Computational Biology
The modern biology researcher faces vast amounts of data produced by high-throughput analytical technologies for DNA, RNA, and proteins.
This rich and complex mix of data is also confounded by a variety of biological and nonbiological factors, which makes it difficult, inefficient, and inaccurate to draw the right research conclusions.
By understanding and exploiting properties of the underlying biology, instruments, technologies, and experiment designs, we develop advanced methods to process and analyse these large amounts of complex, biological data – which help solve problems in biology, biotechnology, and medicine.
What We Do
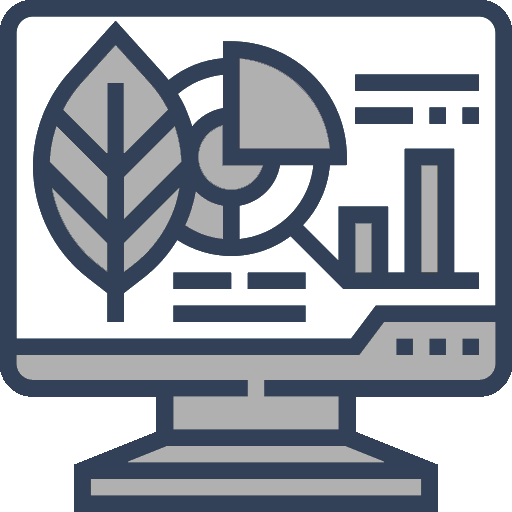
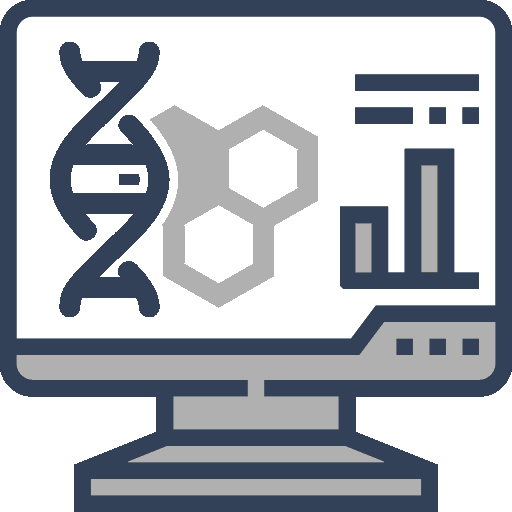
Sub Areas
- Bioinformatics Algorithms
- Omics Data Analysis
- Learning Theory
- Machine Learning
Our Research Projects
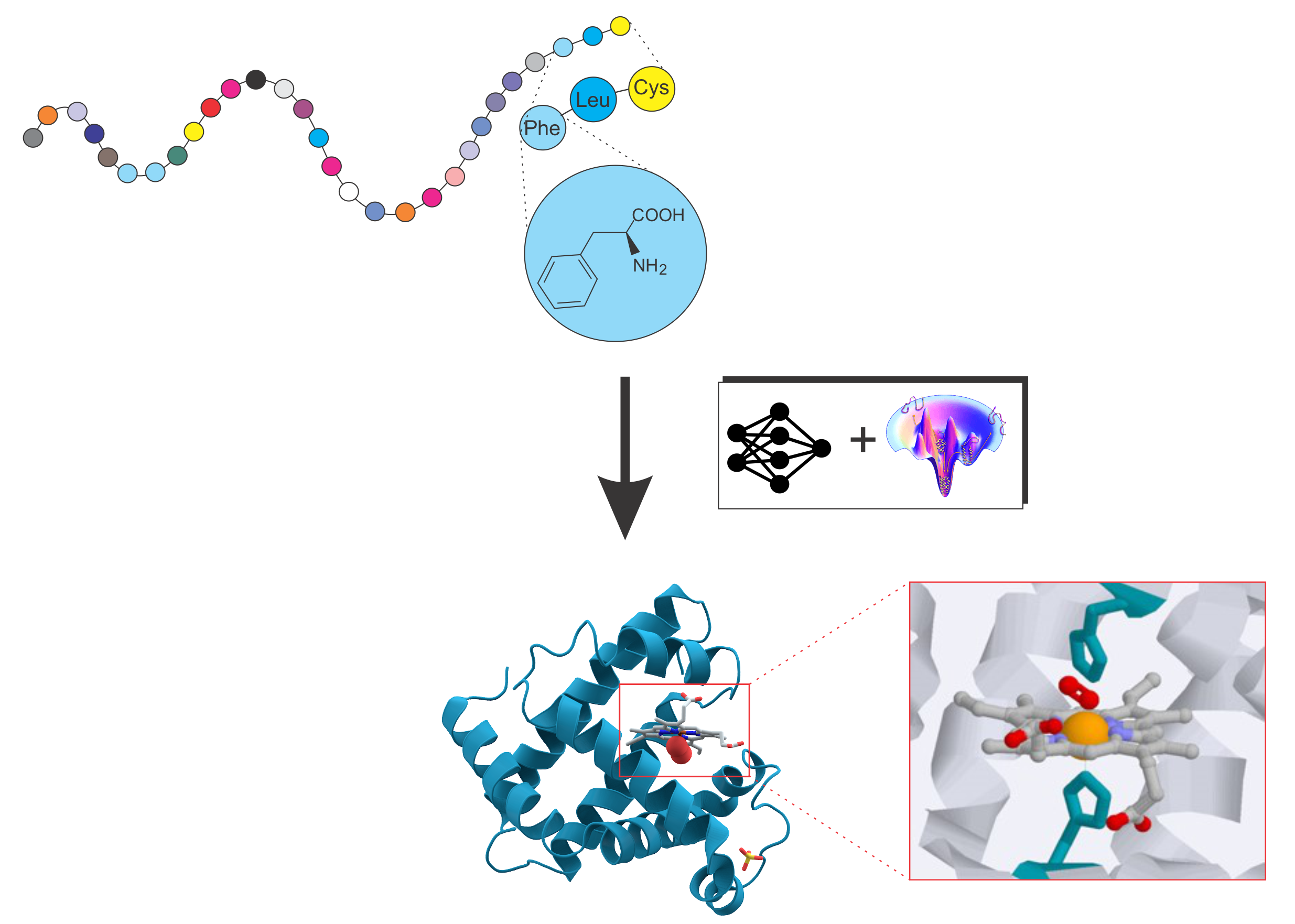
Protein folding and protein structure prediction
Deciphering the structure and function of proteins is pivotal in modern biology and medicine. Our work focuses on pioneering computational methods, integrating advanced AI techniques and physics-based force fields, to precisely model protein structure and function. A key objective is uncovering the fundamental interplay between protein sequence, structure, and function.
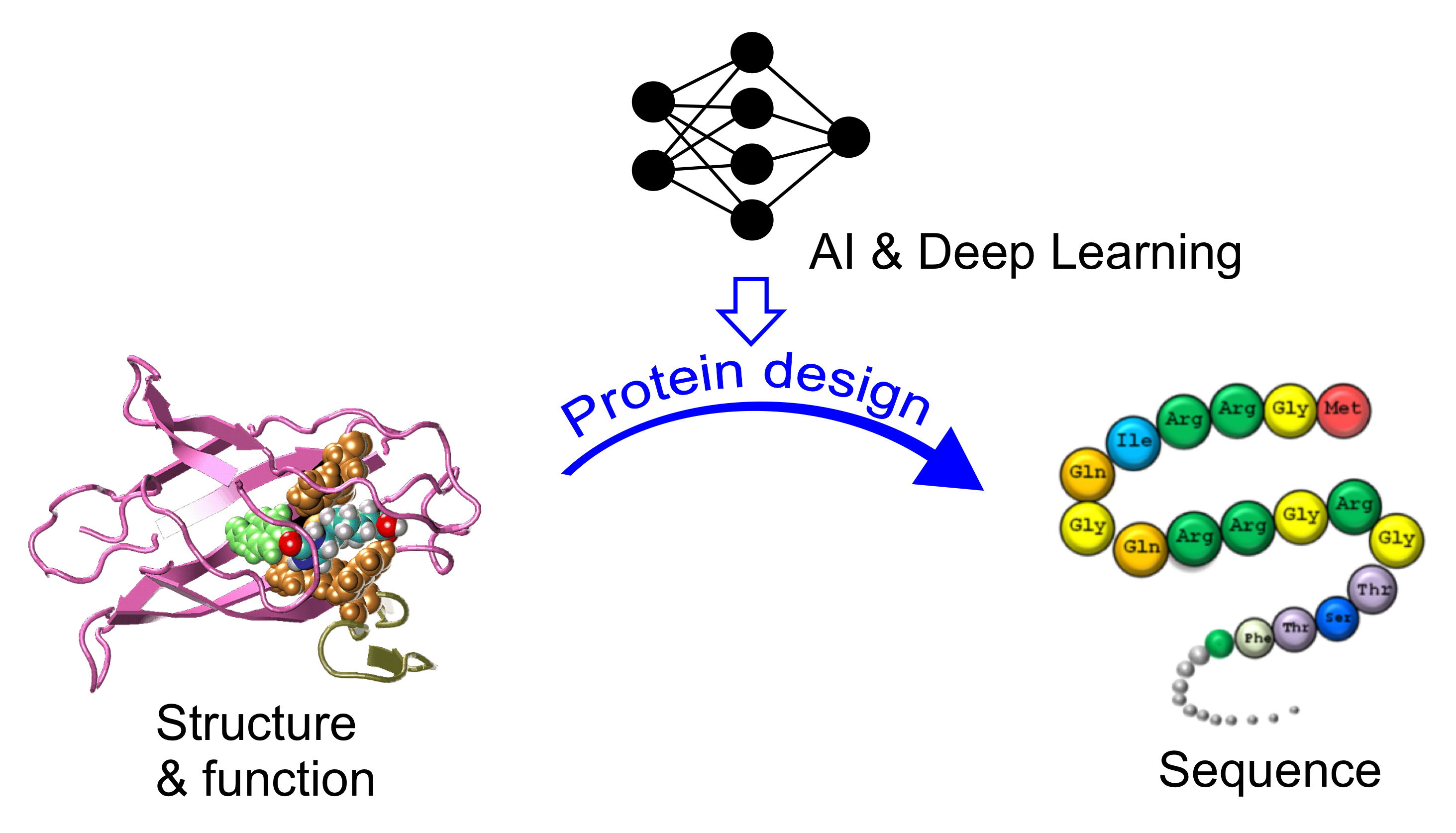
AI-based protein design and drug discovery
Nature proteins exhibit limited structural folds and functions, shaped over billions of years of evolution. This project seeks to leverage AI and deep learning to craft novel protein sequences, surpassing natural constraints. The computationally designed proteins and peptides hold promise as drugs, offering innovative treatments for diverse human diseases, including cancer and Alzheimer's.
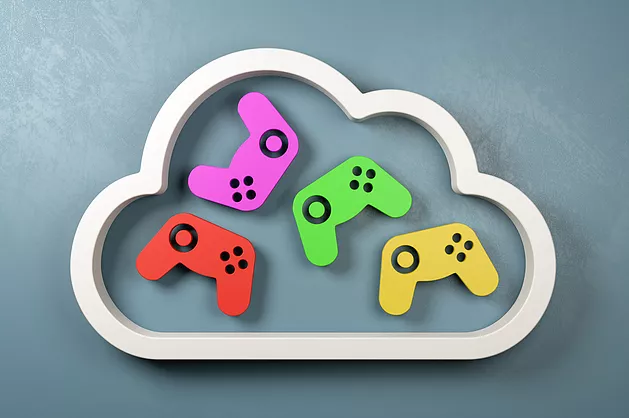
Enabling more sophisticated proteomic profile analysis
Quantitative comparison of samples is central to proteomics. However, biomarkers identified in one batch are quite often not consistent and not reproducible in another batch of samples. We developed techniques based on biological networks to more reproducibly and consistently identify biomarkers and achieve more reliable proteomic-based diagnosis.
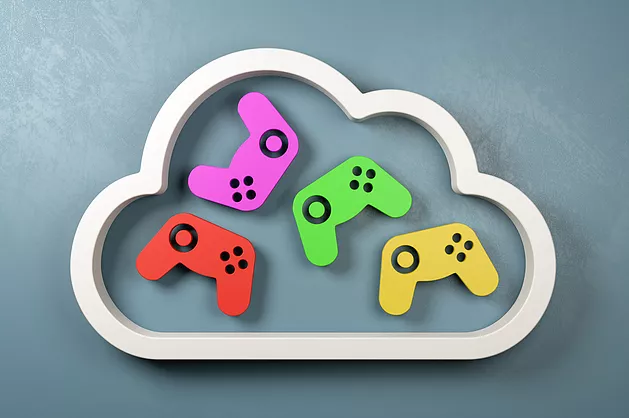
From iteration on multiple collections in synchrony to fast general interval joins
Synchrony iterator captures a programming pattern for synchronized iterations. It is a conservative extension that enhances the repertoire of algorithms expressible in comprehension syntax. In particular, efficient general synchronized iterations, e.g. linear-time algorithms for low-selectivity database non-equijoins, become expressible naturally in comprehensinon syntax.
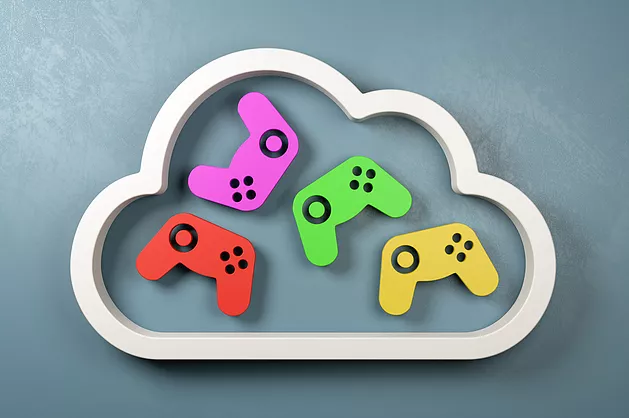
Recovering missing proteins based on biological complexes
We propose a novel ranking strategy for missing-protein recovery based on protein complexes. We postulate that protein complexes provide a good context for making inference of a protein's presence and its abundance. Notably, it is applicable for predicting whether a protein is present even when there is only one sample.
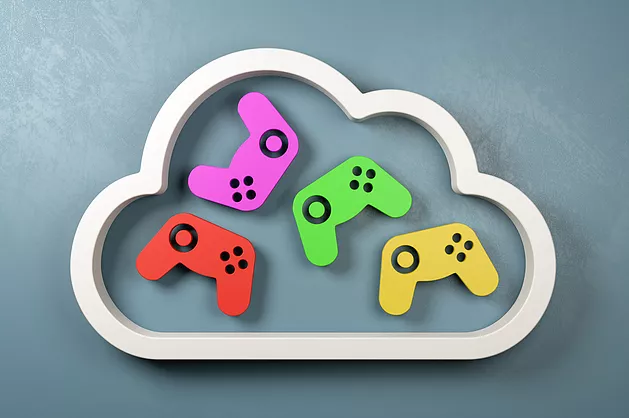
Dealing with confounders in omics analysis
Universality and reproducibility problems are commonly encountered in analyzing omics data due to etiology and human variability, but also batch effects, poor experiment design, inappropriate sample size, and misapplied statistics. Here, we explore a deeper rethink on the mechanics of applying statistical tests, and design analysis techniques that are robust on omics data.
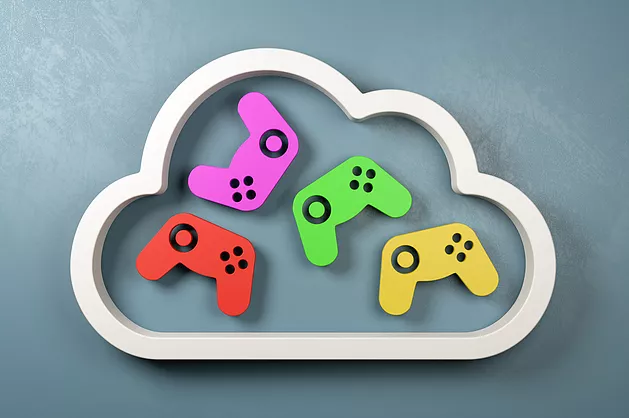
Transcription factor interaction prediction and classification
Regulatory mechanisms often involve several transcription factors (TF), binding together and attaching to the DNA as a single complex. But only a fraction of the regulation partners of each TF is currently known. We developed techniques for predicting the physical interaction between TFs, as well as for predicting the nature of their interactions (i.e. co-operative, competitive, or others).