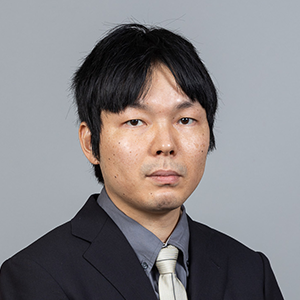
Kenji KAWAGUCHI
NUS Presidential Young Professor- Postdoctoral Fellow, Harvard University
- Ph.D. in Computer Science, MIT
- S.M. in EECS, MIT
Kenji Kawaguchi is a Presidential Young Professor in the Department of Computer Science at NUS. Kenji Kawaguchi received his Ph.D. in Computer Science from the Massachusetts Institute of Technology (MIT). He then joined Harvard University as a postdoctoral fellow. He was also an invited participant at the University of Cambridge, Isaac Newton Institute for Mathematical Sciences programme on "Mathematics of Deep Learning". He was one of 77 invited participants from around the world. His research interests include deep learning, as well as artificial intelligence (AI) in general. His research group aims to have a positive feedback loop between theory and practice in deep learning research through collaborations with researchers from both the practice and theory sides.
RESEARCH AREAS
RESEARCH INTERESTS
Deep Learning
Large Language Models (LLMs)
RESEARCH PROJECTS
RESEARCH GROUPS
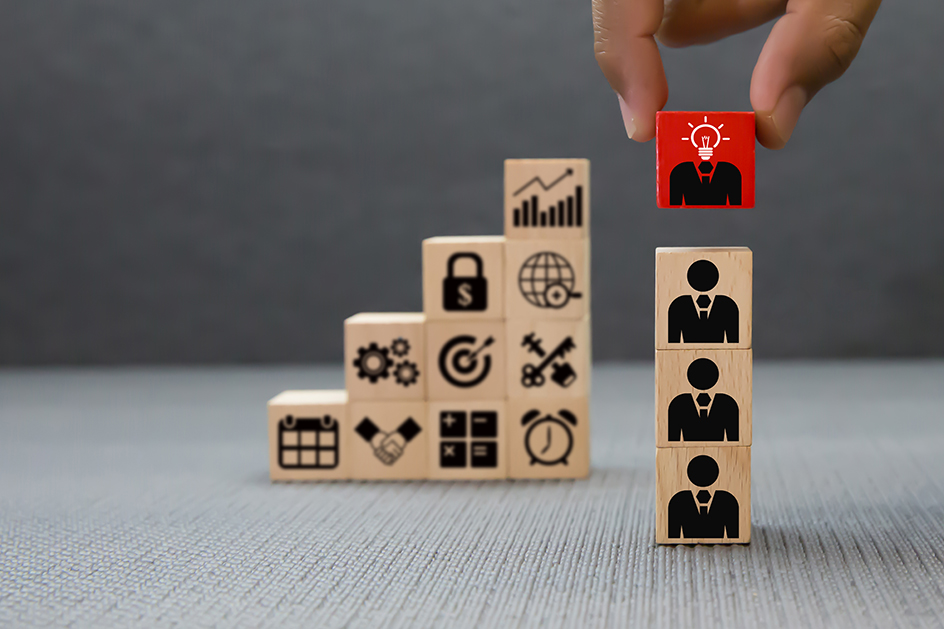
Deep Learning Lab
Our lab aims to contribute to the development of deep learning methods and the understanding of intelligence.
TEACHING INNOVATIONS
SELECTED PUBLICATIONS
- Zekun Shi, Zheyuan Hu, Min Lin and Kenji Kawaguchi. Stochastic Taylor Derivative Estimator: Efficient amortization for arbitrary differential operators. In Advances in Neural Information Processing Systems (NeurIPS), 2024. [Received the Best Paper Award at NeurIPS 2024].
- Qianli Shen, Yezhen Wang, Zhouhao Yang, Xiang Li, Haonan Wang, Yang Zhang, Jonathan Scarlett, Zhanxing Zhu and Kenji Kawaguchi. Memory-Efficient Gradient Unrolling for Large-Scale Bi-level Optimization. In Advances in Neural Information Processing Systems (NeurIPS), 2024.
- Yiran Zhao, Wenyue Zheng, Tianle Cai, Do Xuan Long, Kenji Kawaguchi, Anirudh Goyal and Michael Shieh. Accelerating Greedy Coordinate Gradient and General Prompt Optimization via Probe Sampling. In Advances in Neural Information Processing Systems (NeurIPS), 2024.
- Yiran Zhao, Wenxuan Zhang, Guizhen Chen, Kenji Kawaguchi and Lidong Bing. How do Large Language Models Handle Multilingualism?. In Advances in Neural Information Processing Systems (NeurIPS), 2024.
- Haonan Wang, Qianli Shen, Yao Tong, Yang Zhang and Kenji Kawaguchi. The Stronger the Diffusion Model, the Easier the Backdoor: Data Poisoning to Induce Copyright Breaches Without Adjusting Finetuning Pipeline. International Conference on Machine Learning (ICML), 2024. [ICML oral presentation]
- Brian K Chen, Tianyang Hu, Hui Jin, Hwee Kuan Lee and Kenji Kawaguchi. Exact Conversion of In-Context Learning to Model Weights in Linearized-Attention Transformers. International Conference on Machine Learning (ICML), 2024.
- Md Rifat Arefin, Yan Zhang, Aristide Baratin, Francesco Locatello, Irina Rish, Dianbo Liu and Kenji Kawaguchi. Unsupervised Concept Discovery Mitigates Spurious Correlations. International Conference on Machine Learning (ICML), 2024.
- Jiajun Ma, Shuchen Xue, Tianyang Hu, Wenjia Wang, Zhaoqiang Liu, Zhenguo Li, Zhi-Ming Ma and Kenji Kawaguchi. The Surprising Effectiveness of Skip-Tuning in Diffusion Sampling. International Conference on Machine Learning (ICML), 2024.
- Chang Chen, Junyeob Baek, Fei Deng, Kenji Kawaguchi, Caglar Gulcehre and Sungjin Ahn. PlanDQ: Hierarchical Plan Orchestration via D-Conductor and Q-Performer. International Conference on Machine Learning (ICML), 2024.
- Seul Lee, Seanie Lee, Kenji Kawaguchi and Sung Ju Hwang. Drug Discovery with Dynamic Goal-aware Fragments. International Conference on Machine Learning (ICML), 2024.
- Shihao Zhang, Kenji Kawaguchi and Angela Yao. Deep Regression Representation Learning with Topology. International Conference on Machine Learning (ICML), 2024.
- Xiang Li, Qianli Shen and Kenji Kawaguchi. VA3: Virtually Assured Amplification Attack on Probabilistic Copyright Protection for Text-to-Image Generative Models. Computer Vision and Pattern Recognition Conference (CVPR), 2024.
- Sihang Li, Zhiyuan Liu, Yanchen Luo, Xiang Wang, Xiangnan He, Kenji Kawaguchi, Tat-Seng Chua and Qi Tian. Towards 3D Molecule-Text Interpretation in Language Models. In International Conference on Learning Representations (ICLR), 2024.
- Chang Chen, Fei Deng, Kenji Kawaguchi, Caglar Gulcehre and Sungjin Ahn. Simple Hierarchical Planning with Diffusion. In International Conference on Learning Representations (ICLR), 2024.
- Yingtian Zou, Kenji Kawaguchi, Yingnan Liu, Jiashuo Liu, Mong-Li Lee and Wynne Hsu. Towards Robust Out-of-Distribution Generalization Bounds via Sharpness. In International Conference on Learning Representations (ICLR), 2024.
- Juncheng Liu, Bryan Hooi, Kenji Kawaguchi, Yiwei Wang, Chaosheng Dong and Xiaokui Xiao. Scalable and Effective Implicit Graph Neural Networks on Large Graphs. In International Conference on Learning Representations (ICLR), 2024.
- Dong Bok Lee, Seanie Lee, Joonho Ko, Kenji Kawaguchi, Juho Lee and Sung Ju Hwang. Self-Supervised Dataset Distillation for Transfer Learning. In International Conference on Learning Representations (ICLR), 2024.
- Xuan Long Do, Yiran Zhao, Hannah Brown, Yuxi Xie, James Xu Zhao, Nancy F Chen, Kenji Kawaguchi, Michael Qizhe Xie and Junxian He. Prompt Optimization via Adversarial In-Context Learning. Annual Meeting of the Association for Computational Linguistics (ACL), 2024.
- Zhiyuan Liu, An Zhang, Hao Fei, Enzhi Zhang, Xiang Wang, Kenji Kawaguchi and Tat-Seng Chua. ProtT3: Protein-to-Text Generation for Text-based Protein Understanding. Annual Meeting of the Association for Computational Linguistics (ACL), 2024.
- Zhiyuan Liu, Yaorui Shi, An Zhang, Sihang Li, Enzhi Zhang, Xiang Wang, Kenji Kawaguchi and Tat-Seng Chua. ReactXT: Understanding Molecular "Reaction-ship" via Reaction-Contextualized Molecule-Text Pretraining. In Findings of the Association for Computational Linguistics (Findings of ACL), 2024.
- Yang Zhang, Teoh Tze Tzun, Lim Wei Hern, Tiviatis Sim and Kenji Kawaguchi. Enhancing Semantic Fidelity in Text-to-Image Synthesis: Attention Regulation in Diffusion Models. European Conference on Computer Vision (ECCV), 2024.
- Kenji Kawaguchi*, Zhun Deng*, Xu Ji* and Jiaoyang Huang. How Does Information Bottleneck Help Deep Learning? International Conference on Machine Learning (ICML), 2023.
- Yingtian Zou*, Vikas Verma*, Sarthak Mittal, Wai Hoh Tang, Hieu Pham, Juho Kannala, Yoshua Bengio, Arno Solin, Kenji Kawaguchi. MixupE: Understanding and improving mixup from directional derivative perspective. Uncertainty in Artificial Intelligence (UAI), 2023. [Received the Best Student Paper Award of UAI 2023]
- Qianli Shen, Wai Hoh Tang, Zhun Deng, Apostolos Psaros, Kenji Kawaguchi. PICProp: Physics-Informed Confidence Propagation for Uncertainty Quantification. In Advances in Neural Information Processing Systems (NeurIPS), 2023.
- Yuxi Xie, Kenji Kawaguchi, Yiran Zhao, Xu Zhao, Min-Yen Kan, Junxian He, Qizhe Xie. Decomposition Enhances Reasoning via Self-Evaluation Guided Decoding. In Advances in Neural Information Processing Systems (NeurIPS), 2023.
- Zhiyuan Liu, Yaorui Shi, An Zhang, Enzhi Zhang, Kenji Kawaguchi, Xiang Wang, Tat-Seng Chua. Rethinking Tokenizer and Decoder in Masked Graph Modeling for Molecules. In Advances in Neural Information Processing Systems (NeurIPS), 2023.
- Minki Kang, Seanie Lee, Jinheon Baek, Kenji Kawaguchi, Sung Ju Hwang. Knowledge-Augmented Reasoning Distillation for Small Language Models in Knowledge-Intensive Tasks. In Advances in Neural Information Processing Systems (NeurIPS), 2023.
- Ravid Shwartz-Ziv, Randall Balestriero, Kenji Kawaguchi, Tim G. J. Rudner, Yann LeCun. An Information Theory Perspective on Variance-Invariance-Covariance Regularization. In Advances in Neural Information Processing Systems (NeurIPS), 2023.
- Dianbo Liu, Moksh Jain, Bonaventure F. P. Dossou, Qianli Shen, Salem Lahlou, Anirudh Goyal, Nikolay Malkin, Chris Chinenye Emezue, Dinghuai Zhang, Nadhir Hassen, Xu Ji, Kenji Kawaguchi and Yoshua Bengio. GFlowOut: Dropout with Generative Flow Networks. International Conference on Machine Learning (ICML), 2023.
- Frederik Träuble, Anirudh Goyal, Nasim Rahaman, Michael Curtis Mozer, Kenji Kawaguchi, Yoshua Bengio and Bernhard Schölkopf. Discrete Key-Value Bottleneck. International Conference on Machine Learning (ICML), 2023.
- Jeffrey Willette, Seanie Lee, Bruno Andreis, Kenji Kawaguchi, Juho Lee and Sung Ju Hwang. Scalable Set Encoding with Universal Mini-Batch Consistency and Unbiased Full Set Gradient Approximation. International Conference on Machine Learning (ICML), 2023.
- Aviv Shamsian, Aviv Navon, Neta Glazer, Kenji Kawaguchi, Gal Chechik and Ethan Fetaya. Auxiliary Learning as an Asymmetric Bargaining Game. International Conference on Machine Learning (ICML), 2023.
- Seanie Lee, Minki Kang, Juho Lee, Sung Ju Hwang and Kenji Kawaguchi. Self-Distillation for Further Pre-training of Transformers. In International Conference on Learning Representations (ICLR), 2023.
- Samuel Lavoie, Christos Tsirigotis, Max Schwarzer, Ankit Vani, Michael Noukhovitch, Kenji Kawaguchi and Aaron Courville. Simplicial Embeddings in Self-Supervised Learning and Downstream Classification. In International Conference on Learning Representations (ICLR), 2023. [notable-top-25%]
- Tianbo Li, Min Lin, Zheyuan Hu, Kunhao Zheng, Giovanni Vignale, Kenji Kawaguchi, A.H. Castro Neto, Kostya S. Novoselov and Shuicheng YAN. D4FT: A Deep Learning Approach to Kohn-Sham Density Functional Theory. In International Conference on Learning Representations (ICLR), 2023. [notable-top-25%]
- Dong Bok Lee, Seanie Lee, Kenji Kawaguchi, Yunji Kim, Jihwan Bang, Jung-Woo Ha and Sung Ju Hwang. Self-Supervised Set Representation Learning for Unsupervised Meta-Learning. In International Conference on Learning Representations (ICLR), 2023.
- Zhiyuan Liu, Sihang Li, Yanchen Luo, Hao Fei, Yixin Cao, Kenji Kawaguchi, Xiang Wang, Tat-Seng Chua. MolCA: Molecular Graph-Language Modeling with Cross-Modal Projector and Uni-Modal Adapter. In Empirical Methods in Natural Language Processing (EMNLP), 2023.
- Xu Zhao, Yuxi Xie, Kenji Kawaguchi, Junxian He, Qizhe Xie. Automatic Model Selection with Large Language Models for Reasoning. In Findings of Empirical Methods in Natural Language Processing (Findings of EMNLP), 2023.
- Kenji Kawaguchi, Zhun Deng, Kyle Luh, Jiaoyang Huang. Robustness Implies Generalization via Data-Dependent Generalization Bounds. International Conference on Machine Learning (ICML), 2022. [Selected for ICML long presentation (2% accept rate)]
- Aviv Navon, Aviv Shamsian, Idan Achituve, Haggai Maron, Kenji Kawaguchi, Gal Chechik, Ethan Fetaya. Multi-Task Learning as a Bargaining Game. International Conference on Machine Learning (ICML), 2022.
- Linjun Zhang*, Zhun Deng*, Kenji Kawaguchi, James Zou. When and How Mixup Improves Calibration. International Conference on Machine Learning (ICML), 2022.
- Juncheng Liu, Bryan Hooi, Kenji Kawaguchi and Xiaokui Xiao. MGNNI: Multiscale Graph Neural Networks with Implicit Layers. Advances in Neural Information Processing Systems (NeurIPS), 2022.
- Riashat Islam, Hongyu Zang, Anirudh Goyal, Alex Lamb, Kenji Kawaguchi, Xin Li, Romain Laroche, Yoshua Bengio and Remi Tachet des Combes. Discrete Compositional Representations as an Abstraction for Goal Conditioned Reinforcement Learning. Advances in Neural Information Processing Systems (NeurIPS), 2022.
- Seanie Lee*, Bruno Andreis*, Kenji Kawaguchi, Juho Lee and Sung Ju Hwang. Set-based Meta-Interpolation for Few-Task Meta-Learning. Advances in Neural Information Processing Systems (NeurIPS), 2022.
- Zheyuan Hu, Ameya Jagtap, George Em Karniadakis and Kenji Kawaguchi. When Do Extended Physics-Informed Neural Networks (XPINNs) Improve Generalization?. SIAM Journal on Scientific Computing, 44 (5), pp. A3158-A3182, 2022.
- Kenji Kawaguchi. On the Theory of Implicit Deep Learning: Global Convergence with Implicit Layers. In International Conference on Learning Representations (ICLR), 2021. [Selected for ICLR Spotlight (5% accept rate)]
- Linjun Zhang*, Zhun Deng*, Kenji Kawaguchi*, Amirata Ghorbani and James Zou. How Does Mixup Help With Robustness and Generalization? In International Conference on Learning Representations (ICLR), 2021. [Selected for ICLR Spotlight (5% accept rate)]
- Keyulu Xu*, Mozhi Zhang, Stefanie Jegelka and Kenji Kawaguchi*. Optimization of Graph Neural Networks: Implicit Acceleration by Skip Connections and More Depth. International Conference on Machine Learning (ICML), 2021.
- Vikas Verma, Minh-Thang Luong, Kenji Kawaguchi, Hieu Pham and Quoc V Le. Towards Domain-Agnostic Contrastive Learning. International Conference on Machine Learning (ICML), 2021.
- Dianbo Liu*, Alex Lamb*, Kenji Kawaguchi, Anirudh Goyal, Chen Sun, Michael Curtis Mozer and Yoshua Bengio. Discrete-Valued Neural Communication. Advances in Neural Information Processing Systems (NeurIPS), 2021.
- Ferran Alet*, Dylan Doblar*, Allan Zhou, Joshua B. Tenenbaum, Kenji Kawaguchi and Chelsea Finn. Noether Networks: meta-learning useful conserved quantities. Advances in Neural Information Processing Systems (NeurIPS), 2021.
- Zhun Deng, Linjun Zhang, Kailas Vodrahalli, Kenji Kawaguchi and James Zou. Adversarial Training Helps Transfer Learning via Better Representations. Advances in Neural Information Processing Systems (NeurIPS), 2021.
- Clement Gehring, Kenji Kawaguchi, Jiaoyang Huang, and Leslie Pack Kaelbling. Understanding End-to-End Model-Based Reinforcement Learning Methods as Implicit Parameterization. Advances in Neural Information Processing Systems (NeurIPS), 2021.
- Ferran Alet, Maria Bauza Villalonga, Kenji Kawaguchi, Nurullah Giray Kuru, Tomas Lozano-Perez and Leslie Pack Kaelbling. Tailoring: encoding inductive biases by optimizing unsupervised objectives at prediction time. Advances in Neural Information Processing Systems (NeurIPS), 2021.
- Juncheng Liu, Kenji Kawaguchi, Bryan Hooi, Yiwei Wang and Xiaokui Xiao. EIGNN: Efficient Infinite-Depth Graph Neural Networks. Advances in Neural Information Processing Systems (NeurIPS), 2021.
- Ameya D. Jagtap, Kenji Kawaguchi and George E. Karniadakis. Adaptive Activation Functions Accelerate Convergence in Deep and Physics-informed Neural Networks. Journal of Computational Physics, 404, 109136, 2020.
- Ameya D. Jagtap*, Kenji Kawaguchi* and George E. Karniadakis. Locally adaptive activation functions with slope recovery for deep and physics-informed neural networks. Proceedings of the Royal Society A, 476, 20200334, 2020.
- Kenji Kawaguchi. Deep Learning without Poor Local Minima. Advances in Neural Information Processing (NeurIPS), 2016. [Selected for NeurIPS oral presentation (2% accept rate)]
- Kenji Kawaguchi, Leslie Pack Kaelbling and Tomas Lozano-Perez. Bayesian Optimization with Exponential Convergence. Advances in Neural Information Processing (NeurIPS), 2015.
AWARDS & HONOURS
COURSES TAUGHT