Most pundits gazing into the crystal ball will likely shout two words in their prediction of healthcare’s future: precision medicine. Increasingly, there is growing recognition that tailoring treatments based on an individual’s lifestyle, genes, and environmental factors can yield much improved outcomes.
Majority of therapy options today adopt a one-size-fits-all approach, but this is hardly optimal given the vast differences that exist from patient to patient, despite them having the same disease. These differences can significantly impact how the disease develops and progresses in each patient, as well as how responsive they are to various drugs. Measuring these factors and using them to slot patients into different sub-populations can have a significant impact on their treatment outcomes.
“When we have a particular person’s data, such as their molecular or genetic information, we may be able to see what subtype or group he or she belongs to. Then we can potentially make things more personalised,” says Vaibhav Rajan, an assistant professor from NUS Computing, who studies healthcare informatics. Doctors can use this information to determine treatment strategies. “They may know that for this group, these drugs work well and for a different group, another set of drugs work better,” he says.
Today’s breast cancer treatments are perhaps the furthest we have come to realising the goal of precision medicine. There are four to five well-accepted breast cancer subtypes. Patients have their genes analysed to determine which subtype they have and doctors devise a treatment strategy based on the results. A woman who has triple-negative breast cancer, for example, is likely to benefit from chemotherapy. In contrast, a woman with luminal A breast cancer, a different subtype, is likely to be put on hormone therapy in addition to chemotherapy.
Clustering with copulas
Determining what the subgroups of a particular disease is typically begins with studying the biomedical data of patients. The discovered groups are called subtypes. Patient subtyping is especially useful when it comes to tackling diseases like cancer. Not only does a multitude of factors affect how and where a tumour grows, but cancer itself is ever evolving. “It’s not a static thing,” says Rajan. “The cancer tissue can also have different clusters that can evolve as time goes by.”
To carry out the kind of patient subtyping Rajan describes, researchers use a technique called clustering. This involves the use of computer algorithms to statistically analyse large amounts of biomedical data and identify patterns within them — in other words, clustering patients into subtypes based on the characteristics they share.
There are many different kinds of clustering algorithms. Model-based clustering algorithms assume an underlying statistical model (e.g. Gaussian mixture distribution) for the data and then attempt to infer model parameters such as the distribution mean from the data. These algorithms are commonly used because of the interpretability they offer. For instance, after fitting the model, one can “see” how different clinical variables are correlated within each cluster. This allows us to understand the subtypes in greater detail and characterise them based on the statistics of the clinical variables.
However, most standard model-based clustering algorithms make the simplifying assumption that the variables in question must have the same type of data distribution — in other words, that they must all be Gaussian, exponential, and so on. “But this may restrict their modeling flexibility and deteriorates their clustering performance,” says Rajan.
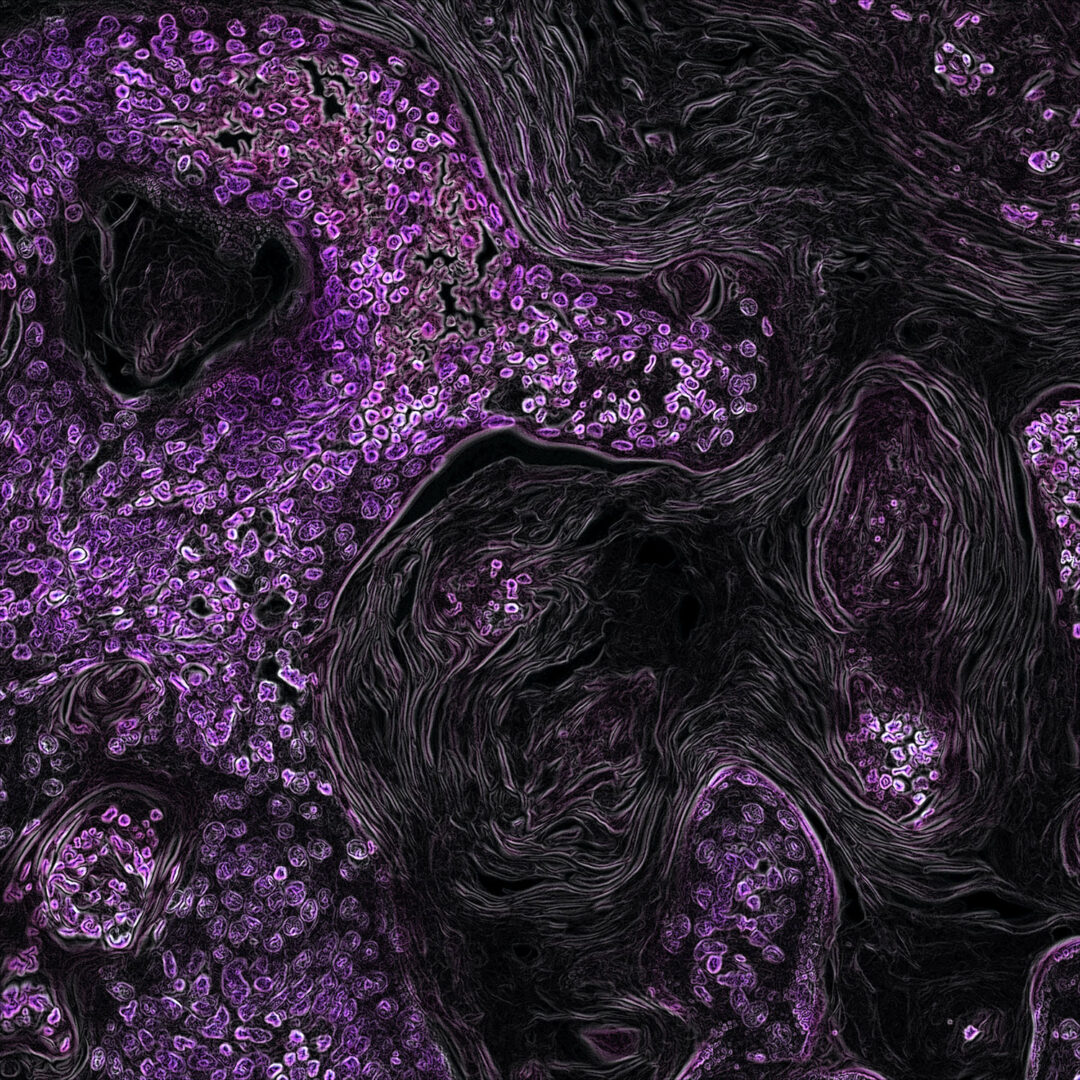
To overcome this limitation, Rajan and his group have been exploring statistical tools called copulas. Meaning “tie” or “link” in Latin, copulas are used to describe the dependence between random variables. Using copulas allow greater flexibility in modeling the data because it enables distinct assumptions to be made about the different distributions of different clinical variables.
In addition, copulas enable us to model complex, non-linear correlations in the data that many other simpler models do not. For instance, consider two variables that are highly correlated at their lower values but uncorrelated in higher values. Copulas can be effectively used in such cases to model the strength and type of correlations. Thus, copulas are highly flexible statistical tools that are useful for modeling complex clinical and genomic data.
However, copula-based mixture models could not be used with modern high-dimensional data, which can contain up to thousands of clinical variables. This is due to technical limitations that adversely affect their accuracy and scalability.
To overcome this problem, Rajan — together with his PhD student Siva Rajesh Kasa and collaborator Sakyajit Bhattacharya from the TCS Innovations Labs in India — worked to develop a new inference algorithm for a copula-based clustering model that in turn can be used effectively to find subtypes from high-dimensional clinical data. In 2019, they published a paper in the journal Bioinformatics announcing their new technique: HD-GMCM or high dimensional-Gaussian mixture copula model.
“We have a specific way of finding intrinsic patterns in the data and we are able to do it at high dimensions, which is important for a lot of biomedical datasets, especially in cancer,” says Rajan. “Our model is set up in such a way that it reduces the number of parameters to be inferred, without adversely affecting the clustering accuracy.”
“To our knowledge, nobody has ever used copulas for patient subtyping before,” he says.
Helping real patients
To test how robust GMCM is, Rajan and the team applied it to a number of real gene-expression datasets, as well as a simulation study. They also used it in a case study to characterise lung cancer patients into different subtypes, and examined the survival rates of those in each cluster, and the pair-wise correlations among survival rate, smoking and age.
In all instances, the new method outperformed state-of-the-art clustering methods. Not only did it lead to better clustering, but also potential subtypes that were clinically meaningful. “For the case study, there was a statistically significant difference in the survival probability across the two clusters that the algorithm discovered,” says Rajan. “This implies that the clusters HD-GMCM found has potential clinical significance.”
His team is now working to improve the algorithm further. They are also working closely with oncologists in Singapore to develop more personalised treatment strategies.
“What I would like to have, and I’m trying to set it up in my group, is the entire research pipeline, says Rajan. “From the development of new models and algorithms to innovative, practically useful healthcare applications, and finally, actual implementations that may be used in hospitals.”
Paper: Gaussian mixture copulas for high-dimensional clustering and dependency-based subtyping