5 Jun 2023 — NUS Computing Assistant Professor Nakyung Kyung and collaborators won the Health Information Technology (IT) in Action award at the 13th Conference on Health IT and Analytics (CHITA) for their paper on the application of machine-learning techniques on a mobile healthcare platform.
CHITA is a research summit and doctoral consortium that draws over 100 scholars and thought leaders from more than 40 institutions every year. The conference champions the importance of analytics and health IT in improving individual and population health, and health system quality and performance.
Dr Kyung and her team of collaborators, Associate Professor Idris Adjerid at Virginia Tech, and Assistant Professors Sriram Somanchi and Yoonseock Son at the University of Notre Dame, presented their paper under the Novel Techniques track at the conference on 5th May 2023.
Their paper, “Machine Learning-Based Precision Nudging: Evidence from a Randomised Field Experiment on a Mobile Healthcare Platform,” examines the effectiveness of using machine learning techniques on a fitness application to get users to walk more.
Previous studies have found that using a one-size-fits-all approach and overlooking individual heterogeneity in intervention design (nudges) have not been very effective in nudging the user to action. Realising the need for individual heterogeneity, the team developed “the next generation of nudging—Nudging 2.0,” which employs Machine Learning-based Nudging (ML-Nudging) to deliver more personalised and contextualised nudges.
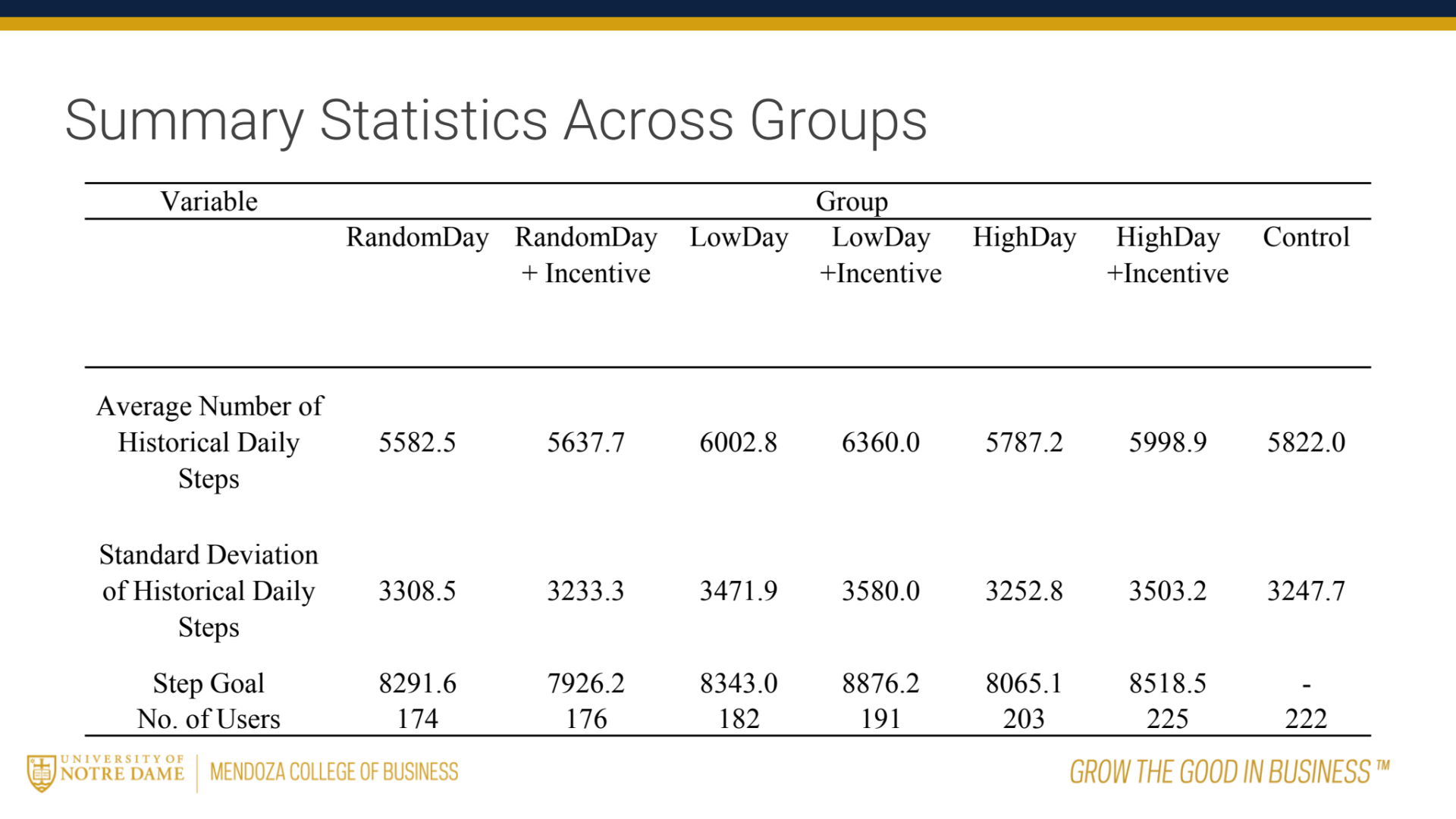
To identify the effect of ML-Nudging and the heterogeneous impact of incentives, the team carried out experiments with temporal and economic dimensions (incentive-driven). Overall results showed that users assigned to ML-Nudging groups walked more non-ML-Nudging groups. They also observed that users who were prompted with ML-Nudging continued to walk on days when they were not prompted.
The team’s findings are astounding as most literature put considerable effort into designing the intervention while only limited studies considered individual heterogeneity. Doing so can also improve the cost-effectiveness of businesses.
Dr Kyung elaborates: “Our results indicate that inattentive offerings of incentives may have no significant impact on the actual performance of individuals. Instead, providing them at the right moment is the key to maximising both the efficiency and effectiveness of incentives. Considering the availability of over 350,000 health and fitness apps, inducing effective physical activity is pivotal for their success. In this context, our strategy can aid businesses in boosting their revenue by offering services that cater to customer satisfaction.”
Taking into account individual heterogeneity also meant being mindful of other potential setbacks that can happen in a real-world setting. For example, some users may be more resistant to intervention, so the team had to carefully set the intervention timing and frequency of nudges to minimise user resistance.
Carrying out the experiments was an uphill task that requires extensive effort from the team and staff too. “We divided the tasks and assigned responsibilities to ensure efficiency. While machine learning technology was the core of this project, I learned that it was the effort and cooperation of the team members that ultimately made it possible to apply the technology to reality,” reflected Dr Kyung.