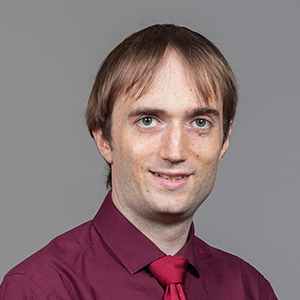
Jonathan Mark SCARLETT
Associate ProfessorAssistant Dean, Graduate Studies
Department of Mathematics, National University of Singapore
Institute for Data Science, National University of Singapore
- Ph.D. (Information Engineering, University of Cambridge, 2014)
- B.Eng. (Electrical Engineering, University of Melbourne, 2010)
- B.Sci. (Computer Science, University of Melbourne, 2010)
Jonathan is an assistant professor jointly in the Department of Computer Science, Department of Mathematics, and Institute of Data Science, National University of Singapore. His research interests are in the areas of information theory, machine learning, and high-dimensional statistics. In 2010, Jonathan received the B.Eng. degree in electrical engineering and the B.Sci. degree in computer science from the University of Melbourne, Australia. From October 2011 to August 2014, he was a Ph.D. student in the Signal Processing and Communications Group at the University of Cambridge, United Kingdom. From September 2014 to September 2017, he was a post-doctoral researcher with the Laboratory for Information and Inference Systems at the École Polytechnique Fédérale de Lausanne (EPFL), Switzerland. He is a recipient of the Singapore National Research Foundation (NRF) fellowship, and the NUS Presidential Young Professorship.
RESEARCH AREAS
RESEARCH INTERESTS
Machine Learning
Information Theory
High-Dimensional Statistics
Bayesian Optimization
Group Testing
RESEARCH PROJECTS
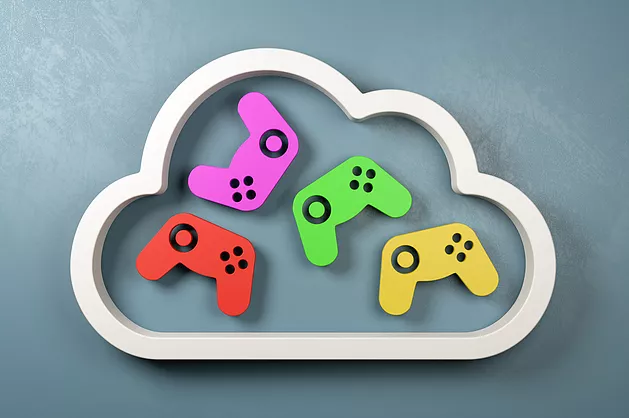
Safety and Reliability in Black-Box Optimization
This project seeks to enhance safety, reliability, and robustness in black-box optimization, exploring new function structures and addressing limitations. This includes extending decision-making frameworks to grey-box settings and multi-agent learning, utilizing a methodology blending theoretical analyses and algorithm development.
RESEARCH GROUPS
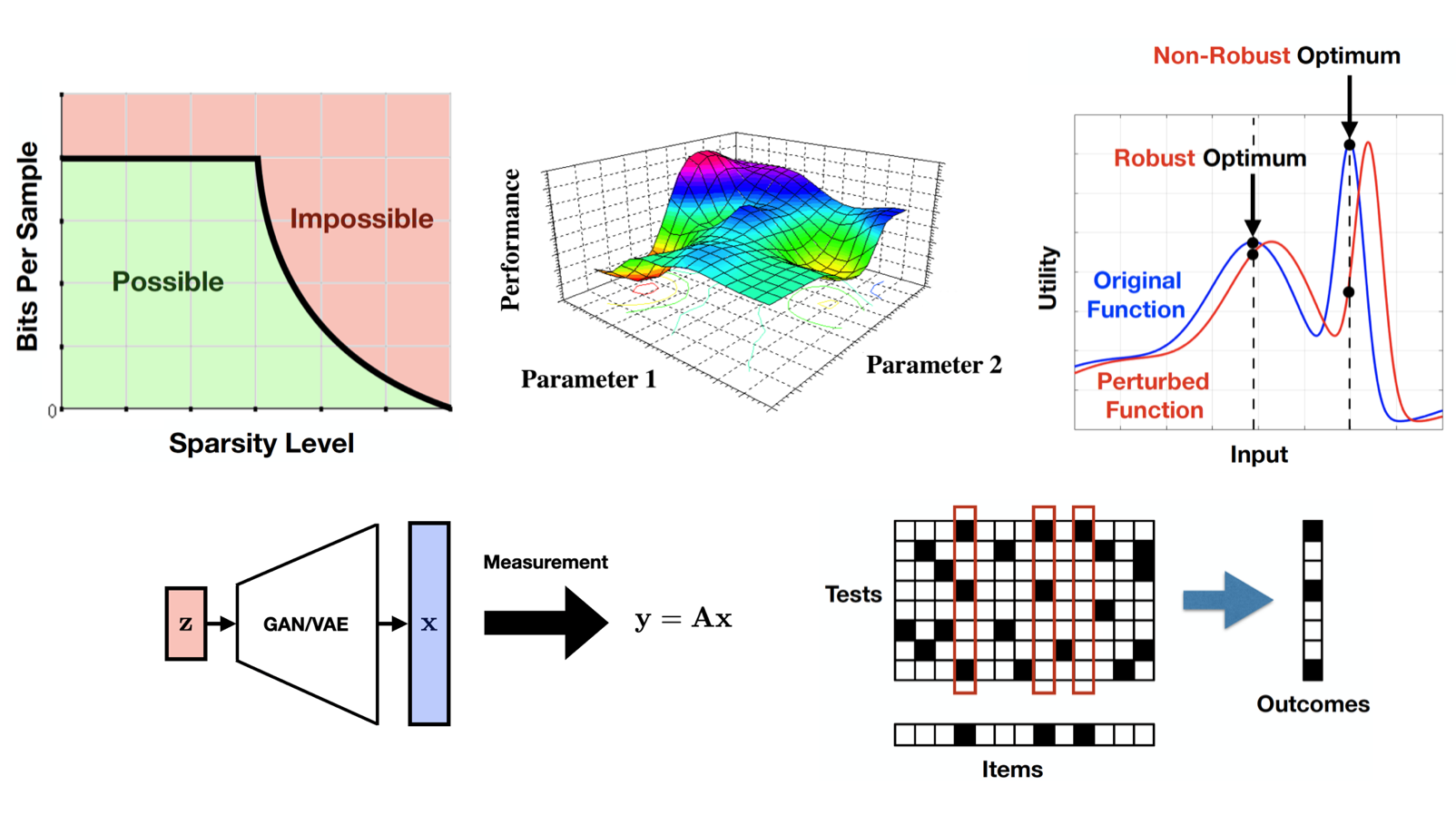
Information Theory and Statistical Learning Group
Our group performs research at the intersection of information theory, machine learning, and high-dimensional statistics, with ongoing areas of interest including information-theoretic limits of learning, adaptive decision-making under uncertainty, scalable algorithms for large-scale inference and learning, and robustness considerations in machine learning.
TEACHING INNOVATIONS
SELECTED PUBLICATIONS
- Matthew Aldridge, Oliver Johnson, and Jonathan Scarlett, "Group testing: An information theory perspective,"Foundations and Trends in Communications and Information Theory, Volume 15, Issue 3-4, pp. 196-392, Dec. 2019.
- Jonathan Scarlett, "Tight regret bounds for Bayesian optimization in one dimension,"International Conference on Machine Learning ICML, 2018.
- Ilija Bogunovic, Jonathan Scarlett, Stefanie Jegelka, and Volkan Cevher, "Adversarially robust optimization with Gaussian processes,"Conference on Neural Information Processing Systems NeurIPS, 2018.
- Jonathan Scarlett, Ilija Bogunovic, and Volkan Cevher, “Lower bounds on regret for noisy Gaussian process bandit optimization,” Conference on Learning Theory COLT, Amsterdam, 2017.
- Jonathan Scarlett, "Noisy adaptive group testing: Bounds and algorithms," IEEE Transactions on Information Theory, Volume 65, Issue 6, pp. 3646-3661, June 2019.
AWARDS & HONOURS
Singapore National Research Foundation (NRF) Fellowship
NUS Early Career Research Award
COURSES TAUGHT