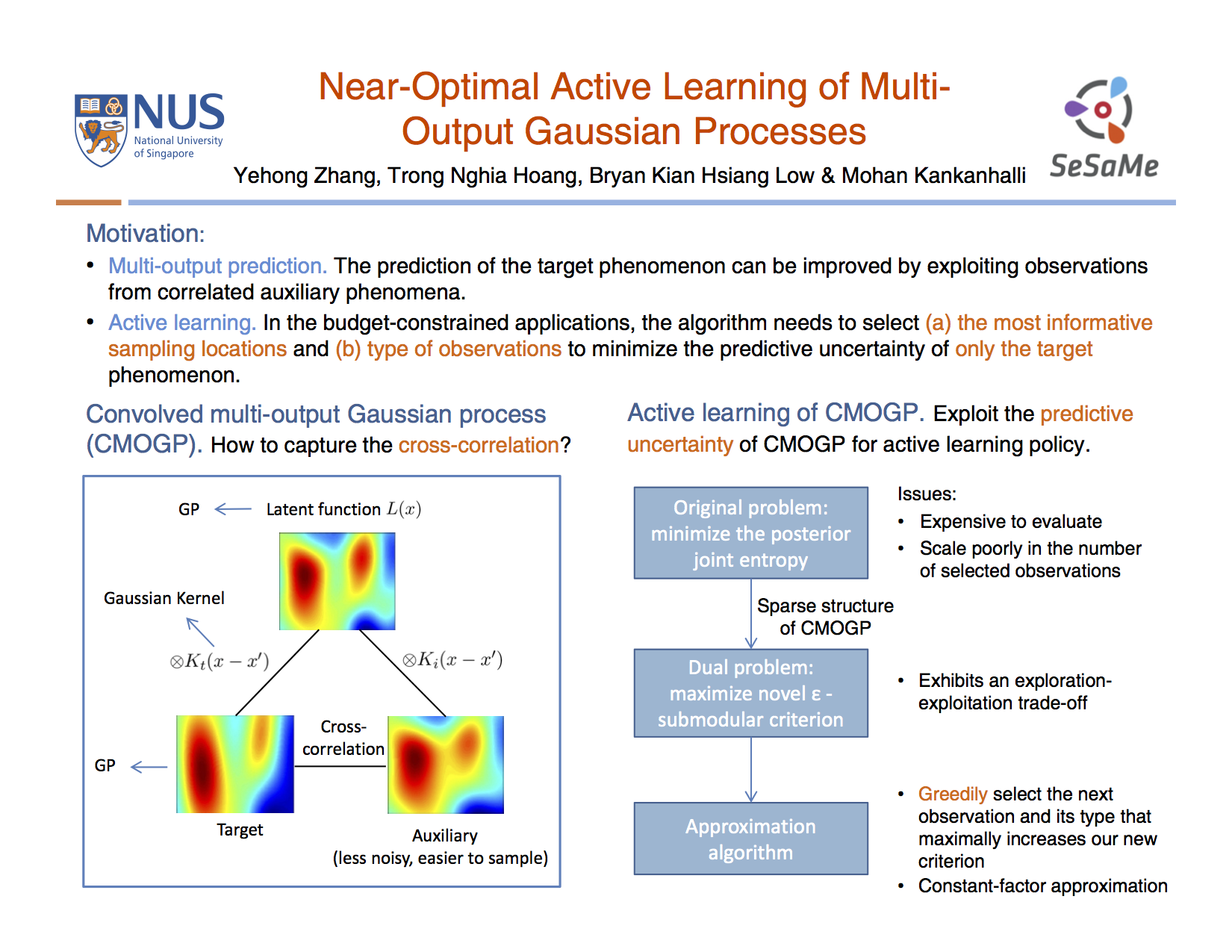
To achieve this, we model all types of coexisting phenomena (i.e., target and auxiliary) jointly as a multi-output Gaussian process (MOGP), which allows the spatial correlation structure of each type of phenomenon and the cross-correlation structure between different types of phenomena to be formally characterized. More importantly, unlike the non-probabilistic multivariate regression methods, the probabilistic MOGP regression model allows the predictive uncertainty of the target phenomenon (as well as the auxiliary phenomena) to be formally quantified (e.g., based on entropy or mutual information criterion) and consequently exploited for deriving the active learning criterion.
PROPOSED METHODOLOGY
- Non-Stationary Gaussian Processes. To address the first problem, our first work presents a decentralized multi-robot active sensing (DEC-MAS) algorithm that can efficiently coordinate the exploration of multiple robots to jointly optimize the above trade-off for sampling unknown, non-stationary environmental phenomena. Our DEC-MAS algorithm models a non-stationary phenomenon as a Dirichlet process mixture of Gaussian processes (DPM-GPs): Using the gathered observations, DPM-GPs can learn to automatically partition the phenomenon into separate local areas, each of which comprises measurements that vary according to a stationary spatial correlation structure and can thus be modeled by a locally stationary GP. The main contributions of our work here are novel in demonstrating how DPM-GPs and its structural properties can be exploited to (a) formalize an active sensing criterion that trades off between gathering the most informative observations for estimating the unknown partition (i.e., a key component of the non-stationary correlation structure) vs. that for predicting the phenomenon given the current, possibly imprecise estimate of the partition, and (b) support effective and efficient decentralized coordination. We also provide a theoretical performance guarantee for DEC-MAS and analyze its time complexity. Finally, we empirically demonstrate using two real-world datasets that DEC-MAS outperforms the state-of-the-art MAS algorithms.
- Nonmyopic Active Learning. To tackle the second problem, our second work presents an efficient decision-theoretic planning approach to nonmyopic active sensing/learning that can still preserve and exploit the principled Bayesian sequential decision problem framework for jointly and naturally optimizing the exploration-exploitation trade-off and consequently does not incur the limitations of the algorithm of Krause & Guestrin (2007). In particular, although the exact Bayes-optimal policy to the active sensing problem cannot be derived, we show that it is in fact possible to solve for a nonmyopic ϵ-Bayes-optimal active learning (ϵ-BAL) policy given a user-defined bound ϵ, which is the main contribution of our work here. In other words, our proposed ϵ-BAL policy can approximate the optimal expected active sensing performance arbitrarily closely (i.e., within an arbitrary loss bound ϵ). In contrast, the algorithm of Krause & Guestrin (2007) can only yield a sub-optimal performance bound. To meet the real-time requirement in time-critical applications, we then propose an asymptotically ϵ-optimal, branch-and-bound anytime algorithm based on ϵ-BAL with performance guarantee. We empirically demonstrate using both synthetic and real-world datasets that, with limited budget, our proposed approach outperforms state-of-the-art algorithms.
- Multi-Output Gaussian Processes. To solve the third problem,
our third work is the first to present an efficient algorithm for active learning of a MOGP model. We consider utilizing the entropy criterion to measure the predictive uncertainty of a target phenomenon, which is widely used for active learning of a single-output GP model. Unfortunately, for the MOGP model, such a criterion scales poorly in the number of candidate sampling locations of the target phenomenon and even more so in the number of selected observations (i.e., sampling budget) when optimized. To resolve this scalability issue, we first exploit a structure common to a unifying framework of sparse MOGP models for deriving a novel active learning criterion.
Our novel active learning criterion exhibits an interesting exploration-exploitation trade-off between
selecting locations with the most uncertain measurements of the target phenomenon to be observed given the latent structure of the sparse MOGP model (i.e., exploitation) vs. selecting locations to be observed (i.e., possibly of auxiliary types of phenomena) so as to rely less on measurements at the remaining unobserved locations (i.e., won't be sampled) of the target phenomenon to infer the latent model structure (i.e., exploration).
Then, we define a relaxed notion of submodularity called ϵ-submodularity and exploit the ϵ-submodularity property of our new criterion for devising a polynomial-time approximation algorithm that guarantees a constant-factor approximation of that achieved by the optimal set of selected observations. We empirically evaluate the performance of our proposed algorithm using three real-world datasets.
PUBLICATIONS
- An Information-Theoretic Framework for Unifying Active Learning Problems.
Quoc Phong Nguyen, Kian Hsiang Low & Patrick Jaillet.
In Proceedings of the 35th AAAI Conference on Artificial Intelligence (AAAI-21), pages 9126-9134, Feb 2-9, 2021.
21.4% acceptance rate
Abstract. This paper presents an information-theoretic framework for unifying active learning problems: level set estimation (LSE), Bayesian optimization (BO), and their generalized variant. We first introduce a novel active learning criterion that subsumes an existing LSE algorithm and achieves state-of-the-art performance in LSE problems with a continuous input domain. Then, by exploiting the relationship between LSE and BO, we design a competitive information-theoretic acquisition function for BO that has interesting connections to upper confidence bound and max-value entropy search (MES). The latter connection reveals a drawback of MES which has important implications on not only MES but also on other MES-based acquisition functions. Finally, our unifying information-theoretic framework can be applied to solve a generalized problem of LSE and BO involving multiple level sets in a data-efficient manner. We empirically evaluate the performance of our proposed algorithms using synthetic benchmark functions, a real-world dataset, and in hyperparameter tuning of machine learning models.
- Data-Efficient Machine Learning with Multiple Output Types and High Input Dimensions.
Yehong Zhang.
Ph.D. Thesis, Department of Computer Science, National University of Singapore, Dec 2017.
Abstract.
Recent research works in machine learning (ML) have focused on learning some target variables of interest to achieve competitive (or state-of-the-art) predictive performance in less time but without requiring large quantities of data, which is known as data-efficient ML. This thesis focuses on two important data-efficient ML approaches: active learning (AL) and Bayesian optimization (BO) which, instead of learning passively from a given small set of data, need to select and gather the most informative observations for learning the target variables of interest more accurately given some budget constraints. To advance the state-of-the-art of data-efficient ML, novel generalizations of AL and BO algorithms are proposed in this thesis for addressing the issues arising from multiple output types and high input dimensions which are the practical settings in many real-world applications.
In particular, this thesis aims to (a) exploit the auxiliary types of outputs which usually coexist and correlate well with the target output types, and more importantly, are less noisy and/or less tedious to sample for improving the learning performance of the target output type in both AL and BO algorithms and (b) scale up the state-of-the-art BO algorithm to high input dimensions. To achieve this, the specific data with multiple output types or high input dimensions is represented using some form of Gaussian process (GP)-based probabilistic regression models which allow the predictive uncertainty of the outputs to be formally quantified and consequently exploited for developing efficient AL and BO algorithms.
To achieve above objectives, an AL algorithm of multi-output GP (MOGP) is first developed for minimizing the predictive uncertainty (i.e., posterior joint entropy) of the target output type. In contrast to existing works, our AL problems involve selecting not just the most informative sampling inputs to be observed but also the types of outputs at each selected input for improving the learning performance of only the target output type given a sampling budget. Unfortunately, such an entropy criterion scales poorly in the numbers of candidate sampling inputs and selected observations when optimized. To resolve this issue, we exploit a structure common to sparse MOGP models for deriving a novel AL criterion. Furthermore, we exploit a relaxed form of submodularity property of our new criterion for devising a polynomial-time approximation algorithm that guarantees a constant-factor approximation of that achieved by the optimal set of selected observations. Empirical evaluation on real-world datasets shows that our proposed approach outperforms existing algorithms for AL of MOGP and single-output GP models.
Secondly, to boost the BO performance by exploiting the cheaper and/or less noisy observations of some auxiliary functions with varying fidelities, we proposed a novel generalization of predictive entropy search (PES) for multi-fidelity BO called multi-fidelity PES (MF-PES). In contrast to existing multi-fidelity BO algorithms, our proposed MF-PES algorithm can naturally trade off between exploitation vs. exploration over the target and auxiliary functions with varying fidelities without needing to manually tune any such parameters. To achieve this, we model the unknown target and auxiliary functions jointly as a convolved MOGP (CMOGP) whose convolutional structure is exploited to formally characterize the fidelity of each auxiliary function through its cross-correlation with the target function. Although the exact acquisition function of MF-PES cannot be computed in closed form, we show that it is in fact possible to derive an efficient approximation of MF-PES via a novel multi-output random features approximation of the CMOGP model whose cross-correlation (i.e., multi-fidelity) structure between the target and auxiliary functions can be exploited for improving the belief of the global target maximizer using the observations from evaluating these functions. Practical constraints are proposed to relate the global target maximizer to that of auxiliary functions. Empirical evaluation on synthetic and real-world experiments shows that MF-PES outperforms the state-of-the-art multi-fidelity BO algorithms.
Lastly, to improve the BO performance in real-world applications with high input
dimensions (e.g., computer vision, biology), we generalize PES for high-dimensional BO by exploiting an additive structure of the target function. New practical constraints are proposed and approximated efficiently such that the proposed acquisition function of additive PES (add-PES) can be optimized independently for each local and low-dimensional input component. The empirical results show that our add-PES considerably improves the performance of the state-of-the-art high-dimensional BO algorithms by using a simple and common setting for optimizing different tested functions with varying input dimensions, which makes it a superior alternative to existing high-dimensional BO algorithms.
- Exploiting Decentralized Multi-Agent Coordination for Large-Scale Machine Learning Problems.
Ruofei Ouyang.
Ph.D. Thesis, Department of Computer Science, National University of Singapore, Dec 2016.
Abstract.
Nowadays, the scale of machine learning problems becomes much larger than before. It raises a huge demand in distributed perception and distributed computation. A multi-agent system provides exceptional scalability for problems like active sensing and data fusion. However, many rich characteristics of large-scale machine learning problems have not been addressed yet such as large input domain, nonstationarity, and high dimensionality. This thesis identifies the challenges related to these characteristics from multi-agent perspective. By exploiting the correlation structure of data in large-scale problems, we propose multi-agent coordination schemes that can improve the scalability of the machine learning models while preserving the computation accuracy. To elaborate, the machine learning problems we are solving with multi-agent coordination techniques are:
- Gaussian process regression. To perform distributed regression on a large-scale environmental phenomenon, data compression is often required due to the communication costs. Currently, decentralized data fusion methods encapsulate the data into local summaries based on a fixed support set. However in a large-scale field, this fixed support set, acting as a centralized component in the decentralized system, cannot approximate the correlation structure of the entire phenomenon well. It leads to evident losses in data summarization. Consequently, the regression performance will be significantly reduced.
In order to approximate the correlation structure accurately, we propose an agent-centric support set to allow every agent in the data fusion system to choose a possibly different support set and dynamically switch to another one during execution for encapsulating its own data into a local summary which, perhaps surprisingly, can still be assimilated with the other agents’ local summaries into a globally consistent summary. Together with an information sharing mechanism we designed, the new decentralized data fusion methods with agent-centric support set can be applied to regression problems on a much larger environmental phenomenon with high performance.
- Active learning. In the context of environmental sensing, active learning/active sensing is a process of taking observations to minimize the uncertainty in an environmental field. The uncertainty is quantified based on the correlation structure of the phenomenon which is traditionally assumed to be stationary for computational sake. In a large-scale environmental field, this stationary assumption is often violated. Therefore, existing active sensing algorithms perform sub-optimally for a nonstationary environmental phenomenon.
To the best of our knowledge, our decentralized multi-robot active sensing (DEC-MAS) algorithm is the first work to address nonstationarity issue in the context of active sensing. The uncertainty in the phenomenon is quantified based on the nonstationary correlation structure estimated by Dirichlet process mixture of Gaussian processes. Further, our DEC-MAS algorithm can efficiently coordinate the exploration of multiple robots to automatically trade-off between learning the unknown, nonstationary correlation structure and minimizing the uncertainty of the environmental phenomenon. It enables multi-agent active sensing techniques to be applied to a large-scale nonstationary environmental phenomenon.
- Bayesian optimization. Optimizing an unknown objective function is challenging for traditional optimization methods. Alternatively, in this situation, people use Bayesian optimization which is a modern optimization technique that can optimize a function by only utilizing the observation information (input and output values) collected through simulations. When the input dimension of the function is low, a few simulated observations can generate good result already. However, for high dimensional function, a huge number of observations are required which is impractical when the simulation consumes lots of time and resources.
Fortunately, many high dimensional problems have sparse correlation structure. Our ANOVA-DCOP work can decompose the correlation structure in the original high-dimensional problem into many correlation structures of subsets of dimensions based on ANOVA kernel function. It significantly reduces the size of input space into a collection of lower-dimensional subspaces. Additionally, we reformulate the Bayesian optimization problem as a decentralized constrained optimization problem (DCOP) that can be efficiently solved by multi-agent coordination techniques so that it can scale up to problems with hundreds of dimensions.
- Near-Optimal Active Learning of Multi-Output Gaussian Processes.
Yehong Zhang, Trong Nghia Hoang, Kian Hsiang Low & Mohan Kankanhalli.
In Proceedings of the 30th AAAI Conference on Artificial Intelligence (AAAI-16), pages 2351-2357, Phoenix, AZ, Feb 12-17, 2016.
25.75% acceptance rate
Abstract. This paper addresses the problem of active learning of a multi-output Gaussian process (MOGP) model representing multiple types of coexisting correlated environmental phenomena. In contrast to existing works, our active learning problem involves selecting not just the most informative sampling locations to be observed but also the types of measurements at each selected location for minimizing the predictive uncertainty (i.e., posterior joint entropy) of a target phenomenon of interest given a sampling budget. Unfortunately, such an entropy criterion scales poorly in the numbers of candidate sampling locations and selected observations when optimized. To resolve this issue, we first exploit a structure common to sparse MOGP models for deriving a novel active learning criterion. Then, we exploit a relaxed form of sub-modularity property of our new criterion for devising a polynomial-time approximation algorithm that guarantees a constant-factor approximation of that achieved by the optimal set of selected observations. Empirical evaluation on real-world datasets shows that our proposed approach outperforms existing algorithms for active learning of MOGP and single-output GP models.
- New Advances on Bayesian and Decision-Theoretic Approaches for Interactive Machine Learning.
Trong Nghia Hoang.
Ph.D. Thesis, Department of Computer Science, National University of Singapore, Feb 2015.
Abstract.
The exploration-exploitation trade-off is a fundamental dilemma in many interactive learning scenarios which include both aspects of reinforcement learning (RL) and active learning (AL): An autonomous agent, situated in an unknown environment, has to actively extract knowledge from the environment by taking actions (or conducting experiments) based on its previously collected information to make accurate predictions or to optimize some utility functions. Thus, to make the most effective use of their resource-constrained budget (e.g., processing time, experimentation cost), the agent must choose carefully between (a) exploiting options (e.g., actions, experiments) which are recommended by its current, possibly incomplete model of the environment, and (b) exploring the other ostensibly sub-optimal choices to gather more information.
For example, an RL agent has to face a dilemma between (a) exploiting the most-rewarding action according to the current statistical model of the environment at the risk of running into catastrophic situations if the model is not accurate, and (b) exploring a sub-optimal action to gather more information so as to improve the model's accuracy at the potential price of losing the short-term reward. Similarly, an AL algorithm/agent has to consider between (a) conducting the most informative experiments according to its current estimation of the environment model's parameters (i.e., exploitation), and (b) running experiments that help improving the estimation accuracy of these parameters (i.e., exploration).
More often, learning strategies that ignore exploration will likely exhibit sub-optimal performance due to their imperfect knowledge while, conversely, those that entirely focus on exploration might suffer the cost of learning without benefitting from it. Therefore, a good exploration-exploitation trade-off is critical to the success of those interactive learning agents: In order to perform well, they must strike the right balance between these two conflicting objectives. Unfortunately, while this trade-off has been well-recognized since the early days of RL, the studies of exploration-exploitation have mostly been developed for theoretical settings in the respective field of RL and, perhaps surprisingly, glossed over in the existing AL literature. From a practical point of view, we see three limiting factors:
- Previous works addressing the exploration-exploitation trade-off in RL have largely focused on simple choices of the environment model and consequently, are not practical enough to accommodate real-world applications that have far more complicated environment structures. In fact, we find that most recent advances in Bayesian reinforcement learning (BRL) have only been able to analytically trade off between exploration and exploitation under a simple choice of models such as Flat-Dirichlet-Multinomial (FDM) whose independence and modeling assumptions do not hold for many real-world applications.
- Nearly all of the notable works in the AL literature primarily advocate the use of greedy/myopic algorithms whose rates of convergence (i.e., the number of experiments required by the learning algorithm to achieve a desired performance in the worst case) are provably minimax optimal for simple classes of learning tasks (e.g., threshold learning). While these results have greatly ad- vanced our understanding about the limit of myopic AL in worst-case scenarios, significantly less is presently known about whether it is possible to devise nonmyopic AL strategies which optimize the exploration-exploitation trade-off to achieve the best expected performance in budgeted learning scenarios.
- The issue of scalability of the existing predictive models (e.g., Gaussian processes) used in AL has generally been underrated since the majority of literature considers small-scale environments which only consist of a few thousand candidate experiments to be selected by single-mode AL algorithms one at a time prior to retraining the model. In contrast, large-scale environments usually have a massive set of million candidate experiments among which tens or hundreds of thousands should be actively selected for learning. For such data-intensive problems, it is often more cost-effective to consider batch-mode AL algorithms which select and conduct multiple experiments in parallel at each stage to collect observations in batch. Retraining the predictive model after incorporating each batch of observations then becomes a computational bottleneck as the collected dataset at each stage quickly grows up to tens or even hundreds of thousand data points.
This thesis outlines some recent progresses that we have been able to make while working toward satisfactory answers to the above challenges, along with practical algorithms that achieve them:
- In particular, in order to put BRL into practice for more complicated and practical problems, we propose a novel framework called Interactive Bayesian Reinforcement Learning (I-BRL) to integrate the general class of parametric models and model priors, thus allowing the practitioners' domain knowledge to be exploited to produce a fine-grained and compact representation of the environment as often required in many real-world applications. Interestingly, we show how the nonmyopic Bayes-optimal policy can be derived analytically by solving I-BRL exactly and propose an approximation algorithm to compute it efficiently in polynomial time. Our empirical studies show that the proposed approach performs competitively with the existing state-of-the-art algorithms.
- Then, to establish a theoretical foundation for the exploration-exploitation trade-off in single-mode active learning scenarios with resource-constrained budgets, we present a novel ϵ-Bayes-optimal Decision-Theoretic Active Learning (ϵ-BAL) framework which advocates the use of differential entropy as a performance measure and consequently, derives a learning policy that can approximate the optimal expected performance arbitrarily closely (i.e., within an arbitrary loss bound ϵ). To meet the real-time requirement in time-critical applications, we then propose an asymptotically ϵ-optimal, branch-and-bound anytime algorithm based on ϵ-BAL with performance guarantees. In practice, we empirically demonstrate with both synthetic and real-world datasets that the proposed approach outperforms the state-of-the-art algorithms in budgeted scenarios.
- Lastly, to facilitate the future developments of large-scale, nonmyopic AL applications, we further introduce a highly scalable family of anytime predictive models for AL which provably converge toward a well-known class of sparse Gaussian processes (SGPs). Unlike the existing predictive models of AL which cannot be updated incrementally and are only capable of processing middle-sized datasets (i.e., a few thousands of data points), our proposed models can process massive datasets in an anytime fashion, thus providing a principled trade-off between the processing time and the predictive accuracy. The efficiency of our framework is then demonstrated empirically on a variety of large-scale real-world datasets which contains hundreds of thousand data points.
- Nonmyopic ϵ-Bayes-Optimal Active Learning of Gaussian Processes.
Trong Nghia Hoang, Kian Hsiang Low, Patrick Jaillet and Mohan Kankanhalli.
In Proceedings of the 31st International Conference on Machine Learning (ICML-14), pages 739-747, Beijing, China, Jun 21-26, 2014.
22.4% acceptance rate (cycle 2)
Also appeared in
RSS-14 Workshop on Non-Parametric Learning in Robotics, Berkeley, CA, Jul 12, 2014.
Abstract. A fundamental issue in active learning of Gaussian processes is that of the exploration-exploitation trade-off.
This paper presents a novel nonmyopic ϵ-Bayes-optimal active learning (ϵ-BAL) approach that jointly and naturally optimizes the trade-off.
In contrast, existing works have primarily developed myopic/greedy algorithms or performed exploration and exploitation separately.
To perform active learning in real time, we then propose an anytime algorithm based on ϵ-BAL with performance guarantee and empirically demonstrate using synthetic and real-world datasets that, with limited budget, it outperforms the state-of-the-art algorithms.
- Active Learning is Planning: Nonmyopic ϵ-Bayes-Optimal Active Learning of Gaussian Processes.
Trong Nghia Hoang, Kian Hsiang Low, Patrick Jaillet and Mohan Kankanhalli.
In T. Calders, F. Esposito, E. Hüllermeier, R. Meo, editors, Machine Learning and Knowledge Discovery in Databases - European Conference, ECML/PKDD-14 Nectar (New Scientific and Technical Advances in Research) Track, Part III, LNCS 8726, pages 494-498, Springer Berlin Heidelberg, Nancy, France, Sep 15-19, 2014.
Abstract. A fundamental issue in active learning of Gaussian processes is that of the exploration-exploitation trade-off. This paper presents a novel nonmyopic ϵ-Bayes-optimal active learning (ϵ-BAL) approach that jointly optimizes the trade-off. In contrast, existing works have primarily developed greedy algorithms or performed exploration and exploitation separately. To perform active learning in real time, we then propose an anytime algorithm based on ϵ-BAL with performance guarantee and empirically demonstrate using a real-world dataset that, with limited budget, it outperforms the state-of-the-art algorithms.
- Multi-Robot Active Sensing of Non-Stationary Gaussian Process-Based Environmental Phenomena.
Ruofei Ouyang, Kian Hsiang Low, Jie Chen & Patrick Jaillet.
In Proceedings of the
13th International Conference on Autonomous Agents and MultiAgent Systems (AAMAS-14), pages 573-580, Paris, France, May 5-9, 2014.
23.8% acceptance rate
Also appeared in
RSS-14 Workshop on Non-Parametric Learning in Robotics, Berkeley, CA, Jul 12, 2014.
Abstract. A key challenge of environmental sensing and monitoring is that of sensing, modeling, and predicting large-scale, spatially correlated environmental phenomena, especially when they are unknown and non-stationary.
This paper presents a decentralized multi-robot active sensing (DEC-MAS) algorithm that can efficiently coordinate the exploration of multiple robots to gather the most informative observations for predicting an unknown, non-stationary phenomenon.
By modeling the phenomenon using a Dirichlet process mixture of Gaussian processes (DPM-GPs), our work here is novel in demonstrating how DPM-GPs and its structural properties can be exploited to (a) formalize an active sensing criterion that trades off between gathering the most informative observations for estimating the unknown, non-stationary spatial correlation structure vs. that for predicting the phenomenon given the current, imprecise estimate of the correlation structure, and (b) support efficient decentralized coordination.
We also provide a theoretical performance guarantee for DEC-MAS and analyze its time complexity.
We empirically demonstrate using two real-world datasets that DEC-MAS outperforms state-of-the-art MAS algorithms.
ONLINE AND ANYTIME SPARSE GAUSSIAN PROCESSES FOR BIG DATA
PROJECT DURATION : Aug 2013 - Present
PROJECT AFFILIATION
-
Singapore-MIT Alliance for Research and Technology (SMART) Future Urban Mobility (FM) IRG (Collaborators: Emilio Frazzoli, MIT; Daniela Rus, MIT)
-
Sensor-Enhanced Social Media (SeSaMe) Centre (Collaborator: Mohan Kankanhalli)
PROJECT FUNDING
- RIE2020 AME Programmatic Fund : Learning with Less Data, SGD $1,218,600, Apr 2021 - Mar 2024
- MOE AcRF Tier 1 Reimagine Research Scheme Funding : Scalable AI Phenome Platform towards Fast-Forward Plant Breeding (Machine Learning),
SGD $348,600, Mar 2021 - Mar 2024
- RIE2020 AME IAF-PP : High Performance Precision Agriculture (HiPPA) System, S$1,197,960, Mar 2020 - Feb 2024
- Research Collaboration Agreement with Info-Communications Media Development Authority (IMDA) : Robust and Scalable Computer Vision for Scene Understanding, SGD $260,000, Oct 2020 - Oct 2021
- MOE AcRF Tier 2 Grant : Scaling up Gaussian Process Predictive Models for Big Data, SGD $737,461, Jul 2017 - Jul 2020
- SMART Subaward Agreements - FM IRG :
Autonomy in Mobility-On-Demand Systems,
SGD $1,348,638.22, Aug 2010 - Dec 2015
- Research Collaboration Agreements with Panasonic R&D Center Singapore : Sonar Data Fusion Algorithm for Object Distance Estimation, SGD $84,230.40, Feb 2016 - Jul 2016, Dec 2016 - Jul 2017
PROBLEM MOTIVATION
A Gaussian process regression (GPR) model is a Bayesian nonparametric model for performing nonlinear regression that provides a Gaussian predictive distribution with formal measures of predictive uncertainty. The expressivity of a full-rank GPR (FGPR) model, however, comes at a cost of cubic time in the size of the data, thus rendering it computationally impractical for training with massive datasets. To improve its scalability, a number of sparse GPR (SGPR) models
exploiting low-rank approximate representations have been proposed, many of which share a similar structural assumption of conditional independence (albeit of varying degrees) based on the notion of inducing variables and consequently incur only linear time in the data size. The work of Quinonero-Candela & Rasmussen (2005) has in fact presented a unifying view of such SGPR models, which include the subset of regressors (SoR), deterministic training conditional (DTC), fully independent training conditional (FITC), fully independent conditional (FIC), partially independent training conditional (PITC), and partially independent conditional (PIC) approximations.
To scale up these SGPR models further for performing real-time predictions necessary in many time-critical applications and decision support systems (e.g., ocean sensing, traffic monitoring), the work of Gal et al. (2014) has parallelized DTC while that of Chen et al. (2013) has parallelized FITC, FIC, PITC, and PIC to be run on multiple machines. The recent work of Low et al. (2015) has produced a spectrum of SGPR models with PIC and FGPR at the two extremes that are also amenable to parallelization on multiple machines. Ideally, these parallel SGPR models can reduce the incurred time of their centralized counterparts by a factor close to the number of machines. In practice, since the number of machines is limited due to budget constraints, their incurred time will still grow with an increasing size of data. Like their centralized counterparts, they can be trained using all the data.
When the data is expected to stream in over a (possibly indefinitely) long time, it is also computationally impractical to repeatedly use these existing offline sparse GP approximation methods or even the online GP model (i.e., quadratic time in the data size) for training at each time step.
PROPOSED METHODOLOGY
A more affordable alternative is to instead train a SGPR model in either an (1) online or (2) anytime fashion with a small, randomly sampled subset of the data at each iteration, which requires only a single machine:
- Our first work presents a novel online sparse GP approximation method that, in contrast to existing works mentioned above, is capable of achieving constant time and memory (i.e., independent of the size of the data/observations) per time step. We provide a theoretical guarantee on its predictive performance to be equivalent to that of the offline sparse PITC approximation method. Our proposed method generalizes the sparse online GP model of Csato & Opper (2002) by relaxing its conditional independence assumption significantly, hence potentially improving the predictive performance. We empirically demonstrate the practical feasibility of using our generalized online sparse GP approximation method through a real-world persistent mobile robot localization experiment.
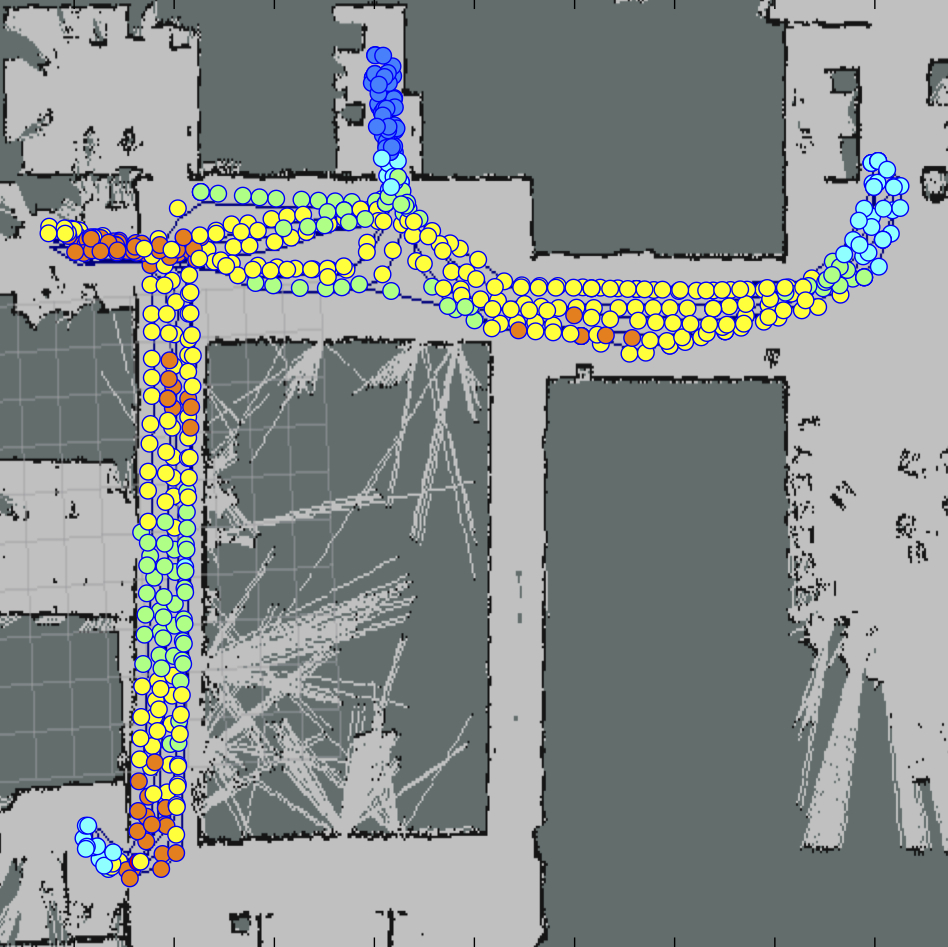
- To the best of our knowledge, the only notable anytime SGPR model exploits a result of Titsias (2009) that DTC can alternatively be obtained using variational inference by minimizing the Kullback-Leibler (KL) distance between the variational approximation and the GP posterior distribution of some latent variables given the data, from which a stochastic natural gradient ascent (SNGA) method can be derived to achieve an asymptotic convergence of its predictive performance to that of DTC while incurring constant time per iteration.
This anytime variant of DTC promises a huge speedup if the number of sampled subsets of data needed for convergence is much smaller than the total number of possible disjoint subsets that can be formed and sampled from all the data. But, it can be observed in our experiments that DTC often does not predict as well as the other SGPR models (except SoR) encompassed by the unifying view of Quinonero-Candela & Rasmussen (2005) because it imposes the most restrictive structural assumption. This motivates us to consider the possibility of constructing an anytime variant of any SGPR model of our choice whose derived SNGA method can achieve an asymptotic convergence of its predictive performance to that of the chosen SGPR model while preserving constant time per iteration.
However, no alternative formulation based on variational inference exists for any SGPR model other than DTC in order to derive such a SNGA method.
To address the above challenge, our second work presents a novel unifying framework of anytime SGPR models that can produce good predictive performance fast and improve their predictive performance over time. Our proposed unifying framework, perhaps surprisingly, reverses the variational inference procedure to theoretically construct a non-trivial, concave functional (i.e., of distributions) that is maximized at the predictive distribution of any SGPR model of our choice. Consequently, a SNGA method can be derived that involves iteratively following the stochastic natural gradient of the functional to improve its estimate of the predictive distribution of the chosen SGPR model and is guaranteed to achieve asymptotic convergence to it.
Interestingly, we show that if the predictive distribution of the chosen SGPR model satisfies certain decomposability conditions (e.g., DTC, FITC, PIC), then the stochastic natural gradient is an unbiased estimator of the exact natural gradient and can be computed in constant time (i.e., independent of data size) at each iteration. We empirically evaluate the trade-off between the predictive performance vs. time efficiency of the anytime SGPR models spanned by our unifying framework (i.e., including state-of-the-art anytime variant of DTC) on two real-world million-sized datasets.
PUBLICATIONS
- Convolutional Normalizing Flows for Deep Gaussian Processes.
Haibin Yu, Dapeng Liu, Kian Hsiang Low & Patrick Jaillet.
In Proceedings of the International Joint Conference on Neural Networks (IJCNN'21), Jul 18-22, 2021.
Abstract. Deep Gaussian processes (DGPs), a hierarchical composition of GP models, have successfully boosted the expressive power of their single-layer counterpart. However, it is impossible to perform exact inference in DGPs, which has motivated the recent development of variational inference-based methods. Unfortunately, either these methods yield a biased posterior belief or it is difficult to evaluate their convergence. This paper introduces a new approach for specifying flexible, arbitrarily complex, and scalable approximate posterior distributions. The posterior distribution is constructed through a normalizing flow (NF) which transforms a simple initial probability into a more complex one through a sequence of invertible transformations. Moreover, a novel convolutional normalizing flow (CNF) is developed to improve the time efficiency and capture dependency between layers. Empirical evaluation shows that CNF DGP outperforms the state-of-the-art approximation methods for DGPs.
- Scalable Variational Bayesian Kernel Selection for Sparse Gaussian Process Regression.
Tong Teng, Jie Chen, Yehong Zhang & Kian Hsiang Low.
In Proceedings of the 34th AAAI Conference on Artificial Intelligence (AAAI-20), pages 5997-6004, New York, NY, Feb 7-12, 2020.
20.6% acceptance rate
Abstract. This paper presents a variational Bayesian kernel selection (VBKS) algorithm for sparse Gaussian process regression (SGPR) models. In contrast to existing GP kernel selection algorithms that aim to select only one kernel with the highest model evidence, our proposed VBKS algorithm considers the kernel as a random variable and learns its belief from data such that the uncertainty of the kernel can be interpreted and exploited to avoid overconfident GP predictions. To achieve this, we represent the probabilistic kernel as an additional variational variable in a variational inference (VI) framework for SGPR models where its posterior belief is learned together with that of the other variational variables (i.e., inducing variables and kernel hyperparameters). In particular, we transform the discrete kernel belief into a continuous parametric distribution via reparameterization in order to apply VI. Though it is computationally challenging to jointly optimize a large number of hyperparameters due to many kernels being evaluated simultaneously by our VBKS algorithm, we show that the variational lower bound of the log-marginal likelihood can be decomposed into an additive form such that each additive term depends only on a disjoint subset of the variational variables and can thus be optimized independently. Stochastic optimization is then used to maximize the variational lower bound by iteratively improving the variational approximation of the exact posterior belief via stochastic gradient ascent, which incurs constant time per iteration and hence scales to big data. We empirically evaluate the performance of our VBKS algorithm on synthetic and massive real-world datasets.
- New Advances in Bayesian Inference for Gaussian Process and Deep Gaussian Process Models.
Haibin Yu.
Ph.D. Thesis, Department of Computer Science, National University of Singapore, May 2020.
Abstract.
Machine learning is the study of letting computers learn to perform a specific task in a data-driven manner. In particular, Bayesian machine learning has attracted enor- mous attention mainly due to their ability to provide uncertainty estimates following Bayesian inference. This thesis focuses on Gaussian processes (GPs), a rich class of Bayesian nonparametric models for performing Bayesian machine learning with formal measures of predictive uncertainty.
However, the applicability of GP in large datasets and in hierarchical composition of GPs is severely limited by computational issues and intractabilities. Therefore, it is crucial to develop accurate and efficient inference algorithms to address these challenges. To this end, this thesis aims at proposing a series of novel approximate Bayesian inference methods for a wide variety of GP models, which unifies the previous literatures, significantly extends them and hopefully lays the foundation for future inference methods.
To start with, this thesis presents a unifying perspective of existing inducing variables-based GP models, sparse GP (SGP) models and variational inference for SGP models (VSGP). Then, to further mitigate the issue of overfitting during optimization, we present a novel variational inference framework for deriving a family of Bayesian SGP regression models, referred to as variational Bayesian SGP (VBSGP) regression models.
Next, taking into account the fact that the expressiveness of GP and SGP depends heavily on the design of the kernel function, we further extend the expressive power of GP by introducing Deep GP (DGP), which is a hierarchical composition of GP models. Unfortunately, exact inference in DGP is intractable, which has motivated the recent development of deterministic and stochastic approximation methods. However, the deterministic approximation methods yield a biased posterior belief while the stochastic one is computationally costly. In this regard, we present the implicit posterior variational inference (IPVI) framework for DGPs that can ideally recover an unbiased posterior belief and still preserve time efficiency. Inspired by generative adversarial networks, our IPVI framework casts the DGP inference problem as a two- player game in which a Nash equilibrium, interestingly, coincides with an unbiased posterior belief.
We hope this thesis at least provides additional confidence and clarity for researchers who are devoting themselves to Bayesian nonparametric models, Gaussian process models in particular. Moreover, we also wish this thesis to offer inspirations for future works, and some thoughts that could be useful for future solutions.
- Implicit Posterior Variational Inference for Deep Gaussian Processes.
Haibin Yu, Yizhou Chen, Zhongxiang Dai, Kian Hsiang Low & Patrick Jaillet.
In Advances in Neural Information Processing Systems 32: 33rd Annual Conference on Neural Information Processing Systems (NeurIPS'19), pages 14475-14486, Vancouver, Canada, Dec 7-12, 2019.
3% acceptance rate (spotlight presentation)
Abstract. A multi-layer deep Gaussian process (DGP) model is a hierarchical composition of GP models with a greater expressive power. Exact DGP inference is intractable, which has motivated the recent development of deterministic and stochastic approximation methods. Unfortunately, the deterministic approximation methods yield a biased posterior belief while the stochastic one is computationally costly. This paper presents an implicit posterior variational inference (IPVI) framework for DGPs that can ideally recover an unbiased posterior belief and still preserve time efficiency. Inspired by generative adversarial networks, our IPVI framework achieves this by casting the DGP inference problem as a two-player game in which a Nash equilibrium, interestingly, coincides with an unbiased posterior belief. This consequently inspires us to devise a best-response dynamics algorithm to search for a Nash equilibrium (i.e., an unbiased posterior belief). Empirical evaluation shows that IPVI outperforms the state-of-the-art approximation methods for DGPs.
- Stochastic Variational Inference for Bayesian Sparse Gaussian Process Regression.
Haibin Yu, Trong Nghia Hoang, Kian Hsiang Low & Patrick Jaillet.
In Proceedings of the International Joint Conference on Neural Networks (IJCNN'19), Budapest, Hungary, Jul 14-19, 2019.
52.4% acceptance rate
Abstract. This paper presents a novel variational inference framework for deriving a family of Bayesian sparse Gaussian process regression (SGPR) models whose approximations are variationally optimal with respect to the full-rank GPR model enriched with various corresponding correlation structures of the observation noises. Our variational Bayesian SGPR (VBSGPR) models jointly treat both the distributions of the inducing variables and hyperparameters as variational parameters, which enables the decomposability of the variational lower bound that in turn can be exploited for stochastic optimization. Such a stochastic optimization involves iteratively following the stochastic gradient of the variational lower bound to improve its estimates of the optimal variational distributions of the inducing variables and hyperparameters (and hence the predictive distribution) of our VBSGPR models and is guaranteed to achieve asymptotic convergence to them. We show that the stochastic gradient is an unbiased estimator of the exact gradient and can be computed in constant time per iteration, hence achieving scalability to big data. We empirically evaluate the performance of our proposed framework on two real-world, massive datasets.
- A Generalized Stochastic Variational Bayesian Hyperparameter Learning Framework for Sparse Spectrum Gaussian Process Regression.
Quang Minh Hoang, Trong Nghia Hoang & Kian Hsiang Low.
In Proceedings of the 31st AAAI Conference on Artificial Intelligence (AAAI-17), pages 2007-2014, San Francisco, CA, Feb 4-9, 2017.
24.6% acceptance rate (oral presentation)
Abstract. While much research effort has been dedicated to scaling up sparse Gaussian process (GP) models based on inducing variables for big data, little attention is afforded to the other less explored class of low-rank GP approximations that exploit the sparse spectral representation of a GP kernel. This paper presents such an effort to advance the state of the art of sparse spectrum GP models to achieve competitive predictive performance for massive datasets. Our generalized framework of stochastic variational Bayesian sparse spectrum GP (sVBSSGP) models addresses their shortcomings by adopting a Bayesian treatment of the spectral frequencies to avoid overfitting, modeling these frequencies jointly in its variational distribution to enable their interaction a posteriori, and exploiting local data for boosting the predictive performance. However, such structural improvements result in a variational lower bound that is intractable to be optimized. To resolve this, we exploit a variational parameterization trick to make it amenable to stochastic optimization. Interestingly, the resulting stochastic gradient has a linearly decomposable structure that can be exploited to refine our stochastic optimization method to incur constant time per iteration while preserving its property of being an unbiased estimator of the exact gradient of the variational lower bound. Empirical evaluation on real-world datasets shows that sVBSSGP outperforms state-of-the-art stochastic implementations of sparse GP models.
- A Unifying Framework of Anytime Sparse Gaussian Process Regression Models with Stochastic Variational Inference for Big Data.
Trong Nghia Hoang, Quang Minh Hoang & Kian Hsiang Low.
In Proceedings of the 32nd International Conference on Machine Learning (ICML-15), pages 569-578, Lille, France, Jul 6-11, 2015.
26.0% acceptance rate
Abstract. This paper presents a novel unifying framework of anytime sparse Gaussian process regression (SGPR) models that can produce good predictive performance fast and improve their predictive performance over time. Our proposed unifying framework reverses the variational inference procedure to theoretically construct a non-trivial, concave functional that is maximized at the predictive distribution of any SGPR model of our choice.
As a result, a stochastic natural gradient ascent method can be derived that involves iteratively following the stochastic natural gradient of the functional to improve its estimate of the predictive distribution of the chosen SGPR model
and is guaranteed to achieve asymptotic convergence to it. Interestingly, we show that if the predictive distribution of the chosen SGPR model
satisfies certain decomposability conditions, then the stochastic natural gradient is an unbiased estimator of the exact natural gradient and can be computed in constant time (i.e., independent of data size) at each iteration. We empirically evaluate the trade-off between the predictive performance vs. time efficiency of the anytime SGPR models on two real-world million-sized datasets.
- New Advances on Bayesian and Decision-Theoretic Approaches for Interactive Machine Learning.
Trong Nghia Hoang.
Ph.D. Thesis, Department of Computer Science, National University of Singapore, Feb 2015.
Abstract.
The exploration-exploitation trade-off is a fundamental dilemma in many interactive learning scenarios which include both aspects of reinforcement learning (RL) and active learning (AL): An autonomous agent, situated in an unknown environment, has to actively extract knowledge from the environment by taking actions (or conducting experiments) based on its previously collected information to make accurate predictions or to optimize some utility functions. Thus, to make the most effective use of their resource-constrained budget (e.g., processing time, experimentation cost), the agent must choose carefully between (a) exploiting options (e.g., actions, experiments) which are recommended by its current, possibly incomplete model of the environment, and (b) exploring the other ostensibly sub-optimal choices to gather more information.
For example, an RL agent has to face a dilemma between (a) exploiting the most-rewarding action according to the current statistical model of the environment at the risk of running into catastrophic situations if the model is not accurate, and (b) exploring a sub-optimal action to gather more information so as to improve the model's accuracy at the potential price of losing the short-term reward. Similarly, an AL algorithm/agent has to consider between (a) conducting the most informative experiments according to its current estimation of the environment model's parameters (i.e., exploitation), and (b) running experiments that help improving the estimation accuracy of these parameters (i.e., exploration).
More often, learning strategies that ignore exploration will likely exhibit sub-optimal performance due to their imperfect knowledge while, conversely, those that entirely focus on exploration might suffer the cost of learning without benefitting from it. Therefore, a good exploration-exploitation trade-off is critical to the success of those interactive learning agents: In order to perform well, they must strike the right balance between these two conflicting objectives. Unfortunately, while this trade-off has been well-recognized since the early days of RL, the studies of exploration-exploitation have mostly been developed for theoretical settings in the respective field of RL and, perhaps surprisingly, glossed over in the existing AL literature. From a practical point of view, we see three limiting factors:
- Previous works addressing the exploration-exploitation trade-off in RL have largely focused on simple choices of the environment model and consequently, are not practical enough to accommodate real-world applications that have far more complicated environment structures. In fact, we find that most recent advances in Bayesian reinforcement learning (BRL) have only been able to analytically trade off between exploration and exploitation under a simple choice of models such as Flat-Dirichlet-Multinomial (FDM) whose independence and modeling assumptions do not hold for many real-world applications.
- Nearly all of the notable works in the AL literature primarily advocate the use of greedy/myopic algorithms whose rates of convergence (i.e., the number of experiments required by the learning algorithm to achieve a desired performance in the worst case) are provably minimax optimal for simple classes of learning tasks (e.g., threshold learning). While these results have greatly ad- vanced our understanding about the limit of myopic AL in worst-case scenarios, significantly less is presently known about whether it is possible to devise nonmyopic AL strategies which optimize the exploration-exploitation trade-off to achieve the best expected performance in budgeted learning scenarios.
- The issue of scalability of the existing predictive models (e.g., Gaussian processes) used in AL has generally been underrated since the majority of literature considers small-scale environments which only consist of a few thousand candidate experiments to be selected by single-mode AL algorithms one at a time prior to retraining the model. In contrast, large-scale environments usually have a massive set of million candidate experiments among which tens or hundreds of thousands should be actively selected for learning. For such data-intensive problems, it is often more cost-effective to consider batch-mode AL algorithms which select and conduct multiple experiments in parallel at each stage to collect observations in batch. Retraining the predictive model after incorporating each batch of observations then becomes a computational bottleneck as the collected dataset at each stage quickly grows up to tens or even hundreds of thousand data points.
This thesis outlines some recent progresses that we have been able to make while working toward satisfactory answers to the above challenges, along with practical algorithms that achieve them:
- In particular, in order to put BRL into practice for more complicated and practical problems, we propose a novel framework called Interactive Bayesian Reinforcement Learning (I-BRL) to integrate the general class of parametric models and model priors, thus allowing the practitioners' domain knowledge to be exploited to produce a fine-grained and compact representation of the environment as often required in many real-world applications. Interestingly, we show how the nonmyopic Bayes-optimal policy can be derived analytically by solving I-BRL exactly and propose an approximation algorithm to compute it efficiently in polynomial time. Our empirical studies show that the proposed approach performs competitively with the existing state-of-the-art algorithms.
- Then, to establish a theoretical foundation for the exploration-exploitation trade-off in single-mode active learning scenarios with resource-constrained budgets, we present a novel ϵ-Bayes-optimal Decision-Theoretic Active Learning (ϵ-BAL) framework which advocates the use of differential entropy as a performance measure and consequently, derives a learning policy that can approximate the optimal expected performance arbitrarily closely (i.e., within an arbitrary loss bound ϵ). To meet the real-time requirement in time-critical applications, we then propose an asymptotically ϵ-optimal, branch-and-bound anytime algorithm based on ϵ-BAL with performance guarantees. In practice, we empirically demonstrate with both synthetic and real-world datasets that the proposed approach outperforms the state-of-the-art algorithms in budgeted scenarios.
- Lastly, to facilitate the future developments of large-scale, nonmyopic AL applications, we further introduce a highly scalable family of anytime predictive models for AL which provably converge toward a well-known class of sparse Gaussian processes (SGPs). Unlike the existing predictive models of AL which cannot be updated incrementally and are only capable of processing middle-sized datasets (i.e., a few thousands of data points), our proposed models can process massive datasets in an anytime fashion, thus providing a principled trade-off between the processing time and the predictive accuracy. The efficiency of our framework is then demonstrated empirically on a variety of large-scale real-world datasets which contains hundreds of thousand data points.
- GP-Localize: Persistent Mobile Robot Localization using Online Sparse Gaussian Process Observation Model.
Nuo Xu, Kian Hsiang Low, Jie Chen, Keng Kiat Lim & Etkin Baris Ozgul.
In Proceedings of the 28th AAAI Conference on Artificial Intelligence (AAAI-14), pages 2585-2592, Quebec City, Canada, Jul 27-31, 2014.
16.6% acceptance rate (oral presentation)
Also appeared in
RSS-14 Workshop on Non-Parametric Learning in Robotics, Berkeley, CA, Jul 12, 2014.
Abstract. Central to robot exploration and mapping is the task of persistent localization in environmental fields characterized by spatially correlated measurements. This paper presents a Gaussian process localization (GP-Localize) algorithm that, in contrast to existing works, can exploit the spatially correlated field measurements taken during a robot's exploration (instead of relying on prior training data) for efficiently and scalably learning the GP observation model online through our proposed novel online sparse GP. As a result, GP-Localize is capable of achieving constant time and memory (i.e., independent of the size of the data) per filtering step, which demonstrates the practical feasibility of using GPs for persistent robot localization and autonomy. Empirical evaluation via simulated experiments with real-world datasets and a real robot experiment shows that GP-Localize outperforms existing GP localization algorithms.
- Generalized Online Sparse Gaussian Processes with Application to Persistent Mobile Robot Localization.
Kian Hsiang Low, Nuo Xu, Jie Chen, Keng Kiat Lim & Etkin Baris Ozgul.
In T. Calders, F. Esposito, E. Hüllermeier, R. Meo, editors, Machine Learning and Knowledge Discovery in Databases - European Conference, ECML/PKDD-14 Nectar (New Scientific and Technical Advances in Research) Track, Part III, LNCS 8726, pages 499-503, Springer Berlin Heidelberg, Nancy, France, Sep 15-19, 2014.
Abstract. This paper presents a novel online sparse Gaussian process (GP) approximation method that is capable of achieving constant time and memory (i.e., independent of the size of the data) per time step. We theoretically guarantee its predictive performance to be equivalent to that of a sophisticated offline sparse GP approximation method. We empirically demonstrate the practical feasibility of using our online sparse GP approximation method through a real-world persistent mobile robot localization experiment.
PARALLEL AND DISTRIBUTED SPARSE GAUSSIAN PROCESSES FOR BIG DATA
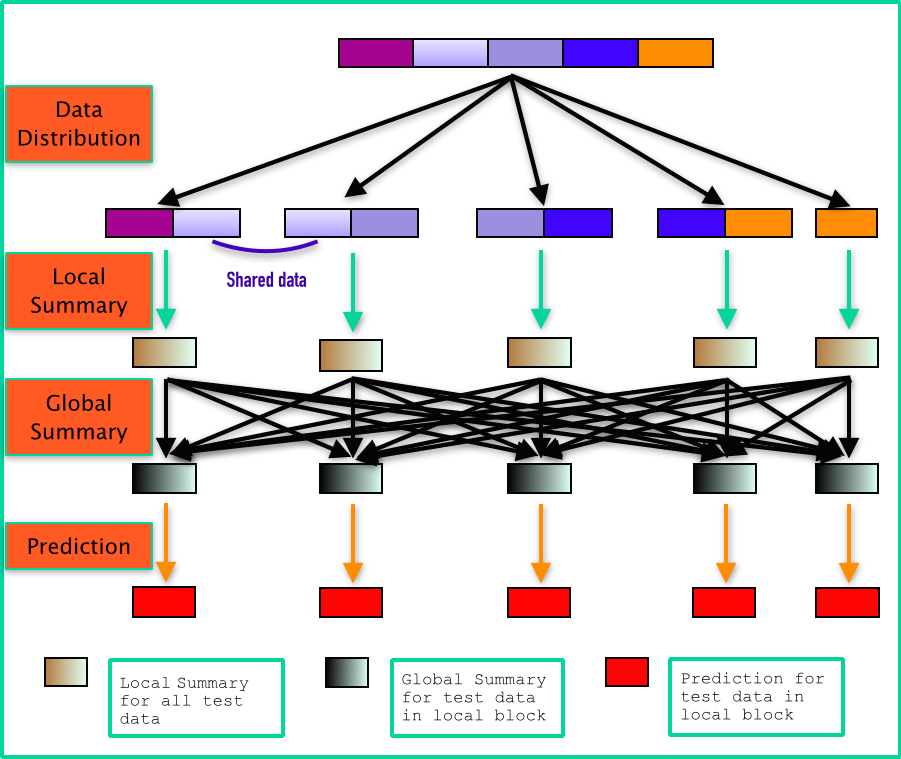
PROJECT DURATION : Aug 2010 - Present
PROJECT AFFILIATION
-
Singapore-MIT Alliance for Research and Technology (SMART) Future Urban Mobility (FM) IRG (Collaborator: Patrick Jaillet, MIT)
-
Sensor-Enhanced Social Media (SeSaMe) Centre (Collaborator: Mohan Kankanhalli)
PROJECT FUNDING
- RIE2020 AME Programmatic Fund : Learning with Less Data, SGD $1,218,600, Apr 2021 - Mar 2024
- MOE AcRF Tier 1 Reimagine Research Scheme Funding : Scalable AI Phenome Platform towards Fast-Forward Plant Breeding (Machine Learning),
SGD $348,600, Mar 2021 - Mar 2024
- RIE2020 AME IAF-PP : High Performance Precision Agriculture (HiPPA) System, S$1,197,960, Mar 2020 - Feb 2024
- MOE AcRF Tier 2 Grant : Scaling up Gaussian Process Predictive Models for Big Data, SGD $737,461, Jul 2017 - Jul 2020
- SMART Subaward Agreements - FM IRG :
Spatiotemporal Modeling and Prediction of Traffic Patterns,
SGD $361,456.17, Oct 2011 - Mar 2017
- Research Collaboration Agreement with Sumitomo Electric Industries, Ltd. : Estimation/Prediction Algorithm for Traffic Volume without Rich Installation of Detectors, JPY $3,000,000, Sep 2013 - Nov 2014
PROBLEM MOTIVATION
Gaussian process (GP) models are a rich class of Bayesian non-parametric models that can perform probabilistic regression by providing Gaussian predictive distributions with formal measures of the predictive uncertainty.
Unfortunately, a GP model is handicapped by its poor scalability in the size of the data, hence limiting its practical use to small data. To improve its scalability, two families of sparse GP regression methods have been proposed: (a) Low-rank approximate representations
of the full-rank GP (FGP) model are well-suited for modeling slowly-varying functions with large correlation and can use all the data for predictions. But, they require a relatively high rank to capture small-scale features/patterns (i.e., of small correlation) with high fidelity, thus losing their computational advantage. (b) In contrast, localized regression and covariance tapering methods (e.g., local GPs and compactly supported covariance functions) are particularly useful for modeling rapidly-varying functions with small correlation. However, they can only utilize local data for predictions, thereby performing poorly in input regions with little/no data. Furthermore, to accurately represent large-scale features/patterns (i.e., of large correlation), the locality/tapering range has to be increased considerably, thus sacrificing their time efficiency.
Recent sparse GP regression methods have unified approaches from the two families described above to harness their complementary modeling and predictive capabilities (hence, eliminating their deficiencies) while retaining their computational advantages. Specifically, after approximating the FGP (in particular, its covariance matrix) with a low-rank representation based on the notion of inducing variables, a sparse covariance matrix approximation of the resulting residual process is made. However, this sparse residual covariance matrix approximation imposes a fairly strong conditional independence assumption given the inducing variables since the number of inducing variables cannot be too large to preserve time efficiency. We argue in this work that such a strong assumption is an overkill: It is in fact possible to construct a more refined, dense residual covariance matrix approximation by exploiting a Markov assumption and, perhaps surprisingly, still achieve scalability, which distinguishes our work here from existing sparse GP regression methods utilizing low-rank representations (i.e., including the unified approaches) described earlier.
As a result, our proposed residual covariance matrix approximation can significantly relax the conditional independence assumption (especially with larger data), hence potentially improving the predictive performance.
PROPOSED METHODOLOGY
This work presents a low-rank-cum-Markov approximation (LMA) of the FGP model that is novel in leveraging the dual computational advantages stemming from complementing the reduced-rank covariance matrix approximation based on the inducing variables with the residual covariance matrix approximation due to the Markov assumption;
the latter approximation is guaranteed to be closest in the Kullback-Leibler distance criterion subject to some constraint. Consequently, our proposed LMA method can trade off between the number of inducing variables and the order of the Markov property to (a) incur lower computational cost than sparse GP regression methods utilizing low-rank representations with only the number of inducing variables or spectral points as the varying parameter while achieving predictive performance comparable to them and (b) accurately represent features/patterns of any scale.
Interestingly, varying the Markov order produces a spectrum of LMAs with the partially independent conditional (PIC) approximation and FGP at the two extremes. An important advantage of LMA over most existing sparse GP regression methods is that it is amenable to parallelization on multiple machines/cores, thus gaining greater scalability for performing real-time predictions necessary in many time-critical applications and decision support systems (e.g., ocean sensing, traffic monitoring). Our parallel LMA method is implemented using the message passing interface (MPI) framework to run in clusters of up to 32 computing nodes and its predictive performance, scalability, and speedup are empirically evaluated on three real-world datasets (i.e., including a million-sized dataset).
PUBLICATIONS
- A Distributed Variational Inference Framework for Unifying Parallel Sparse Gaussian Process Regression Models.
Trong Nghia Hoang, Quang Minh Hoang & Kian Hsiang Low.
In Proceedings of the 33rd International Conference on Machine Learning (ICML-16), pages 382-391, New York City, NY, Jun 19-24, 2016.
24.3% acceptance rate
Abstract. This paper presents a novel distributed variational inference framework that unifies many parallel sparse Gaussian process regression (SGPR) models for scalable hyperparameter learning with big data. To achieve this, our framework exploits a structure of correlated noise process model that represents the observation noises as a finite realization of a high-order Gaussian Markov random process. By varying the Markov order and covariance function for the noise process model, different variational SGPR models result. This consequently allows the correlation structure of the noise process model to be characterized for which a particular variational SGPR model is optimal. We empirically evaluate the predictive performance and scalability of the distributed variational SGPR models unified by our framework on two real-world datasets.
- Parallel Gaussian Process Regression for Big Data: Low-Rank Representation Meets Markov Approximation.
Kian Hsiang Low, Jiangbo Yu, Jie Chen & Patrick Jaillet.
In Proceedings of the 29th AAAI Conference on Artificial Intelligence (AAAI-15), pages 2821-2827, Austin, TX, Jan 25-29, 2015.
26.67% acceptance rate
Abstract. The expressive power of a Gaussian process (GP) model comes at a cost of poor scalability in the data size.
To improve its scalability, this paper presents a low-rank-cum-Markov approximation (LMA) of the GP model that is novel in leveraging the dual computational advantages stemming from complementing a low-rank approximate representation of the full-rank GP based on a support set of inputs with a Markov approximation of the resulting residual process; the latter approximation is guaranteed to be closest in the Kullback-Leibler distance criterion subject to some constraint
and is considerably more refined than that of existing sparse GP models utilizing low-rank representations due to its more relaxed conditional independence assumption (especially with larger data).
As a result, our LMA method can trade off between the size of the support set and the order of the Markov property to (a) incur lower computational cost than such sparse GP models while achieving predictive performance comparable to them and (b) accurately represent features/patterns of any scale.
Interestingly, varying the Markov order produces a spectrum of LMAs
with PIC approximation and full-rank GP at the two extremes.
An advantage of our LMA method is that it is amenable to parallelization on multiple machines/cores, thereby gaining greater scalability.
Empirical evaluation on three real-world datasets in clusters of up to 32 computing nodes shows that our centralized and parallel LMA methods are significantly more time-efficient and scalable than state-of-the-art sparse and full-rank GP regression methods
while achieving comparable predictive performances.
- Parallel Gaussian Process Regression with Low-Rank Covariance Matrix Approximations.
Jie Chen, Nannan Cao, Kian Hsiang Low, Ruofei Ouyang, Colin Keng-Yan Tan & Patrick Jaillet.
In Proceedings of the 29th Conference on Uncertainty in Artificial Intelligence (UAI-13), pages 152-161, Bellevue, WA, Jul 11-15, 2013.
31.3% acceptance rate
Abstract. Gaussian processes (GP) are Bayesian non-parametric models that are widely used for probabilistic regression. Unfortunately, it cannot scale well with large data nor perform real-time predictions due to its cubic time cost in the data size. This paper presents two parallel GP regression methods that exploit low-rank covariance matrix approximations for distributing the computational load among parallel machines to achieve time efficiency and scalability. We theoretically guarantee the predictive performances of our proposed parallel GPs to be equivalent to that of some centralized approximate GP regression methods: The computation of their centralized counterparts can be distributed among parallel machines, hence achieving greater time efficiency and scalability. We analytically compare the properties of our parallel GPs such as time, space, and communication complexity. Empirical evaluation on two real-world datasets in a cluster of 20 computing nodes shows that our parallel GPs are significantly more time-efficient and scalable than their centralized counterparts and exact/full GP while achieving predictive performances comparable to full GP.
- Gaussian Process-Based Decentralized Data Fusion and Active Sensing Agents: Towards Large-Scale Modeling and Prediction of Spatiotemporal Traffic Phenomena.
Jie Chen.
Ph.D. Thesis, Department of Computer Science, National University of Singapore, Dec 2013.
Abstract.
Knowing and understanding the environmental phenomena is important to many real world applications. This thesis is devoted to study large-scale modeling and prediction of spatiotemporal environmental phenomena (i.e., urban traffic phenomena). Towards this goal, our proposed approaches rely on a class of Bayesian non-parametric models: Gaussian processes (GP).
To accurately model spatiotemporal urban traffic phenomena in real world situation, a novel relational GP taking into account both the road segment features and road network topology information is proposed to model real world traffic conditions over road network. Additionally, a GP variant called log-Gaussian process (lGP) is exploited to model an urban mobility demand pattern which contains skewness and extremity in demand measurements.
To achieve efficient and scalable urban traffic phenomenon prediction given a large phenomenon data, we propose three novel parallel GPs: parallel partially independent training conditional (pPITC), parallel partially independent conditional(pPIC) and parallel incomplete Cholesky factorization (pICF)-based approximations of GP model, which can distribute their computational load into a cluster of parallel/multi-core machines, thereby achieving time efficiency. The predictive performances of such parallel GPs are theoretically guaranteed to be equivalent to that of some centralized approaches to approximate full/exact GP regression. The proposed parallel GPs are implemented using the message passing interface (MPI) framework and tested on two large real world datasets. The theoretical and empirical results show that our parallel GPs achieve significantly better time efficiency and scalability than that of full GP, while achieving comparable accuracy. They also achieve fine speedup performance that is the ratio of time required by the parallel algorithms and their centralized counterparts.
To exploit active mobile sensors to perform decentralized perception of the spatiotemporal urban traffic phenomenon, we propose a decentralized algorithm framework: Gaussian process-based decentralized data fusion and active sensing (D2FAS) which is composed of a decentralized data fusion (DDF) component and a decentralized active sensing (DAS) component. The DDF component includes a novel Gaussian process-based decentralized data fusion (GP-DDF) algorithm that can achieve remarkably efficient and scalable prediction of phenomenon and a novel Gaussian process-based decentralized data fusion with local augmentation (GP-DDF+) algorithm that can achieve better predictive accuracy while preserving time efficiency of GP-DDF. The predictive performances of both GP-DDF and GP-DDF+ are theoretically guaranteed to be equivalent to that of some sophisticated centralized sparse approximations of exact/full GP. For the DAS component, we propose a novel partially decentralized active sensing (PDAS) algorithm that exploits property in correlation structure of GP-DDF to enable mobile sensors cooperatively gathering traffic phenomenon data along a near-optimal joint walk with theoretical guarantee, and a fully decentralized active sensing (FDAS) algorithm that guides each mobile sensor gather phenomenon data along its locally optimal walk.
Lastly, to justify the practicality of the D2FAS framework, we develop and test D2FAS algorithms running with active mobile sensors on real world datasets for monitoring traffic conditions and sensing/servicing urban mobility demands. Theoretical and empirical results show that the proposed algorithms are significantly more time-efficient, more scalable in the size of data and in the number of sensors than the state-of-the-art centralized approaches, while achieving comparable predictive accuracy.
PRESENTATIONS
- Gaussian Process-Based Decentralized Data Fusion
and Active Sensing Agents:
Towards Large-Scale Modeling & Prediction of Spatiotemporal Traffic Phenomena.
Kian Hsiang Low.
Invited speaker at the RSS-13 Workshop on Robotic Exploration, Monitoring, and Information Collection: Nonparametric Modeling, Information-based Control, and Planning under Uncertainty, Berlin, Germany, Jun 27-28, 2013.
INTENTION-AWARE PLANNING UNDER UNCERTAINTY FOR INTERACTING OPTIMALLY WITH SELF-INTERESTED AGENTS
PROJECT DURATION : May 2011 - Present
PROJECT AFFILIATION :
Singapore-MIT Alliance for Research and Technology (SMART) Future Urban Mobility (FM) IRG (Collaborators: Emilio Frazzoli, MIT; Daniela Rus, MIT)
PROJECT FUNDING : SMART Subaward Agreements - FM IRG :
Autonomy in Mobility-On-Demand Systems,
SGD $1,348,638.22, Aug 2010 - Dec 2015
PROBLEM MOTIVATION
Designing and developing efficient planning algorithms for intelligent agents to interact and perform effectively among other self-interested agents has recently emerged as a grand challenge in non-cooperative multi-agent systems. Such a challenge is posed by many real-world applications, which include automated electronic trading markets where software agents interact, and traffic intersections where autonomous cars have to negotiate with human-driven vehicles to cross them, among others. Modeling, predicting, and learning the other agents' intentions efficiently is therefore critical to overcoming this challenge.
In practice, it is highly non-trivial to model and predict the other agents' intentions efficiently. Existing works addressing this challenge are often undermined due to either the restrictive assumptions on the agents' behaviors or the prohibitively expensive cost of modeling and predicting their intentions:
- Game-theoretic approaches tend to assume the agents' behaviors to be perfectly rational using the well-founded solution concepts of classical game theory such as Nash equilibrium that suffers from the following drawbacks: (a) Multiple equilibria may exist, (b) only the optimal actions corresponding to the equilibria are specified, and (c) they assume that the agents do not collaborate to beneficially deviate from the equilibrium, which is often violated by human agents.
- In contrast, decision-theoretic approaches propose to extend single-agent sequential decision making frameworks under partial observability such as POMDP to explicitly characterize the bounded rationality of self-interested agents. In particular, Interactive POMDP (I-POMDP) replaces a POMDP's flat belief over physical states with an interactive belief of k levels of hierarchy over both the physical state space and the other agent's beliefs, the latter of which are recursively defined as interactive beliefs of k-1 levels of hierarchy.
As a consequence, the agent's "optimal" behavior computed at hierarchical level k is expected to be the best response to the other agent's expected behavior at hierarchical level k-1. This surprisingly coincides with the well-founded cognitive hierarchy model of games where k is referred to as the reasoning depth. Here, the bounded rationality of the agents is explicitly accounted for by making k finite and defining their expected behaviors at level 0 as uniformly random. Empowered by such enriched and highly expressive belief space, I-POMDP can explicitly model and predict the other agent's intention. Unfortunately, solving I-POMDP (e.g., solving for the agent's expected behavior at level k) is fraught with computational curses of dimensionality, history, and nested reasoning due to its highly sophisticated structure.
Furthermore, there is another practical concern for these decision-theoretic approaches: They often require the behavioral model's parameters (e.g., hierarchical level k) to be completely specified by the practitioners, which can be very impractical in many situations where it is non-trivial to do so or the prior knowledge is insufficient to reliably derive these parameters. This essentially boils down to the need of learning while interacting with the other self-interested agents and, interestingly, an exploitation-exploration trade-off to be made while doing so: Should an agent exploit the "best" action based on its (possibly misleading) knowledge to maximize the payoff or explore a "sub-optimal" action to refine its knowledge?
Naively, one may attempt to solve it by directly tapping into the huge body of existing works in Bayesian Reinforcement Learning (BRL), which offers a broad range of principled treatments of this issue under single-agent contexts. However, most of these works often assume very simple and specific parameterizations of the unknown environments, thus rendering them inapplicable to the context where the other agent's behavior has a far more complicated parameterization. More importantly, the other agent's behavior often needs to be modeled differently depending on the specific context. Grounding in the context of existing BRL frameworks, either the domain expert struggles to best fit his prior knowledge to the supported set of parameterizations or the agent developer has to re-design the framework to incorporate a new modeling scheme. Arguably, there is no free lunch when it comes to modeling the agent's behavior across various applications.
The main focus of our work here is thus to investigate and address the following questions:
- How can intention prediction be efficiently exploited and made practical in planning under partial observability? In particular, how can the bounded rationality of the other agents be explicitly modeled without incurring prohibitive computational cost?
- How can existing BRL frameworks be refined to allow a domain expert to freely incorporate his choice of design in modeling the other agents' behaviors?
This question is signficant in putting theory into practice and, when answered, can potentially bridge the gap between learning in single- and (self-interested) multi-agent systems.
PROPOSED METHODOLOGY
- To answer the first question, we first develop a novel intention-aware nested MDP framework for planning in fully observable multi-agent environments. Inspired by the cognitive hierarchy model of games, nested MDP constitutes a recursive reasoning formalism to predict the other agent's intention and then exploit it to plan our agent's optimal interaction policy. We show that nested MDP incurs linear time in the planning horizon length and reasoning depth. Then, we propose an intention-aware I-POMDP Lite framework for planning in partially observable multi-agent environments that, in particular, exploits a practical structural assumption: The intention of the other agent is driven by nested MDP, which is demonstrated theoretically to be an effective surrogate of its true intention when the agents have fine sensing and actuation capabilities. This assumption will allow the other agent's intention to be predicted efficiently and, consequently, I-POMDP Lite to be solved effectively, as demonstrated theoretically and empirically in our work.
- To tackle the second question, we present a novel generalization of BRL called Interactive BRL (I-BRL) to integrate any parametric model and model prior of the other agent's behavior specified by domain experts, thus effectively allowing the other agent's sophisticated behavior to be represented in a fine-grained manner based on the practitioners' prior knowledge. In particular, we show how the non-myopic Bayes-optimal policy can be derived analytically by solving I-BRL exactly and propose an approximation algorithm to compute it efficiently in polynomial time. Empirically, we demonstrate I-BRL's performance using an interesting traffic problem modeled after a real-world situation.
PUBLICATIONS
- New Advances on Bayesian and Decision-Theoretic Approaches for Interactive Machine Learning.
Trong Nghia Hoang.
Ph.D. Thesis, Department of Computer Science, National University of Singapore, Feb 2015.
Abstract.
The exploration-exploitation trade-off is a fundamental dilemma in many interactive learning scenarios which include both aspects of reinforcement learning (RL) and active learning (AL): An autonomous agent, situated in an unknown environment, has to actively extract knowledge from the environment by taking actions (or conducting experiments) based on its previously collected information to make accurate predictions or to optimize some utility functions. Thus, to make the most effective use of their resource-constrained budget (e.g., processing time, experimentation cost), the agent must choose carefully between (a) exploiting options (e.g., actions, experiments) which are recommended by its current, possibly incomplete model of the environment, and (b) exploring the other ostensibly sub-optimal choices to gather more information.
For example, an RL agent has to face a dilemma between (a) exploiting the most-rewarding action according to the current statistical model of the environment at the risk of running into catastrophic situations if the model is not accurate, and (b) exploring a sub-optimal action to gather more information so as to improve the model's accuracy at the potential price of losing the short-term reward. Similarly, an AL algorithm/agent has to consider between (a) conducting the most informative experiments according to its current estimation of the environment model's parameters (i.e., exploitation), and (b) running experiments that help improving the estimation accuracy of these parameters (i.e., exploration).
More often, learning strategies that ignore exploration will likely exhibit sub-optimal performance due to their imperfect knowledge while, conversely, those that entirely focus on exploration might suffer the cost of learning without benefitting from it. Therefore, a good exploration-exploitation trade-off is critical to the success of those interactive learning agents: In order to perform well, they must strike the right balance between these two conflicting objectives. Unfortunately, while this trade-off has been well-recognized since the early days of RL, the studies of exploration-exploitation have mostly been developed for theoretical settings in the respective field of RL and, perhaps surprisingly, glossed over in the existing AL literature. From a practical point of view, we see three limiting factors:
- Previous works addressing the exploration-exploitation trade-off in RL have largely focused on simple choices of the environment model and consequently, are not practical enough to accommodate real-world applications that have far more complicated environment structures. In fact, we find that most recent advances in Bayesian reinforcement learning (BRL) have only been able to analytically trade off between exploration and exploitation under a simple choice of models such as Flat-Dirichlet-Multinomial (FDM) whose independence and modeling assumptions do not hold for many real-world applications.
- Nearly all of the notable works in the AL literature primarily advocate the use of greedy/myopic algorithms whose rates of convergence (i.e., the number of experiments required by the learning algorithm to achieve a desired performance in the worst case) are provably minimax optimal for simple classes of learning tasks (e.g., threshold learning). While these results have greatly advanced our understanding about the limit of myopic AL in worst-case scenarios, significantly less is presently known about whether it is possible to devise nonmyopic AL strategies which optimize the exploration-exploitation trade-off to achieve the best expected performance in budgeted learning scenarios.
- The issue of scalability of the existing predictive models (e.g., Gaussian processes) used in AL has generally been underrated since the majority of literature considers small-scale environments which only consist of a few thousand candidate experiments to be selected by single-mode AL algorithms one at a time prior to retraining the model. In contrast, large-scale environments usually have a massive set of million candidate experiments among which tens or hundreds of thousands should be actively selected for learning. For such data-intensive problems, it is often more cost-effective to consider batch-mode AL algorithms which select and conduct multiple experiments in parallel at each stage to collect observations in batch. Retraining the predictive model after incorporating each batch of observations then becomes a computational bottleneck as the collected dataset at each stage quickly grows up to tens or even hundreds of thousand data points.
This thesis outlines some recent progresses that we have been able to make while working toward satisfactory answers to the above challenges, along with practical algorithms that achieve them:
- In particular, in order to put BRL into practice for more complicated and practical problems, we propose a novel framework called Interactive Bayesian Reinforcement Learning (I-BRL) to integrate the general class of parametric models and model priors, thus allowing the practitioners' domain knowledge to be exploited to produce a fine-grained and compact representation of the environment as often required in many real-world applications. Interestingly, we show how the nonmyopic Bayes-optimal policy can be derived analytically by solving I-BRL exactly and propose an approximation algorithm to compute it efficiently in polynomial time. Our empirical studies show that the proposed approach performs competitively with the existing state-of-the-art algorithms.
- Then, to establish a theoretical foundation for the exploration-exploitation trade-off in single-mode active learning scenarios with resource-constrained budgets, we present a novel ϵ-Bayes-optimal Decision-Theoretic Active Learning (ϵ-BAL) framework which advocates the use of differential entropy as a performance measure and consequently, derives a learning policy that can approximate the optimal expected performance arbitrarily closely (i.e., within an arbitrary loss bound ϵ). To meet the real-time requirement in time-critical applications, we then propose an asymptotically ϵ-optimal, branch-and-bound anytime algorithm based on ϵ-BAL with performance guarantees. In practice, we empirically demonstrate with both synthetic and real-world datasets that the proposed approach outperforms the state-of-the-art algorithms in budgeted scenarios.
- Lastly, to facilitate the future developments of large-scale, nonmyopic AL applications, we further introduce a highly scalable family of anytime predictive models for AL which provably converge toward a well-known class of sparse Gaussian processes (SGPs). Unlike the existing predictive models of AL which cannot be updated incrementally and are only capable of processing middle-sized datasets (i.e., a few thousands of data points), our proposed models can process massive datasets in an anytime fashion, thus providing a principled trade-off between the processing time and the predictive accuracy. The efficiency of our framework is then demonstrated empirically on a variety of large-scale real-world datasets which contains hundreds of thousand data points.
- Interactive POMDP Lite: Towards Practical Planning to Predict and Exploit Intentions for Interacting with Self-Interested Agents.
Trong Nghia Hoang & Kian Hsiang Low.
In Proceedings of the 23rd International Joint Conference on Artificial Intelligence (IJCAI-13), pages 2298-2305, Beijing, China, Aug 3-9, 2013.
13.2% acceptance rate (oral presentation)
Abstract. A key challenge in non-cooperative multi-agent systems is that of developing efficient planning algorithms for intelligent agents to interact and perform effectively among boundedly rational, self-interested agents (e.g., humans). The practicality of existing works addressing this challenge is being undermined due to either the restrictive assumptions of the other agents' behavior, the failure in accounting for their rationality, or the prohibitively expensive cost of modeling and predicting their intentions. To boost the practicality of research in this field, we investigate how intention prediction can be efficiently exploited and made practical in planning, thereby leading to efficient intention-aware planning frameworks capable of predicting the intentions of other agents and acting optimally with respect to their predicted intentions. We show that the performance losses incurred by the resulting planning policies are linearly bounded by the error of intention prediction. Empirical evaluations through a series of stochastic games demonstrate that our policies can achieve better and more robust performance than the state-of-the-art algorithms.
- A General Framework for Interacting Bayes-Optimally with Self-Interested Agents using Arbitrary Parametric Model and Model Prior.
Trong Nghia Hoang & Kian Hsiang Low.
In Proceedings of the 23rd International Joint Conference on Artificial Intelligence (IJCAI-13), pages 1394-1400, Beijing, China, Aug 3-9, 2013.
28.0% acceptance rate
Abstract. Recent advances in Bayesian reinforcement learning (BRL) have shown that Bayes-optimality is theoretically achievable by modeling the environment's latent dynamics using Flat-Dirichlet-Multinomial (FDM) prior. In self-interested multi-agent environments, the transition dynamics are mainly controlled by the other agent's stochastic behavior for which FDM's independence and modeling assumptions do not hold. As a result, FDM does not allow the other agent's behavior to be generalized across different states nor specified using prior domain knowledge. To overcome these practical limitations of FDM, we propose a generalization of BRL to integrate the general class of parametric models and model priors, thus allowing practitioners' domain knowledge to be exploited to produce a fine-grained and compact representation of the other agent's behavior. Empirical evaluation shows that our approach outperforms existing multi-agent reinforcement learning algorithms.
- Intention-Aware Planning under Uncertainty for Interacting with Self-Interested, Boundedly Rational Agents.
Trong Nghia Hoang & Kian Hsiang Low.
In Proceedings of the
11th International Conference on Autonomous Agents and MultiAgent Systems (AAMAS-12), pages 1233-1234, Valencia, Spain, June 4-8, 2012.
Abstract. A key challenge in non-cooperative multi-agent systems is that of developing efficient planning algorithms for intelligent agents to perform effectively among boundedly rational, self-interested (i.e., non-cooperative) agents (e.g., humans). To address this challenge, we investigate how intention prediction can be efficiently exploited and made practical in planning, thereby leading to efficient intention-aware planning frameworks capable of predicting the intentions of other agents and acting optimally with respect to their predicted intentions.
GAUSSIAN PROCESS DECENTRALIZED DATA FUSION & ACTIVE SENSING AGENTS FOR MOBILITY-ON-DEMAND SYSTEMSTowards Large-Scale Spatiotemporal Traffic Modeling and Prediction
PROJECT DURATION : Aug 2010 - May 2021
PROJECT AFFILIATION :
Singapore-MIT Alliance for Research and Technology (SMART) Future Urban Mobility (FM) IRG (Collaborator: Patrick Jaillet, MIT)
PROJECT FUNDING
- SMART Subaward Agreements - FM IRG :
Spatiotemporal Modeling and Prediction of Traffic Patterns,
SGD $361,456.17, Oct 2011 - Mar 2017
- Research Collaboration Agreement with Sumitomo Electric Industries, Ltd. : Estimation/Prediction Algorithm for Traffic Volume without Rich Installation of Detectors, JPY $3,000,000, Sep 2013 - Nov 2014
PROBLEM MOTIVATION
PRIVATE automobiles are becoming unsustainable personal mobility solutions in densely populated urban cities because the addition of parking and road spaces cannot keep pace with their escalating numbers due to limited urban land. For example, Hong Kong and Singapore have, respectively, experienced 27.6% and 37% increase in private vehicles from 2003 to 2011. However, their road networks have only expanded less than 10% in size. Without implementing sustainable measures, traffic congestions and delays will grow more severe and frequent, especially during peak hours.
Mobility-on-demand (MoD) systems (e.g., Velib system of over 20000 shared bicycles in Paris, experimental carsharing systems) have recently emerged as a promising paradigm of one-way vehicle sharing for sustainable personal urban mobility, specifically, to tackle the problems of low vehicle utilization rate and parking space caused by private automobiles. Conventionally, a MoD system provides stacks and racks of light electric vehicles distributed throughout a city: When a user wants to go somewhere, he simply walks to the nearest rack, swipes a card to pick up a vehicle, drives it to the rack nearest to his destination, and drops it off. In this work, we assume the capability of a MoD system to be enhanced by deploying robotic shared vehicles (e.g., General Motors Chevrolet EN-V 2.0 prototype) that can autonomously drive and cruise the streets of a densely populated urban city to be hailed by users (like taxis) instead of just waiting at the racks to be picked up. Compared to the conventional MoD system, the fleet of autonomous robotic vehicles provides greater accessibility to users who can be picked up and dropped off at any location in the road network. As a result, it can service regions of high mobility demand but with poor coverage of stacks and racks due to limited space for their installation.
The key factors in the success of a MoD system are the costs to the users and system latencies, which can be minimized by managing the MoD system effectively. To achieve this, two main technical challenges need to be addressed: (a) Real-time, fine-grained mobility demand sensing and prediction, and (b) real-time active fleet management to balance vehicle supply and demand and satisfy latency requirements at sustainable operating costs. Existing works on load balancing in MoD systems, dynamic traffic assignment problems, dynamic one-to-one pickup and delivery problems, and location recommendation and dispatch for cruising taxis have tackled variants of the second challenge by assuming the necessary inputs of mobility demand and traffic flow information to be perfectly or accurately known using prior knowledge or offline processing of historic data. Such an assumption does not hold for densely populated urban cities because their mobility demand patterns and traffic flow are often subject to short-term random fluctuations and perturbations due to frequent special events (e.g., storewide sales, exhibitions), unpredictable weather conditions, unforeseen emergencies (e.g., breakdowns in public transport services), or traffic incidents (e.g., accidents, vehicle breakdowns, roadworks). So, in order for the active fleet management strategies to perform well in fleet rebalancing and route planning to service the mobility demands, they require accurate, fine-grained predictive information of the spatiotemporally varying mobility demand patterns and traffic flow in real time, the former of which is the desired outcome of addressing the first challenge. To the best of our knowledge, there is little progress in the algorithm design and development to take on the first challenge, which will be a focus of our work here.
In practice, it is non-trivial to achieve real-time, accurate prediction of spatiotemporally varying traffic phenomena such as mobility demand patterns and traffic flow because the quantity of sensors that can be deployed to observe an entire road network is cost-constrained. For example, static sensors such as loop detectors are traditionally placed at designated locations in a road network to collect data for predicting the traffic flow. However, they provide sparse coverage (i.e., many road segments are not observed, thus leading to data sparsity), incur high installation and maintenance costs, and cannot reposition by themselves in response to changes in the traffic flow. Low-cost GPS technology allows the collection of traffic flow data using passive mobile probes (e.g., taxis/cabs). Unlike static sensors, they can directly measure the travel times along road segments. But, they provide fairly sparse coverage due to low GPS sampling frequency (i.e., often imposed by taxi/cab companies) and no control over their routes, incur high initial implementation cost, pose privacy issues, and produce highly-varying speeds and travel times while traversing the same road segment due to inconsistent driving behaviors. A critical mass of probes is needed on each road segment to ease the severity of the last drawback but is often hard to achieve on non-highway segments due to sparse coverage. In contrast, we propose using the autonomous robotic vehicles as active mobile probes to overcome the limitations of static and passive mobile probes. In particular, they can be directed to explore any segments of a road network to gather real-time mobility demand data (e.g., pickup counts of different regions) and traffic flow data (e.g., speeds and travel times along road segments) at a desired GPS sampling rate while enforcing consistent driving behavior.
How then can the vacant autonomous robotic vehicles in a MoD system actively cruise a road network to gather and assimilate the most informative data for predicting a spatiotemporally varying traffic phenomenon like a mobility demand pattern or traffic flow? To solve this problem, a centralized approach to data fusion and active sensing is poorly suited because it suffers from a single point of failure and incurs huge communication, space, and time overheads with large data and fleet.
PROPOSED METHODOLOGY
Our work proposes novel decentralized data fusion and active sensing algorithms for real-time, fine-grained traffic sensing, modeling, and prediction with a fleet of autonomous robotic vehicles in a MoD system. Note that the decentralized data fusion component of our proposed algorithms can also be used for static sensors and passive mobile probes.
The specific contributions of our work here include:
- Modeling and predicting a mobility demand pattern and traffic flow using, respectively, rich classes of Bayesian nonparametric models called a log-Gaussian process (lGP) model and a relational Gaussian process model, the latter of whose spatiotemporal correlation structure can exploit both the road segment features and road network topology information;
- Developing novel Gaussian process decentralized data fusion algorithms for cooperative perception of traffic phenomena called GP-DDF and GP-DDF+ whose predictive performance are theoretically guaranteed to be equivalent to that of sophisticated centralized sparse approximations of the full-rank Gaussian process (full GP) model: The computation of such sparse approximate GP models can thus be distributed among the MoD vehicles, thereby achieving efficient and scalable probabilistic prediction;
- Deriving consensus filtering variants of GP-DDF and GP-DDF+ that require only local communication between neighboring MoD vehicles instead of assuming all-to-all communication between MoD vehicles;
- Devising decentralized active sensing algorithms (a) whose performance, when coupled with GP-DDF, can be theoretically guaranteed to realize the effect of the spatiotemporal correlation structure of the traffic phenomenon and various parameter settings of the MoD system, and (b) that, when used for sampling a mobility demand pattern, can be analytically shown to exhibit, interestingly, a cruising behavior of simultaneously exploring demand hotspots and sparsely sampled regions that have higher likelihood of picking up users, hence achieving a dual effect of fleet rebalancing to service the mobility demands;
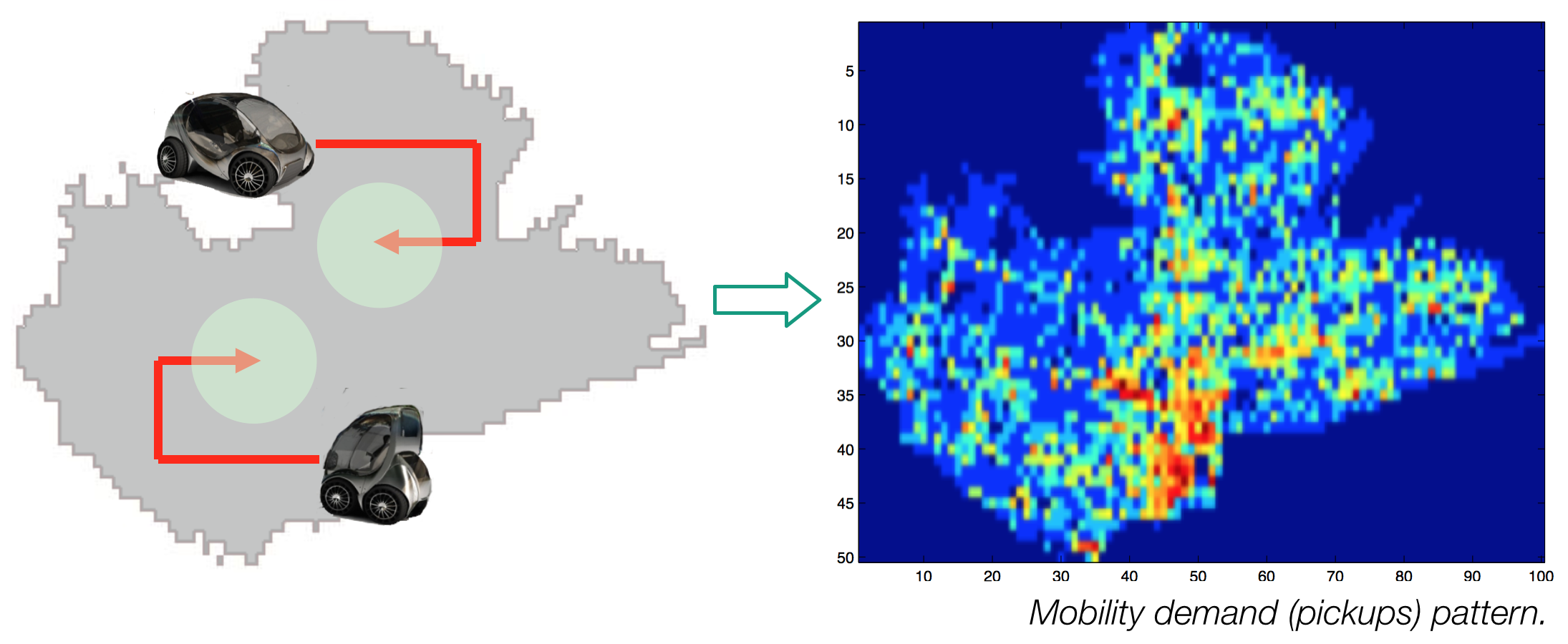
- Analyzing the time and communication overheads of our proposed algorithms: We prove that our algorithms can scale better than existing state-of-the-art centralized algorithms in the size of the data and fleet;
- Empirically evaluating the predictive accuracy, time efficiency, scalability, and performance of servicing mobility demands (i.e., average cruising length of vehicles, average waiting time of users, total number of pickups) of our proposed algorithms on two datasets featuring real-world traffic phenomena such as a mobility demand pattern over the central business district of Singapore and speeds of road segments over an urban road network in Singapore.
PUBLICATIONS
- Collective Online Learning of Gaussian Processes in Massive Multi-Agent Systems.
Trong Nghia Hoang, Quang Minh Hoang, Kian Hsiang Low & Jonathan P. How.
In Proceedings of the 33rd AAAI Conference on Artificial Intelligence (AAAI-19), pages 7850-7857, Honolulu, Hawaii, Jan 27-Feb 1, 2019.
16.2% acceptance rate
Abstract. This paper presents a novel Collective Online Learning of Gaussian Processes (COOL-GP) framework for enabling a massive number of GP inference agents to simultaneously perform (a) efficient online updates of their GP models using their local streaming data with varying correlation structures and (b) decentralized fusion of their resulting online GP models with different learned hyperparameter settings and inducing inputs. To realize this, we exploit the notion of a common encoding structure to encapsulate the local streaming data gathered by any GP inference agent into summary statistics based on our proposed representation, which is amenable to both an efficient online update via an importance sampling trick as well as multi-agent model fusion via decentralized message passing that can exploit sparse connectivity among agents for improving efficiency and enhance the robustness of our framework against transmission loss. We provide a rigorous theoretical analysis of the approximation loss arising from our proposed representation to achieve efficient online updates and model fusion. Empirical evaluations show that COOL-GP is highly effective in model fusion, resilient to information disparity between agents, robust to transmission loss, and can scale to thousands of agents.
- Gaussian Process Decentralized Data Fusion Meets Transfer Learning in Large-Scale Distributed Cooperative Perception.
Ruofei Ouyang & Kian Hsiang Low.
Autonomous Robots (Special Issue on Multi-Robot and Multi-Agent Systems), volume 44, issue 3, pages 359-376, Mar 2020.
Extended version of our
AAAI-18 paper
Abstract. This paper presents novel Gaussian process decentralized data fusion algorithms exploiting the notion of agent-centric support sets for distributed cooperative perception of large-scale environmental phenomena. To overcome the limitations of scale in existing works, our proposed algorithms allow every mobile sensing agent to utilize a different support set and dynamically switch to another during execution for encapsulating its own data into a local summary that, perhaps surprisingly, can still be assimilated with the other agents' local summaries (i.e., based on their current choices of support sets) into a globally consistent summary to be used for predicting the phenomenon. To achieve this, we propose a novel transfer learning mechanism for a team of agents capable of sharing and transferring information encapsulated in a summary based on a support set to that utilizing a different support set with some loss that can be theoretically bounded and analyzed. To alleviate the issue of information loss accumulating over multiple instances of transfer learning, we propose a new information sharing mechanism to be incorporated into our algorithms in order to achieve memory-efficient lazy transfer learning. Empirical evaluation on three real-world datasets for up to 128 agents show that our algorithms outperform the state-of-the-art methods.
- Gaussian Process Decentralized Data Fusion Meets Transfer Learning in Large-Scale Distributed Cooperative Perception.
Ruofei Ouyang & Kian Hsiang Low.
In Proceedings of the 32nd AAAI Conference on Artificial Intelligence (AAAI-18), pages 3876-3883, New Orleans, LA, Feb 2-8, 2018.
24.55% acceptance rate
Abstract. This paper presents novel Gaussian process decentralized data fusion algorithms exploiting the notion of agent-centric support sets for distributed cooperative perception of large-scale environmental phenomena. To overcome the limitations of scale in existing works, our proposed algorithms allow every mobile sensing agent to choose a different support set and dynamically switch to another during execution for encapsulating its own data into a local summary that, perhaps surprisingly, can still be assimilated with the other agents' local summaries (i.e., based on their current choices of support sets) into a globally consistent summary to be used for predicting the phenomenon. To achieve this, we propose a novel transfer learning mechanism for a team of agents capable of sharing and transferring information encapsulated in a summary based on a support set to that utilizing a different support set with some loss that can be theoretically bounded and analyzed. To alleviate the issue of information loss accumulating over multiple instances of transfer learning, we propose a new information sharing mechanism to be incorporated into our algorithms in order to achieve memory-efficient lazy transfer learning. Empirical evaluation on real-world datasets show that our algorithms outperform the state-of-the-art methods.
- Exploiting Decentralized Multi-Agent Coordination for Large-Scale Machine Learning Problems.
Ruofei Ouyang.
Ph.D. Thesis, Department of Computer Science, National University of Singapore, Dec 2016.
Abstract.
Nowadays, the scale of machine learning problems becomes much larger than before. It raises a huge demand in distributed perception and distributed computation. A multi-agent system provides exceptional scalability for problems like active sensing and data fusion. However, many rich characteristics of large-scale machine learning problems have not been addressed yet such as large input domain, nonstationarity, and high dimensionality. This thesis identifies the challenges related to these characteristics from multi-agent perspective. By exploiting the correlation structure of data in large-scale problems, we propose multi-agent coordination schemes that can improve the scalability of the machine learning models while preserving the computation accuracy. To elaborate, the machine learning problems we are solving with multi-agent coordination techniques are:
- Gaussian process regression. To perform distributed regression on a large-scale environmental phenomenon, data compression is often required due to the communication costs. Currently, decentralized data fusion methods encapsulate the data into local summaries based on a fixed support set. However in a large-scale field, this fixed support set, acting as a centralized component in the decentralized system, cannot approximate the correlation structure of the entire phenomenon well. It leads to evident losses in data summarization. Consequently, the regression performance will be significantly reduced.
In order to approximate the correlation structure accurately, we propose an agent-centric support set to allow every agent in the data fusion system to choose a possibly different support set and dynamically switch to another one during execution for encapsulating its own data into a local summary which, perhaps surprisingly, can still be assimilated with the other agents’ local summaries into a globally consistent summary. Together with an information sharing mechanism we designed, the new decentralized data fusion methods with agent-centric support set can be applied to regression problems on a much larger environmental phenomenon with high performance.
- Active learning. In the context of environmental sensing, active learning/active sensing is a process of taking observations to minimize the uncertainty in an environmental field. The uncertainty is quantified based on the correlation structure of the phenomenon which is traditionally assumed to be stationary for computational sake. In a large-scale environmental field, this stationary assumption is often violated. Therefore, existing active sensing algorithms perform sub-optimally for a nonstationary environmental phenomenon.
To the best of our knowledge, our decentralized multi-robot active sensing (DEC-MAS) algorithm is the first work to address nonstationarity issue in the context of active sensing. The uncertainty in the phenomenon is quantified based on the nonstationary correlation structure estimated by Dirichlet process mixture of Gaussian processes. Further, our DEC-MAS algorithm can efficiently coordinate the exploration of multiple robots to automatically trade-off between learning the unknown, nonstationary correlation structure and minimizing the uncertainty of the environmental phenomenon. It enables multi-agent active sensing techniques to be applied to a large-scale nonstationary environmental phenomenon.
- Bayesian optimization. Optimizing an unknown objective function is challenging for traditional optimization methods. Alternatively, in this situation, people use Bayesian optimization which is a modern optimization technique that can optimize a function by only utilizing the observation information (input and output values) collected through simulations. When the input dimension of the function is low, a few simulated observations can generate good result already. However, for high dimensional function, a huge number of observations are required which is impractical when the simulation consumes lots of time and resources.
Fortunately, many high dimensional problems have sparse correlation structure. Our ANOVA-DCOP work can decompose the correlation structure in the original high-dimensional problem into many correlation structures of subsets of dimensions based on ANOVA kernel function. It significantly reduces the size of input space into a collection of lower-dimensional subspaces. Additionally, we reformulate the Bayesian optimization problem as a decentralized constrained optimization problem (DCOP) that can be efficiently solved by multi-agent coordination techniques so that it can scale up to problems with hundreds of dimensions.
- Gaussian Process Decentralized Data Fusion and Active Sensing for Spatiotemporal Traffic Modeling and Prediction in Mobility-on-Demand Systems.
Jie Chen, Kian Hsiang Low, Patrick Jaillet & Yujian Yao.
IEEE Transactions on Automation Science and Engineering
(Special Issue on Networked Cooperative Autonomous Systems), volume 12, issue 3, pages 901-921, Jul 2015.
Extended version of our UAI-12 and
RSS-13 papers
Abstract. Mobility-on-demand (MoD) systems have recently emerged as a
promising paradigm of one-way vehicle sharing for sustainable personal
urban mobility in densely populated cities. We assume the capability of
a MoD system to be enhanced by deploying robotic shared vehicles that
can autonomously cruise the streets to be hailed by users. A key
challenge of the MoD system is that of real-time, fine-grained mobility
demand and traffic flow sensing and prediction. This paper presents
novel Gaussian process (GP) decentralized data fusion and active
sensing algorithms for real-time, fine-grained traffic modeling and
prediction with a fleet of MoD vehicles. The predictive performance of
our decentralized data fusion algorithms are theoretically guaranteed to
be equivalent to that of sophisticated centralized sparse GP
approximations. We derive consensus filtering variants requiring only
local communication between neighboring vehicles. We theoretically
guarantee the performance of our decentralized active sensing
algorithms. When they are used to gather informative data for mobility
demand prediction, they can achieve a dual effect of fleet rebalancing
to service mobility demands. Empirical evaluation on real-world datasets
shows that our algorithms are significantly more time-efficient and
scalable in the size of data and fleet while achieving predictive
performance comparable to that of state-of-the-art algorithms.
- Gaussian Process-Based Decentralized Data Fusion and Active Sensing Agents: Towards Large-Scale Modeling and Prediction of Spatiotemporal Traffic Phenomena.
Jie Chen.
Ph.D. Thesis, Department of Computer Science, National University of Singapore, Dec 2013.
Abstract.
Knowing and understanding the environmental phenomena is important to many real world applications. This thesis is devoted to study large-scale modeling and prediction of spatiotemporal environmental phenomena (i.e., urban traffic phenomena). Towards this goal, our proposed approaches rely on a class of Bayesian non-parametric models: Gaussian processes (GP).
To accurately model spatiotemporal urban traffic phenomena in real world situation, a novel relational GP taking into account both the road segment features and road network topology information is proposed to model real world traffic conditions over road network. Additionally, a GP variant called log-Gaussian process (lGP) is exploited to model an urban mobility demand pattern which contains skewness and extremity in demand measurements.
To achieve efficient and scalable urban traffic phenomenon prediction given a large phenomenon data, we propose three novel parallel GPs: parallel partially independent training conditional (pPITC), parallel partially independent conditional(pPIC) and parallel incomplete Cholesky factorization (pICF)-based approximations of GP model, which can distribute their computational load into a cluster of parallel/multi-core machines, thereby achieving time efficiency. The predictive performances of such parallel GPs are theoretically guaranteed to be equivalent to that of some centralized approaches to approximate full/exact GP regression. The proposed parallel GPs are implemented using the message passing interface (MPI) framework and tested on two large real world datasets. The theoretical and empirical results show that our parallel GPs achieve significantly better time efficiency and scalability than that of full GP, while achieving comparable accuracy. They also achieve fine speedup performance that is the ratio of time required by the parallel algorithms and their centralized counterparts.
To exploit active mobile sensors to perform decentralized perception of the spatiotemporal urban traffic phenomenon, we propose a decentralized algorithm framework: Gaussian process-based decentralized data fusion and active sensing (D2FAS) which is composed of a decentralized data fusion (DDF) component and a decentralized active sensing (DAS) component. The DDF component includes a novel Gaussian process-based decentralized data fusion (GP-DDF) algorithm that can achieve remarkably efficient and scalable prediction of phenomenon and a novel Gaussian process-based decentralized data fusion with local augmentation (GP-DDF+) algorithm that can achieve better predictive accuracy while preserving time efficiency of GP-DDF. The predictive performances of both GP-DDF and GP-DDF+ are theoretically guaranteed to be equivalent to that of some sophisticated centralized sparse approximations of exact/full GP. For the DAS component, we propose a novel partially decentralized active sensing (PDAS) algorithm that exploits property in correlation structure of GP-DDF to enable mobile sensors cooperatively gathering traffic phenomenon data along a near-optimal joint walk with theoretical guarantee, and a fully decentralized active sensing (FDAS) algorithm that guides each mobile sensor gather phenomenon data along its locally optimal walk.
Lastly, to justify the practicality of the D2FAS framework, we develop and test D2FAS algorithms running with active mobile sensors on real world datasets for monitoring traffic conditions and sensing/servicing urban mobility demands. Theoretical and empirical results show that the proposed algorithms are significantly more time-efficient, more scalable in the size of data and in the number of sensors than the state-of-the-art centralized approaches, while achieving comparable predictive accuracy.
- Gaussian Process-Based Decentralized Data Fusion and Active Sensing for Mobility-on-Demand System.
Jie Chen, Kian Hsiang Low & Colin Keng-Yan Tan.
In Proceedings of the
Robotics: Science and Systems Conference (RSS-13), Berlin, Germany, Jun 24-28, 2013.
30.1% acceptance rate
Abstract. Mobility-on-demand (MoD) systems have recently emerged as a promising paradigm of one-way vehicle sharing for sustainable personal urban mobility in densely populated cities. In this paper, we enhance the capability of a MoD system by deploying robotic shared vehicles that can autonomously cruise the streets to be hailed by users. A key challenge to managing the MoD system effectively is that of real-time, fine-grained mobility demand sensing and prediction. This paper presents a novel decentralized data fusion and active sensing algorithm for real-time, fine-grained mobility demand sensing and prediction with a fleet of autonomous robotic vehicles in a MoD system. Our Gaussian process (GP)-based decentralized data fusion algorithm can achieve a fine balance between predictive power and time efficiency. We theoretically guarantee its predictive performance to be equivalent to that of a sophisticated centralized sparse approximation for the GP model: The computation of such a sparse approximate GP model can thus be distributed among the MoD vehicles, hence achieving efficient and scalable demand prediction. Though our decentralized active sensing strategy is devised to gather the most informative demand data for demand prediction, it can achieve a dual effect of fleet rebalancing to service the mobility demands. Empirical evaluation on real-world mobility demand data shows that our proposed algorithm can achieve a better balance between predictive accuracy and time efficiency than state-of-the-art algorithms.
- Decentralized Data Fusion and Active Sensing with Mobile Sensors for Modeling and Predicting Spatiotemporal Traffic Phenomena.
Jie Chen, Kian Hsiang Low, Colin Keng-Yan Tan, Ali Oran, Patrick Jaillet, John M. Dolan & Gaurav S. Sukhatme.
In Proceedings of the 28th Conference on Uncertainty in Artificial Intelligence (UAI-12), pages 163-173, Catalina Island, CA, Aug 15-17, 2012.
31.6% acceptance rate
Also appeared in AAMAS-12 Workshop on Agents in Traffic and Transportation (ATT-12), Valencia, Spain, June 4-8, 2012.
Abstract. The problem of modeling and predicting spatiotemporal traffic phenomena over an urban road network is important to many traffic applications such as detecting and forecasting congestion hotspots. This paper presents a decentralized data fusion and active sensing (D2FAS) algorithm for mobile sensors to actively explore the road network to gather and assimilate the most informative data for predicting the traffic phenomenon. We analyze the time and communication complexity of D2FAS and demonstrate that it can scale well with a large number of observations and sensors. We provide a theoretical guarantee on its predictive performance to be equivalent to that of a sophisticated centralized sparse approximation for the Gaussian process (GP) model: The computation of such a sparse approximate GP model can thus be parallelized and distributed among the mobile sensors (in a Google-like MapReduce paradigm), thereby achieving efficient and scalable prediction. We also theoretically guarantee its active sensing performance that improves under various practical environmental conditions. Empirical evaluation on real-world urban road network data shows that our D2FAS algorithm is significantly more time-efficient and scalable than state-of-the-art centralized algorithms while achieving comparable predictive performance.
PRESENTATIONS
- Gaussian Process-Based Decentralized Data Fusion
and Active Sensing Agents:
Towards Large-Scale Modeling & Prediction of Spatiotemporal Traffic Phenomena.
Kian Hsiang Low.
Invited speaker at the RSS-13 Workshop on Robotic Exploration, Monitoring, and Information Collection: Nonparametric Modeling, Information-based Control, and Planning under Uncertainty, Berlin, Germany, Jun 27-28, 2013.
PLANNING UNDER UNCERTAINTY FOR LARGE-SCALE ACTIVE MULTI-CAMERA SURVEILLANCE
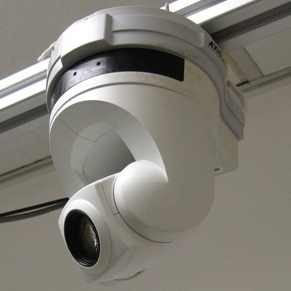
PROJECT DURATION : Mar 2010 - Nov 2014
PROJECT AFFILIATION :
Sensor-Enhanced Social Media (SeSaMe) Centre (Collaborator: Mohan Kankanhalli)
MEDIA NEWS :
TODAY's Science Section (15 May 2015) - 'When CCTV cameras work together as one'
PROBLEM MOTIVATION
The problem of surveillance has grown to be a critical concern in many urban cities worldwide following a recent series of security threats like Mumbai terrorist attacks and London bomb blasts. Central to the problem of surveillance is that of monitoring, tracking, and observing multiple mobile targets of interest distributed over a large-scale obstacle-ridden environment (e.g., airport terminals, railway and subway stations, bus depots, shopping malls, school campuses, military bases). It is often necessary to acquire high-resolution videos/images of these targets for supporting real-world surveillance applications like activity/intention tracking and recognition, biometric analysis like target identification and face recognition, surveillance video mining, forensic video analysis/retrieval, among others.
Traditional surveillance systems consist of a large network of fixed/static CCTV (Closed Circuit Television) cameras that are placed to constantly focus at selected important locations in the buildings like entrance/exit, lobby, etc. Unfortunately, the maximum resolution of these cameras is limited to 720 x 480 pixels. So, they cannot capture high-resolution images/videos of the targets, especially when the targets are far away from the cameras. As a result, they perform poorly in acquiring the close-up views of the targets and their activities. HDTV/Megapixel cameras have recently been introduced to overcome this resolution issue. Similar to CCTV cameras, these fixed/static HDTV/megapixel cameras are placed to constantly focus at specific locations in the environment. A relatively large network of such cameras has to be installed in order to observe the targets in any region of the environment at high resolution, which is impractical in terms of equipment, installation, and maintenance costs.
The use of active PTZ (Pan/Tilt/Zoom) cameras is becoming an increasingly popular alternative to that of fixed/static cameras for surveillance because the active cameras are endowed with pan-tilt-zoom capabilities that can be exploited to focus on and observe the targets at high image/video resolution. Hence, fewer active cameras need to be deployed to be able to capture high-resolution images/videos of the targets in any region of the environment. In order to achieve effective real-time surveillance, an efficient automated mechanism is required to autonomously coordinate and control these cameras' actions.
The objective of this work is thus to address the following central surveillance problem: "How can a network of active cameras be coordinated and controlled to maximize the number of targets observed with a guaranteed image resolution?"
PROPOSED METHODOLOGY
This work presents a novel principled decision-theoretic planning under uncertainty approach to coordinating and controlling a large-scale network of active cameras for performing high-quality surveillance of large crowds of moving targets. In particular, our approach addresses the following practical issues affecting the surveillance problem:
(a) Multiple sources of uncertainty. A typical surveillance environment is fraught with multiple sources of uncertainty such as noisy cameras' observations, stochastic targets' motion, and unknown targets' locations, etc. These uncertainties make it difficult for the active cameras to know where to observe in order to keep the targets within their fields of view (fov). Consequently, they may lose track of the observed targets. To resolve this, our approach models a belief over the targets' states (i.e., locations, directions, and velocities) and updates the belief in a Bayesian paradigm based on probabilistic models of the targets' motion and the active cameras' observations;
(b) Camera-target ratio. In crowded environments, the number of targets to be observed is usually much greater than the number of available cameras. When the number of targets increases, a surveillance system, if poorly designed, tends to incur exponentially increasing computation time, which degrades the real-time performance of the entire surveillance system;
(c) Trade-off between maximizing the expected number of observed targets and the image resolution of observing them. Increasing the resolution of observing some targets through panning, tilting, or zooming may result in the loss of other targets being tracked. To address this trade-off, the cameras' actions are coordinated to simultaneously improve the belief over the targets' states and maximize the expected number of targets observed with a guaranteed pre-defined resolution;
(d) Scalability. By exploiting the inherent structure of the surveillance problem, our approach can scale linearly in the number of targets to be observed;
(e) Real-time requirement. The cameras' actions are computed in real time;
(f) Occlusions. Many real-world surveillance environments contain obstacles that occlude the fov of some or perhaps even all of the cameras, thus preventing the cameras from persistently tracking their observed targets. The regions where the targets cannot be observed by any of the cameras due to obstacles are said to be occluded. When the targets reside in these occluded regions or are not within the fov of any camera, the surveillance system loses track of them, thus degrading the surveillance performance. Such environments are called partially observable in the sense that the exact locations of the targets may not be observed directly by the cameras at all times.
As demonstrated empirically through simulations, our approach can achieve high-quality surveillance of a large number of targets in real time and its surveillance performance degrades gracefully with an increasing number of targets. The real-world experiments show the practicality of our decision-theoretic approach to coordinate and control cameras in surveillance systems.
PUBLICATIONS
- Scalable Decision-Theoretic Coordination and Control for Real-time Active Multi-Camera Surveillance.
Prabhu Natarajan, Trong Nghia Hoang, Yongkang Wong, Kian Hsiang Low & Mohan Kankanhalli.
In Proceedings of the
8th ACM/IEEE International Conference on Distributed Smart Cameras (ICDSC'14) (Invited Paper to Special Session on Smart Cameras for Smart Environments), pages 115-120, Venezia, Italy, Nov 4-7, 2014.
Abstract. This paper presents an overview of our novel decision-theoretic multi-agent approach for controlling and coordinating multiple active cameras in surveillance. In this approach, a surveillance task is modeled as a stochastic optimization problem, where the active cameras are controlled and coordinated to achieve the desired surveillance goal in presence of uncertainties. We enumerate the practical issues in active camera surveillance and discuss how these issues are addressed in our decision-theoretic approach. We focus on two novel surveillance tasks: maximize the number of targets observed in active cameras with guaranteed image resolution and to improve the fairness in observation of multiple targets. We discuss the overview of our novel decision-theoretic frameworks: Markov decision process and partially observable Markov decision process frameworks for coordinating active cameras in uncertain and partially occluded environments.
- No One is Left "Unwatched": Fairness in Observation of Crowds of Mobile Targets in Active Camera Surveillance.
Prabhu Natarajan, Kian Hsiang Low & Mohan Kankanhalli.
In Proceedings of the
21st European Conference on Artificial Intelligence (ECAI-14), including Prestigious Applications of Intelligent Systems (PAIS-14), pages 1155-1160, Prague, Czech Republic, Aug 18-22, 2014.
Abstract. Central to the problem of active multi-camera surveillance is the fundamental issue of fairness in the observation of crowds of targets such that no target is "starved" of observation by the cameras for a long time. This paper presents a principled decision-theoretic multi-camera coordination and control (MC2) algorithm called fair-MC2 that can coordinate and control the active cameras to achieve max-min fairness in the observation of crowds of targets moving stochastically. Our fair-MC2 algorithm is novel in demonstrating how (a) the uncertainty in the locations, directions, speeds, and observation times of the targets arising from the stochasticity of their motion can be modeled probabilistically, (b) the notion of fairness in observing targets can be formally realized in the domain of multi-camera surveillance for the first time by exploiting the max-min fairness metric to formalize our surveillance objective, that is, to maximize the expected minimum observation time over all targets while guaranteeing a predefined image resolution of observing them, and (c) a structural assumption in the state transition dynamics of a surveillance environment can be exploited to improve its scalability to linear time in the number of targets to be observed during surveillance. Empirical evaluation through extensive simulations in realistic surveillance environments shows that fair-MC2 outperforms the state-of-the-art and baseline MC2 algorithms. We have also demonstrated the feasibility of deploying our fair-MC2 algorithm on real AXIS 214 PTZ cameras.
- Decision-Theoretic Approach to Maximizing Fairness in Multi-Target Observation in Multi-Camera Surveillance.
Prabhu Natarajan, Kian Hsiang Low & Mohan Kankanhalli.
In Proceedings of the
13th International Conference on Autonomous Agents and MultiAgent Systems (AAMAS-14), pages 1521-1522, Paris, France, May 5-9, 2014.
Abstract. Central to the problem of active multi-camera surveillance is the fundamental issue of fairness in the observation of multiple targets such that no target is left unobserved by the cameras for a long time. To address this important issue, we propose a novel principled decision-theoretic approach to control and coordinate multiple active cameras to achieve fairness in the observation of multiple moving targets.
- A Decision-Theoretic Approach for Controlling and Coordinating Multiple Active Cameras in Surveillance.
Prabhu Natarajan.
Ph.D. Thesis, Department of Computer Science, National University of Singapore, Dec 2013.
Abstract.
The use of active cameras in surveillance is becoming increasingly popular as they try to meet the demands of capturing high-resolution images/videos of targets in surveillance for face recognition, target identification, forensic video analysis, etc. These active cameras are endowed with pan, tilt, and zoom capabilities, which can be exploited to provide high-quality surveillance. In order to achieve effective, real-time surveillance, an efficient collaborative mechanism is needed to control and coordinate these cameras' actions, which is the focus of this thesis. The central problem in surveillance is to monitor a set of targets with guaranteed image resolution. Controlling and coordinating multiple active cameras to achieve this surveillance task is non-trivial and challenging because: (a) presence of inherent uncertainties in the surveillance environment (targets motion, location, and noisy camera observation); (b) there exists a non-trivial trade-off between number of targets and the resolution of observing these targets; and (c) more importantly, the coordination framework should be scalable with increasing number of targets and cameras.
In this thesis, we formulate a novel decision-theoretic multi-agent planning approach for controlling and coordinating multiple active cameras in surveillance. Our decision-theoretic approach offers advantages of (a) accounting the uncertainties using probabilistic models; (b) the non-trivial trade-off is addressed by coordinating the active cameras' actions to maximize the number of targets with guaranteed resolution; and (c) the scalability in number of targets and cameras is achieved by exploiting the structures and properties that are present in our surveillance problem. We focus on two novel problems in active camera surveillance: (a) maximizing observations of multiple targets (MOMT), i.e., maximizing the number of targets observed in active cameras with guaranteed image resolution; and (b) improving fairness in observation of multiple targets (FOMT), i.e., no target is "starved" of observation by active cameras for long duration of time.
We propose two formal decision-theoretic frameworks (a) Markov Decision Process (MDP) and (b) Partially Observable Markov Decision Process (POMDP) frameworks for coordinating active cameras in surveillance. MDP framework controls active cameras in fully observable surveillance environments where the active cameras are supported by one or more wide-view static/fixed cameras to observe the entire surveillance environment at low-resolution. POMDP framework controls active cameras in partially observable surveillance environments where it is impractical to observe the entire surveillance environment using static/fixed cameras due to occlusions caused by physical infrastructures. Hence the POMDP framework do not have a complete view of the surveillance environment.
Specifically, we propose (a) MDP frameworks to solve MOMT problem and FOMT problem in fully observable surveillance environment; and (b) POMDP framework to solve MOMT problem in partially observable surveillance environment. As proven analytically, our MDP and POMDP frameworks incurs time that is linear in number of targets to be observed during surveillance. We have used max-plus algorithm with our MDP framework to improve its scalability in number of cameras for MOMT problem. Empirical evaluation through simulations in realistic surveillance environment reveals that our proposed approach can achieve high-quality surveillance in real time. We also demonstrate our pro- posed approach with real Axis 214 PTZ cameras to show the practicality of our approach in real world surveillance. Both the simulations and real camera experiments show that our decision-theoretic approach can control and coordinate active cameras efficiently and hence contributes significantly towards improving the active camera surveillance research.
- Decision-Theoretic Coordination and Control for Active Multi-Camera Surveillance in Uncertain, Partially Observable Environments.
Prabhu Natarajan, Trong Nghia Hoang, Kian Hsiang Low & Mohan Kankanhalli.
In Proceedings of the
6th ACM/IEEE International Conference on Distributed Smart Cameras (ICDSC'12), pages 1-6, Hong Kong, Oct 30 - Nov 2, 2012.
Abstract. A central problem of surveillance is to monitor multiple targets moving in a large-scale, obstacle-ridden environment with occlusions. This paper presents a novel principled Partially Observable Markov Decision Process-based approach to coordinating and controlling a network of active cameras for tracking and observing multiple mobile targets at high resolution in such surveillance environments. Our proposed approach is capable of (a) maintaining a belief over the targets' states (i.e., locations, directions, and velocities) to track them, even when they may not be observed directly by the cameras at all times, (b) coordinating the cameras' actions to simultaneously improve the belief over the targets' states and maximize the expected number of targets observed with a guaranteed resolution, and (c) exploiting the inherent structure of our surveillance problem to improve its scalability (i.e., linear time) in the number of targets to be observed. Quantitative comparisons with state-of-the-art multi-camera coordination and control techniques show that our approach can achieve higher surveillance quality in real time. The practical feasibility of our approach is also demonstrated using real AXIS 214 PTZ cameras.
- PhD Forum: Decision-Theoretic Coordination and Control for Active Multi-Camera Surveillance.
Prabhu Natarajan.
In Proceedings of the
6th ACM/IEEE International Conference on Distributed Smart Cameras (ICDSC'12), pages 1-2, Hong Kong, Oct 30 - Nov 2, 2012.
Best PhD Forum Paper Award
Abstract. In this thesis, we present novel decision-theoretic
multi-agent approaches for controlling and coordinating multiple
active cameras in surveillance. Decision-theoretic approaches
models the interaction between active camera network and the
uncertain surveillance environment effectively. The goal of the
surveillance is to maximize the number of targets observed in
active cameras with guaranteed image resolution. We enumerate
the practical issues in active camera surveillance and discuss how
these issues are addressed in our decision-theoretic approaches.
The existing camera control approaches have serious limitations
in terms of scalability in number of targets. Where as in
our approaches, the scalability in number of targets has been
improved by exploiting the structure and properties that are
present in our surveillance problem. We proposed two novel
decision-theoretic frameworks: Markov Decision Process (MDP)
and Partially Observable Markov Decision Process (POMDP)
frameworks for coordinating active cameras in fully observable
and partially observable surveillance settings.
- Decision-Theoretic Approach to Maximizing Observation of Multiple Targets in Multi-Camera Surveillance.
Prabhu Natarajan, Trong Nghia Hoang, Kian Hsiang Low & Mohan Kankanhalli.
In Proceedings of the
11th International Conference on Autonomous Agents and MultiAgent Systems (AAMAS-12), pages 155-162, Valencia, Spain, June 4-8, 2012.
20.4% acceptance rate
Abstract. This paper presents a novel decision-theoretic approach to control and coordinate multiple active cameras for observing a number of moving targets in a surveillance system. This approach offers the advantages of being able to (a) account for the stochasticity of targets' motion via probabilistic modeling, and (b) address the trade-off between maximizing the expected number of observed targets and the resolution of the observed targets through stochastic optimization. One of the key issues faced by existing approaches in multi-camera surveillance is that of scalability with increasing number of targets. We show how its scalability can be improved by exploiting the problem structure: as proven analytically, our decision-theoretic approach incurs time that is linear in the number of targets to be observed during surveillance. As demonstrated empirically through simulations, our proposed approach can achieve high-quality surveillance of up to 50 targets in real time and its surveillance performance degrades gracefully with increasing number of targets. We also demonstrate our proposed approach with real AXIS 214 PTZ cameras in maximizing the number of Lego robots observed at high resolution over a surveyed rectangular area. The results are promising and clearly show the feasibility of our decision-theoretic approach in controlling and coordinating the active cameras in real surveillance system.
- Decision-Theoretic Approach for Controlling and
Coordinating Multiple Active Cameras in Surveillance.
Prabhu Natarajan.
In Proceedings of the
11th International Conference on Autonomous Agents and MultiAgent Systems (AAMAS-12), Valencia, Spain, June 4-8, 2012.
Doctoral consortium abstract
Abstract. This paper presents a novel decision-theoretic approach to control and coordinate multiple active cameras for observing a number of moving targets in a surveillance system. This approach offers the advantages of being able to (a) account for the stochasticity of targets' motion via probabilistic modeling, and (b) address the trade-off between maximizing the expected number of observed targets and the resolution of the observed targets through stochastic optimization. One of the key issues faced by existing approaches in multi-camera surveillance is that of scalability with increasing number of targets. We show how its scalability can be improved by exploiting the problem structure: as proven analytically, our decision-theoretic approach incurs time that is linear in the number of targets to be observed during surveillance. As demonstrated empirically through simulations, our proposed approach can achieve high-quality surveillance of up to 50 targets in real time and its surveillance performance degrades gracefully with increasing number of targets. We also demonstrate our proposed approach with real AXIS 214 PTZ cameras in maximizing the number of Lego robots observed at high resolution over a surveyed rectangular area. The results are promising and clearly show the feasibility of our decision-theoretic approach in controlling and coordinating the active cameras in real surveillance system.
MULTI-ROBOT INFORMATIVE PATH PLANNING FOR ACTIVE SENSING OF SPATIOTEMPORAL ENVIRONMENTAL PHENOMENA
PROJECT DURATION : Jan 2010 - May 2013
PROJECT AFFILIATION :
Collaborative Multi-Robot Exploration of the Coastal Ocean (Collaborators: John M. Dolan, CMU; Gaurav S. Sukhatme, USC; Kanna Rajan, MBARI)
PROJECT FUNDING : MOE AcRF Tier 1 Grant :
Active Robotic Exploration and Mapping for Environmental Sensing Applications,
SGD $165,377, Apr 2010 - Mar 2013
PROBLEM MOTIVATION
Research in environmental sensing and monitoring has recently gained significant attention and practical interest, especially in supporting environmental sustainability efforts worldwide. A key direction of this research aims at sensing, modeling, and predicting the various types of environmental phenomena spatially distributed over our natural and built-up habitats so as to improve our knowledge and understanding of their economic, environmental, and health impacts and implications. This is non-trivial to achieve due to a trade-off between the quantity of sensing resources (e.g., number of deployed sensors, energy consumption, mission time) and the uncertainty in predictive modeling. In the case of deploying a limited number of mobile robotic sensing assets, such a trade-off motivates the need to plan the most informative resource-constrained observation paths to minimize the uncertainty in modeling and predicting a spatially varying environmental phenomenon, which constitutes the active sensing problem to be addressed in this work.
A wide multitude of natural and urban environmental phenomena is characterized by spatially correlated field measurements, which raises the following fundamental issue faced by the active sensing problem:
How can the spatial correlation structure of an environmental phenomenon be exploited to improve the active sensing performance and computational efficiency of robotic path planning?
In this work, we will investigate the above issue for an important broad class of environmental phenomena called anisotropic fields that exhibit a (often much) higher spatial correlation along one direction than along its per- pendicular direction. Such fields occur widely in natural and built-up environments and some of them include (a) ocean and freshwater phenomena like plankton density, fish abundance, temperature and salinity; (b) soil and atmospheric phenomena like peat thickness, surface soil moisture, rainfall; (c) mineral deposits like radioactive ore; (d) pollutant and contaminant concentration like air, heavy metals; and (e) ecological abundance like vegetation density.
PROPOSED METHODOLOGY
This work presents two principled approaches to efficient information-theoretic path planning based on entropy and mutual information criteria for in situ active sensing of environmental phenomena. In contrast to the existing methods described above, our proposed path planning algorithms are novel in addressing a trade-off between active sensing performance and computational efficiency. An important practical consequence is that our algorithms can exploit the spatial correlation structure of anisotropic fields to improve time efficiency while preserving near-optimal active sensing performance.
PUBLICATIONS
- Multi-Robot Informative Path Planning for Active Sensing of Environmental Phenomena: A Tale of Two Algorithms.
Nannan Cao, Kian Hsiang Low & John M. Dolan.
In Proceedings of the
12th International Conference on Autonomous Agents and MultiAgent Systems (AAMAS-13), pages 7-14, Saint Paul, MN, May 6-10, 2013.
22.9% acceptance rate
Abstract. A key problem of robotic environmental sensing and monitoring is that of active sensing: How can a team of robots plan the most informative observation paths to minimize the uncertainty in modeling and predicting an environmental phenomenon? This paper presents two principled approaches to efficient information-theoretic path planning based on entropy and mutual information criteria for in situ active sensing of an important broad class of widely-occurring environmental phenomena called anisotropic fields. Our proposed algorithms are novel in addressing a trade-off between active sensing performance and time efficiency. An important practical consequence is that our algorithms can exploit the spatial correlation structure of Gaussian process-based anisotropic fields to improve time efficiency while preserving near-optimal active sensing performance. We analyze the time complexity of our algorithms and prove analytically that they scale better than state-of-the-art algorithms with increasing planning horizon length. We provide theoretical guarantees on the active sensing performance of our algorithms for a class of exploration tasks called transect sampling, which, in particular, can be improved with longer planning time and/or lower spatial correlation along the transect. Empirical evaluation on real-world anisotropic field data shows that our algorithms can perform better or at least as well as the state-of-the-art algorithms while often incurring a few orders of magnitude less computational time, even when the field conditions are less favorable.
- Information-Theoretic Multi-Robot Path Planning.
Nannan Cao.
M.Sc. Thesis, Department of Computer Science, National University of Singapore, Sep 2012.
Abstract.
Research in environmental sensing and monitoring is especially important in supporting environmental sustainability efforts worldwide, and has recently attracted significant attention and interest. A key direction of this research lies in modeling and predicting the spatiotemporally varying environmental phenomena. One approach is to use a team of robots to sample the area and model the measurement values at unobserved points. For smoothly varying and hotspot fields, there is some work which has been done to model the fields well. However, there is still a class of common environmental fields called anisotropic fields in which the spatial phenomena are highly correlated along one direction and less correlated along the perpendicular direction. We exploit the environmental structure to improve the sampling performance and time efficiency of planning for anisotropic fields.
In this thesis, we cast the planning problem into a stagewise decision-theoretic problem. we adopt Gaussian Process to model spatial phenomena. Maximum entropy criterion and maximum mutual information criterion are used to measure the informativeness of the observation paths. It is found that for many GPs, correlation of two points exponentially decreases with the distance between the two points. With this property, for maximum entropy criterion, we propose a polynomial-time approximation algorithm, MEPP, to find the maximum entropy paths. We also provide a theoretical performance guarantee for this algorithm. For maximum mutual information criterion, we propose another polynomial-time approximation algorithm, M2IPP. Similar to the MEPP, a performance guarantee is also provided for this algorithm. We demonstrate the performance advantages of our algorithms on two real data sets. To get lower prediction error, three priciples have also been proposed to select the criterion for different environmental fields.
- Active Markov Information-Theoretic Path Planning for Robotic Environmental Sensing.
Kian Hsiang Low, John M. Dolan & Pradeep Khosla.
In Proceedings of the
10th International Conference on Autonomous Agents and MultiAgent Systems (AAMAS-11), pages 753-760, Taipei, Taiwan, May 2-6, 2011.
22.1% acceptance rate
Abstract. Recent research in multi-robot exploration and mapping has focused on sampling environmental fields, which are typically modeled using the Gaussian process (GP). Existing information-theoretic exploration strategies for learning GP-based environmental field maps adopt the non-Markovian problem structure and consequently scale poorly with the length of history of observations. Hence, it becomes computationally impractical to use these strategies for in situ, real-time active sampling. To ease this computational burden, this paper presents a Markov-based approach to efficient information-theoretic path planning for active sampling of GP-based fields. We analyze the time complexity of solving the Markov-based path planning problem, and demonstrate analytically that it scales better than that of deriving the non-Markovian strategies with increasing length of planning horizon. For a class of exploration tasks called the transect sampling task, we provide theoretical guarantees on the active sampling performance of our Markov-based policy, from which ideal environmental field conditions and sampling task settings can be established to limit its performance degradation due to violation of the Markov assumption. Empirical evaluation on real-world temperature and plankton density field data shows that our Markov-based policy can generally achieve active sampling performance comparable to that of the widely-used non-Markovian greedy policies under less favorable realistic field conditions and task settings while enjoying significant computational gain over them.
ENVIRONMENTAL BOUNDARY TRACKING & ESTIMATION WITH MULTIPLE ROBOTS
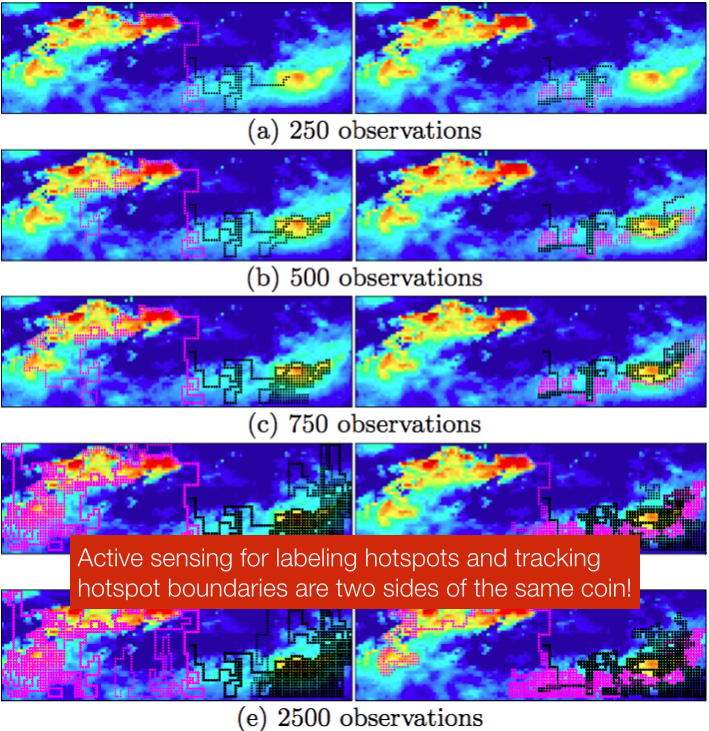
PROJECT DURATION : Jan 2010 - May 2012
PROJECT COLLABORATORS : John M. Dolan, CMU; Steve Chien, JPL, Caltech
PROJECT FUNDING : MOE AcRF Tier 1 Grant :
Active Robotic Exploration and Mapping for Environmental Sensing Applications,
SGD $165,377, Apr 2010 - Mar 2013
PROBLEM MOTIVATION
A fundamental problem in environmental sensing and monitoring is to identify and delineate the hotspot regions in a large-scale environmental field. It involves partitioning the area spanned by the field into one class of regions called the hotspot regions in which the field measurements exceed a predefined threshold, and the other class of regions where they do not. Such a problem arises in many real-world applications such as precision agriculture, monitoring of ocean and freshwater phenomena (e.g., plankton bloom), forest ecosystems, rare species, pollution (e.g., oil spill), or contamination (e.g., radiation leak). In these applications, it is necessary to assess the spatial extent and shape of the hotspot regions accurately due to severe economic, environmental, and health implications. In practice, this aim is non-trivial to achieve because the constraints on the sampling assets' resources (e.g., energy consumption, mission time, sensing range) limit the number and coverage of in situ observations over the large field that can be used to infer the hotspot regions. Subject to limited observations, the most informative ones should therefore be selected in order to minimize the uncertainty of estimating the hotspot regions (or, equivalently, classifying/labeling the hotspots) in the large field, which motivates our adaptive sampling work in this work.
Mobile robot teams are particularly desirable for performing the above environmental sensing task because they can actively explore to map the hotspot regions at high resolution. On the other hand, static sensors lack mobility and are therefore not capable of doing this well unless a large quantity is deployed. While research in multi-robot exploration and mapping have largely focused on the conventional task of building occupancy grids, some recent efforts are put into the more complex, general task of sampling spatially distributed environmental fields. In contrast to occupancy grids that assume discrete, independent cell occupancies, environmental fields are characterized by continuous-valued, spatially correlated measurements, properties of which cannot be exploited by occupancy grid mapping strategies to select the most informative observation paths. To exploit such properties, exploration strategies for learning environmental field maps have recently been developed and can be classified into two regimes: (a) wide-area coverage strategies consider sparsely sampled (i.e., largely unexplored) areas to be of high uncertainty and consequently spread observations evenly across the field; (b) hotspot sampling strategies assume areas of high uncertainty and interest to contain extreme, highly-varying measurements and hence produce clustered observations.
Formal, principled approaches of exploration have also been devised to simultaneously perform hotspot sampling when a hotspot region is found as well as wide-area coverage to search for new hotspot regions in sparsely sampled areas. These strategies optimize their observation paths to minimize the uncertainty (e.g., in terms of mean-squared error or entropy) of mapping the entire continuous-valued field. They are, however, suboptimal for classifying/labeling the hotspots in the field, which we will discuss and demonstrate theoretically and empirically in this work.
PROPOSED METHODOLOGY
This work presents a novel decentralized active robotic exploration (DARE) strategy for probabilistic classification/labeling of hotspots in a large-scale environmental field. The environmental field is assumed to be realized from a rich class of probabilistic spatial models called Gaussian process (GP) that can formally characterize its spatial correlation structure. More importantly, it can provide formal measures of classification/labeling uncertainty (i.e., in the form of cost functions) such as the misclassification and entropy criteria for directing the robots to explore highly uncertain areas of the field. The chief impediment to using these formal criteria is that they result in cost-minimizing exploration strategies, which cannot be solved in closed form. To resolve this, they are reformulated as reward-maximizing dual strategies, from which we can then derive the approximate DARE strategy to be solved in closed form efficiently. The specific contributions of our work include:
- Analyzing the time complexity of solving the DARE strategy: We prove that its incurred time is independent of the map resolution and the number of robots, thus making it practical for in situ, real-time active sampling. In contrast, existing state-of-the-art exploration strategies for learning environmental field maps scale poorly with increasing map resolution and/or number of robots;
- Analyzing the exploration behavior of the DARE strategy through its formulation: It exhibits an interesting formal trade-off between that of boundary tracking until the hotspot region boundary can be accurately predicted and wide-area coverage to find new boundaries in sparsely sampled areas to be tracked. In contrast, ad hoc, reactive boundary tracking strategies typically require a hotspot region boundary to be located manually or via random exploration and are not driven by the need to maximize the fidelity of estimating multiple hotspot regions given limited observations;
- Providing theoretical guarantee on the active exploration performance of the DARE strategy: We prove that, under a reasonable conditional independence assumption, it produces the same optimal observation paths as that of the centralized cost-minimizing strategies, the latter of which otherwise cannot be solved in closed form. This result has a simple but important implication: The uncertainty of labeling the hotspots in a GP-based field is greatest at or close to the hotspot region boundaries;
- Empirically evaluating the active exploration performance and time efficiency of the DARE strategy on real-world plankton density and temperature field data: Subject to limited observations, the DARE strategy can achieve better classification of the hotspots than state-of-the-art active exploration strategies while being significantly more time-efficient than those performing wide-area coverage and hotspot sampling.
PUBLICATIONS
- Decentralized Active Robotic Exploration and Mapping for Probabilistic Field Classification in Environmental Sensing.
Kian Hsiang Low, Jie Chen, John M. Dolan, Steve Chien & David R. Thompson.
In Proceedings of the
11th International Conference on Autonomous Agents and MultiAgent Systems (AAMAS-12), pages 105-112, Valencia, Spain, June 4-8, 2012.
20.4% acceptance rate
Also appeared in
IROS'11 Workshop on Robotics for Environmental Monitoring (WREM-11), San Francisco, CA, Sep 30, 2011.
Abstract. A central problem in environmental sensing and monitoring is to classify/label the hotspots in a large-scale environmental field. This paper presents a novel decentralized active robotic exploration (DARE) strategy for probabilistic classification/labeling of hotspots in a Gaussian process (GP)-based field. In contrast to existing state-of-the-art exploration strategies for learning environmental field maps, the time needed to solve the DARE strategy is independent of the map resolution and the number of robots, thus making it practical for in situ, real-time active sampling. Its exploration behavior exhibits an interesting formal trade-off between that of boundary tracking until the hotspot region boundary can be accurately predicted and wide-area coverage to find new boundaries in sparsely sampled areas to be tracked. We provide a theoretical guarantee on the active exploration performance of the DARE strategy: under reasonable conditional independence assumption, we prove that it can optimally achieve two formal cost-minimizing exploration objectives based on the misclassification and entropy criteria. Importantly, this result implies that the uncertainty of labeling the hotspots in a GP-based field is greatest at or close to the hotspot region boundaries. Empirical evaluation on real-world plankton density and temperature field data shows that, subject to limited observations, DARE strategy can achieve more superior classification of hotspots and time efficiency than state-of-the-art active exploration strategies.
MULTI-ROBOT ADAPTIVE SAMPLING FOR ENVIRONMENTAL SENSING & MONITORING
PROJECT DURATION : Jul 2005 - Mar 2010
PROJECT LAB : T-SAR
PROBLEM MOTIVATION
Recent research in multi-robot exploration and mapping has focused on sampling environmental fields, some of which typically feature a few small hotspots in a large region.
Such a hotspot field often arises in two real-world applications:
(1) planetary exploration such as geologic reconnaissance and prospecting for mineral deposits or natural gases, and
(2) environment and ecological sensing such as precision agriculture, and monitoring of ocean phenomena (e.g., plankton bloom, anoxic zones), forest ecosystems, rare species, pollution (e.g., oil spill), or contamination (e.g., radiation leak).
In particular, the hotspot field is characterized by continuous-valued, spatially correlated measurements with the hotspots exhibiting extreme measurements and much higher spatial variability than the rest of the field.
With limited (e.g., point-based) robot sensing range, a complete coverage becomes impractical in terms of resource costs (e.g., resource consumption).
So, to accurately map the field, the hotspots have to be sampled at a higher resolution.
The hotspot field discourages static sensor placement because a large number of sensors has to be positioned to detect and refine the sampling of hotspots. If these static sensors are not placed in any hotspot initially, they cannot reposition by themselves to locate one. In contrast, a robot team is capable of performing high-resolution hotspot sampling due to its mobility. Hence, it is desirable to build a mobile robot team that can actively explore to map a hotspot field.
PROPOSED METHODOLOGY
To learn a hotspot field map, the exploration strategy of the robot team has to plan the most informative resource-constrained observation paths that minimize the uncertainty of mapping the hotspot field.
By representing the hotspot field using rich classes of Bayesian non-parametric models such as the Gaussian process or log-Gaussian process,
formal measures of mapping uncertainty (e.g., based on mean-squared error [ AAMAS-08] or entropy [ ICAPS-09] criterion) can be defined and subsequently exploited by our proposed adaptive sampling algorithms for directing the robot team to explore highly uncertain areas of the field.
In contrast to non-adaptive sampling strategies that only perform well with smoothly-varying fields,
our non-myopic adaptive sampling algorithms can exploit clustering phenomena (i.e., hotspots) to plan observation paths that produce lower mapping uncertainty.
PUBLICATIONS
- Adaptive Sampling of Time Series with Application to Remote Exploration.
David R. Thompson, Nathalie Cabrol, Michael Furlong, Craig Hardgrove, Kian Hsiang Low, Jeffrey Moersch & David Wettergreen.
In Proceedings of the IEEE International Conference on Robotics and Automation (ICRA'13), pages 3463-3468, Karlsruhe, Germany, May 6-10, 2013.
Abstract. We address the problem of adaptive information-optimal data collection in time series. Here a remote sensor or explorer agent throttles its sampling rate in order to track anomalous events while obeying constraints on time and power. This problem is challenging because the agent has limited visibility - all collected datapoints lie in the past, but its resource allocation decisions require predicting far into the future. Our solution is to continually fit a Gaussian process model to the latest data and optimize the sampling plan on line to maximize information gain. We compare the performance characteristics of stationary and nonstationary Gaussian process models. We also describe an application based on geologic analysis during planetary rover exploration. Here adaptive sampling can improve coverage of localized anomalies and potentially benefit mission science yield of long autonomous traverses.
- Telesupervised Remote Surface
Water Quality Sensing.
Gregg Podnar, John M. Dolan, Kian Hsiang Low & Alberto Elfes.
In Proceedings of the IEEE Aerospace Conference, Big Sky, MT, Mar 6-13, 2010.
Abstract. We present a fleet of autonomous Robot Sensor Boats (RSBs) developed for lake and river fresh water quality assessment and controlled by our Multilevel Autonomy Robot Telesupervision Architecture (MARTA). The RSBs are low cost, highly maneuverable, shallow draft sensor boats, developed as part of the Sensor Web program supported under the Advanced Information Systems Technology program of NASA's Earth Systems Technology Office. They can scan large areas of lakes, and navigate up tributaries to measure water quality near outfalls that larger research vessels cannot reach. The MARTA telesupervision architecture has been applied to a number of domains from multi-platform autonomous wide area planetary mineral prospecting, to multi-platform ocean monitoring. The RSBs are a complementary expansion of a fleet of NOAA/NASA-developed extended-deployment surface autonomous vehicles that enable in-situ study of meteorological factors of the ocean/atmosphere interface, and which have been adapted to investigate harmful algal blooms under this program. The flexibility of the MARTA telesupervision architecture was proven as it supported simultaneous operation of these heterogenous autonomous sensor platforms while geographically widely separated. Results and analysis are presented of multiple tests carried out over three months using a multi-sensor water sonde to assess water quality in a small recreational lake. Inference Grids were used to produce maps representing temperature, pH, and dissolved oxygen. The tests were performed under various water conditions (clear vs. hair algae-laden) and both before and after heavy rains. Data from each RSB was relayed to a data server in our lab in Pittsburgh, Pennsylvania, and made available over the World Wide Web where it was acquired by team members at the Jet Propulsion Laboratory of NASA in Pasadena, California who monitored the boats and their sensor readings in real time, as well as using these data to model the water quality by producing Inference Grid-based maps.
- Multi-Robot Adaptive Exploration and Mapping for Environmental Sensing Applications.
Kian Hsiang Low.
Ph.D. Thesis, Technical Report CMU-ECE-2009-024, Department of Electrical and Computer Engineering, Carnegie Mellon University, Pittsburgh, PA, Aug 2009.
Abstract.
Recent research in robot exploration and mapping has focused on sampling hotspot fields, which often arise in environmental and ecological sensing applications. Such a hotspot field is characterized by continuous, positively skewed, spatially correlated measurements with the hotspots exhibiting extreme measurements and much higher spatial variability than the rest of the field.
To map a hotspot field of the above characterization, we assume that it is realized from non-parametric probabilistic models such as the Gaussian and log-Gaussian processes (respectively, GP and lGP), which can provide formal measures of map uncertainty. To learn a hotspot field map, the exploration strategy of a robot team then has to plan resource-constrained observation paths that minimize the uncertainty of a spatial model of the hotspot field. This exploration problem is formalized in a sequential decision-theoretic planning under uncertainty framework called the multi-robot adaptive sampling problem (MASP). So, MASP can be viewed as a sequential, non-myopic version of active learning. In contrast to finite-state Markov decision problems, MASP adopts a more complex but realistic continuous-state, non-Markovian problem structure so that its induced exploration policy can be informed by the complete history of continuous, spatially correlated observations for selecting paths. It is unique in unifying formulations of non-myopic exploration problems along the entire adaptivity spectrum, thus subsuming existing non-adaptive formulations and allowing the performance advantage of a more adaptive policy to be theoretically realized. Through MASP, it is demonstrated that a more adaptive strategy can exploit clustering phenomena in a hotspot field to produce lower expected map uncertainty. By measuring map uncertainty using the mean-squared error criterion, a MASP-based exploration strategy consequently plans adaptive observation paths that minimize the expected posterior map error or equivalently, maximize the expected map error reduction.
The time complexity of solving MASP (approximately) depends on the map resolution, which limits its practical use in large-scale, high-resolution exploration and mapping. This computational difficulty is alleviated through an information-theoretic approach to MASP (iMASP), which measures map uncertainty based on the entropy criterion instead. As a result, an iMASP-based exploration strategy plans adaptive observation paths that minimize the expected posterior map entropy or equivalently, maximize the expected entropy of observation paths. Unlike MASP, reformulating the cost-minimizing iMASP as a reward-maximizing dual problem causes its time complexity of being solved approximately to be independent of the map resolution and less sensitive to larger robot team size as demonstrated both analytically and empirically. Furthermore, this reward-maximizing dual transforms the widely-used non-adaptive maximum entropy sampling problem into a novel adaptive variant, thus improving the performance of the induced exploration policy.
One advantage stemming from the reward-maximizing dual formulations of MASP and iMASP is that they allow observation selection properties of the induced exploration policies to be realized for sampling the hotspot field. These properties include adaptivity, hotspot sampling, and wide-area coverage. We show that existing GP-based exploration strategies may not explore and map the hotspot field well with the selected observations because they are non-adaptive and perform only wide-area coverage. In contrast, the lGP-based exploration policies can learn a high-quality hotspot field map because they are adaptive and perform both wide-area coverage and hotspot sampling.
The other advantage is that even though MASP and iMASP are non-trivial to solve due to their continuous state components, the convexity of their reward-maximizing duals can be exploited to derive, in a computationally tractable manner, discrete-state monotone-bounding approximations and subsequently, approximately optimal exploration policies with theoretical performance guarantees. Anytime algorithms based on approximate MASP and iMASP are then proposed to alleviate the computational difficulty that arises from their non-Markovian structure.
It is of practical interest to be able to quantitatively characterize the "hotspotness" of an environmental field. We propose a novel "hotspotness" index, which is defined in terms of the spatial correlation properties of the hotspot field. As a result, this index can be related to the intensity, size, and diffuseness of the hotspots in the field.
We also investigate how the spatial correlation properties of the hotspot field affect the performance advantage of adaptivity. In particular, we derive sufficient and necessary conditions of the spatial correlation properties for adaptive exploration to yield no performance advantage.
Lastly, we develop computationally efficient approximately optimal exploration strategies for sampling the GP by assuming the Markov property in iMASP planning. We provide theoretical guarantees on the performance of the Markov-based policies, which improve with decreasing spatial correlation. We evaluate empirically the effects of varying spatial correlations on the mapping performance of the Markov-based policies as well as whether these Markov-based path planners are time-efficient for the transect sampling task.
Through the abovementioned work, this thesis establishes the following two claims: (1) adaptive, non-myopic exploration strategies can exploit clustering phenomena to plan observation paths that produce lower map uncertainty than non-adaptive, greedy methods; and (2) Markov-based exploration strategies can exploit small spatial correlation to plan observation paths which achieve map uncertainty comparable to that of non-Markovian policies using significantly less planning time.
- Information-Theoretic Approach to Efficient Adaptive Path Planning for Mobile Robotic Environmental Sensing.
Kian Hsiang Low, John M. Dolan & Pradeep Khosla.
In Proceedings of the 19th International Conference on Automated Planning and Scheduling (ICAPS-09), pages 233-240, Thessaloniki, Greece, Sep 19-23, 2009.
33.9% acceptance rate
Also appeared in IPSN-09 Workshop on Sensor Networks for Earth and Space Science Applications (ESSA-09), San Francisco, CA, Apr 16, 2009.
Also orally presented in RSS-09 Workshop on Aquatic Robots and Ocean Sampling, Seattle, WA, Jun 29, 2009.
Abstract. Recent research in robot exploration and mapping has focused on sampling environmental hotspot fields. This exploration task is formalized by Low, Dolan, and Khosla (2008) in a sequential decision-theoretic planning under uncertainty framework called MASP. The time complexity of solving MASP approximately depends on the map resolution, which limits its use in large-scale, high-resolution exploration and mapping. To alleviate this computational difficulty, this paper presents an information-theoretic approach to MASP (iMASP) for efficient adaptive path planning; by reformulating the cost-minimizing iMASP as a reward-maximizing problem, its time complexity becomes independent of map resolution and is less sensitive to increasing robot team size as demonstrated both theoretically and empirically. Using the reward-maximizing dual, we derive a novel adaptive variant of maximum entropy sampling, thus improving the induced exploration policy performance. It also allows us to establish theoretical bounds quantifying the performance advantage of optimal adaptive over non-adaptive policies and the performance quality of approximately optimal vs. optimal adaptive policies. We show analytically and empirically the superior performance of iMASP-based policies for sampling the log-Gaussian process to that of policies for the widely-used Gaussian process in mapping the hotspot field. Lastly, we provide sufficient conditions that, when met, guarantee adaptivity has no benefit under an assumed environment model.
- Cooperative Aquatic Sensing using the Telesupervised Adaptive Ocean Sensor Fleet.
John M. Dolan, Gregg W. Podnar, Stephen Stancliff, Kian Hsiang Low, Alberto Elfes, John Higinbotham, Jeffrey C. Hosler, Tiffany A. Moisan & John Moisan.
In Proceedings of the SPIE Conference on Remote Sensing of the Ocean, Sea Ice, and Large Water Regions, volume 7473, Berlin, Germany, Aug 31 - Sep 3, 2009.
Abstract. Earth science research must bridge the gap between the atmosphere and the ocean to foster understanding of Earth's climate and ecology. Typical ocean sensing is done with satellites or in situ buoys and research ships which are slow to reposition. Cloud cover inhibits study of localized transient phenomena such as Harmful Algal Blooms (HAB). A fleet of extended-deployment surface autonomous vehicles will enable in situ study of characteristics of HAB, coastal pollutants, and related phenomena. We have developed a multiplatform telesupervision architecture that supports adaptive reconfiguration based on environmental sensor inputs. Our system allows the autonomous repositioning of smart sensors for HAB study by networking a fleet of NOAA OASIS (Ocean Atmosphere Sensor Integration System) surface autonomous vehicles. In situ measurements intelligently modify the search for areas of high concentration. Inference Grid and complementary information-theoretic techniques support sensor fusion and analysis. Telesupervision supports sliding autonomy from high-level mission tasking, through vehicle and data monitoring, to teleoperation when direct human interaction is appropriate. This paper reports on experimental results from multi-platform tests conducted in the Chesapeake Bay and in Pittsburgh, Pennsylvania waters using OASIS platforms, autonomous kayaks, and multiple simulated platforms to conduct cooperative sensing of chlorophyll-a and water quality.
- Robot Boats as a Mobile Aquatic Sensor Network.
Kian Hsiang Low, Gregg Podnar, Stephen Stancliff, John M. Dolan & Alberto Elfes.
In Proceedings of the IPSN-09 Workshop on Sensor Networks for Earth and Space Science Applications (ESSA-09), San Francisco, CA, Apr 16, 2009.
Abstract. This paper describes the Multilevel Autonomy Robot Telesupervision Architecture (MARTA), an architecture for supervisory control of a heterogeneous fleet of net-worked unmanned autonomous aquatic surface vessels carrying a payload of environmental science sensors. This architecture allows a land-based human scientist to effectively supervise data gathering by multiple robotic assets that implement a web of widely dispersed mobile sensors for in situ study of physical, chemical or bio-logical processes in water or in the water/atmosphere interface.
- Adaptive Multi-Robot Wide-Area Exploration And Mapping.
Kian Hsiang Low, John M. Dolan & Pradeep Khosla.
In Proceedings of the
7th International Conference on Autonomous Agents and MultiAgent Systems (AAMAS-08), pages 23-30, Estoril, Portugal, May 12-16, 2008.
22.2% acceptance rate
Also presented as a poster in RSS-09 Workshop on Aquatic Robots and Ocean Sampling, Seattle, WA, Jun 29, 2009.
Abstract. The exploration problem is a central issue in mobile robotics. A complete terrain coverage is not practical if the environment is large with only a few small hotspots. This paper presents an adaptive multi-robot exploration strategy that is novel in performing both wide-area coverage and hotspot sampling using non-myopic path planning. As a result, the environmental phenomena can be accurately mapped. It is based on a dynamic programming formulation, which we call the Multi-robot Adaptive Sampling Problem (MASP). A key feature of MASP is in covering the entire adaptivity spectrum, thus allowing strategies of varying adaptivity to be formed and theoretically analyzed in their performance; a more adaptive strategy improves mapping accuracy. We apply MASP to sampling the Gaussian and log-Gaussian processes, and analyze if the resulting strategies are adaptive and maximize wide-area coverage and hotspot sampling. Solving MASP is non-trivial as it comprises continuous state components. So, it is reformulated for convex analysis, which allows discrete-state monotone-bounding approximation to be developed. We provide a theoretical guarantee on the policy quality of the approximate MASP (aMASP) for using in MASP. Although aMASP can be solved exactly, its state size grows exponentially with the number of stages. To alleviate this computational difficulty, anytime algorithms are proposed based on aMASP, one of which can guarantee its policy quality for MASP in real time.
- Adaptive Sampling for Multi-Robot Wide-Area Exploration.
Kian Hsiang Low, Geoffrey J. Gordon, John M. Dolan & Pradeep Khosla.
In Proceedings of the IEEE International Conference on Robotics and Automation (ICRA'07), pages 755-760, Rome, Italy, Apr 10-14, 2007.
Abstract. The exploration problem is a central issue in mobile robotics. A complete coverage is not practical if the environment is large with a few small hotspots, and the sampling cost is high. So, it is desirable to build robot teams that can coordinate to maximize sampling at these hotspots while minimizing resource costs, and consequently learn more accurately about properties of such environmental phenomena. An important issue in designing such teams is the exploration strategy. The contribution of this paper is in the evaluation of an adaptive exploration strategy called adaptive cluster sampling (ACS), which is demonstrated to reduce the resource costs (i.e., mission time and energy consumption) of a robot team, and yield more information about the environment by directing robot exploration towards hotspots. Due to the adaptive nature of the strategy, it is not obvious how the sampled data can be used to provide unbiased, low-variance estimates of the properties. This paper therefore discusses how estimators that are Rao-Blackwellized can be used to achieve low error. This paper also presents the first analysis of the characteristics of the environmental phenomena that favor the ACS strategy and estimators. Quantitative experimental results in a mineral prospecting task simulation show that our approach is more efficient in exploration by yielding more minerals and information with fewer resources and providing more precise mineral density estimates than previous methods.
- Adaptive Sampling for Multi-Robot Wide Area Prospecting.
Kian Hsiang Low, Geoffrey J. Gordon, John M. Dolan, and Pradeep Khosla.
In Technical Report CMU-RI-TR-05-51, Robotics Institute, Carnegie Mellon University, Pittsburgh, PA, Oct 2005.
Abstract. Prospecting for in situ mineral resources is essential for establishing settlements on the Moon and Mars. To reduce human effort and risk, it is desirable to build robotic systems to perform this prospecting. An important issue in designing such systems is the sampling strategy: how do the robots choose where to prospect next? This paper argues that a strategy called Adaptive Cluster Sampling (ACS) has a number of desirable properties: compared to conventional strategies, (1) it reduces the total mission time and energy consumption of a team of robots, and (2) returns a higher mineral yield and more information about the prospected region by directing exploration towards areas of high mineral density, thus providing detailed maps of the boundaries of such areas. Due to the adaptive nature of the sampling scheme, it is not immediately obvious how the resulting sampled data can be used to provide an unbiased, low-variance estimate of the regional mineral density. This paper therefore investigates new mineral density estimators, which have lower error than previously-developed estimators; they are derived from the older estimators via a process called Rao-Blackwellization. Since the efficiency of estimators depends on the type of mineralogical population sampled, the population characteristics that favor ACS estimators are also analyzed. The ACS scheme and our new estimators are evaluated empirically in a detailed simulation of the prospecting task, and the quantitative results show that our approach can yield more minerals with less resources and provide more accurate mineral density estimates than previous methods.
DISTRIBUTED LAYERED ARCHITECTURE FOR SELF-ORGANIZING MOBILE SENSOR NETWORKS
PROJECT DURATION : Nov 2002 - Jun 2005
PROBLEM MOTIVATION
One of the fundamental issues that arises in a sensor network is coverage.
Traditionally, network coverage is maximized by determining the optimal placement of static sensors in a centralized manner,
which can be related to the class of art gallery problems.
However, recent investigations in sensor network mobility reveal that
mobile sensors can self-organize to provide better coverage than static placement.
Existing applications have only utilized uninformed mobility (i.e., random motion or patrol).
In contrast, our work here focuses on informed, intelligent mobility to further improve coverage.
Our network coverage problem is motivated by the following constraints
that discourage static sensor placement or uninformed mobility:
(a) no prior information about the exact target locations, population densities or motion pattern,
(b) limited sensory range, and
(c) very large area to be observed.
All these conditions may cause the sensors to be unable to cover the entire region of interest.
Hence, fixed sensor locations or uninformed mobility will not be adequate in general.
Rather, the sensors have to move dynamically in response to the motion and distribution of targets and other sensors
to maximize coverage.
Inspired by robotics, the above problem may be regarded as that of low-level motion control to coordinate the sensors'
target tracking movements in the continuous workspace.
Alternatively, it can be cast as a high-level task allocation problem by segmenting the workspace into discrete regions
such that each region is assigned a group or coalition of sensors to track the targets within.
PROPOSED METHODOLOGY
This work presents a reactive layered multi-robot architecture
for distributed mobile sensor network coverage in complex, dynamic environments.
At the lower layer, each robot uses a reactive motion control strategy
known as Cooperative Extended Kohonen Maps
to coordinate their target tracking within a region without the need of communication.
This strategy is also responsible for obstacle avoidance, robot separation to minimize task interference,
and navigation between regions via beacons or checkpoints plotted by a motion planner.
At the higher layer, the robots use a dynamic ant-based task allocation scheme to cooperatively self-organize
their coalitions in a decentralized manner according to the target distributions across the regions.
This scheme addresses the following issues, which distinguish it from the other task allocation mechanisms:
Task Allocation for Multi-Robot Tasks: Existing algorithms (e.g., auction-and behavior-based) assume a multi-robot
task can be partitioned into single-robot tasks. But this may not be always possible or the multi-robot task
can be more efficiently performed by coalitions of robots.
Coalition Formation for Minimalist Robots: Existing coalition formation schemes require complex planning, explicit
negotiation, and precise estimation of coalitional cost. Hence, they do not perform well in dynamic, real-time scenarios.
Cooperation of Resource-Limited Robots: Robots with limited communication and sensing capabilities (i.e., partial
observability) can only obtain local, uncertain information of the dynamic environment. With limited computational power,
their cooperative strategies cannot involve complex planning or negotiations.
PUBLICATIONS
- Autonomic Mobile Sensor Network with Self-Coordinated Task Allocation and Execution.
Kian Hsiang Low, Wee Kheng Leow & Marcelo H. Ang, Jr.
IEEE Transactions on Systems, Man, and Cybernetics - Part C: Applications and Reviews
(Special Issue on Engineering Autonomic Systems), volume 36, issue 3, pages 315-327, May 2006.
Extended version of our ICRA'04 and
AAAI-04 papers
Andrew P. Sage Best Transactions Paper Award for the best paper published in IEEE Trans. SMC - Part A, B, and C in 2006
Abstract. This paper describes a distributed layered architecture for resource-constrained multirobot cooperation, which is utilized in autonomic mobile sensor network coverage. In the upper layer, a dynamic task allocation scheme self-organizes the robot coalitions to track efficiently across regions. It uses concepts of ant behavior to self-regulate the regional distributions of robots in proportion to that of the moving targets to be tracked in a nonstationary environment. As a result, the adverse effects of task interference between robots are minimized and network coverage is improved. In the lower task execution layer, the robots use self-organizing neural networks to coordinate their target tracking within a region. Both layers employ self-organization techniques, which exhibit autonomic properties such as self-configuring, self-optimizing, self-healing, and self-protecting. Quantitative comparisons with other tracking strategies such as static sensor placements, potential fields, and auction-based negotiation show that our layered approach can provide better coverage, greater robustness to sensor failures, and greater flexibility to respond to environmental changes.
- Task Allocation via Self-Organizing Swarm Coalitions in Distributed Mobile Sensor Network.
Kian Hsiang Low, Wee Kheng Leow & Marcelo H. Ang, Jr.
In Proceedings of the 19th National Conference on Artificial Intelligence (AAAI-04), pages 28-33, San Jose, CA, Jul 25-29, 2004.
26.7% acceptance rate
Abstract. This paper presents a task allocation scheme via self-organizing swarm coalitions for distributed mobile sensor network coverage. Our approach uses the concepts of ant behavior to self-regulate the regional distributions of sensors in proportion to that of the moving targets to be tracked in a non-stationary environment. As a result, the adverse effects of task interference between robots are minimized and sensor network coverage is improved. Quantitative comparisons with other tracking strategies such as static sensor placement, potential fields, and auction-based negotiation show that our approach can provide better coverage and greater flexibility to respond to environmental changes.
- Reactive, Distributed Layered Architecture for Resource-Bounded Multi-Robot Cooperation: Application to Mobile Sensor Network Coverage.
Kian Hsiang Low, Wee Kheng Leow & Marcelo H. Ang, Jr.
In Proceedings of the IEEE International Conference on Robotics and Automation (ICRA'04), pages 3747-3752, New Orleans, LA, Apr 26 - May 1, 2004.
Abstract. This paper describes a reactive, distributed layered architecture for cooperation of multiple resource-bounded robots, which is utilized in mobile sensor network coverage. In the upper layer, a dynamic task allocation scheme self-organizes the robot coalitions to track efficiently in separate regions. It uses the concepts of ant behavior to self-regulate the regional distributions of robots in proportion to that of the targets to be tracked in the changing environment. As a result, the adverse effects of task interference between robots are minimized and sensor network coverage is improved. In the lower layer, the robots use self-organizing neural networks to coordinate their target tracking within a region. Quantitative comparisons with other tracking strategies such as static sensor placements, potential fields, and auction-based negotiation show that our approach can provide better coverage and greater flexibility in responding to environmental changes.
PRESENTATIONS
- Task Allocation via Self-Organizing Swarm Coalitions in Distributed Mobile Sensor Network.
Kian Hsiang Low.
Presented in 8th National IT Awareness Project Competition (NITA-04), National University of Singapore, Mar 13, 2004
(Overall Best Project, Postgraduate Category).
VIDEO DEMOS
Coverage of 30 targets (green) with 15 ant robots (white)
- Self-organization of swarm coalitions to unknown, time-varying target distribution after
- Robot switching to region of higher task demand (i.e., targets to robots ratio)
ACTION SELECTION MECHANISM FOR MULTI-ROBOT TASKS
PROJECT DURATION : Sep 2002 - Nov 2002
PROBLEM MOTIVATION
A central issue in the design of behavior-based control architectures for autonomous mobile robots
is the formulation of effective mechanisms to coordinate the behaviors.
These mechanisms determine the policy of conflict resolution between behaviors,
which involves behavioral cooperation and competition to select the most appropriate action.
The actions are selected so as to optimize the achievement of the goals or behavioral objectives.
Developing such an action selection methodology is non-trivial
due to realistic constraints such as environmental complexity and unpredictability,
and resource limitations, which include computational and cognitive capabilities of the robot,
incomplete knowledge of the environment, and time constraints.
As a result, action selection can never be absolutely optimal.
Given these constraints, the action selection scheme should be able to choose actions
that are good enough to satisfy multiple concurrent, possibly conflicting, behavioral objectives.
PROPOSED METHODOLOGY
Our motivation of the action selection mechanism is to develop a motion control strategy for autonomous non-holonomic mobile robots
that can perform distributed multi-robot surveillance in unknown, dynamic, complex, and unpredictable environments.
By implementing the action selection framework using an assemblage of self-organizing neural networks,
it induces the following key features that significantly enhance the agent's action selection capability:
self-organization of continuous state and action spaces to provide smooth, efficient and fine motion control,
and action selection via the cooperation and competition of Extended Kohonen Maps to achieve more complex motion tasks:
(1) negotiation of unforeseen concave and narrowly spaced obstacles, and
(2) cooperative tracking of multiple mobile targets by a team of robots.
Qualitative and quantitative comparisons for single- and multi-robot tasks show that
our framework can provide better action selection than do potential fields method.
PUBLICATIONS
- An Ensemble of Cooperative Extended Kohonen Maps for Complex Robot Motion Tasks.
Kian Hsiang Low, Wee Kheng Leow & Marcelo H. Ang, Jr.
Neural Computation, volume 17, issue 6, pages 1411-1445, Jun 2005.
Extended version of our
IJCAI-03 paper
Abstract. Self-organizing feature maps such as extended Kohonen maps (EKMs) have been very successful at learning sensorimotor control for mobile robot tasks. This letter presents a new ensemble approach, cooperative EKMs with indirect mapping, to achieve complex robot motion. An indirect-mapping EKM self-organizes to map from the sensory input space to the motor control space indirectly via a control parameter space. Quantitative evaluation reveals that indirect mapping can provide finer, smoother, and more efficient motion control than does direct mapping by operating in a continuous, rather than discrete, motor control space. It is also shown to outperform basis function neural networks. Furthermore, training its control parameters with recursive least squares enables faster convergence and better performance compared to gradient descent. The cooperation and competition of multiple self-organized EKMs allow a nonholonomic mobile robot to negotiate unforeseen, concave, closely spaced, and dynamic obstacles. Qualitative and quantitative comparisons with neural network ensembles employing weighted sum reveal that our method can achieve more sophisticated motion tasks even though the weighted-sum ensemble approach also operates in continuous motor control space.
- Continuous-Spaced Action Selection for Single- and Multi-Robot Tasks Using Cooperative Extended Kohonen Maps.
Kian Hsiang Low, Wee Kheng Leow & Marcelo H. Ang, Jr.
In Proceedings of the IEEE International Conference on Networking, Sensing and Control (ICNSC'04)
(Invited Paper to Special Session on Visual Surveillance), pages 198-203, Taipei, Taiwan, Mar 21-23, 2004.
Abstract. Action selection is a central issue in the design of behavior-based control architectures for autonomous mobile robots. This paper presents an action selection framework based on an assemblage of self-organizing neural networks called Cooperative Extended Kohonen Maps. This framework encapsulates two features that significantly enhance a robot's action selection capability: self-organization in the continuous state and action spaces to provide smooth, efficient and fine motion control; action selection via the cooperation and competition of Extended Kohonen Maps so that more complex motion tasks can be achieved. Qualitative and quantitative comparisons for both single- and multi-robot motion tasks show that our framework can provide better action selection than do action superposition methods.
- Action Selection for Single- and Multi-Robot Tasks Using Cooperative Extended Kohonen Maps.
Kian Hsiang Low, Wee Kheng Leow & Marcelo H. Ang, Jr.
In Proceedings of the 18th International Joint Conference on Artificial Intelligence (IJCAI-03), pages 1505-1506, Acapulco, Mexico, Aug 9-15, 2003.
27.6% acceptance rate
Abstract. This paper presents an action selection framework based on an assemblage of self-organizing neural networks called Cooperative Extended Kohonen Maps. This framework encapsulates two features that significantly enhance a robot's action selection capability: self-organization in the continuous state and action spaces to provide smooth, efficient and fine motion control; action selection via the cooperation and competition of Extended Kohonen Maps to achieve more complex motion tasks. Qualitative and quantitative comparisons for single- and multi-robot tasks show our framework can provide better action selection than do potential fields method.
- Action Selection in Continuous State and Action Spaces by Cooperation and Competition of Extended Kohonen Maps.
Kian Hsiang Low, Wee Kheng Leow & Marcelo H. Ang, Jr.
In Proceedings of the
2nd International Joint Conference on Autonomous Agents and MultiAgent Systems (AAMAS-03), pages 1056-1057, Melbourne, Australia, Jul 14-18, 2003.
Abstract. This paper presents an action selection framework based on an assemblage of self-organizing neural networks called Cooperative Extended Kohonen Maps. This framework encapsulates two features that significantly enhance a robot's action selection capability: self-organization in the continuous state and action spaces to provide smooth, efficient and fine motion control; action selection via the cooperation and competition of Extended Kohonen Maps to achieve more complex motion tasks. Qualitative tests demonstrate the capability of our action selection method for both single- and multi-robot motion tasks.
VIDEO DEMO
- Cooperative tracking of moving targets by robots
using cooperative Extended Kohonen Maps
INTEGRATED ROBOT PLANNING AND CONTROL
PROJECT DURATION : Jul 2001 - Sep 2002
PROBLEM MOTIVATION
Robot motion research has proceeded along two separate directions:
high-level deliberative planning and low-level reactive control.
Deliberative planning uses a world model to generate an optimal sequence of collision-free actions
that can achieve a globally specified goal in a complex static environment.
However, in a dynamic environment, unforeseen obstacles may obstruct the action sequence,
and replanning to react to these situations can be too computationally expensive.
On the other hand, reactive control directly couples sensed data to appropriate actions.
It allows the robot to respond robustly and timely to unexpected obstacles and environmental changes
but may be trapped by them.
PROPOSED METHODOLOGY
The problem of goal-directed, collision-free motion in a complex, unpredictable environment can be solved
by tightly integrating high-level deliberative planning with low-level reactive control.
This work presents two such architectures for a nonholonomic mobile robot.
To achieve real-time performance, reactive control capabilities have to be fully realized so that
the deliberative planner can be simplified.
These architectures are enriched with reactive target reaching and obstacle avoidance modules.
Their target reaching modules use indirect-mapping Extended Kohonen Map to provide finer and smoother motion control
than direct-mapping methods.
While one architecture fuses these modules indirectly via command fusion,
the other one couples them directly using cooperative Extended Kohonen Maps,
enabling the robot to negotiate unforeseen concave obstacles.
The planner for both architectures use a slippery cells technique to
decompose the free workspace into fewer cells, thus reducing search time.
Any two points in the cell can still be traversed by reactive motion.
PUBLICATIONS
- Enhancing the Reactive Capabilities of Integrated Planning and Control with Cooperative Extended Kohonen Maps.
Kian Hsiang Low, Wee Kheng Leow & Marcelo H. Ang, Jr.
In Proceedings of the
IEEE International Conference on Robotics and Automation (ICRA'03), pages 3428-3433, Taipei, Taiwan, May 12-17, 2003.
Abstract. Despite the many significant advances made in robot motion research, few works have focused on the tight integration of high-level deliberative planning with reactive control at the lowest level. In particular, the real-time performance of existing integrated planning and control architectures is still not optimal because the reactive control capabilities have not been fully realized. This paper aims to enhance the low-level reactive capabilities of integrated planning and control with Cooperative Extended Kohonen Maps for handling complex, unpredictable environments so that the work-load of the high-level planner can be consequently eased. The enhancements include fine, smooth motion control, execution of more complex motion tasks such as overcoming unforeseen concave obstacles and traversing between closely spaced obstacles, and asynchronous execution of behaviors.
- A Hybrid Mobile Robot Architecture with Integrated Planning and Control.
Kian Hsiang Low, Wee Kheng Leow & Marcelo H. Ang, Jr.
In Proceedings of the
1st International Joint Conference on Autonomous Agents and MultiAgent Systems (AAMAS-02), pages 219-226, Bologna, Italy, Jul 15-19, 2002.
26% acceptance rate
Abstract. Research in the planning and control of mobile robots has received much attention in the past two decades. Two basic approaches have emerged from these research efforts: deliberative vs. reactive. These two approaches can be distinguished by their different usage of sensed data and global knowledge, speed of response, reasoning capability, and complexity of computation. Their strengths are complementary and their weaknesses can be mitigated by combining the two approaches in a hybrid architecture. This paper describes a method for goal-directed, collision-free navigation in unpredictable environments that employs a behavior-based hybrid architecture with asynchronously operating behavioral modules. It differs from existing hybrid architectures in two important ways: (1) the planning module produces a sequence of checkpoints instead of a conventional complete path, and (2) in addition to obstacle avoidance, the reactive module also performs target reaching under the control of a self-organizing neural network. The neural network is trained to perform fine, smooth motor control that moves the robot through the checkpoints. These two aspects facilitate a tight integration between high-level planning and low-level control, which permits real-time performance and easy path modification even when the robot is en route to the goal position.
- Integrated Planning and Control of Mobile Robot with Self-Organizing Neural Network.
Kian Hsiang Low, Wee Kheng Leow & Marcelo H. Ang, Jr.
In Proceedings of the
IEEE International Conference on Robotics and Automation (ICRA'02), pages 3870-3875, Washington, DC, May 11-15, 2002.
Abstract. Despite the many significant advances made in robotics research, few works have focused on the tight integration of task planning and motion control. Most integration works involve the task planner providing discrete commands to the low-level controller, which performs kinematics and control computations to command the motor and joint actuators. This paper presents a framework of the integrated planning and control for mobile robot navigation. Unlike existing integrated approaches, it produces a sequence of checkpoints instead of a complete path at the planning level. At the motion control level, a neural network is trained to perform motor control that moves the robot from one checkpoint to the next. This method allows for a tight integration between high-level planning and low-level control, which permits real-time performance and easy modification of motion path while the robot is enroute to the goal position.
- Integrated Robot Planning and Control with Extended Kohonen Maps.
Kian Hsiang Low.
Master's Thesis, Department of Computer Science, School of Computing, National University of Singapore, Jul 2002.
Singapore Computer Society Prize for best M.Sc. Thesis 2002-2003
Abstract. The problem of goal-directed, collision-free motion in a complex, unpredictable environment can be solved by tightly integrating high-level deliberative planning with low-level reactive control. This thesis presents two such architectures for a nonholonomic mobile robot. To achieve real-time performance, reactive control capabilities have to be fully realized so that the deliberative planner can be simplified. These architectures are enriched with reactive target reaching and obstacle avoidance modules. Their target reaching modules use indirect-mapping Extended Kohonen Map to provide finer and smoother motion control than direct-mapping methods. While one architecture fuses these modules indirectly via command fusion, the other one couples them directly using cooperative Extended Kohonen Maps, enabling the robot to negotiate unforeseen concave obstacles. The planner for both architectures use a slippery cells technique to decompose the free workspace into fewer cells, thus reducing search time. Any two points in the cell can still be traversed by reactive motion.
VIDEO DEMOS
- Robot motion in an environment with unforeseen stationary obstacle
using command fusion
- Robot motion in an environment with unforeseen moving obstacle
using command fusion
- Robot motion in an environment that changes using command
fusion
- Robot motion in an environment with
unforeseen stationary concave and narrowly spaced convex obstacles using cooperative Extended Kohonen Maps
- Robot motion in an environment with
unforeseen moving obstacles using cooperative Extended Kohonen Maps
|