When a natural disaster, terrorist attack, or any other crisis strikes, the best time to act isn’t just as it occurs, but rather in the months, even years, before it happens.
Preparation is key, say experts. “The more we prepare beforehand, the better our response will be,” writes the United Nations Office for the Coordination of Humanitarian Affairs (OCHA), the group responsible for organising relief efforts across the globe, on their website.
It is a statement that Gary Tan agrees with wholeheartedly. The associate professor at NUS Computing, who has spent the last decade studying crisis management, says: “Every second counts in a crisis. Delays can lead to higher fatalities, which is why it’s critical to have effective strategies for evacuation and rescue.”
Tan’s work focuses on simulating what happens when disaster strikes. In particular, he is interested in modelling how humans run or flee in such scenarios. “People behave very differently when they panic,” he says. “What we’re trying to do is predict what happens when, say, we have to evacuate an MRT station because of a bomb threat or a fire.”
Tracking movement
To achieve this aim, Tan and his students — PhD candidates Wang Chengxin and Muhammad Shalihin bin Othman — devised a special framework, which they describe in this paper. The framework analyses real-life video feeds, tracks how pedestrians in them move about, and translates these into something that can be used in a virtual simulator. The technique makes use of deep learning methods to detect objects in individual video frames, correctly tracking them throughout the video feed.
“With that, we are able to reconstruct scenes and simulate events that may be too costly or dangerous to carry out in real life,” says Tan. “This allows us to simulate different evacuation and rescue plans to derive ideal strategies to employ in a crisis.”
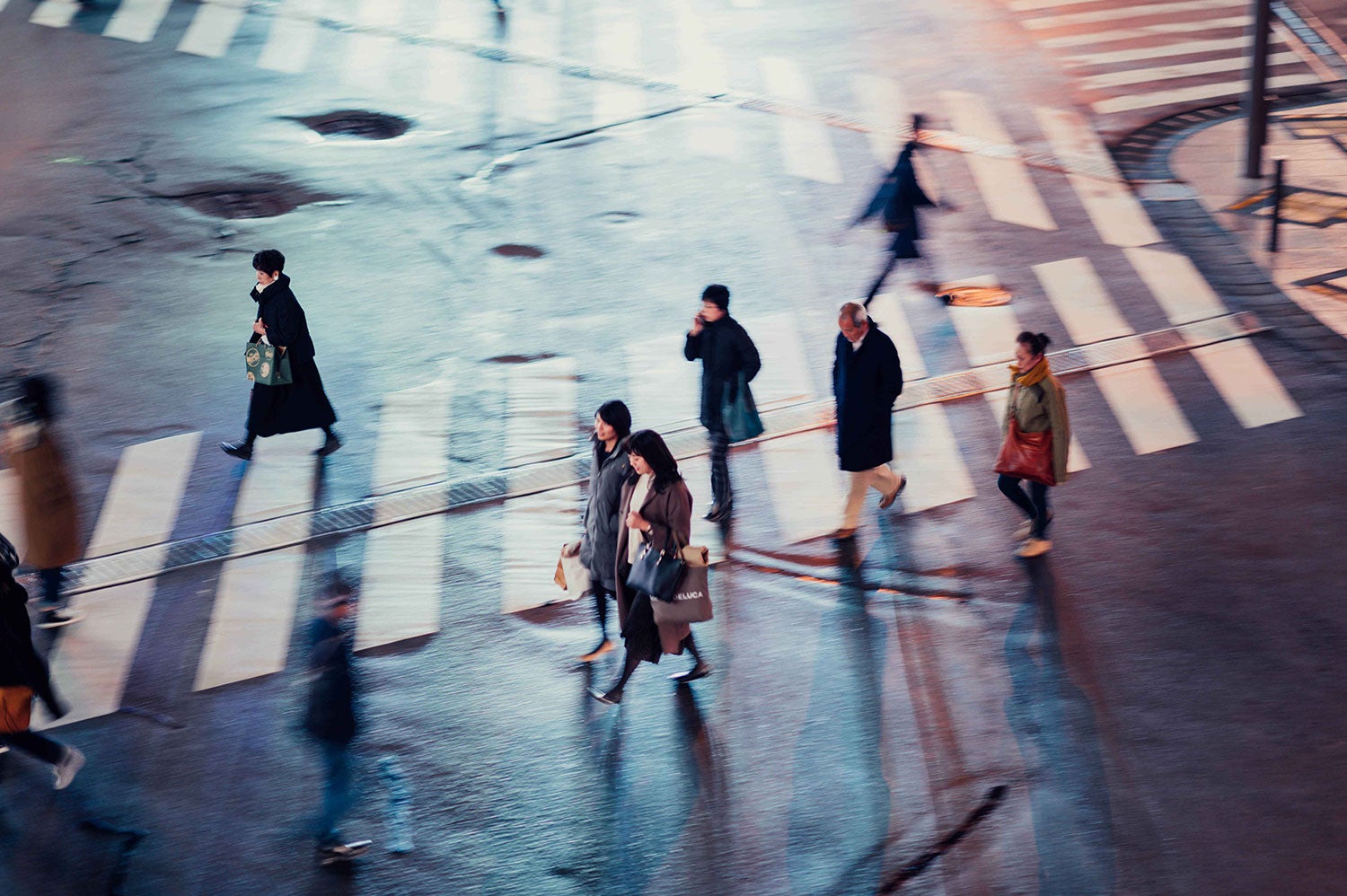
Tan’s framework is unique because it is a data-driven approach that seeks to study human behaviour directly from real-life videos, unlike previous pedestrian simulation methods. “This helps improve the level of realism since they are adapted from actual footage,” he says.
Tan and his team worked especially hard to refine their tracking algorithm that analyses how people in the videos move. “A good tracking algorithm is essential in extracting realistic trajectories from real-life videos,” he explains. “With highly accurate trajectory data, we can mimic realistic human movements in a simulation that allows us to have more effective predictions.”
Testing, he adds, revealed that the number of trajectories successfully extracted from the videos into the simulator was “more than expected”.
From prediction to prescription
Since publishing their findings, the team have turned their attention to creating other pedestrian tracking algorithms. One, called Graph-based Temporal Convolutional Network (GraphTCN), makes use of artificial intelligence to track not only individuals, but also how they interact with their fellow pedestrians temporally and spatially. The result, which they describe in this 2020 paper, is a behavioural model that can mimic human movement in a simulation more accurately, says Tan.
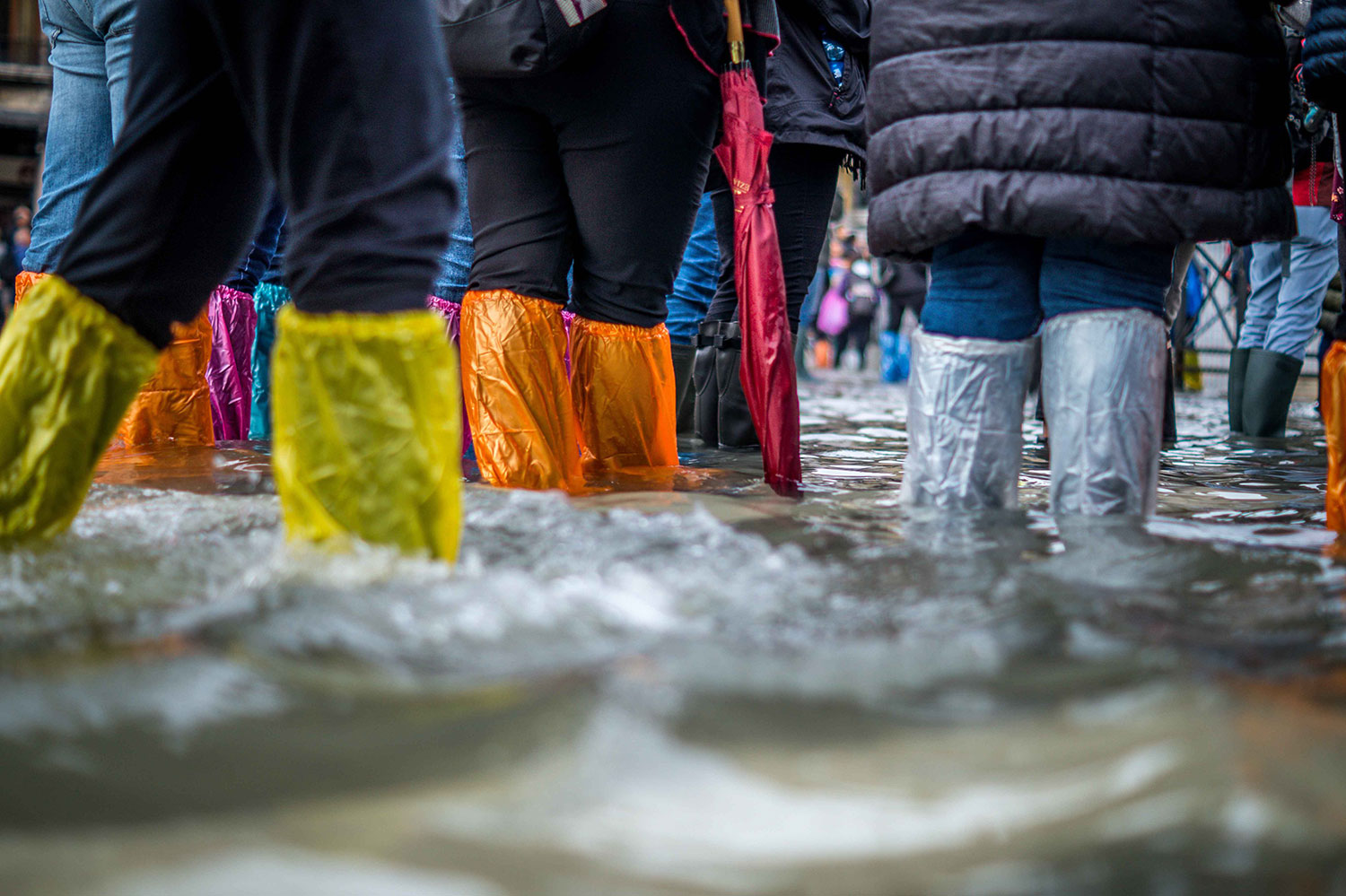
His team is now working on a new model that thinks one step further. The Conscious Movement Model, or CMM, extracts human behavioural patterns from CCTV footage and other real-life videos. It uses these patterns to train a deep learning model that will later influence a pedestrian’s movements during the simulation.
“By incorporating realistic pedestrian movements, we can improve the accuracy of predictive simulations, allowing us to automatically run optimisation algorithms and prescribe the best strategies to adopt in different what-if scenarios,” explains Tan.
“By doing so, we take a step further after predictive simulation to generative prescriptive analytics that authorities can employ in times of crisis,” he adds.
Apart from disaster scenarios, Tan’s research can be applied to simulations involving traffic congestion and accidents, to model the movement of both pedestrians and cars.
Tan says: “If our system can save even one additional life when deployed, it will be worth the effort.”