Today’s virtual assistants and smart devices have come a long way. They can tell you if you’re running low on milk, what the weather will be like tomorrow, or change the TV channel without you having to lift a finger. But if researcher Desmond Ong has his way, the Google Homes and Alexas of the future might be able to add another attribute to their already impressive resume — emotional intelligence.
One day, he imagines, such artificial intelligence (AI) systems might be able to look at your calendar and see that it’s been packed with back-to-back meetings. Predicting that this will make you arrive home irritable and too tired to cook, your home assistant might put on some soothing music and order in your favourite comfort food, creating the perfect environment for you to relax and unwind after a hard day’s work.
But before we get to that stage, machines need to learn how to understand emotions the way humans do, says Ong, an assistant professor of Information Systems and Analytics at NUS Computing. “This will become even more important in the future as our relationship with AI becomes more personal — when we have social robots in our homes, or a personal AI on our phones.”
Apart from enhancing our home lives and creating a more personalised experience, emotion-comprehending AIs could make for more effective teaching assistants and robot nurses, or help people with autism better recognise others’ emotions, among other applications.
For AI to successfully integrate into our lives, they need to be able to “understand” our thoughts and emotions, so that they can respond accordingly. Children intuitively learn how to do this by the time they are toddlers, but training a machine to be similarly skilled is a complicated task.
“We just don’t have that capability right now. The technology we have is very shallow,” says Ong, a computational cognitive psychologist. While today’s machine learning models have advanced to the stage of being reasonably good at pattern recognition, they are a long way off from performing true reasoning, especially about people. “An AI might be able to detect a smile on your face and thus classify you as feeling happy, but it cannot infer what made you happy, it doesn’t understand what it means to be happy, and it doesn’t know what happy people will do next,” says Ong.
“Deep learning is currently considered state-of-the-art, but I don’t think that deep learning alone will give us a solution to human-like understanding of emotions,” he elaborates. “It’s not going to help us reach the next stage, which is ‘How do I take that emotion I’ve detected and use that to predict what’s going to happen next?’”
“We’re going to need more sophisticated technology in order to address this,” says Ong.
Throwing psychology into the mix
One reason why it is so difficult to train machines to be empathetic is because human emotions are incredibly complex. Smiles don’t always signal happiness — research has shown there are 19 types of smiles, only six of which are associated with having a good time — and tears are shed out of sadness and pain, but also due to intense joy.
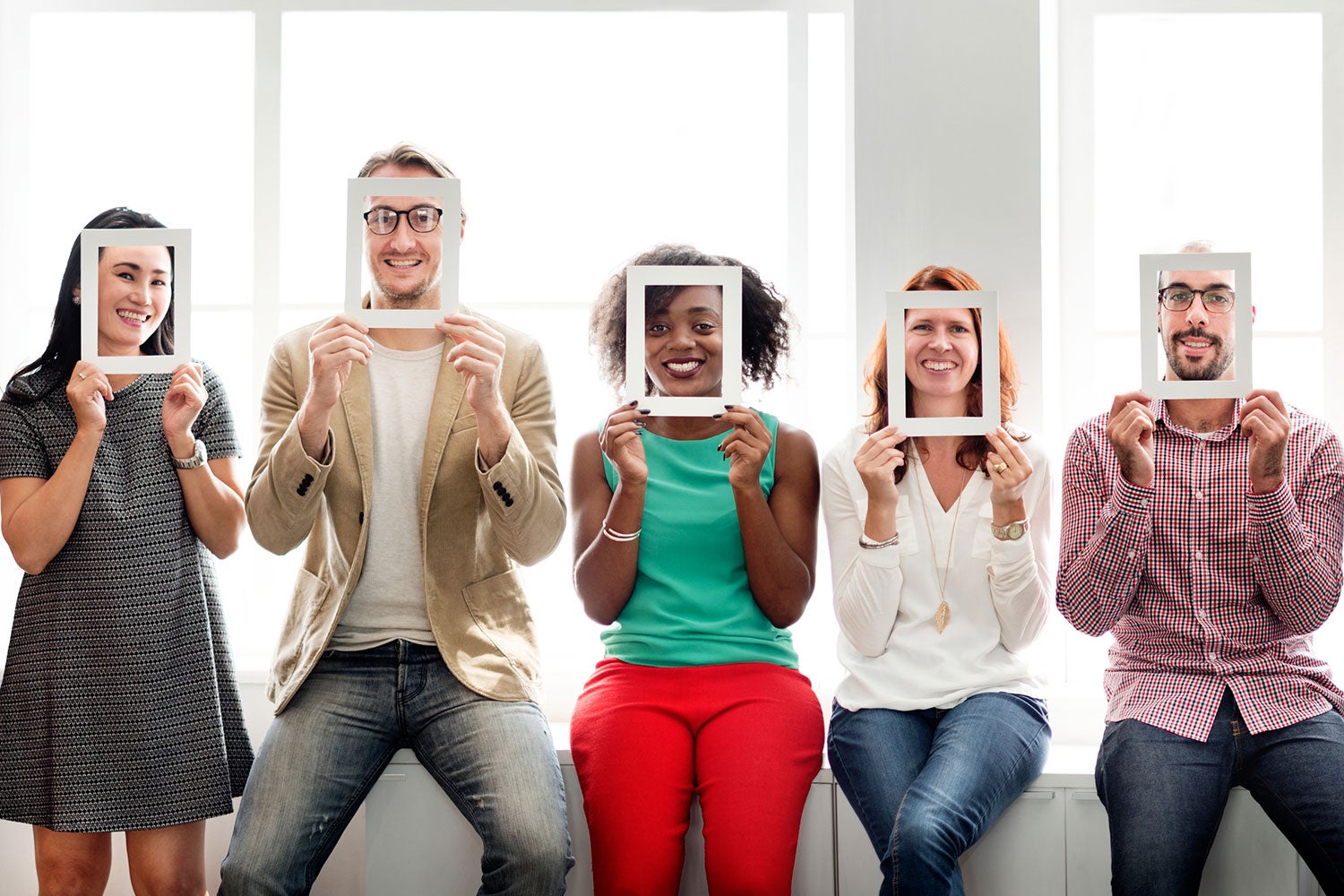
“Emotions don’t just result from simple X-causes-Y scenarios. Instead, they are incredibly rich, multi-layered psychological processes which makes capturing and representing them in computer models one of the biggest challenges,” says Ong.
The key to succeeding, he believes, is integrating psychology with computer science. It is a unique approach — one that Ong is incredibly suited to do. Originally trained in physics and economics, he pivoted to psychology for his PhD. The turning point came when Ong was working on his undergraduate thesis about “paying it forward”, in which a person receives a kind deed and in return does something nice for another person, rather than simply accepting or repaying the original benefactor.
“‘To this day, I haven’t found an economic explanation for why people pay it forward,” says Ong. “It is often emotions like gratitude that drive these decisions — that insight led me to study emotions.”
“So a lot of my work has been taking what we know from psychology and trying to distill that into something a computer can understand,” he says.
A key component of such affective computing is grasping the situational context surrounding a person’s emotions — similar to what people do when interacting with others. To that end, Ong and his collaborators propose taking a probabilistic programming approach. The technique, which combines deep learning with probabilistic modeling, involves asking questions such as “Was this event desirable?” and “Was it expected?”, to better understand context.
“These are called appraisals,” Ong explains. “We know from decades of psychological theory that when people experience events like winning a prize or receiving negative news, they evaluate, or appraise, what that event means to them personally. These appraisals lead people to feel happy or disappointed.”
As it turns out, only a few of these appraisal features are important to understanding most of the context surrounding an emotional response. “So, the hope is that we try to build these appraisals into a model, and then the computation to figure out what emotion this person is feeling becomes a lot simpler,” he says.
“Deep probabilistic programming is a novel approach where you specify what you know of the causal structure from theory, and then you fill in the rest that you don’t know by learning from the data, using deep learning techniques,” he says.
A myriad of emotions
In addition to innovating probabilistic programming approaches, Ong and his collaborators have worked to tackle another challenge in affective computing: that people can experience a myriad of emotions in a short span of time.
You may, for example, wake up feeling happy that you’ve had a good night’s sleep, but then feel sad about leaving your warm bed to go to work. You glance at your phone and irritation registers when you see that your boss has already texted you with instructions for the day. As you get dressed, you listen to the news and can’t help feeling worried about the pandemic. It’s a mini roller-coaster of emotions, and all before you even have your first cup of coffee.
“Everyday emotional experiences are so dynamic,” says Ong, “and it’s important to model how emotions arise in this naturalistic context.” This is especially useful for creating social robots that can engage in conversations with a user, to enable them to assess the continuous stream of sensor data collected so they may adjust their responses accordingly.
But training AI to perform such time-series emotion recognition requires data that is difficult to acquire and expensive to construct. As a result, few high-quality datasets exist for researchers to use. In response, Ong and his collaborators created the Stanford Emotional Narratives Dataset, an annotated set of videos comprising close to 200 clips of 49 participants narrating personally-meaningful positive and negative life events, which he hopes will power more research into AI that can better ‘understand’ naturalistic emotions.
In the long term, Ong hopes that his work to develop emotion-understanding AI will help improve people’s lives, such as in areas of education and mental health. “People are great at a lot of things, but an AI is great at other things,” he says. “We should be focusing our efforts on developing AI technologies that complement people and help individuals to maximise their potential.”
Papers:
Applying Probabilistic Programming to Affective Computing
Modeling Emotion in Complex Stories: the Stanford Emotional Narratives Dataset