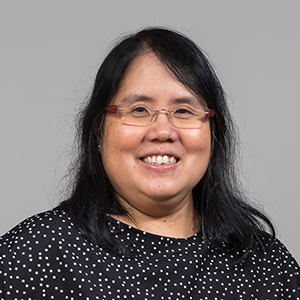
HSU, Wynne
Provost's Chair ProfessorDirector, Institute of Data Science (IDS), NUS
- Ph.D. (Electrical & Computer Engineering, Purdue University, U.S.A)
- M.Sc. (Computer Science, Purdue University, U.S.A)
- B.Sc. (Computer Science, National University of Singapore)
Wynne Hsu received her BSc in Computer Science at National University of Singapore and her M.Sc. and Ph.D in Electrical Engineering from Purdue University, West Lafayette, U.S.A., in 1989 and 1994, respectively. She is currently a Provost's Chair Professor at the Department of Computer Science, School of Computing, National University of Singapore (NUS). Her research interests include: data analytics in the context of social networks, machine learning, as well as retina image analysis.
RESEARCH AREAS
Artificial Intelligence
- Knowledge Representation & Reasoning
- Machine Learning
RESEARCH INTERESTS
AI in Healthcare
Social Media Analytics
Spatio-temporal Data Mining
Retinal Image Analysis
RESEARCH PROJECTS
RESEARCH GROUPS
TEACHING INNOVATIONS
SELECTED PUBLICATIONS
- Wee Yong Lim, Mong Li Lee, Wynne Hsu. iFact: An Interactive Framework to Assess Claims from Tweets, inInternational Conference on Information and Knowledge Management CIKM, Singapore, November 2017.
- Lahari Poddar, Wynne Hsu, Mong Li Lee. Author-aware Aspect Topic Sentiment Model to Retrieve Supporting Opinions from Reviews, inInternational Conference on Empirical Methods in Natural Language Processing EMNLP, Copenhagen, Denmark, September 2017.
- Lahari Poddar, Wynne Hsu, Mong Li Lee. Quantifying Aspect Bias in Ordinal Ratings using a Bayesian Approach, in26th International Joint Conference on Artificial Intelligence IJCAI, Melbourne, Australia, August 2017.
- Furong Li, Mong Li Lee, Wynne Hsu. Profiling Entities over Time in the Presence of Unreliable Sources, inIEEE Transactions on Knowledge and Data Engineering TKDE, 2017.
- Furong Li, Mong Li Lee, Wynne Hsu. MAROON+: A System for Profiling Entities over Time Demo, in33rd IEEE International Conference on Data Engineering ICDE, San Diego, CA, USA, April 2017.
- Chonggang Song, Wynne Hsu, Mong Li Lee. Temporal Influence Blocking: Minimizing the Effect of Misinformation in Social Networks, in33rd IEEE International Conference on Data Engineering ICDE, San Diego, CA, USA, April 2017.
- Chonggang Song, Wynne Hsu, Mong Li Lee. Targeted Influence Maximization in Social Networks, in25th ACM International Conference on Information and Knowledge Management CIKM,Indianapolis, United States, October 2016.
- Tien Yin Wong, Daniel Ting, Gavin Tan, Wynne Hsu, Mong Li Lee, Haslina Hamzah, Gilbert Lim, Rina Rudyanto, Yuan Cheng, Alfred Gan and Carol Y. Cheung. Cloud-based Automated Software for Diabetic Retinopathy Screening and Monitoring in a National Screening Program, in 39th Annual Macula Society Meeting, February 2016.
AWARDS & HONOURS
President’s Technology Award, Singapore, 2014.
SIGKDD Test-of-Time Award, 2014
COURSES TAUGHT
CS2309
CS Research Methodology