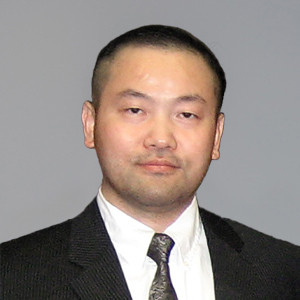

Yang ZHANG
ProfessorDepartment of Computer Science, the School of Computing
Department of Biochemistry, Yong Loo Lin School of Medicine
Cancer Science Institute of Singapore
Dr Yang Zhang is a Professor in the Department of Computer Science at the School of Computing, National University of Singapore (NUS). He also holds joint appointments as a Professor in the Department of Biochemistry at the Yong Loo Lin School of Medicine, NUS, and at the Cancer Science Institute of Singapore. Prior to joining NUS, Dr Zhang was a Professor in the Department of Computational Medicine and Bioinformatics, the Department of Biological Chemistry, and the Department of Macromolecular Science and Engineering at the University of Michigan. His research group, the Zhang Lab, focuses on artificial intelligence and deep neural network learning, protein folding and structure prediction, as well as protein design and engineering. The I-TASSER algorithm (https://zhanggroup.org/I-TASSER/), developed in his laboratory, has been ranked the most accurate method for automated protein structure prediction in the community-wide CASP experiments for nine consecutive rounds since 2006. Dr Zhang has received numerous accolades, including the Alfred P. Sloan Award, the US National Science Foundation CAREER Award, and the University of Michigan Basic Science Research Award. Since 2015, he has been recognised seven times as a Thomson Reuters/Clarivate Analytics Highly Cited Researcher.
RESEARCH AREAS
Computational Biology
- Bioinformatics Algorithms
Artificial Intelligence
- Machine Learning
RESEARCH INTERESTS
AI and deep learning
Protein and RNA structure prediction
Protein and peptide design
AI-based drug discovery
RESEARCH PROJECTS
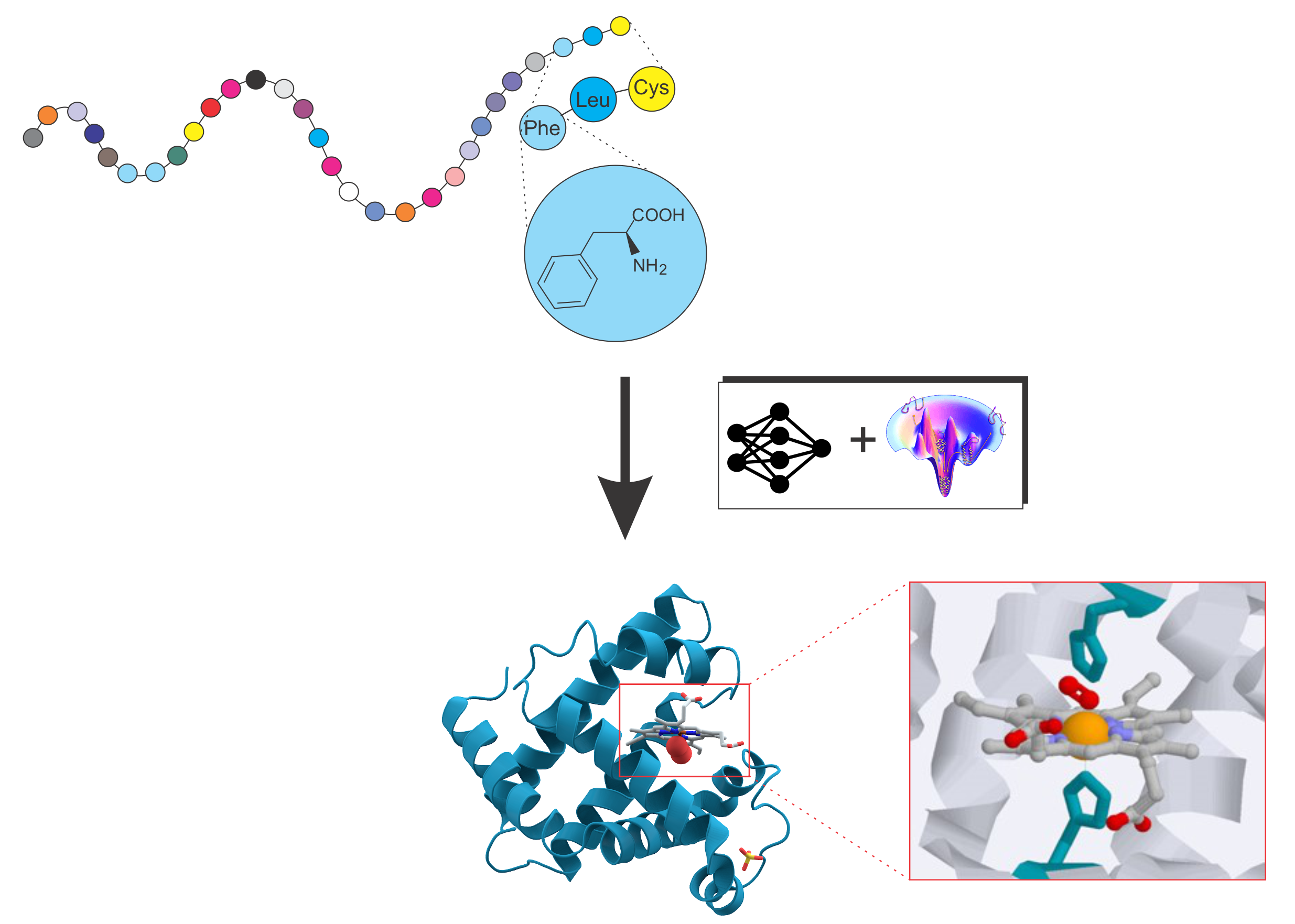
Protein folding and protein structure prediction
Deciphering the structure and function of proteins is pivotal in modern biology and medicine. Our work focuses on pioneering computational methods, integrating advanced AI techniques and physics-based force fields, to precisely model protein structure and function. A key objective is uncovering the fundamental interplay between protein sequence, structure, and function.
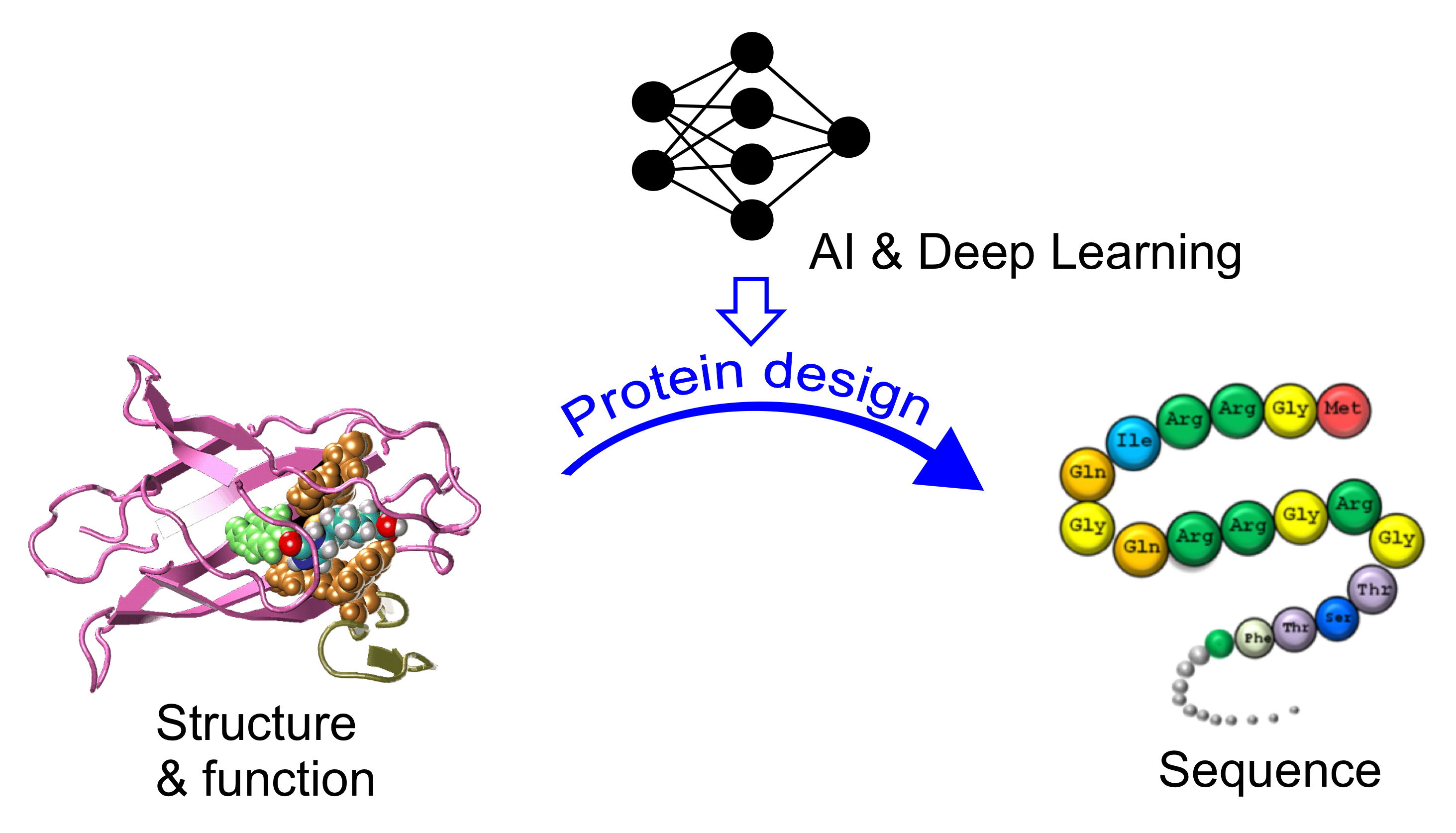
AI-based protein design and drug discovery
Nature proteins exhibit limited structural folds and functions, shaped over billions of years of evolution. This project seeks to leverage AI and deep learning to craft novel protein sequences, surpassing natural constraints. The computationally designed proteins and peptides hold promise as drugs, offering innovative treatments for diverse human diseases, including cancer and Alzheimer's.
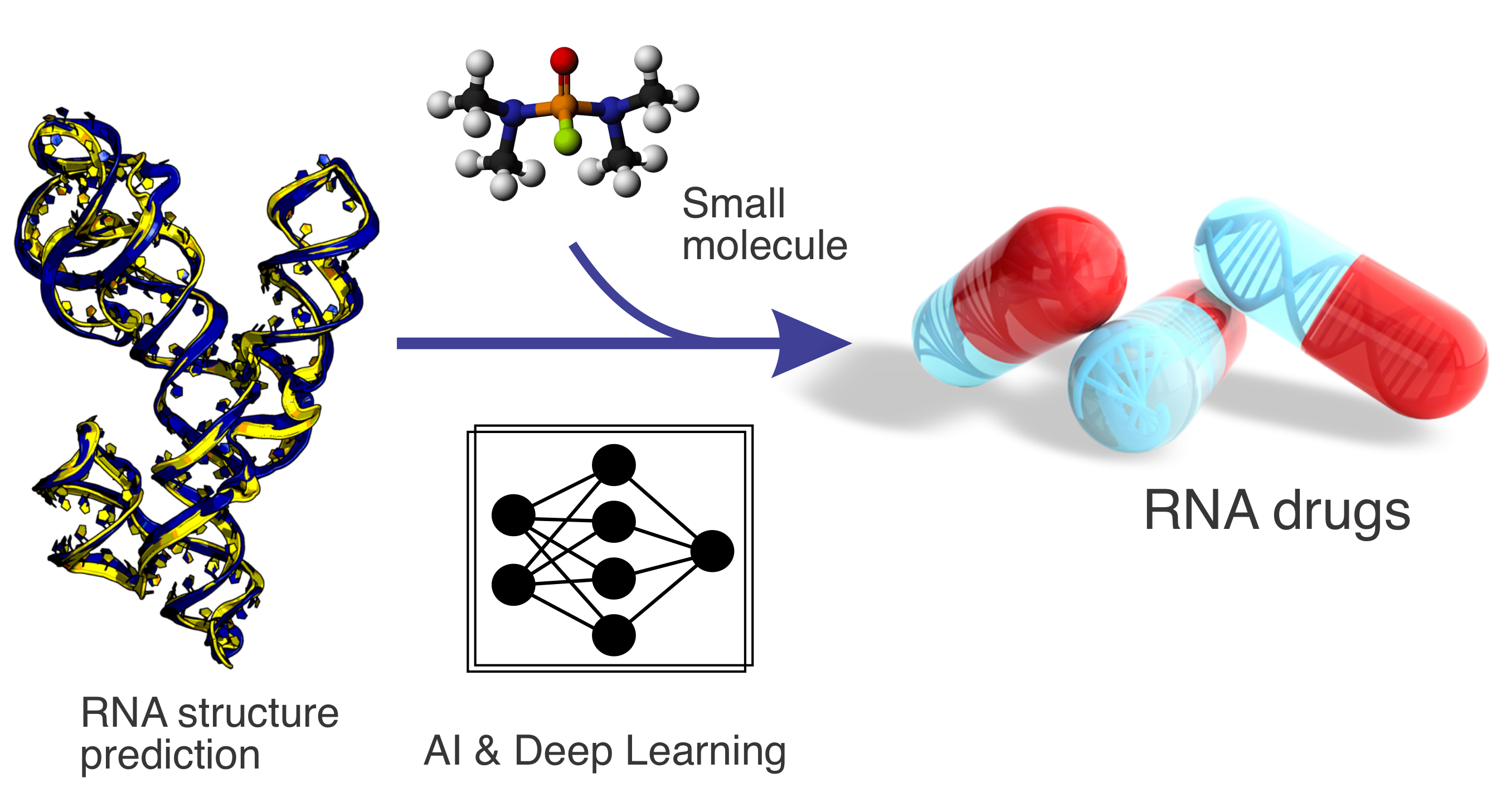
RNA structure prediction and small-molecule drug design
Non-coding RNAs, single-chain nucleotide biomolecules, play crucial roles as regulators of diverse biological processes. Our development of advanced AI and deep-learning algorithms, for precise RNA structure prediction and small molecule interactions, promises over a 10-fold expansion in drug design possibilities, surpassing traditional protein-targeting drug discovery approaches.
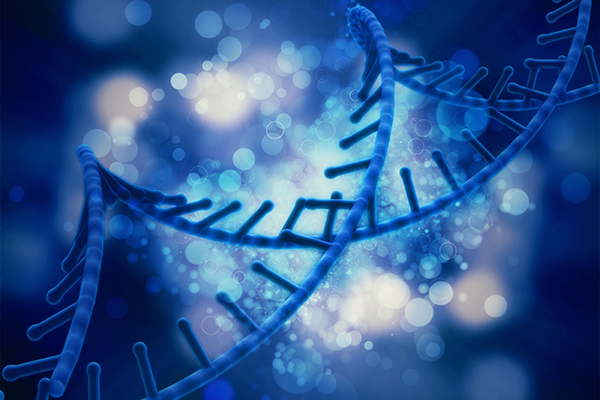
Deep Learning-Based Pipeline for High-Accuracy RNA Structure Prediction
Predicting RNA structures is crucial for drug discovery, but current methods lack accuracy. This project uses AI trained on vast RNA data to create precise 3D models from RNA sequences. This could significantly improve drug development and impact human health.
RESEARCH GROUPS
TEACHING INNOVATIONS
SELECTED PUBLICATIONS
- (A full list of Dr. Yang Zhang's publications can be seen at https://scholar.google.com/citations?user=MtBs-kMAAAAJ)
- W Zheng, Q Wuyun, Y Li, Q Liu, X Zhou, C Peng, Y Zhu, L Freddolino, Y Zhang. Deep-learning-based single-domain and multidomain protein structure prediction with D-I-TASSER. Nature Biotechnology, https://doi.org/10.1038/s41587-025-02654-4 (2025).
- W Zheng, Q Wuyun, Y Li, C Zhang, PL Freddolino, Y Zhang. Improving deep learning protein monomer and complex structure prediction using DeepMSA2 with huge metagenomics data. Nature Methods, 21: 279-289 (2024).
- R Pearce, X Huang, GS Omenn, Y Zhang. De novo protein fold design through sequence-independent fragment assembly simulations. PNAS, 120: e2208275120 (2023).
- Y Li, C Zhang, C Feng, R Pearce, PL Freddolino, Y Zhang. Integrating end-to-end learning with deep geometrical potentials for ab initio RNA structure prediction. Nature Communications, 14: 5745 (2023).
- C Zhang, M Shine, AM Pyle, Y Zhang. US-align: Universal structure alignment of proteins, nucleic acids and macromolecular complexes. Nature Methods, 19: 1109-1115 (2022).
- X Zhou, W Zheng, Y Li, R Pearce, C Zhang, EW Bell, G Zhang, Y Zhang. I-TASSER-MTD: A deep-learning based platform for multi-domain protein structure and function prediction. Nature Protocols, 17: 2326-2353 (2022).
- X Zhou, Y Li, C Zhang, W Zheng, G Zhang, Y Zhang. Progressive assembly of multi-domain protein structures from cryo-EM density maps. Nature Computational Science, 2: 265-275 (2022).
- X Zhang, B Zhang, PL Freddolino, Y Zhang. CR-I-TASSER: Assemble protein structures from cryo-EM density maps using deep convolutional neural networks. Nature Methods, 19: 195-204 (2022).
- SM Mortuza, W Zheng, C Zhang, Y Li, R Pearce, Y Zhang. Improving fragment-based ab initio protein structure assembly using low-accuracy contact-map predictions. Nature Communications, 12: 5011 (2021).
- P Yang, W Zheng, K Ning, Y Zhang. Decoding the link of microbiome niches with homologous sequences enables accurately targeted protein structure prediction. PNAS, 118: e2110828118 (2021).
AWARDS & HONOURS
COURSES TAUGHT