In recent years, some companies, including Amazon, JP Morgan, and Unilever, began asking prospective employees to do a curious thing — to film themselves answering a fixed set of questions. The firms would then run the videos through an AI-powered software, scanning faces and eye movements for signs of empathy, dependability, and other ‘desirable’ personality traits.
Apart from screening job applicants, affectively-aware AI — artificial intelligence that can recognise emotional expressions — have been applied to a number of scenarios today. It’s been used to detect deception in courtroom videos, monitor workers’ emotional states and customers’ moods, and even track the attention levels of students.
While emotion recognition is still a nascent technology, the market — projected to be worth US$43.4 billion by 2027 — is a booming one. “The question is not if research can produce affectively-aware AI, but when it will,” says Desmond Ong, an assistant professor of information systems and analytics at NUS Computing.
As the technology begins to mature and proliferate, Ong says it is crucial to consider its wider implications. “What will it mean for society when machines, and the corporations and governments they serve, can ‘read’ people’s minds and emotions?” asks the computational cognitive psychologist.
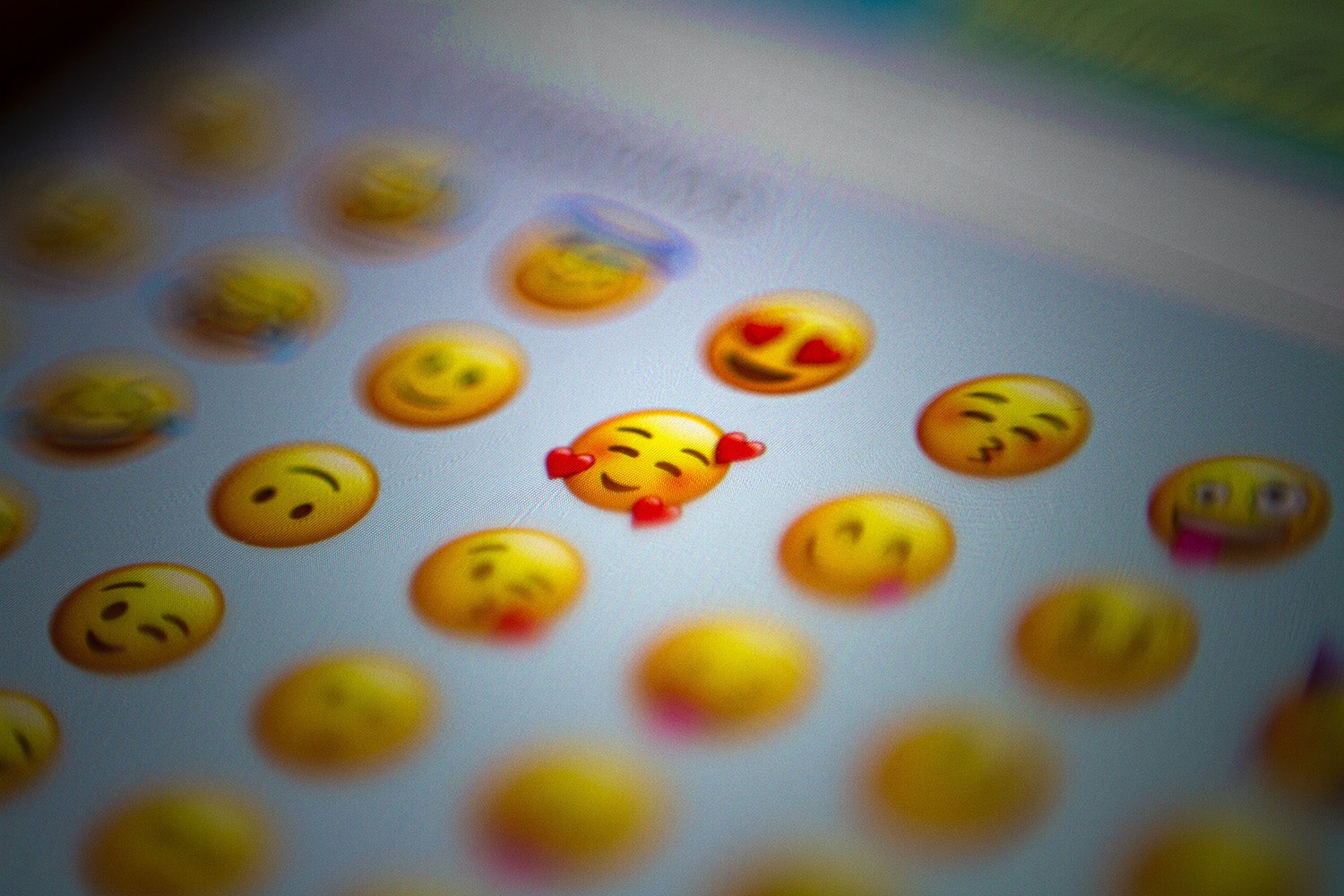
Already, there has been some backlash. HireVue, a leading provider of AI-based interview platforms, announced in 2020 that its algorithms would no longer analyse facial expressions. Laws governing the use of AI are also being increasingly strengthened — in April 2021, for instance, the European Commission unveiled a draft regulatory framework called the Artificial Intelligence Act, in which emotionally intelligent AI systems were specifically mentioned as a high-risk application.
“People are starting to realise that AI ethics are important,” says Ong. While certain professions, such as medicine and engineering, bind their members to professional codes of conduct, computer science “just doesn’t have that,” he says. “Anybody can write code in their backyard, and some of this code can become applications that have an impact on people’s lives.”
And although there have been numerous conference panels and discussions surrounding the ethics of affectively-aware AI in recent years, little action has transpired from these talks, he says. “There hasn’t been much progress towards a formal, guiding framework.”
Ong, however, felt it was time for things to change. And so in 2021, after years of pondering over and researching the issue, he proposed a set of guidelines to help people navigate the ethical consequences of affectively-aware AI. He published this framework in July and presented it two months later at the 9th International Conference on Affective Computing & Intelligent Interaction (ACII), winning the award for Best Paper.
“I think a lot of people really appreciated the paper,” reflects Ong. “It also helped me connect to researchers in Microsoft, Google, and other companies, who are also thinking deeply about these issues. It was heartening to see that people recognised the need for such guidelines and the importance of having these conversations on AI ethics.”
Provable beneficence
As Ong sat down to begin work on his guidelines, one thing was clear in his mind: he wanted it to be “more practical in daily life” rather than a “purely academic discussion about ethical principles like contractualism and utilitarianism.” The aim, he adds, was to create something that will be actionable by researchers, industry professionals, and policymakers.
To do so, Ong split his framework into two pillars, targeting the two main stakeholders — those who develop AI, and those who use it. “My framework is different because it’s the first to make this distinction,” he says. “It’s a way of separating out the responsibilities so people can take ownership.”
The first pillar, which he named Provable Beneficence, is centered around the notion that AI developers are responsible for ensuring that the technology they develop is effective and can make credible predictions. Furthermore, the benefits must outweigh the costs to those who use it.
In order to achieve this, developers have to make sure their machine learning models are scientifically valid. For instance, developers should be aware that facial expressions do not equate to emotion, and that the context in which emotions arise are crucial. It’s also important, Ong says, that an independent third party audits the AI developed in order to verify its validity.
Additionally, developers have to ensure that the data used to train their models are sufficiently representative of various groups of people. If not, the resulting model might turn out biased and will not generalise to different groups of people. A model trained mainly on data points obtained from Caucasian people, for example, cannot be counted on to generate reliable predictions for an Asian population. Likewise, a model that used only young university students as samples cannot be extrapolated to the elderly.
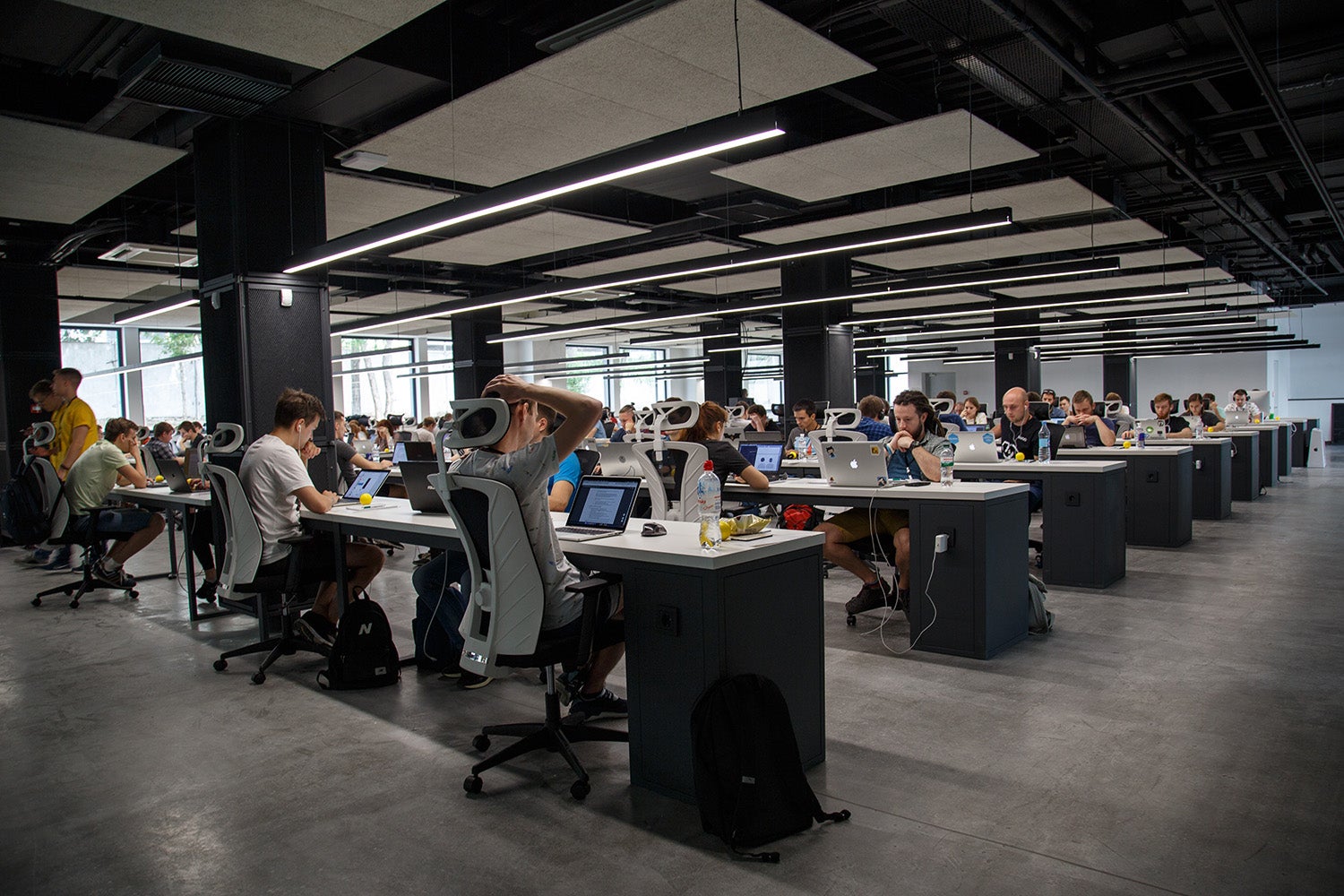
“There’s a lot of variability in data, especially for emotions,” says Ong. Most datasets contain between six to eight different types of emotions — with the common ones being anger, disgust, fear, happiness, sadness, and surprise. Including more data points, and from a wider swathe of the population (such as underrepresented or vulnerable groups), can lead to noisier datasets, he admits. “But developers must be willing to accept more variance in their data in order to more accurately capture the vast heterogeneity of human emotional experience and expression.”
Lastly, for AI to have provable beneficence, companies must be transparent about how they created their models. Ong suggests having datasheets that include information such as how the data was collected and who it was collected from. Firms can also provide examples of the technology’s intended use-cases.
Responsible stewardship
The guidelines’ second pillar, Responsible Stewardship, is aimed at those who deploy the technology. A key notion of this involves using AI only for its stated, pre-specified purpose. For instance, a bank may wish to request consent to scan their customers’ faces to analyse their emotions when customers visit a branch, with the ultimate aim of improving customer service. But if the bank later decides to use the emotional information collected to predict a customer’s credit-worthiness — an aim that customers did not give consent to and which may also not be scientifically valid — that would be an irresponsible use of the data, says Ong. Using the same data for different purposes, sometimes called ‘function creep,’ is a slippery slope that may lead to unethical applications of the data, and companies need to consciously put in place policies to avoid this.
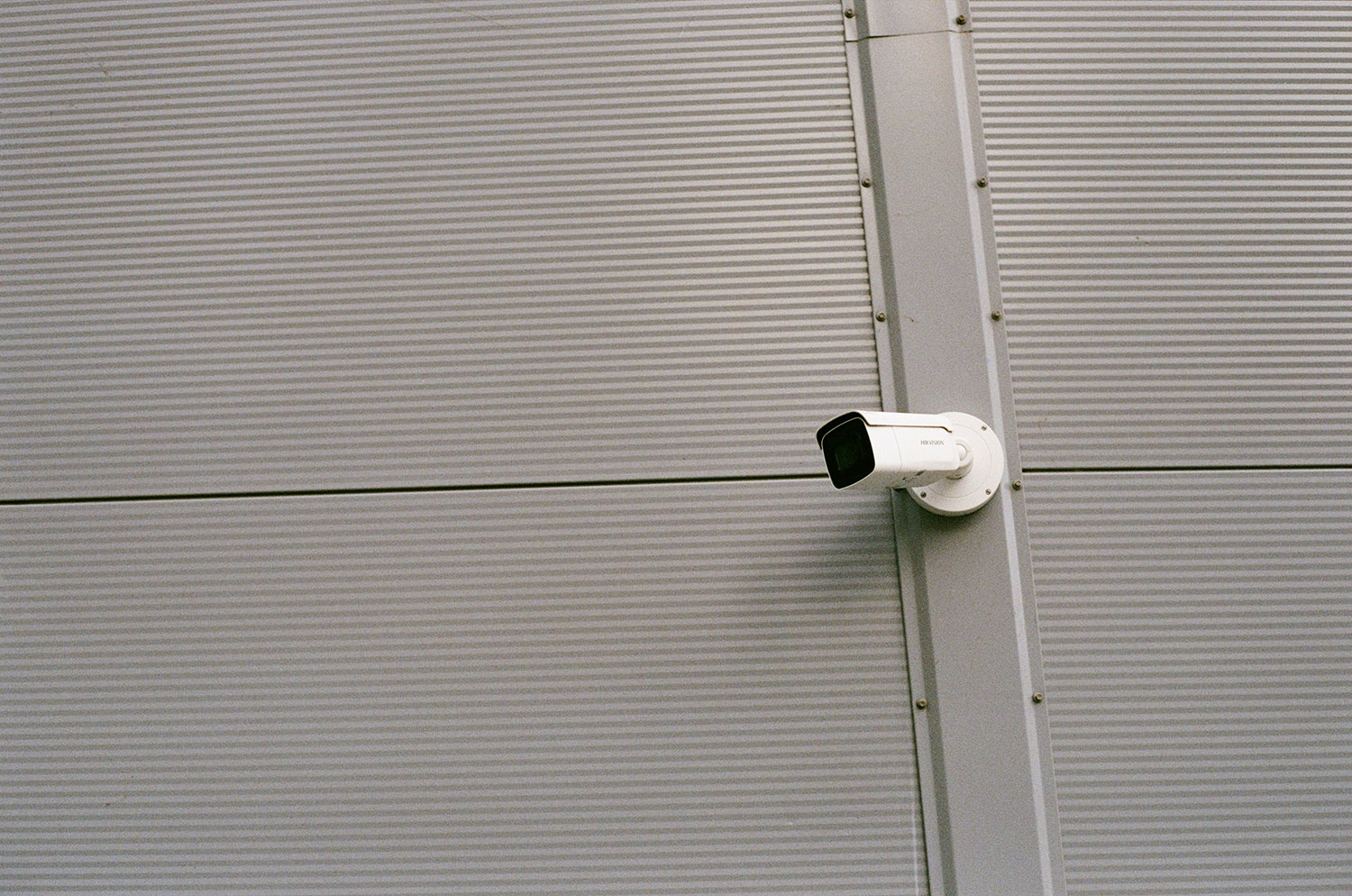
Additionally, those in charge of deploying the AI must ensure its intended effects match the actual outcomes. “There should be no unintended negative side-effects,” he says. This is especially important when it comes to vulnerable populations. Take the example of a school using ‘engagement detection’ AI tools to improve the learning material offered. If instead, teachers begin using the tools to call out inattentive students, that might unfairly penalise those with attention learning disorders, says Ong.
Responsible stewardship also encompasses the notions of protecting the privacy of those whose emotions are collected and seeking their permission to use their derived data. And finally, it involves ensuring that the AI is used properly by employees who know how to interpret its recommendations and troubleshoot issues, in order to uphold the quality of services offered.
“Operators should designate regular internal oversight to examine why the data is being collected, as well as how the data is being collected and stored,” says Ong. “It’s helpful for organisations to appoint a “devil’s advocate”, or in this case a “customer’s advocate”, to ask questions such as: ‘Do we really need to collect this data? Will the customer be comfortable with this?’”
In general, the guidelines make for a good starting point to begin debating the ethics of affectively-aware AI, but the biggest challenge lies in convincing companies to adopt them. “Right now, there’s no incentive for them to do so, or for them to restructure so that they prioritise some of these ethical values,” admits Ong. “But it’s important that we encourage more of this kind of thinking. And it is encouraging to see some large technology companies start lead by example.”
He adds: “We will not achieve ethical affectively-aware AI overnight, but it’s a shared responsibility that we have to collectively strive for.”
Paper: An Ethical Framework for Guiding the Development of Affectively-Aware Artificial Intelligence