One of the most famous folklore in marketing and data mining goes like this: many years ago, Walmart noticed that on Fridays, men would head to the store, pick up some diapers for their babies and grab a six-pack at the same time. To take advantage of this, Walmart placed the diaper and beer aisles adjacent to each other, resulting in skyrocketing sales for both items.
As with many myths, it’s debatable whether the incident actually happened. But the lesson prevails: stores can find patterns in the data they collect and apply that knowledge to increase product sales. The same remains true today, even if a big part of shopping has moved online and many store shelves have now become virtual.
To boost sales, e-commerce stores (Amazon, Asos, and Alibaba, just to name a few) make use of algorithm-based product recommendation systems. Such a system recognises that you’re buying a pair of sneakers, for example, and recommends a new workout top and jogging shorts to complete the look — similar to how a savvy salesperson might try to push more products on you in a store. However, what is different online is that many such recommendations in e-commerce stores are actually inter-linked with one another, thus forming a network of product recommendations.
“The idea is that e-commerce stores not only want you to buy things online, but also channel you to buy things through various inter-connected recommendations,” says Goh Khim Yong, Associate Professor of Information Systems and Analytics at the NUS School of Computing. “In business terms, it’s also to increase the share of wallet for each customer.”
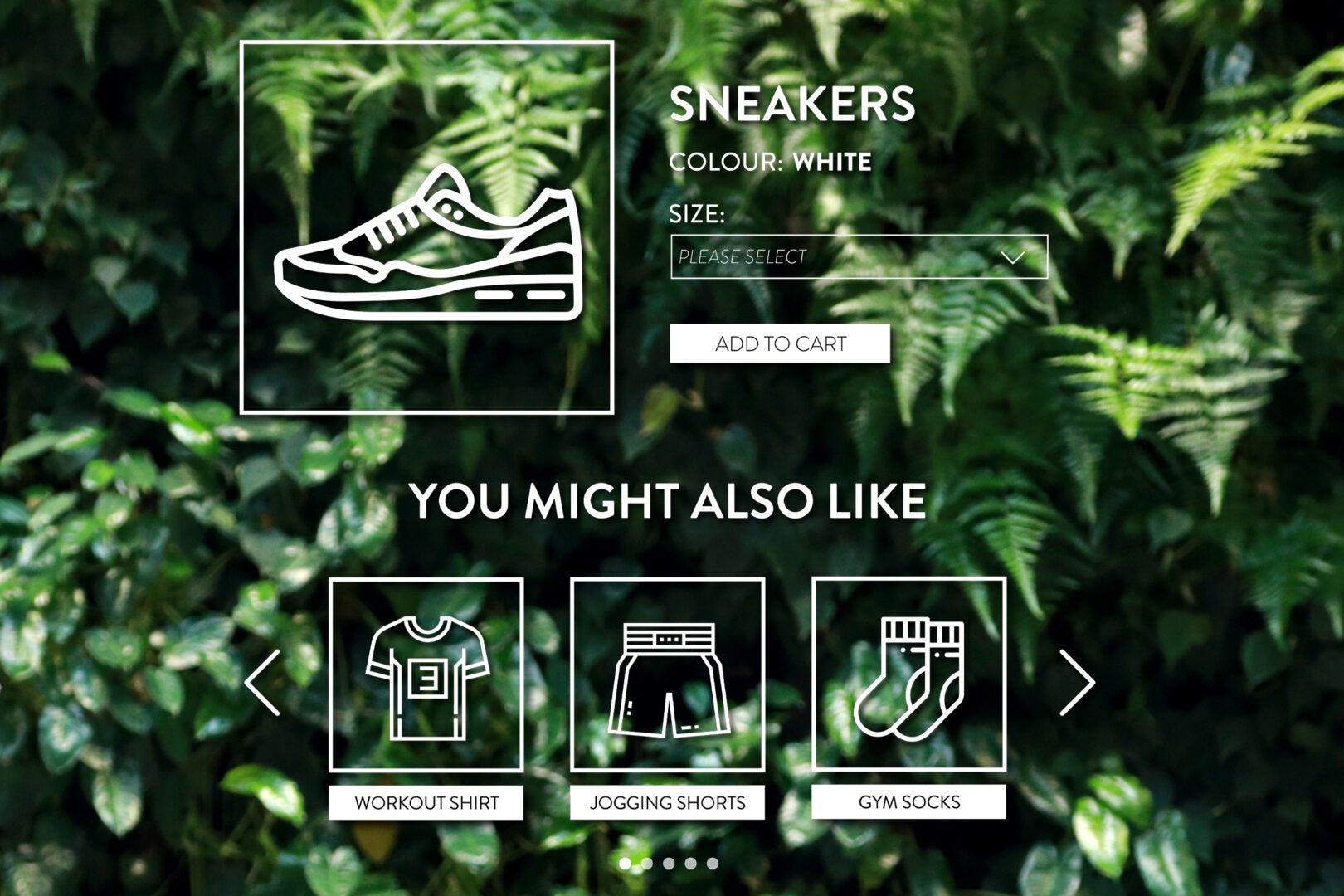
“In order to do that, you need to have recommendation agents,” says Goh. But how can online stores manipulate these recommendation networks in order to boost their sales?
Intrigued, Goh set out to investigate this question. Specifically, what he and his colleagues Heng Cheng Suang (Associate Professor, School of Computing) and Lin Zhijie (previously NUS School of Computing, now at Nanjing University Business School) wanted to explore was how the diversity and stability of recommendation networks impacted product demand. Diversity refers to the assortment of goods offered in the network, while stability is used to describe the frequency with which the goods are rotated.
The researchers were also keen to study how a person’s purchasing decisions could be influenced by what fellow consumers were browsing or buying on the e-platform, called “co-view” and “co-purchase” recommendations respectively. For example, an algorithm may recognise that someone is looking to buy a new smartphone and suggest certain popular models based on what other people — like-minded ones who are also looking to upgrade their phones — have viewed on the platform (co-view recommendations). The algorithm also looks at what fellow consumers purchased alongside their new smartphones, and makes suggestions for smartphone cases, power banks, Bluetooth headsets, among other things (co-purchase recommendations). Either type of recommendation can possibly affect purchasing decisions.
A third aspect the researchers wanted to examine was how incoming and outgoing network links can influence demand. Incoming links refer to when other product pages on the e-platform link to the target product. For example, if the target product was a healthy recipe cookbook, a person looking at buying organic ingredients could be directed towards the book. Outgoing links on a target product’s page, on the other hand, direct the consumer towards other products. So the person looking at the healthy cookbook, for instance, may now receive recommendations to purchase bamboo cooking utensils, a BPA-free cutting board, or a slow juicer.
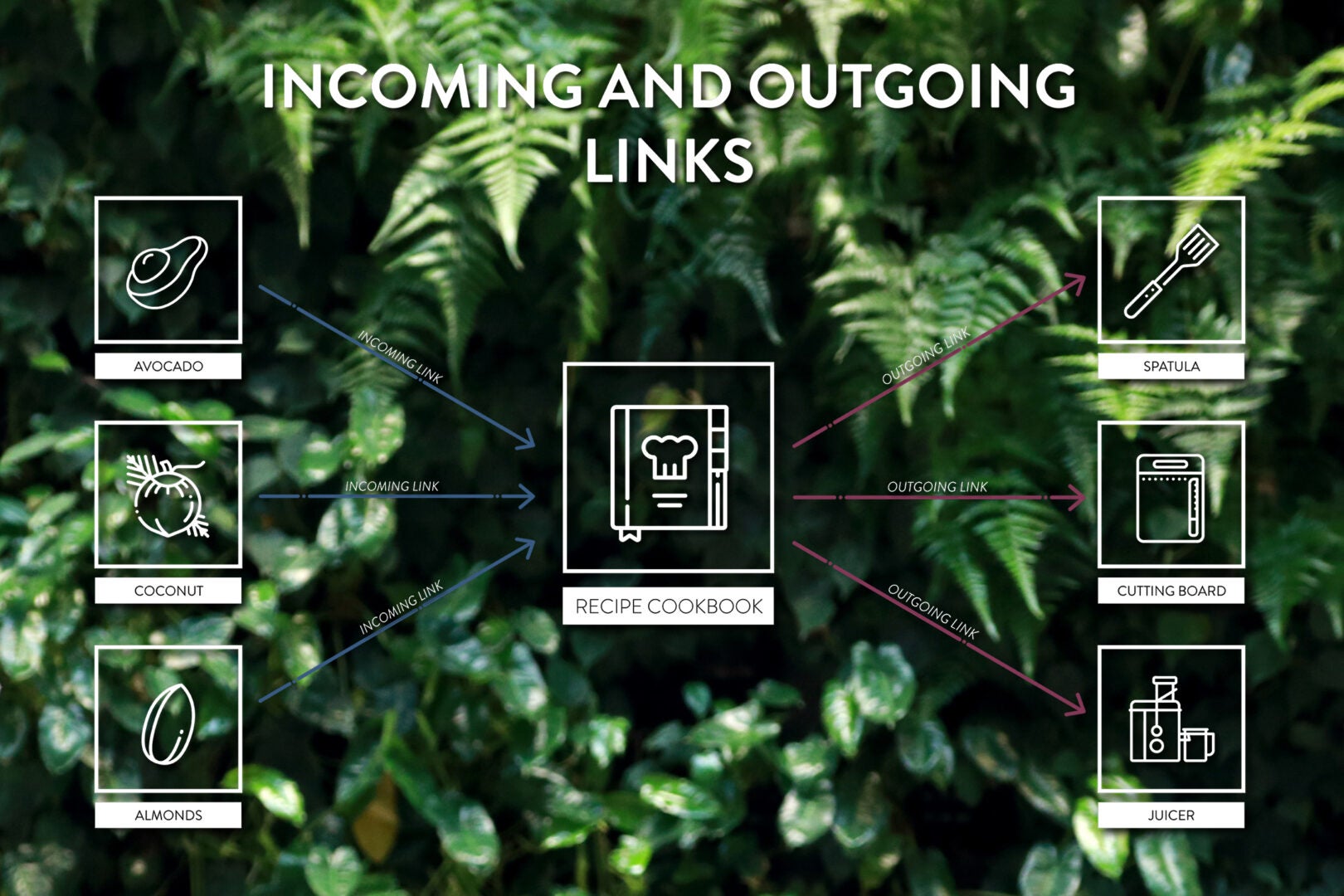
Craft platforms carefully
To carry out their research, the trio analyzed data from four different stores on Tmall.com, China’s largest business-to-consumer online retailer. They examined the sales of four products — digital cameras, personal computers, mobile phones and cosmetics — over the course of seven months, looking at how price, consumer reviews and product network structure translated into actual product sales.
Their findings were published last year in the journal MIS Quarterly, and is one of the few studies to use actual information from e-commerce retailers, rather than being purely theoretical in nature.
Based on their observations, the team noted that both network diversity and stability affected product demand. For every one percent increase in the diversity of incoming links, demand for the product in question rose by 0.011 percent. Conversely, a one percent increase in diversity and stability of outgoing links were both associated with a 0.012 percent drop in demand.
To understand this, think of selling AAA batteries, says Heng. Batteries have many incoming links because “more often than not, a consumer has to first purchase an electrical product, for example a camera, before purchasing a battery,” he explains. Because so many electrical products, apart from cameras, are battery-operated, the more you link those products to batteries on your e-platform network, the more batteries you’re likely to sell.
Conversely, when a consumer purchases a new camera, AAA batteries may not necessarily be the first thing he or she thinks about co-purchasing. Instead, a consumer may buy tripods, various lens or filters, and a protective camera bag. If you want to increase the sales of AAA batteries following a camera purchase, “you have to be mindful about this and carefully construct the outgoing network that follows from the sale of a product,” says Heng.
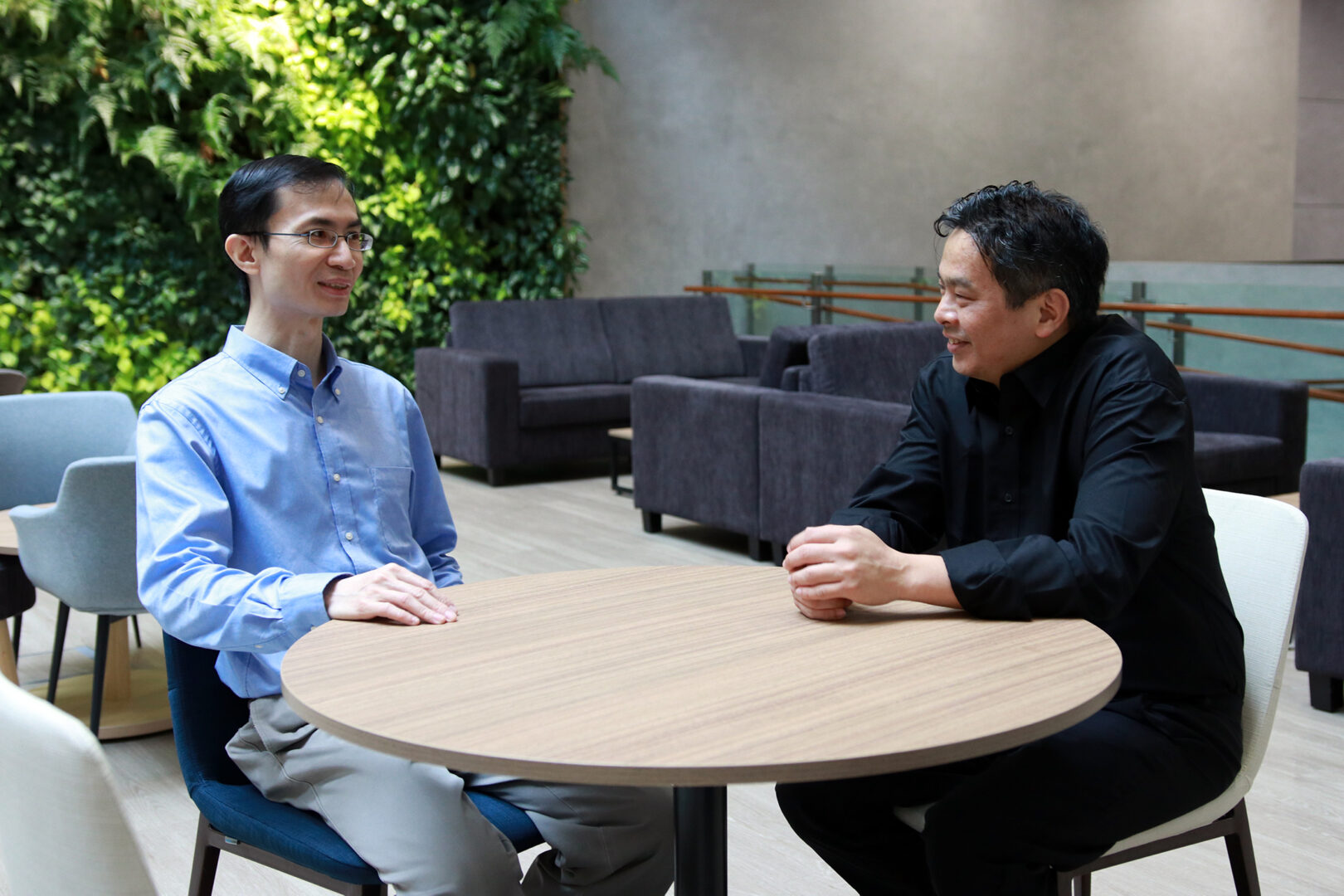
It’s also important for retailers to pay attention to how many products are recommended from the target product’s page itself, and periodically rotate the items recommended to boost sales of the target product. “We quantified that if the stability is too high, that tends to be related to a drop in demand,” says Goh. “If you keep recommending the same old products, the interest from your customers will decline.”
Another finding the researchers noted was how the demand effects were greater in the co-purchase network compared with the co-view network. In other words, consumers tended to pay more attention to recommendations of “consumers who bought this item also bought this”, as opposed to “consumers who viewed this item also viewed this.”
That’s probably because co-purchasing indicates what other consumers actually bought, rather than what they were merely interested in browsing through, says Goh. In addition, co-purchase recommendations are often complementary products (such as cameras and batteries or tripods), whereas co-view recommendations tend to be substitute products (such as cameras of varying brands). Thus to capitalise on this, the authors recommend that retailers use a larger proportion of each product page to display co-purchase recommendations in order to boost sales.
The team’s study is significant because it marks the first time anyone has examined the effects of network stability and diversity on product demand. It’s also the first study to quantify the economic impact of both incoming and outgoing product networks on sales (prior studies have mostly focused on the incoming product network).
At the end of the day, one thing all e-commerce companies are trying to do “in terms of tools is to stimulate demand using recommendation agents,” says Goh.
“These companies grapple with the dilemma of whether to display more complementary or substitute products, co-purchase and co-view products, as well as how often to inject change,” adds Heng. “What we’ve tried to do is to provide an answer to help them better captialise on the limited space they have on their platforms.”