Artificial Intelligence
As advances in machine learning have grown exponentially in recent years, artificial intelligence has become one of the fastest-moving fields in computer science.
From robot vacuum cleaners to machine learning augmented online shopping recommendations and search engine predictions, our work drives innovations that are now part of our everyday lives.
What We Do
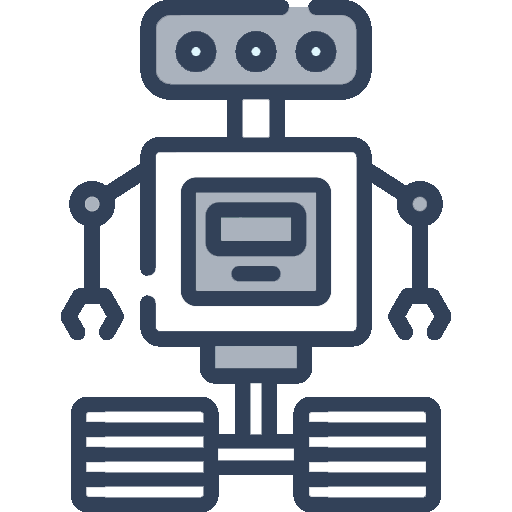
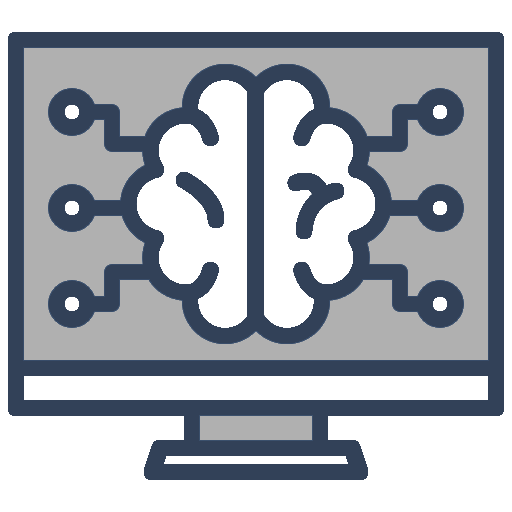
Sub Areas
- Computer Vision
- Decision Making & Planning
- Knowledge Representation & Reasoning
- Learning Theory
- Machine Learning
- Multi-Agent Systems & Algorithmic Game Theory
- Natural Language Processing
- Robotics
- Trustworthy AI
Our Research Projects
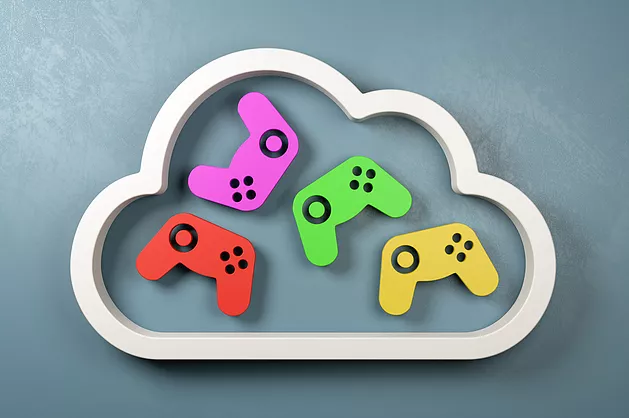
Leveraging large language models (LLM) to enhance research competency, academic motivation, and alleviate academic stress among undergraduate nursing students: A novel approach to research education
Funding Organisation: Ministry of Education Singapore Funding Scheme: Tertiary Research Fund (TRF) Grant Award Role: Co-Investigator Project Title: Leveraging large language models (LLM) to enhance research competency, academic motivation, and alleviate academic stress among undergraduate nursing students: A novel approach to research education Duration: April 2025 – April 2028
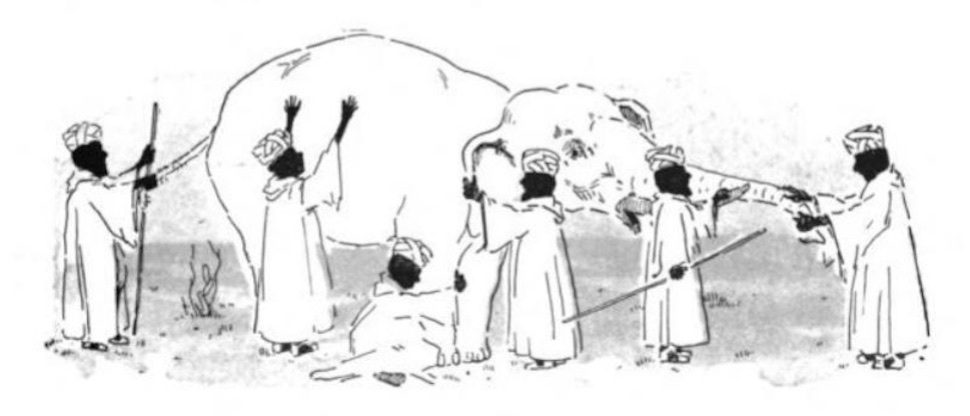
Learning to reason and plan with visual and linguistic inputs
Different subfields of AI, e.g. vision, language, reasoning, learning has been studied separately in depth. However, like the blind men and the elephant, can we truly understand the subject that way? We seek to exploit recent progress, particularly in deep learning, to effectively combine these methods within a single learning architecture. Image credit: Golden Treasury Readers: Primer.
- Computer Vision, Decision Making & Planning, Machine Learning, Natural Language Processing
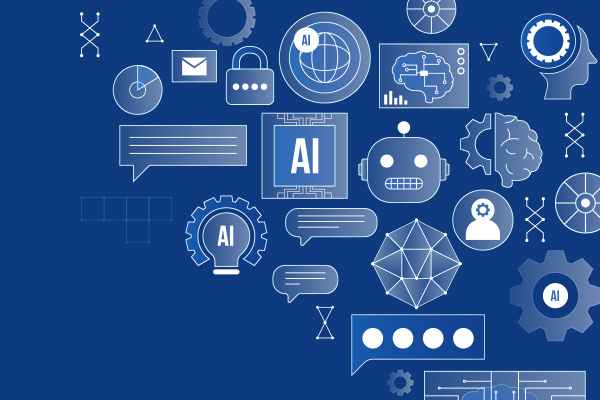
Learning to Decompose for Reasoning and Planning
This research aims to enhance Large Language Models (LLMs) by addressing challenges in reasoning and planning. The project hypothesises that breaking complex problems into smaller, solvable components can improve performance. It proposes developing self-supervised learning methods to enable reliable decomposition of open-world problems, reducing reliance on supervised data.
- Natural Language Processing
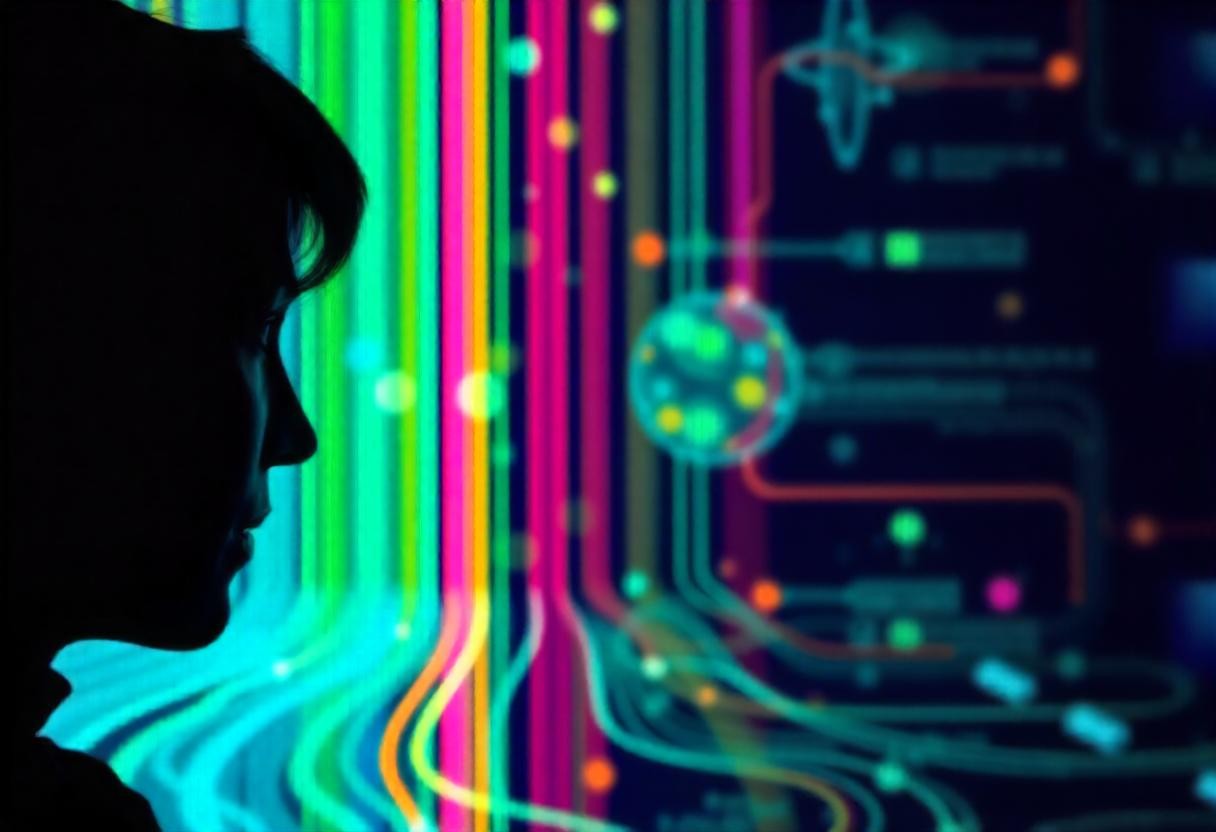
Enhancing Reliability and Relational Understanding of Visual Language Models
This research aims to address the limitations of Visual Language Models (VLMs), including hallucinations and poor relational understanding. The project proposes benchmarks to evaluate and understand these issues, and develops improved decoding approaches to detect and prevent hallucinations during text generation, enhancing VLM reliability and accuracy.
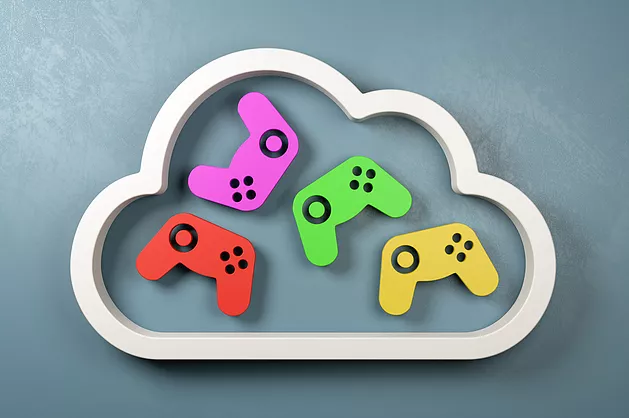
Development of a Swarm of Autonomous AI Agents for Educational and Career Advisory Roles
This project targets the development of a swarm of autonomous AI agents, with a focus on leveraging Large Language Models (LLMs) for specific roles in the education and career advisory domains. The project involves designing and integrating three specialised AI agents: an AI Teacher, a Time Scheduler/Manager, and a Career Coach, each underpinned by advanced LLM technology.
- Multi-Agent Systems & Algorithmic Game Theory
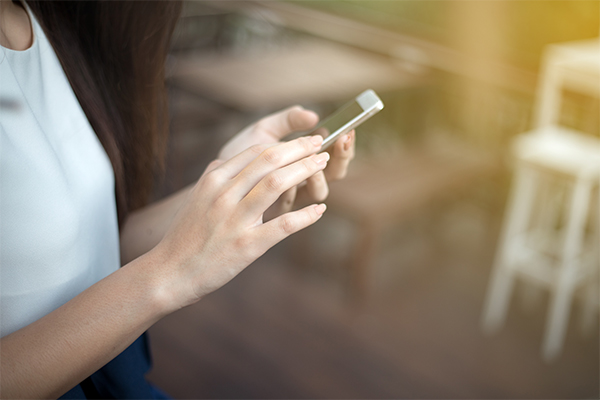
Implementation of Empowering Diabetic Patients
Enhancing diabetes care through EMPOWER app upgrades, integrating wearables, expanding food database, AI health coaching, and continuous glucose monitoring for select participants.
- Decision Making & Planning
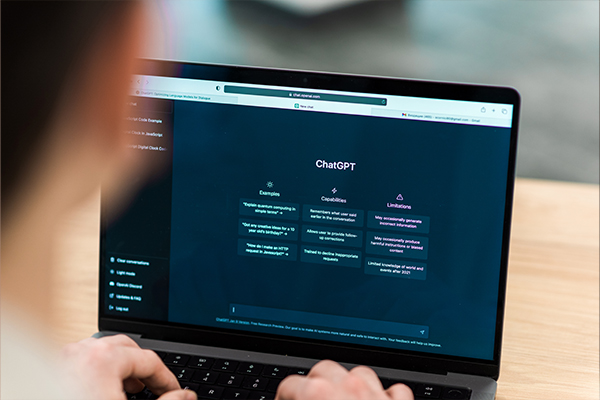
AI Over-reliance in Education
The project investigates whether ChatGPT fosters student over-reliance, potentially impacting learning outcomes. Its goal is to comprehend AI's influence and propose effective integration strategies in education.
- Trustworthy AI
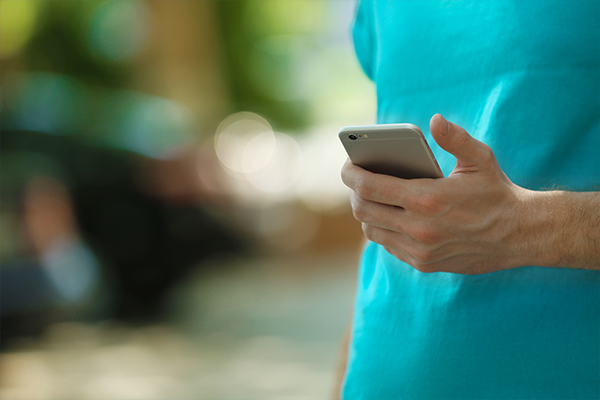
EMPOWERing Patients with Type 2 Diabetes Mellitus (T2DM) in Primary Care through App-based Motivational Interviewing
Enhancing diabetes management involves a mobile app-based approach. By combining motivational interviewing (MI) and AI-powered nudges, we empower behavior change and shared decision-making in patients with type 2 diabetes mellitus (T2DM).
- Decision Making & Planning
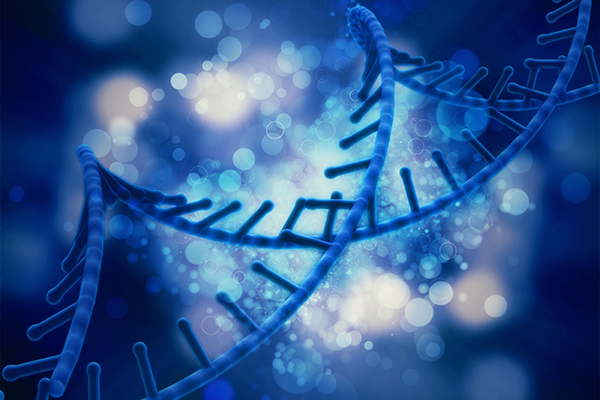
Deep Learning-Based Pipeline for High-Accuracy RNA Structure Prediction
Predicting RNA structures is crucial for drug discovery, but current methods lack accuracy. This project uses AI trained on vast RNA data to create precise 3D models from RNA sequences. This could significantly improve drug development and impact human health.
- Machine Learning
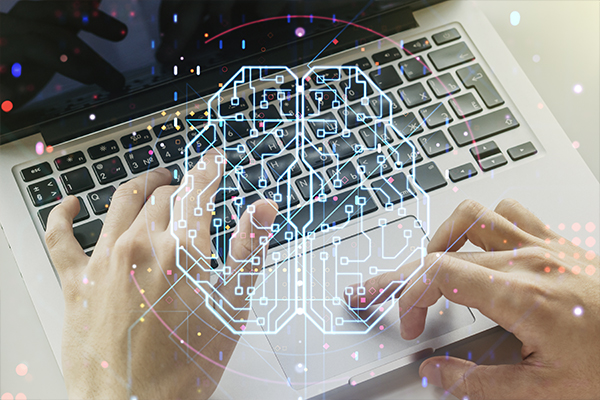
Analytical Framework to Quantify Information Leakage and Memorization in Machine Learning
Machine learning models can "memorize" specific data points from their training data, impacting their predictions and potentially leaking sensitive information. This project aims to understand how this memorization affects models and develop methods to mitigate it.
- Machine Learning
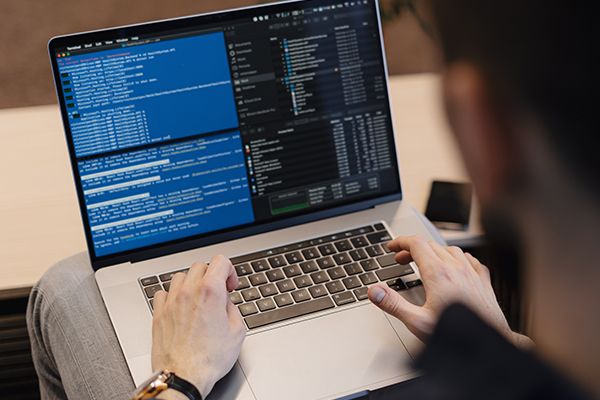
A Hybrid Approach to Automatic Programming
The project introduces an innovative approach that combines traditional program analysis, neural machine translation, and human guidance to enhance accuracy and generalization in automated programming tasks, thereby making coding accessible to non-experts.
- Machine Learning
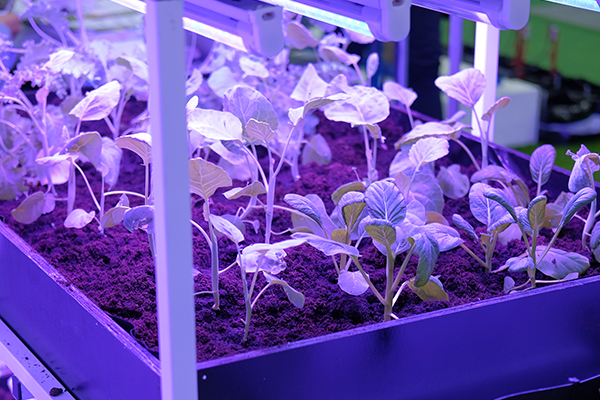
Scalable AI Phenome Platform Towards Fast-Forward Plant Breeding
The Low Lab aims to create a high-throughput screening platform for 100 leafy vegetable/plant lines. They’ll design black-box optimizers with Bayesian models to identify stress-resilient lines with fast growth. The challenge lies in complex correlations between stress resilience, growth, and environmental conditions.
- Decision Making & Planning, Machine Learning, Multi-Agent Systems & Algorithmic Game Theory, Robotics
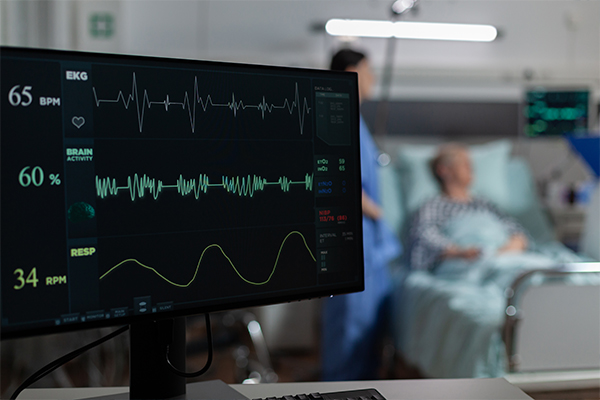
Explainable Risk Models for Heterogeneous Patient Populations in Critical Care
This project aims to improve the accuracy and explainability of clinical risk prediction (e.g., of unforeseen adverse events) in critical care by explicitly modeling underlying heterogeneous subpopulations and through the use of auxiliary knowledge.
- Decision Making & Planning
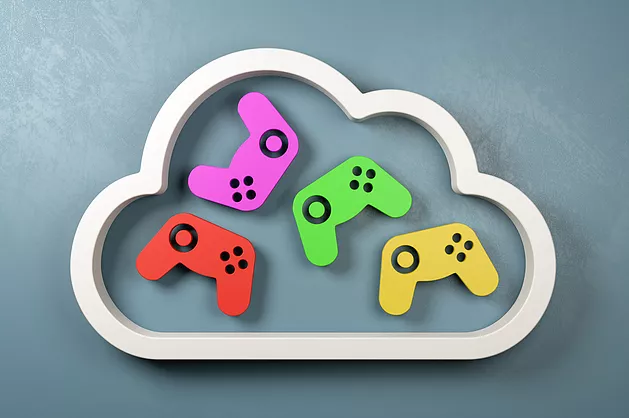
Safety and Reliability in Black-Box Optimization
This project seeks to enhance safety, reliability, and robustness in black-box optimization, exploring new function structures and addressing limitations. This includes extending decision-making frameworks to grey-box settings and multi-agent learning, utilizing a methodology blending theoretical analyses and algorithm development.
- Machine Learning
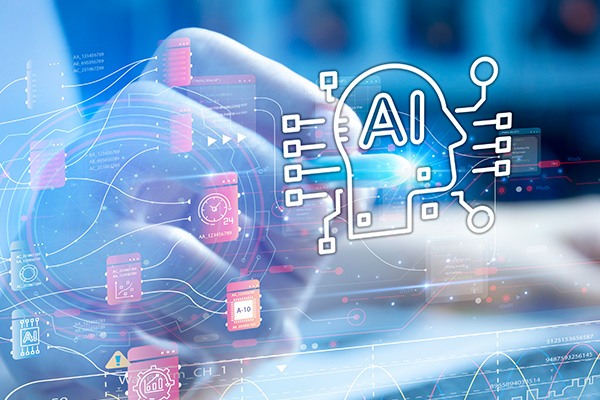
"Small Data" AI with Synthesis and Augmentation
This project focuses on overcoming small data sets in AI through the generation of synthesized training samples, effectively achieving knowledge-based synthesis and high-dimensional data efficiency, especially with images and videos.
- Knowledge Representation & Reasoning, Learning Theory, Machine Learning
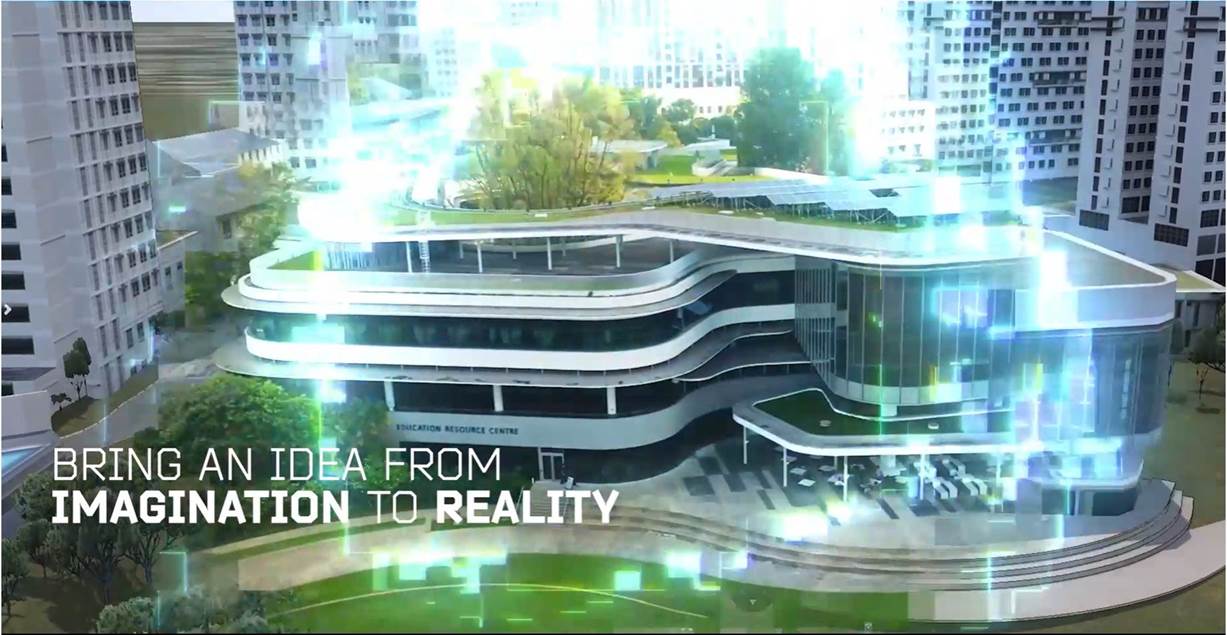
NUS Digital Twin for Research and Services
HUANG Zhiyong, HE Bingsheng, Anthony TUNG
This project aims to create a virtual twin of the NUS campus integrating the built and natural environment with static and dynamic data for modelling, visualization, simulation, analysis and AI. By creating a high-fidelity model, it harmonizes diverse data sources, optimizing performance for applications including smart transport, utility planning, climate studies and sustainable campus design.
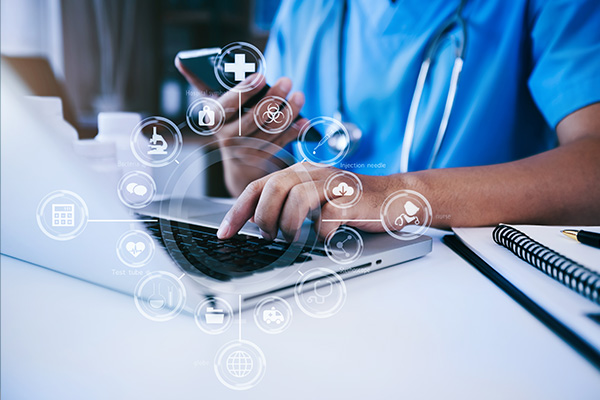
Designing and Evaluating Healthcare QA Assistants: A Knowledge Based Approach
Healthcare question answer (QA) assistants address user health queries, tackling challenges like misinformation and information overload. This project explores integrating language models (LMs) and knowledge graphs (KG) to enhance the design and evaluation of healthcare QA systems.
- Machine Learning, Natural Language Processing
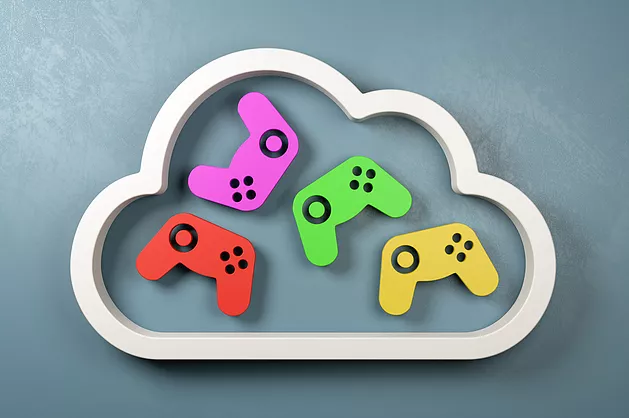
The “Other Me”: Human-Centered AI Assistance In Situ
We propose an integrative program of fundamental research towards a vision in which every human will have an AI assistant for daily life and work. Our overall aim is to build conceptual understanding of human-AI collaboration, to develop representations, models, and algorithms for situated assistance, and to integrate them in an experimental device platform for evaluation.
- Machine Learning, Natural Language Processing
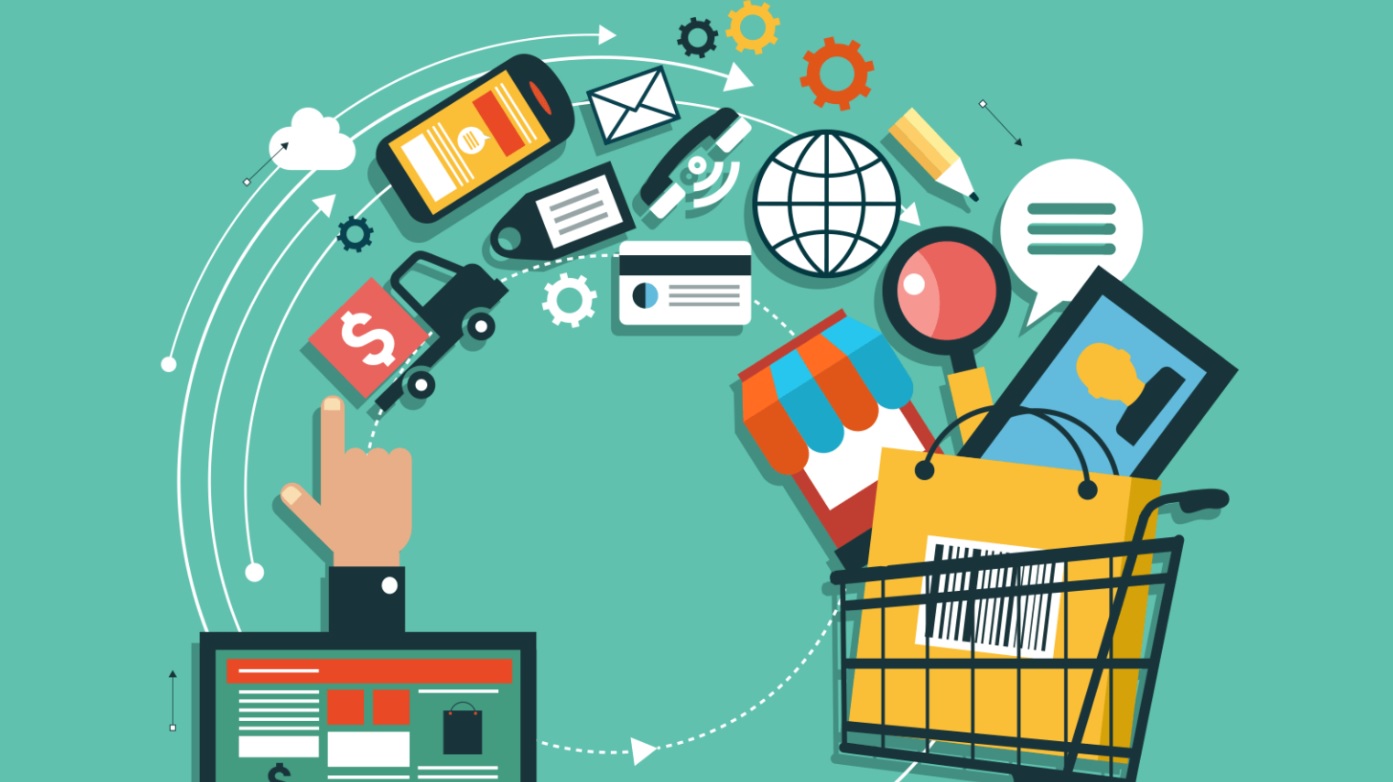
Economics of AI Pricing on E-Commerce Platforms
The use of artificial intelligence (AI) pricing agents has become prevalent, with many digital platforms including e-commerce platforms (ECPs), introducing AI pricing agents to provide sellers with AI-generated price recommendations. This project aims to apply relevant theoretical perspectives to examine factors that influence AI recommendation adoption, and the impact of such adoption on ECPs.
- Decision Making & Planning
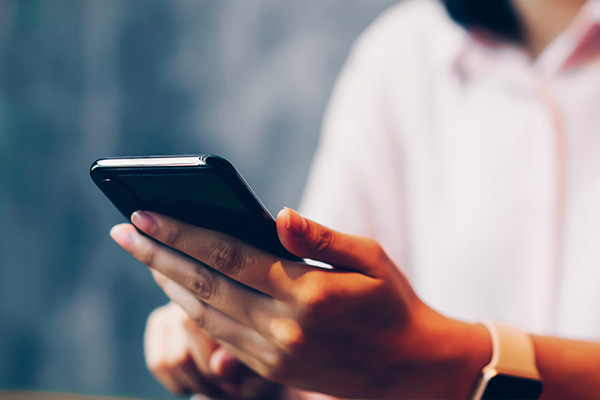
Designing New App Features: Imitate, Innovate or Follow the Crowd
Traditionally, product development ideas came from firms to the users, but now, user reviews on online platforms offer valuable insights. This research helps developers know when to take user suggestions into account and when to trust their instincts for creating better apps, especially when it comes to incorporating innovative and imitative features.
- Natural Language Processing
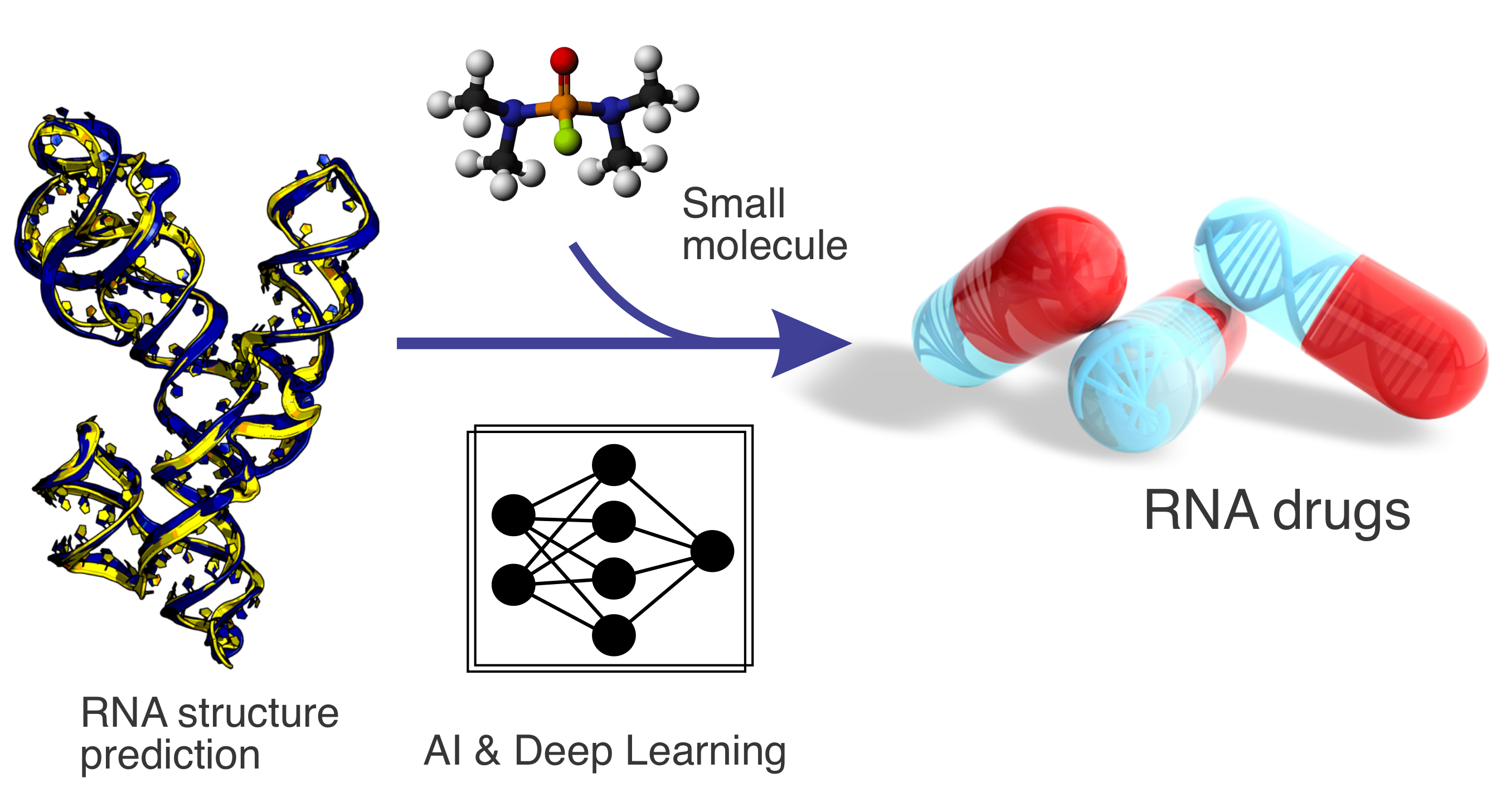
RNA structure prediction and small-molecule drug design
Non-coding RNAs, single-chain nucleotide biomolecules, play crucial roles as regulators of diverse biological processes. Our development of advanced AI and deep-learning algorithms, for precise RNA structure prediction and small molecule interactions, promises over a 10-fold expansion in drug design possibilities, surpassing traditional protein-targeting drug discovery approaches.
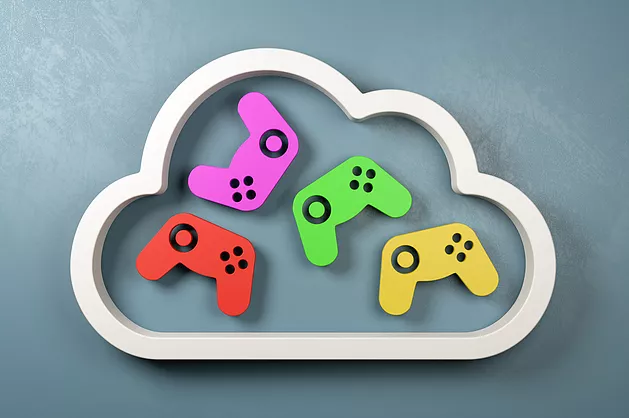
Multimodal AI for IoT
This project is partially funded by Microsoft's Accelerate Foundation Models Research Program.
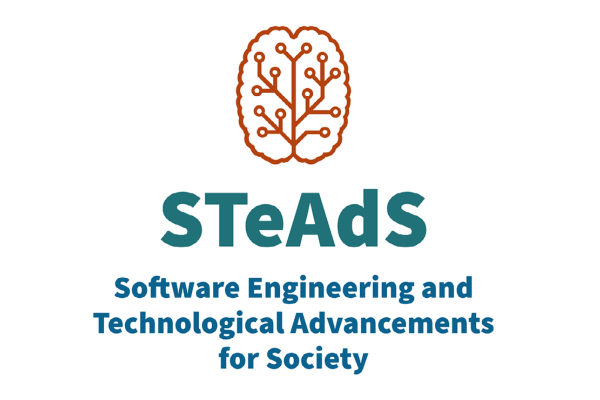
Using Machine Learning to reconstruct historical objects in culturally-situated ways
The aim is to use ML to explore how historical artefacts might have been used in their original cultural contexts. Our goal is to generate movable 3D objects from static 2D images. These can be used in many digital environments such as videos and AR/VR settings to enable scholars/general public to explore the usage of such objects and deepen their understanding of their context and function.
- Computer Vision, Machine Learning
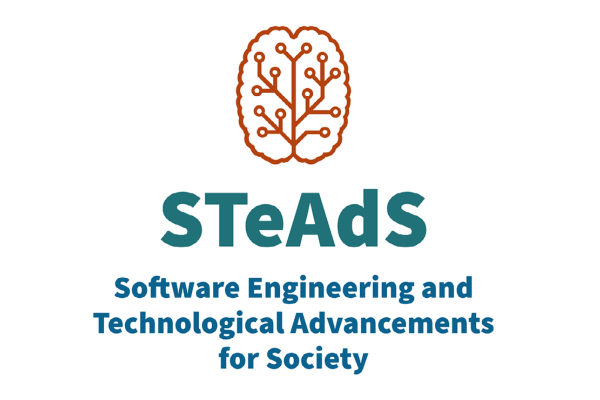
Using Machine Learning to reconstruct historical objects in culturally-situated ways
The aim is to use ML to explore how historical artefacts might have been used in their original cultural contexts. Our goal is to generate movable 3D objects from static 2D images. These can be used in many digital environments such as videos and AR/VR settings to enable scholars/general public to explore the usage of such objects and deepen their understanding of their context and function.
- Computer Vision, Machine Learning
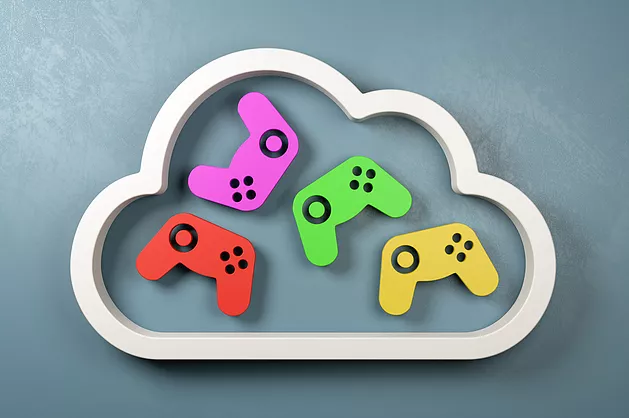
DesCartes WP4: Human-AI Collaboration
OOI Wei Tsang, Brian LIM, ZHAO Shengdong
WP4 focuses on how humans can interact with AI to (i) bring humanity aspects that cannot be computationally modeled into AI systems and algorithms, forming a hybrid AI with human interaction at its core, and (ii) allow hybrid AI to augment human perception and cognition (especially assisting humans in decision-making). Within this WP, we propose to develop interaction and visualization techniques

Recommendation Systems
Recommendations Systems curate our news feeds, and show products for us to buy, shows to watch and music to listen to. Our work examines the use of temporal and prerequisite constraints in improving recommendation systems quality in sparse data application areas, such as module and course recommendation.

Task Oriented Dialogue Systems
We now use voice- and text-enabled chatbots and dialogue systems often to accomplish tasks. We examine ways to improve such systems by incorporating everyday knowledge in the form of knowledge graphs and incorporating means to adapt trained systems to new domain application areas.
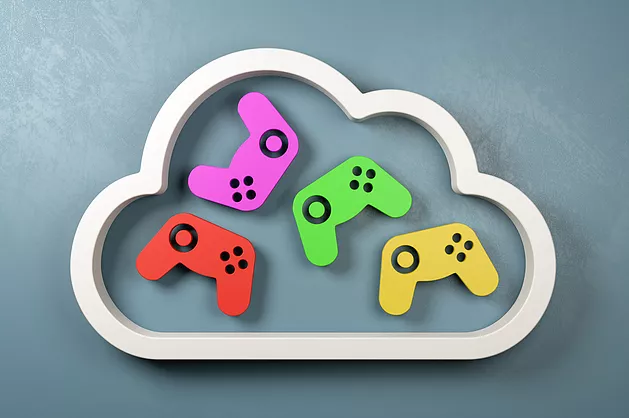
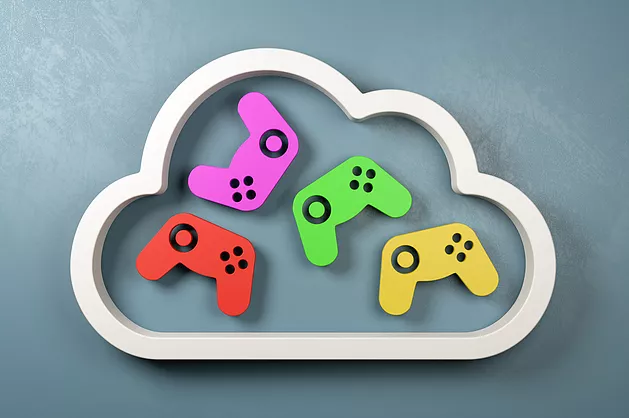
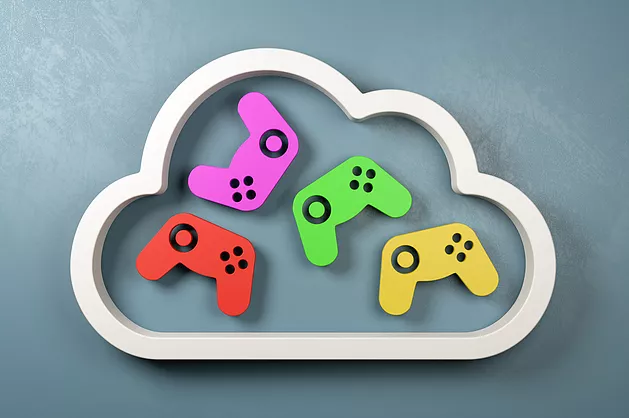
Our Research Groups
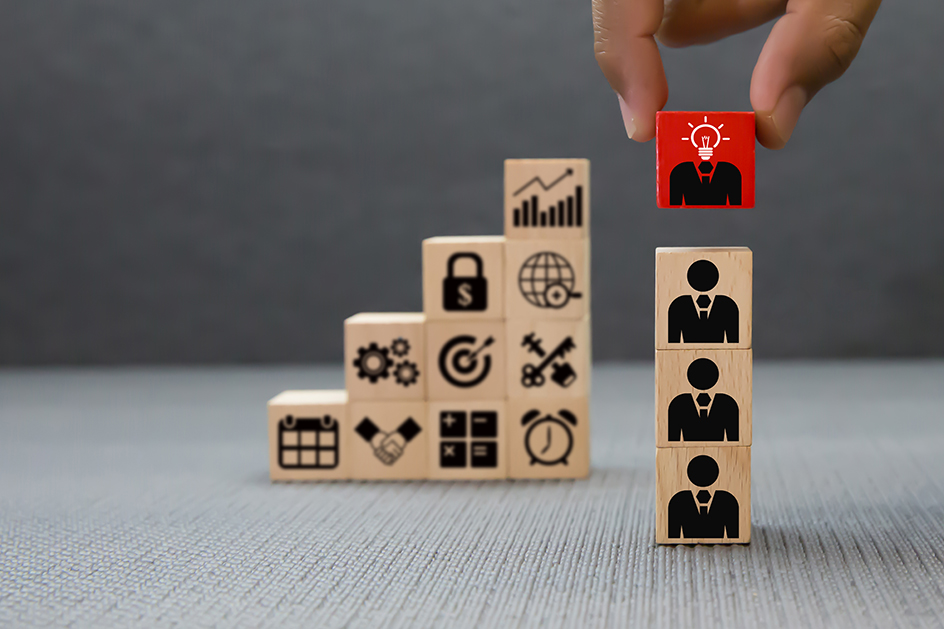
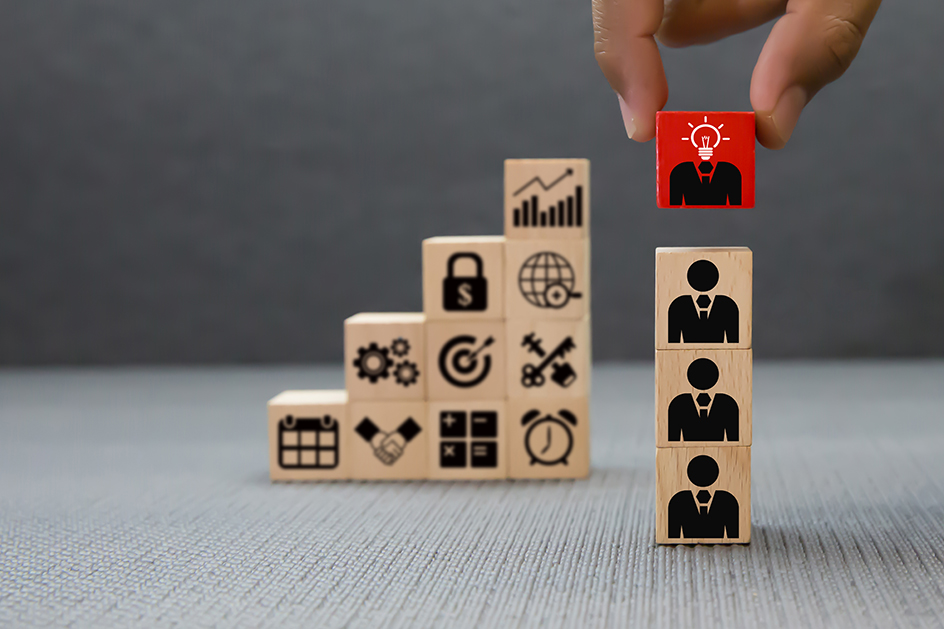
Deep Learning Lab
Our lab aims to contribute to the development of deep learning methods and the understanding of intelligence.
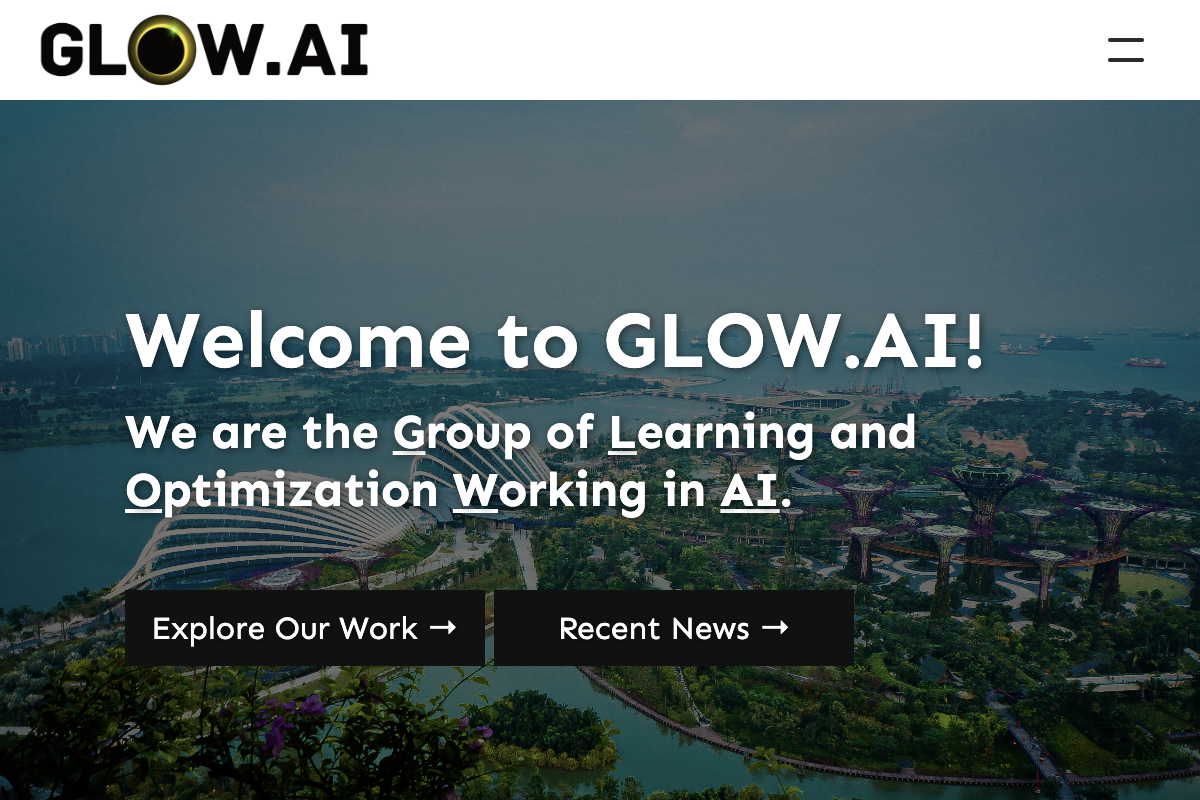
Group of Learning and Optimization Working in AI (GLOW.AI)
At GLOW.AI, we are interested in learning & optimization on data-centric AI, collaborative AI, automated AI, and AI for science problems, their applications to LLMs & MLLMs, among others. Our group is multi-disciplinary: CS, math, stats, physics, engineering, data science. We believe in theory & practice.
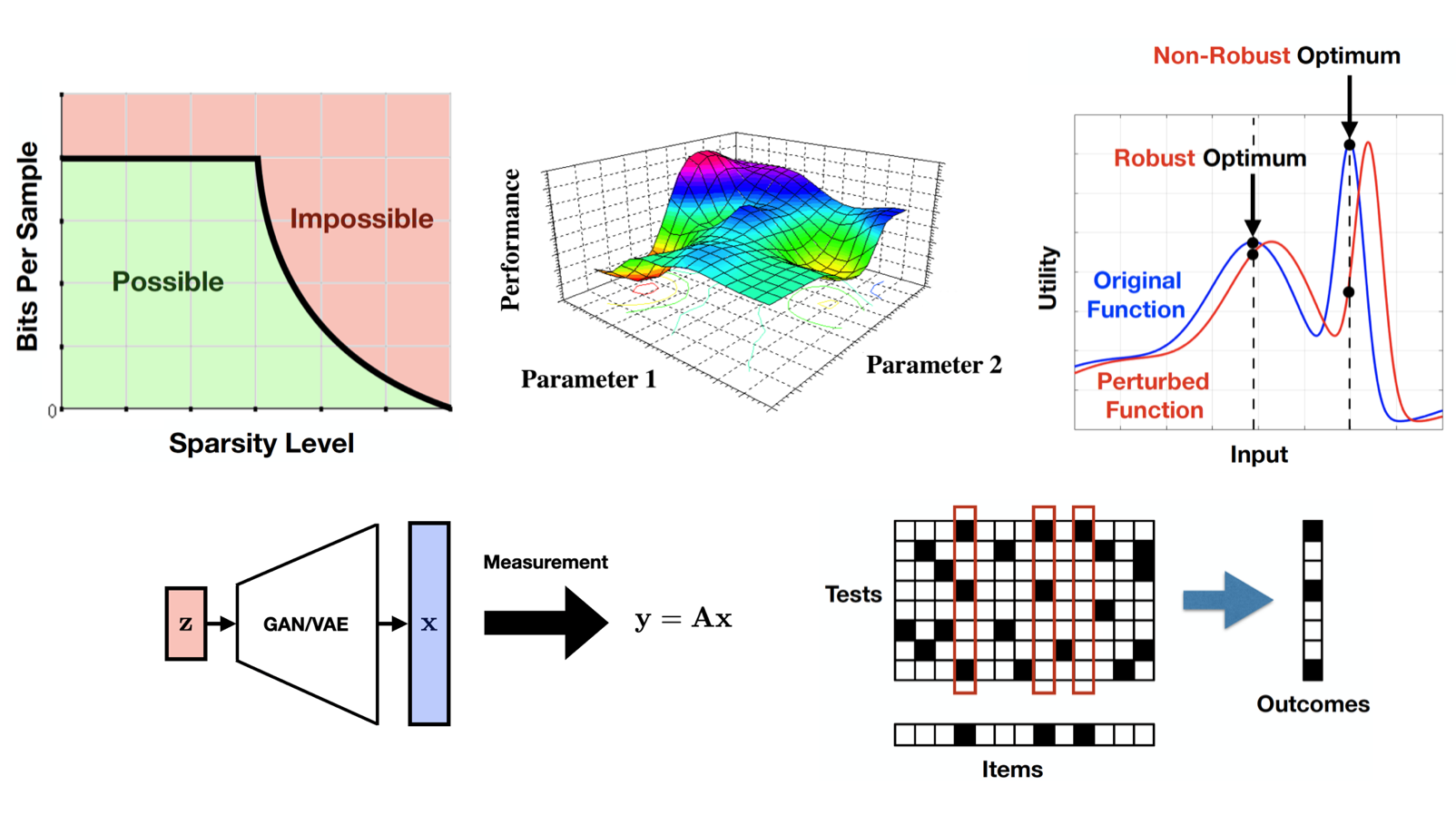
Information Theory and Statistical Learning Group
Our group performs research at the intersection of information theory, machine learning, and high-dimensional statistics, with ongoing areas of interest including information-theoretic limits of learning, adaptive decision-making under uncertainty, scalable algorithms for large-scale inference and learning, and robustness considerations in machine learning.
- Learning Theory, Machine Learning
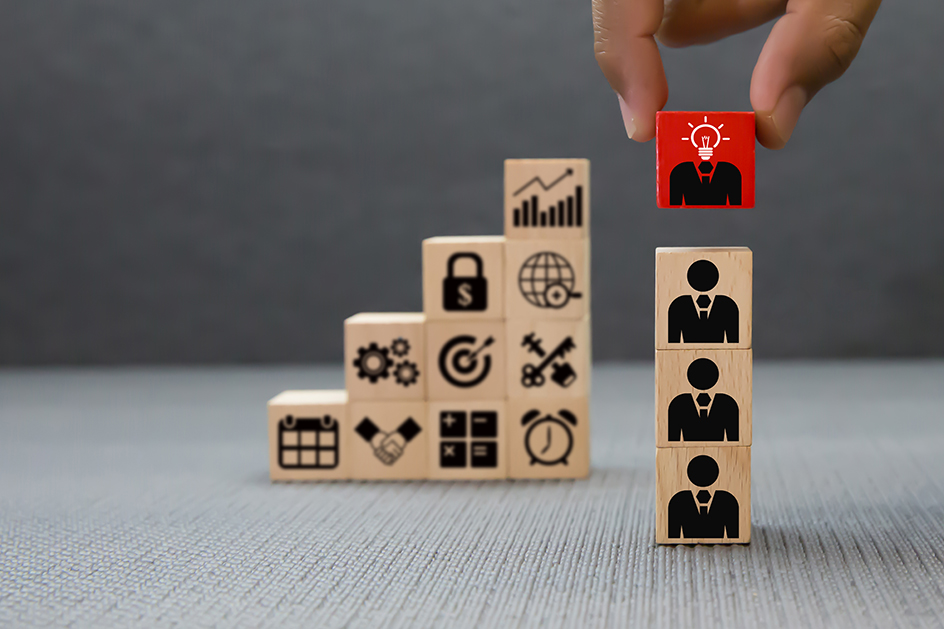
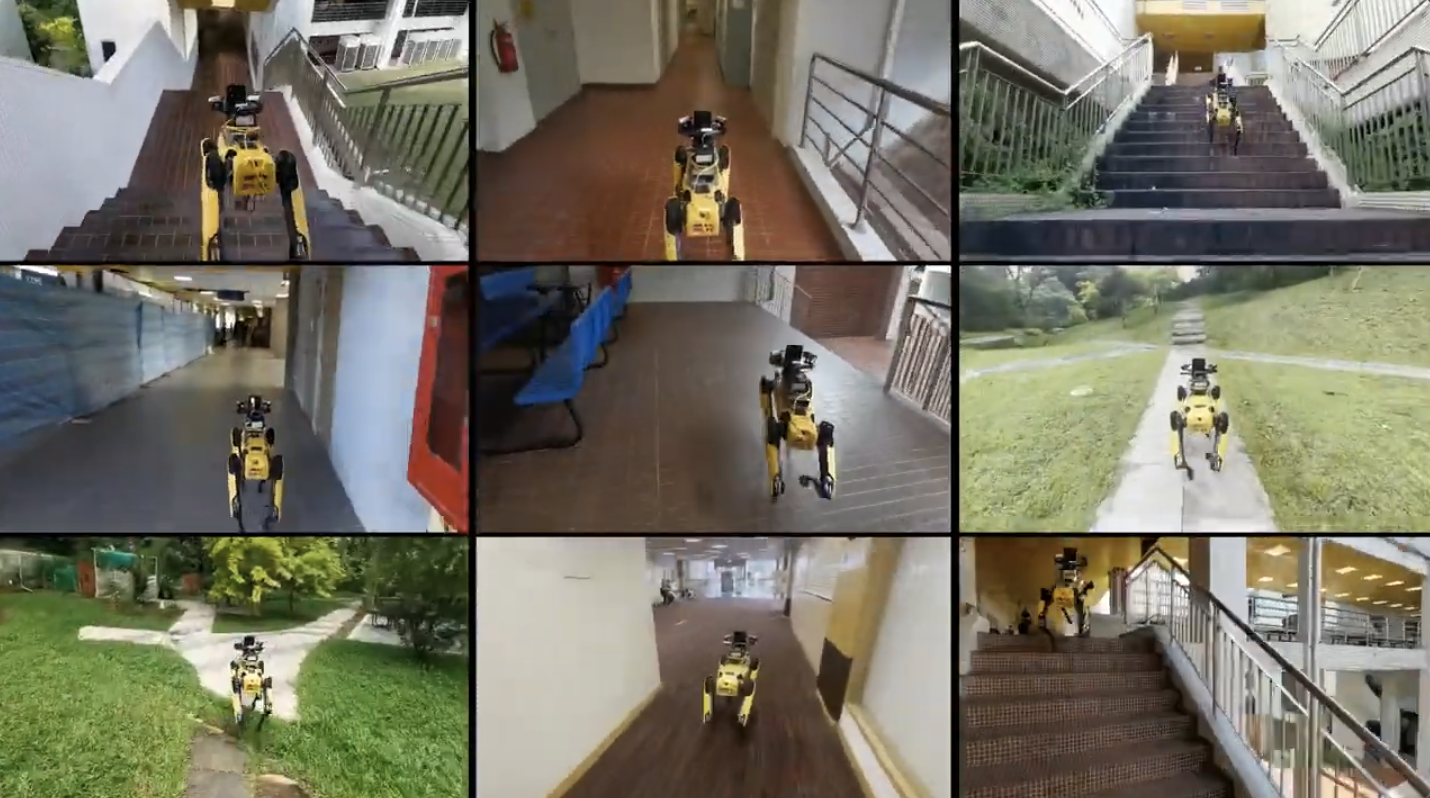
Adaptive Computing Laboratory
Our long-term goal is to understand the fundamental computational questions that enable fluid human-robot interaction, collaboration, and ultimately co-existence. Our current research focuses on robust robot decision-making under uncertainty by integrating planning and machine learning.
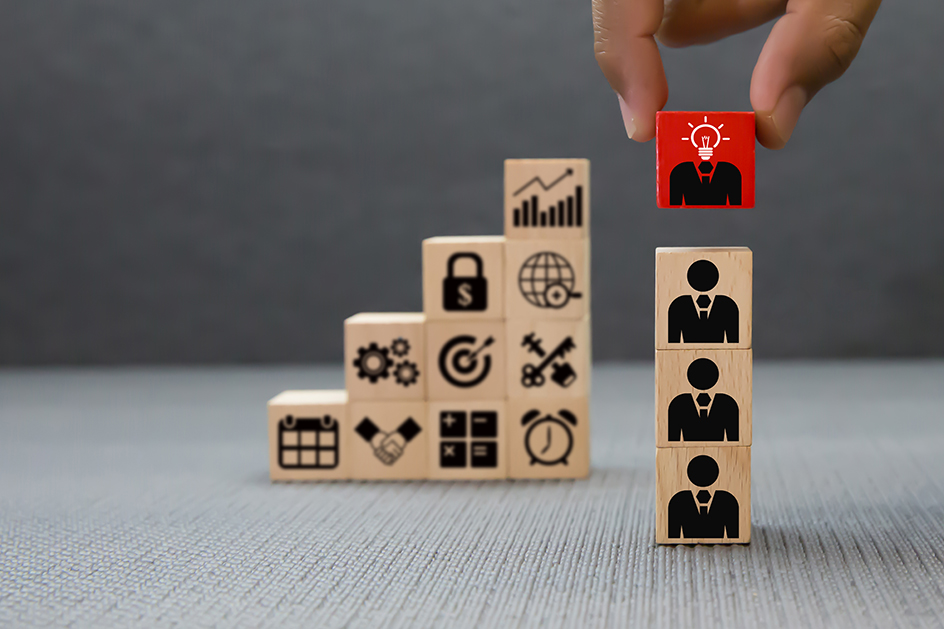
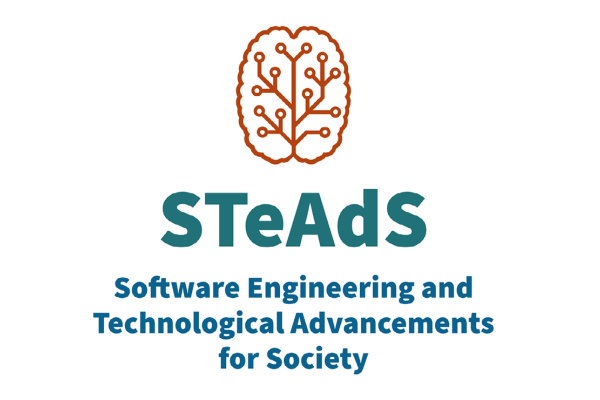
STeAdS Virtual Group
Software Engineering and Technological Advancements for Society. A virtual group that uses Software engineering practices and Technological advancements (Cloud computing, Artificial Intelligence (EdgeAI, ML)) for the benefit of various aspects of society (healthcare, education, art & culture). Looking for students to collaborate on different projects. Look at ganeshniyer.github.io for details.
- Decision Making & Planning, Machine Learning, Multi-Agent Systems & Algorithmic Game Theory