01 December 2021 – Computer Science PhD student Ma Yunshan, Kwan Im Thong Hood Cho Temple Chair Professor Chua Tat Seng and their collaborators have won the Best Student Paper Award at the ACM International Conference on Multimedia Retrieval (ICMR) 2021.
The conference is a premier scientific conference for multimedia retrieval and was held online from 16 – 19 November this year.
Ma, Prof Chua, Ding Yujuan, a visiting research intern at NUS Computing, and Professor Wong Wai Keung from the Institute of Textile and Clothing at Hong Kong Polytechnic University, presented their paper on Leveraging Two Types of Global Graph for Sequential Fashion Recommendation at the conference.
In the paper, the team proposed a more efficient and effective approach to develop a sequential fashion recommendation model. This refers to a fashion recommender system capable of recommending items that take into consideration a shopper’s sequential actions when shopping online.
Recommender systems used in online shopping platforms – an important part of the fashion industry – can improve users’ shopping experience and give retailers’ a boost in sales volume by directing users to their preferred product items.
However, building an effective fashion recommender system is not an easy feat, explained Ma on behalf of the team. They had to consider two important factors when developing a better system: shoppers’ long-term and short-term fashion preferences.
Long-term preferences refer to users’ individual fashion tastes (for example, a preference for edgy streetwear over vintage clothes), while short-term preferences refer to the purchases that may sequentially correlate to the items a shopper picks in that moment (for example, buying a matching skirt and blouse set, or looking for shirts that are currently in trend).
As such, Prof Chua and his team proposed using two types of global graphs – the user-item interaction graph and item-item transition graph – to capture both long-term and short-term preferences in shoppers.
“In addition, we took advantage of an improved GNN model, named LightGCN, as the graph learning kernel to learn both user and item representations,” said Ma. “We conducted experiments on two public datasets from Alibaba iFashion and Amazon Fashion, and the experimental results in our paper demonstrate the effectiveness of our method.”
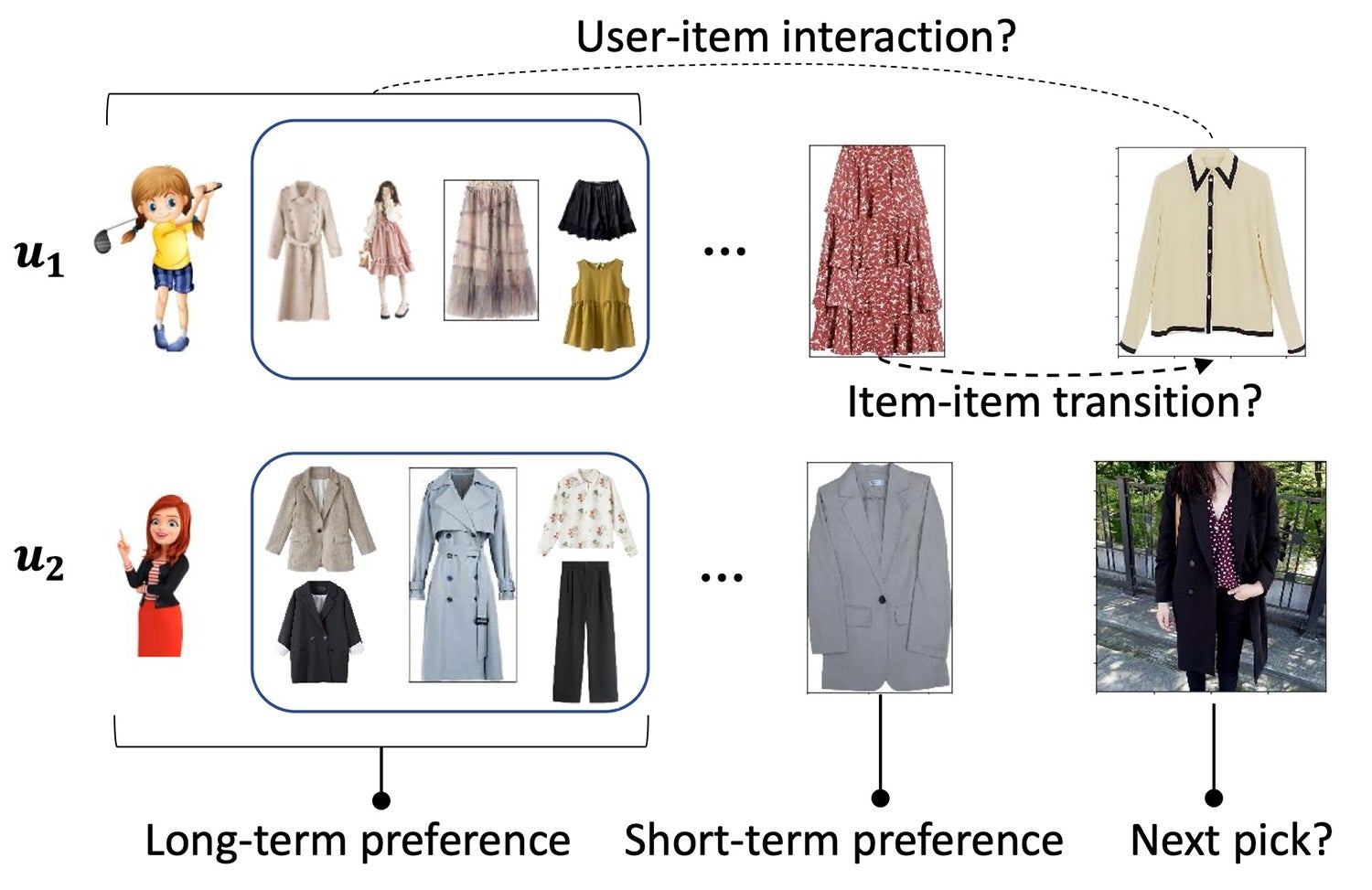
Said Prof Chua, who is also Director of the NUS-Tsinghua Extreme Search Center (NExT++), of the team’s win: “This paper rightly observes that a person’s fashion taste is influenced by a myriad of factors. It is not only affected by individual tastes, which is a long term preference, but because of the need to be accepted by the community, it is also affected by global taste – the choice of other users and trends in the fashion industry. Our work transforms this observation into a graph-neural-network (GNN) formulation based on two types of graphs to capture these two types of preferences. The process of transforming observations to solutions based on the latest graph-based formulation is a key contribution of this paper. It is comprehensive, and makes our paper stand out against other related projects.”
For the paper, the researchers relied on their different areas of expertise to develop a cohesive solution.
“The challenging aspect is in coming up with a solution that incorporates all factors in a seamless and effective manner, and demonstrating that the solution works on large-scale real-world datasets. This work incorporates fashion knowledge from Yujuan and Wai Keung, industry knowledge from ViSenze, and state-of-the-art techniques from our group,” Prof Chua explained. ViSenze is an AI-powered product search software used by large retail stores globally, and was co-founded by Prof Chua.
“We had help from ViSenze in contributing their industry knowledge and market insights, as well as research support from other researchers in our group, especially on graph neural network,” he said.